Introduction
Models of the diffusion of innovations have a long tradition in economic research. Early empirical evidence (Ryan & Gross 1943) suggested that the diffusion of new products in a market follows an S-shaped development over time. Starting from the seminal paper of Bass (1969) which introduces a still widely used differential equation growth model for consumer durables, numerous models were developed which are able to reproduce observed adoption curves (Mahajan, Muller, & Bass 1990). The temporal dimension of a diffusion process is supplemented by a spatial dimension of innovation spread. In principle, combined spatiotemporal dynamics of innovation diffusion can be described by mathematical equations (Mahajan & Peterson 1979). Still, such equation-based approaches operate on a regional market level and can barely account for the empirically found heterogeneity of customers for instance in terms of the classical adopter types introduced by Rogers (1962). Likewise, equation-based approaches do not represent the dynamic inter-individual social processes impacting adoption decisions.
Agent-based models (ABM) of innovation diffusion are successful in bridging the outlined gap between the micro-level of interacting, heterogeneous customers and the macro-level of market developments (see Kiesling et al. 2012 for an up-to-date and comprehensive overview). While the majority of the models in the field are abstract in nature and aim at theoretical insights, empirically-grounded, policy-relevant ABM have experienced growing attention in recent years. The latter applications of ABM aim to provide policy makers with a virtual test lab of “citizen agents” which behave in a narrowly-defined real-world application context sufficiently similar to the real citizens in a target population. Goals of such models are to provide case-specific candidate explanations for observed collective behavioural dynamics or to derive policy-relevant recommendations for instance concerning policy measures to accelerate the diffusion of certain innovative behaviours or practices.
Recent application domains include water saving innovations (Schwarz & Ernst 2009), health care provision (Dunn & Gallego 2010), diffusion of smart metering (Zhang & Nuttall 2011), sustainable food consumption (Bravo et al. 2013), diffusion of heating systems (Sopha, Klöckner, & Hertwich 2013), installation of agricultural biogas plants (Sorda, Sunak, & Madlener 2013), homeowners’ insulation activity (Friege, Holtz, & Chappin 2016), and climate change adaptation (Krebs 2016; Krebs, Holzhauer, & Ernst 2013). Common to the stated models is their grounding in rich empirical data sets for very specific application domains and the representation of a detailed household typology (as agent types) based on empirical data from the literature or from specific household surveys. Depending on the particular purpose of the model and the demands of the application context, approaches differ in the degree to which real-world geography is considered: Some do not require geography (e.g. Bravo et al. 2013) or consider abstract geographical proxies (e.g. Friege et al. 2016; Zhang & Nuttall 2011). Others are empirically grounded in case-specific micro data on some real-world geographical scale which is explicitly represented in the simulations (Krebs 2016; Krebs, Holzhauer, & Ernst 2013; Schwarz & Ernst 2009; Sopha, Klöckner, & Hertwich 2013; Sorda, Sunak, & Madlener 2013). The realistic spatial representations included in the latter class of ABMs play a key role for a number of reasons (Rai & Henry 2016, p. 560–560). In particular they allow the models to account for spatially-resolved social interaction between agents. Furthermore, in policy contexts the design of local mobilization measures or infrastructure management and planning can be supported by spatially explicit simulation studies.
In the outlined application contexts, the main argument of the method of ABM concerns the validity of the model structure for instance in terms of a theoretically-founded representation of micro-level individual decision processes or inter-individual social dynamics. On the other hand, the models deliver very rich and detailed simulation results of future scenarios which can for instance be presented in the form of maps of certain model indicators or time series of aggregated model outputs. In stakeholder dialog, the credibility of the simulation assessments increases with the degree to which statements on model validity can be (and are) made. The diversity of approaches and methods as well as the challenges of ABM output analysis was recently reviewed (Lee et al. 2015). In the present paper, we provide a typical instance of such an exercise for the case of innovation diffusion.
The model presented and analysed in the paper builds on previous work from the domain of spatially explicit agent-based policy models. The ABM draws on empirical results from the SPREAD project (Scenarios of Perception and Reaction to Adaptation) on the adoption of green electricity by German households. In line with the recommendations of environmental NGOs and other independent organisations (NaturFreunde Deutschlands 2016; Robin Wood 2013; Stiftung Warentest 2011) the project defines “green” electricity strictly as electricity which is exclusively produced from provider-owned renewable resources like photovoltaics, wind or hydropower. Furthermore, green energy suppliers continuously invest their revenues in the extension of their stock of renewable energy plants and operate independently from the large traditional suppliers of grey energy from nuclear, coal or gas-fired plants. Therefore, by switching to green electricity, households’ electricity tariff choice can contribute to the transition of the energy system towards renewables.
The ABM’s empirical point of departure is given by a historical trace of the adoption of green electricity by German households from January 2005 to December 2011. The dataset stems from one of the big German suppliers of green energy and comprises customer numbers for each of the over 8000 postcode areas in Germany on temporal resolution of months starting from January 2005 to December 2011 (Elektrizitätswerke Schönau (EWS), personal communication 2013). The EWS customer data show distinct spatiotemporal patterns: Initially customers are almost exclusively located in the extreme southwest of Germany, close to the EWS headquarters. Over time, two spatial diffusion patterns become visible. In the southwest of Germany a contagious area is observable which grows starting from the small area where the initial customers were located. Furthermore, adoption appears to leap out of the southwest area to larger cities in other parts of the country and only later to diffuse to rather rural areas around those cities. The spatial pattern of high increase in customer numbers is complemented by an area with very low EWS market share, particularly in eastern Germany. It the goal of the ABM presented in this paper is to provide a psychologically-founded, dynamical reconstruction of the EWS customer development. To achieve this goal, the underlying inter- and intra-individual processes of household electricity choice have to be further specified.
Changing a household’s electricity tariff requires that an individual gathers knowledge on alternative suppliers, compares different tariffs and selects a new tariff that best fits the household’s particular preferences. Because of the cognitive effort involved in this decision process it is common that households tend to stick with their current electricity supplier and only check their contracts from time to time. In addition, external events like for instance significant price changes may trigger a reconsideration of tariff choice.
When individuals feel the need to reassess their household’s electricity choice they do so by deliberating on existing alternative electricity tariff options. This process of deliberation may be conceptualised as a mental simulation processes which allows the individual to assess the expected outcome of choosing one or the other alternative. The underlying concept from behavioural theory is goal-directed, multi-attribute utility decision-making (Baron 2007). In the approach, the evaluation of an individual’s options of behaviour is governed by the subjective preference of the individual for specific outcomes. Preferences reflect the sensitivity of an individual to particular goals, i.e., which goals are given priority over others.
The goals that guide the choice between green and grey electricity tariffs are indicated by research results on environmental behaviour in general (Lindenberg & Steg 2007) and by literature on behaviour in commons situations (Ernst & Spada 1993; Messick & Brewer 1983): Cost minimisation, ecological orientation, and social conformity. The first two goal dimensions are clearly bound to specific outcomes. Selecting a green electricity tariff satisfies the ecology goal more than selecting grey electricity and the cheaper the electricity tariff the better it is from the perspective of the economic goal. The social conformity goal covers the influence of “injunctive social norms” (Cialdini, Kallgren, & Reno 1991) i.e. the perceived normative social pressure to perform or not to perform a given behaviour. In the domain of energy conservation behaviour the importance of such peer effects is indicated by empirical results which found that behaviour is highly correlated between network peers (Göckeritz et al. 2010; Nolan et al. 2008). In the context of innovation diffusion a strong goal of social conformity will hinder the adoption of some innovation by an individual as long as the adopters in the personal network are in the minority. When the number of innovation adopters in a personal network increases towards a majority, the individual will experience social benefit from adopting too.
The interplay between social and non-social utility expectations in the cognitive process of deciding between competing options of behaviour is formalised by the Theory of Planned Behaviour (Ajzen 1991; Ajzen 1985) which is operationally grounded in utility calculations. In the domain of customer behaviour and in environmental contexts the theory is frequently used as a grounding of agent decision-making (Krebs 2016; Schwarz & Ernst 2009; Sopha, Klöckner, & Hertwich 2013; Sorda, Sunak, & Madlener 2013; Zhang & Nuttall 2011). The theory’s particular suitability is outlined elsewhere (Zhang & Nuttall 2011, p. 173–173). The theory states that the strength of the intention of an individual to perform a behaviour (to select a particular type of electricity) depends on subjective weightings between personal attitudinal factors (the economic and ecological goals) and social normative factors (the social conformity goal). In particular, empirically-found household types differ in the weights they attribute to the stated factors during deliberative decision-making. In the ABM presented and analysed in this paper, agent types represent empirical household types according to the Sinus-Milieus® for Germany (Sinus Sociovision GmbH 2007). Depending on their type agents differ in their decision preferences regarding the outlined goals. The behavioural target variable of an agent is the selected electricity tariff. Each agent represents a number of households of the respective type. Furthermore, each agent is characterised by its position in geographical space and its position in an artificial social network.
The research presented in the paper makes three contributions. In Section 2 we report how the theoretical and empirical concepts outlined above are carried forward to an ABM of the diffusion of green electricity in Germany. An early version of the model was described elsewhere (Ernst & Briegel 2016). Here, we present the first full ODD description of the model. The second contribution of the paper is to develop and to illustrate a systematic validation and calibration procedure for the ABM (Section 3.1 and Section 3.2). To do so, we challenge simulation results with existing historical data thus focussing on retrodictive validity (Amblard, Bommel, & Rouchier 2007; Ormerod & Rosewell 2009; Troitzsch 2004). As a third contribution, we present and discuss simulation results from the calibrated model which illustrate the possible insights into different diffusion patterns generated by spatially explicit ABMs (Section 3.3). Section 4 summarises and discusses the reported research.
Modelling the market share of a green energy supplier in Germany
The model description follows the ODD (Overview, Design concepts, Details) protocol (Grimm et al. 2010; Grimm et al. 2006). A more detailed description of the ABM’s psychological underpinning is found elsewhere (Ernst & Briegel 2016).
Purpose
The purpose of the presented ABM is the dynamical reconstruction of the diffusion of green electricity adoption by German households from January 2005 and to December 2011. More specifically, the goal of the presented ABM is to propose agent-based candidate explanations of the temporal development of the green electricity market in general and of the spatiotemporal patterns found in the historical customer development of EWS in particular.
Entities, state variables and scales
In the model, agents represent households in Germany. Agents are characterised by the following state variables (see Table 1).
Name | Description | Domain | Static? |
location | Spatial position in the map of Germany | GIS coordinates | Y |
milieuGroup | Household’s affiliation to a Sinus-Milieu® group (Sinus Sociovision GmbH 2007) | {Post-materialist, Leading, Traditional, Mainstream, Hedonistic} | Y |
wecon | Preference for cost minimisation | [0; 1] | Y |
wsoc | Preference for social conformity | [0; 1] | Y |
wecol | Preference for ecological aspects | [0; 1] | Y |
pdelib | Baseline probability to deliberate on electricity supplier | [0; 1] | Y |
sgreen | Share of customers of green electricity from EWS or from other suppliers among the alteri in the agent’s social network | [0; 1] | N |
sgrey | Share of customers of grey electricity among the alteri in the agent’s social network | [0; 1] | N |
awareOfEWS | Indicates if an agent knows EWS as an electricity supplier | {true, false} | N |
electricitySupplier | The presently selected electricity supplier of an agent. | {Grey, Green from EWS, Green from another supplier} | N |
The second type of entity in the model is the environment which provides global price information on the electricity tariffs. The entity has the following state variables (see Table 2).
Name | Description | Domain | Static? |
rgrey,t | Price of grey electricity at month t | Ct/kWh | N |
rgreen,t | Price of green electricity at month t | Ct/kWh | N |
One time step of the model represents one month. Simulations start at January 2005 and were run for 84 months until December 2011.
Process overview and scheduling
The pseudocode of the model’s process schedule is shown below. After model initialisation, each timestep starts by updating the electricity prices in the environment according to a historical time series for the considered simulation time (see Section 2.26). Following this, each agent perceives the types of electricity selected by its network neighbours in the previous simulation month and updates its state variable sgreen accordingly. Next, each agent executes the submodel "selectElectricitySupplier" which updates (or confirms) the agent state variable electricitySupplier according to an agent’s preferences, its social network and price perceptions, and its awareness of EWS (see Section 2.27). Finally, each agent executes the submodel “communicate” which simulates information exchange on suppliers of green electricity with other agents (see Section 2.39). Through the latter process, agents can become aware of EWS which is a prerequisite of becoming customer of EWS. The pseudocode of the main simulation cycle is as follows:
The model is implemented in Repast Simphony (North et al. 2013) and uses the LARA framework (Briegel et al. 2012; Elbers et al. 2009; Holzhauer 2015).
Design concepts
The model draws on Basic principles from three main scientific theories. Social Network Theory (Wasserman & Faust 2009) is applied to connect household agents with their important peers in a network graph. The social network facilitates the simulation of social normative influences between agents regarding the adoption of green electricity. Furthermore, the network serves as an infrastructure for information exchange between agents in order to simulate the market awareness of the brand EWS. Agent decision-making is theoretically grounded in the Theory of Planned Behaviour (Ajzen 1991; Ajzen 1985) which integrates social normative perceptions of an agent with attitudinal dimensions relating to economic and ecological aspects of adopting green electricity. Finally, the model draws on the concept of sociological lifestyles (Bourdieu 1984) to structure the target population and to parameterise agents by socio-empirical data.
Emergence is found in the form of macro patterns in the diffusion of green electricity. The patterns arise from agent-level decision processes and agent interaction. Patterns comprise the temporal development of the diffusion, the social composition of the green electricity adopters and the spatiotemporal spread of the diffusion.
Adaptation occurs when an agent seeks to increase the subjective utility of its electricity tariff choice regarding the agent’s objectives. An agent’s choice of green or grey electricity changes in response to fluctuating electricity prices and in response to the electricity tariff choices of its social network peers.
Objectives comprise three goals which household agents try to satisfy when comparing between green and grey electricity tariffs. The goals consider the minimisation of electricity costs, the maximisation social conformity of electricity tariff choice and the maximisation of ecological aspects regarding the electricity choice. The satisfaction of each goal is based on agent perceptions (see Sensing) and quantified as a partial utility regarding the particular goal. The overall utility of an electricity choice is represented as a weighted sum of the partial utilities where the weights attached to the goals represent heterogeneous agent-specific preferences. During decision-making agents seek to maximise the overall utility of their electricity choice.
Learning is not considered. In particular agent goal preferences are kept constant over the simulation time.
Prediction only occurs when agents form utility expectations regarding the outcome of one or the other electricity tariff choice.
Sensing occurs when household agents perceive the electricity choices of their social network neighbours. Furthermore, household agents perceive the prices of green and grey electricity in each simulation month.
Interaction occurs through normative social influence which originates from agent perceptions of social network peer electricity choices. In addition, agents interact by exchanging information on existing suppliers of green electricity.
Stochasticity is involved during the initialisation of the agent population when assigning agents their spatial positions, during social network setup and during the initialisation of other agent state variables from empirical data. During simulation, the tendency of an agent to deliberate on its electricity supplier and the likelihood to engage in communication are modelled as random processes. Finally, stochasticity occurs when agents select communication partners.
Collectives are not considered in the model.
Observation of model outputs comprises data on all non-static agent state variables for each simulation step of the model. Results are written to a database during simulation and evaluated when simulations are finalised. For details on model indicators calculated see section 3.
Initialization
This section reports on how socio-empirical data and historical electricity market data are used to setup a representative agent population for Germany. The rationale of agent initialisation is (a) to represent typical German household types as agent types, (b) to set up an agent population representing the 40 million households in Germany with respect to geographical location, household types, social networks, household electricity tariffs and household awareness of EWS as of December 2004.
Household types as agent types
In a first step we define agent types representing typical German household types. Agent types differ with respect to their decision preferences regarding the selection of an electricity tariff (agent state variables wecon, wsoc, wecol) and with respect to the tendency to re-evaluate an existing tariff choice (agent state variable pdelib). In order to parameterise agent types we draw on the Sinus-Milieus® for Germany which are commonly used in commercial market research but also in environmental research (Gröger 2011; Krebs et al. 2013; Schwarz & Ernst 2009). The five milieu groups of the Sinus-Milieus® characterise individuals or households along the classical dimension of social status given by income and education and supplement this grouping by a second dimension that reflects value orientations such as tradition, modernisation and re-orientation. Details on the empirical characterisation of the milieus groups are summarised elsewhere (Krebs et al. 2013, pp. 316–317).
Table 3 shows the agent decision preferences for the five Sinus lifestyles considered in the simulations. The preference values are based on qualitative and quantitative data from Sinus, on expert ratings, on specific survey results (Gellrich 2016) and on data from previous models (Krebs 2016; Schwarz & Ernst 2009; Soboll et al. 2011). The rationale of setting up the table is to keep the column sum of the preference weights for each milieu group to 1 and to represent empirically-found differences between the milieu groups in the table rows. For instance, Traditionals are more cost sensitive than Post-materialists (first row) or Mainstream milieus have the highest desire for social conformity (second row).
Preference parameters by milieu group | |||||
---|---|---|---|---|---|
Post-materialist | Leading | Traditional | Mainstream | Hedonistic | |
wecon | 0.25 | 0.30 | 0.40 | 0.30 | 0.30 |
wsocn | 0.35 | 0.40 | 0.50 | 0.60 | 0.45 |
wecol | 0.40 | 0.30 | 0.10 | 0.10 | 0.25 |
In addition to their preference parameters agent types differ in the likelihood to deliberate on their electricity tariff. Deliberation entails the comparison of an agent’s present tariff choice to possible alternatives considering the agent’s subjective preferences. To parameterise the deliberation probability for the five agent types we use the Need for Cognition (NFC, Cacioppo & Petty 1982) personality variable as a proxy (see section 2.27). The NFC scale is a psychological assessment instrument that quantifies the tendency of an individual to engage in effortful cognitive activities. From empirical research on climate-friendly innovations (Gellrich 2016) we know that post-materialist and leading lifestyles exhibit higher NFC scores than traditional and mainstream while hedonistic lifestyles do not significantly differ from the other lifestyles (Gellrich 2016, p. 232–232). If we now assume that on the agent level a high NFC with respect to climate-friendly behaviour may be represented by a deliberation probability of 10% (more than once a year) and a low NFC by 5% (less than once in 18 months), we can reflect the empirical results in the lifestyle-specific probabilities according to Table 4.
Milieu group | Post-materialist | Leading | Traditional | Mainstream | Hedonistic |
pdelib | 0.1 | 0.1 | 0.05 | 0.05 | 0.075 |
Population setup
Simulations start at January 2005 and are set up with a total of over 300,000 agents representing the 40 million households in Germany. The size of the agent population ensures that the local populations of the 8000 postcode areas in Germany are sufficiently represented regarding local population density and Sinus-Milieus® composition. Initialisation utilises an upscaling method (Ernst 2014) based on fine-grained socio-demographic marketing data on the spatial distribution of Sinus-Milieus® (Microm 2015) on the resolution of postcode areas and an empirically based social network generator (Holzhauer 2016; Holzhauer, Krebs, & Ernst 2013). Accordingly, one agent in the model represents in average 125 households with respect to their residential postcode area, their Sinus lifestyle affiliation and social network characteristics. In addition, as outlined in the previous subsection, agents are assigned different preference sets and deliberation probabilities depending on their milieu group.
In the next step, we set the agents’ initial electricity choices. The rationale is to initialise the early customers of green electricity as of December 2004 using historical data of the German retail green electricity market and to set the electricity supplier of all remaining agents to “Grey”. In line with empirical results (Gellrich et al. 2016, p. 237–237) the early adopters of green electricity are selected exclusively from the agents representing Post-materialist and Hedonistic milieu groups.
We start by setting up agents having already adopted green electricity from EWS. Initialization is done on a spatial resolution of postcode areas using the empirical EWS customer dataset. Following this, we initialise agents as customers of different green electricity suppliers by a dataset on the yearly market shares of the three major suppliers of green energy in Germany from 2004 to 2013. Data comprise absolute customer numbers on the national level and stem from Greenpeace Energy (Greenpeace Energy eG 2013, p. 25–25), Lichtblick (LichtBlick SE, personal communication 2014), and Naturstrom (Naturstrom AG, personal communication 2014). Initialization of green electricity customer agents excluding EWS is done randomly, i.e. each agent not already initialised as EWS customer becomes a green electricity customer with a probability of the total market share of the three other suppliers of green energy.
Finally, the initial market awareness of EWS is set by initialising the agents’ state variable awareOfEWS. To do so, we set the state variable to true for the initial EWS customers and for their direct social network neighbours. The underlying assumption is that all initial EWS customers have already communicated on their tariff choice with their peers which therefore know about the supplier EWS. All remaining agents are initialised unaware of EWS.
Input data
The only input data stream is given by electricity market data from a historical time series from 2005 to 2011 (Verivox 2013). The data is used to update the electricity prices for green and grey electricity in the environment entity in each simulation month.
Submodels
Select electricity supplier
In the following we report on the numerical operationalisation of the individual-level decision-making concepts outlined in Section 1. Two components have to be considered: First, we need to explain how the assessment of a household’s electricity tariff is represented in agents. Second, we have to define the model representation of the mechanisms that trigger households to engage in deliberative decision-making on their electricity tariff.
We start by providing and explaining the partial utility calculations used in the agent-level assessments of the two electricity types. The utility formulas regarding each of the three goals are shown in Table 5.
Goal | Partial utility at timestep t | |
Green electricity | Grey electricity | |
Cost minimisation | Cecon*( 1 – rgreen,t / rgrey,t) | 0 |
Social conformity | 2*sgreen,t-1– 1 | 2*sgrey,t-1– 1 |
Ecological orientation | Cecol | -Cecol |
The partial utilities of the two tariff options with respect to the goal of cost minimisation reflect the relative price difference between the two types of electricity at a given timestep t (rgrey,t and rgreen,t). We use the price of grey electricity as a reference point and therefore we set the respective partial utility constantly to 0 (“neutral”). The partial utility of green electricity varies depending on the price development of the electricity market which is perceived by the agents at each simulation month. Accordingly, the partial utilities of green and grey electricity are both 0 when the two electricity types are equal in price, i.e. rgrey,t = rgreen,t in the utility formula. Likewise, the economic utility of green electricity exceeds 0 if rgrey,t > rgreen,t and it is below 0 if rgrey,t < rgreen,t. The magnitude of these differences is controlled by a constant factor Cecon which is discussed below.
The partial utility of a tariff option with respect to social conformity depends linearly on the share of customers of the respective type of electricity among the alteri in the agent’s simulated social network in the previous time step (sgreen,t-1 and sgrey,t-1). The utility value is -1 if none of the network neighbours has chosen the respective energy supplier in the previous time step and it 1 if all network neighbours have already chosen the respective energy supplier.
The partial utility with respect to ecological orientation represents the personal benefit or satisfaction which may be derived from becoming a customer of green electricity compared to grey electricity. To contrast the difference between the two energy types we set the utility value of green electricity to a constant Cecol and the utility of grey electricity to –Cecol.
The purpose of the factors Cecon and Cecol is to assure that the partial utility calculations operate on compatible domains, thus making the partial utilities comparable between the goal dimensions. The reference values for the scaling factors Cecon and Cecol are reported and discussed in section 3.8.
When households decide about their electricity supplier, they balance between the three goals and consider trade-offs according to their preferences. We model subjective preferences as agent-specific weighting factors attached to each of the goals. Based on its preference set, an agent determines the utility of each of the two electricity types. This overall utility of selecting an electricity tariff is calculated by adding up the partial utilities for each type of electricity multiplied with the respective preference factor. factor. The utility formulas are reported in Equation 1 and Equation 2. Equation 1 (below) shows the subjective utility function of green electricity, while Equation 2 (below) shows the subjective utility function of grey electricity. The weighting factors wecon, wsoc and wecol are agent-specific state variables which are set during model initialisation and remain constant over time.
$$u_{green,t}=w_{econ} \times c_{econ} \times (1-\frac{r_{green,t}}{r_{grey,t}})+w_{soc} \times (2 x s_{green,t-1}-1)+w_{ecol} \times C_{ecol}$$ | (1) |
$$u_{grey,t}=w_{soc} \times (2 \times s_{grey,t-1}-1)+w_{ecol} \times (-C_{ecol})$$ | (2) |
While the outlined utility assessments are the key driver of behavioural change in the model, it has to be further specified when and how the described subjective utility calculations are used during agent decision-making. We assume that the reassessment of a household’s electricity choice occurs with some frequency and that this frequency may increase due to relevant electricity prices changes. We model this frequency as an agent-specific probability of engaging in deliberation on the electricity tariff.
At the beginning of a simulation time step, the probability is set to a constant agent type-specific baseline probability (agent state variable pdelib) which is set during initialisation (see section 2.19). This initial probability reflects the general tendency of a household of a given type to invest cognitive resources in the elaboration on existing alternatives electricity tariffs. In the next step the deliberation probability is adjusted if a significant price change is perceived by the agent. To this end we do not have empirical data and therefore the model representation is based on assumptions. While numerous operationalisations are possible we assume that a price change signal occurs when the absolute relative change of the market price of a given electricity type between two months exceeds some threshold. In the simulation experiments presented here we use a threshold of 1.5% which is set homogenously for all agents. Furthermore, we assume that the price change is not immediately perceived by all agents but it becomes visible and salient in one of the months following the event with an exponential decay (in average six months). Finally, we assume that in the month when the price signal is perceived by an agent its deliberation probability is increased by 25%.
Depending on the adjusted deliberation probability, the agent either reassesses its electricity choice based on utility expectations or it skips further elaboration and keeps its previous electricity supplier. In the former case, the expected utilities for the two tariff options are calculated according to Equation 1 and Equation 2. The selection of a tariff is represented by a probabilistic choice model (Janssen & Ahn 2006) based on the respective expected utility.
Finally, if the agent selects green electricity it becomes an EWS customer if it is aware of EWS; otherwise the agent is counted as green energy customer of a different brand. An agent’s awareness of EWS is represented in the respective agent state variable which set during initialisation and modified by the submodel “Communicate” that is described in the following section.
The described sequence of steps is illustrated in the flowchart of agent decision-making shown in Figure 1.
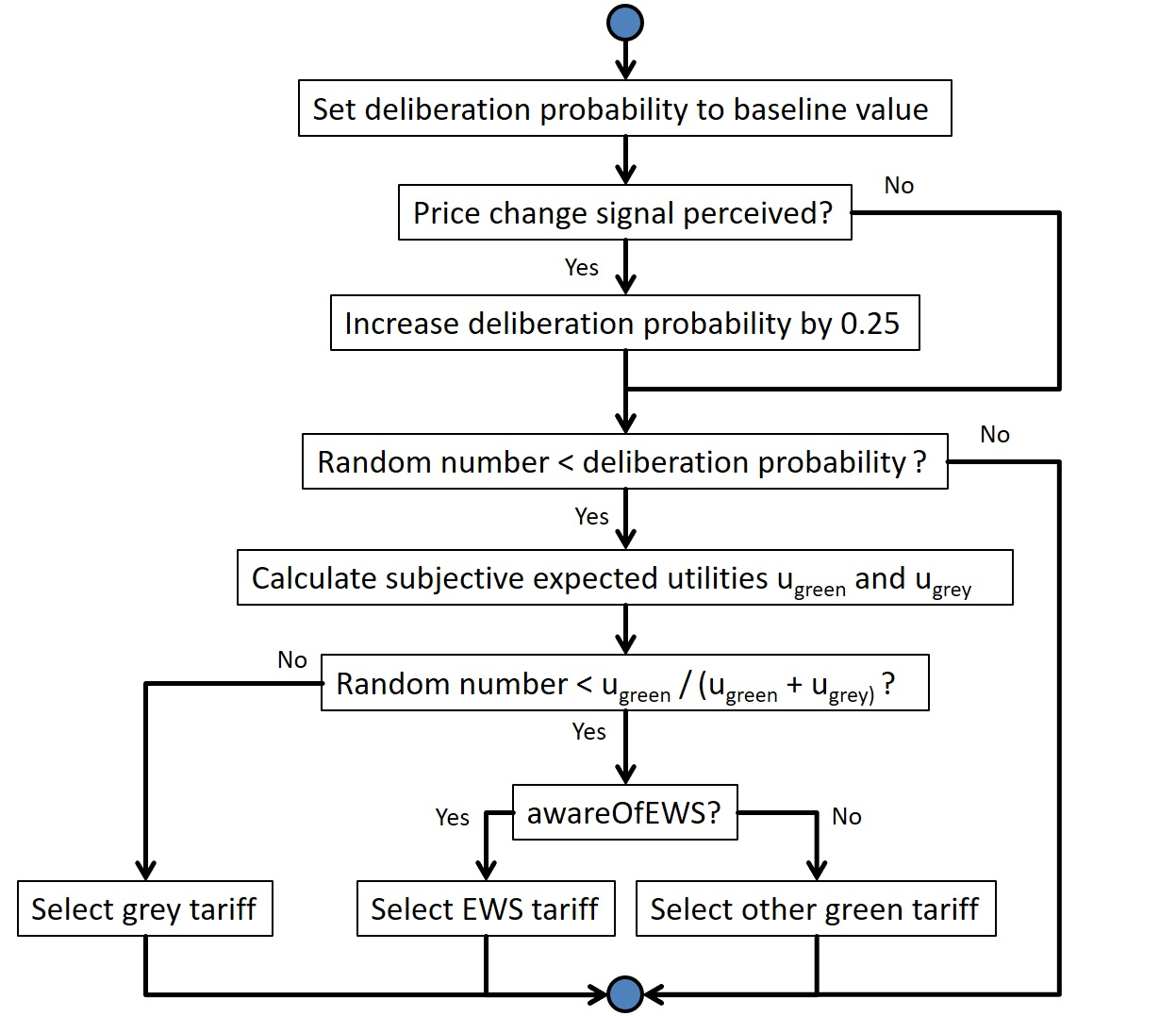
Communicate
The outlined process of individual decision-making is supplemented by a process of information diffusion influencing the individual awareness of EWS as a green energy brand. In the model, an agent can become aware of EWS by a word-of-mouth process of information exchange with another agent. If the other agent knows of EWS, the focal agent also becomes aware of EWS as a green energy supplier. Other sources of information like for instance media appearances of EWS or information campaigns organized by authorities or NGOs are not covered in the presented model. The activity of these information providers overlays the inter-agent processes (Buurma, Hennen, & Verwaart 2017) and can be transparently added to the model. However, we leave this to future work. Accordingly, we represent the information diffusion as a basic susceptible-infected model (Watts & Gilbert 2014, 35ff) with the extension of heterogeneous communication probabilities and a spatial communication bias.
The modelled process of information diffusion depends on the communication probabilities of the agents which reflect their respective desire to obtain information on green electricity. Empirical research on communication behaviour of green electricity customers (Gellrich 2016, p. 228–228) indicates that this communication probability is higher for agents representing green energy adopters (parameter pinfoAdopters) compared to agents using grey electricity (parameter pinfoGrey). Furthermore, we assume that the focal agent selects its communication partner either from a spatial neighbourhood comprising agents from the same postcode area or it selects the communication partner from the social network neighbours. We operationalise the distinction between local and social network communication by a probability pinfoGeo that is set homogenously for all agents. Communication partners are selected locally with probability pinfoGeo, else the communication happens in the focal agent’s social network which may span larger spatial distances. Figure 2 shows a flowchart of the described model of information diffusion. The values for the parameters pinfoAdopters, pinfoGrey and pinfoGeo are reported and discussed in section 3.8.
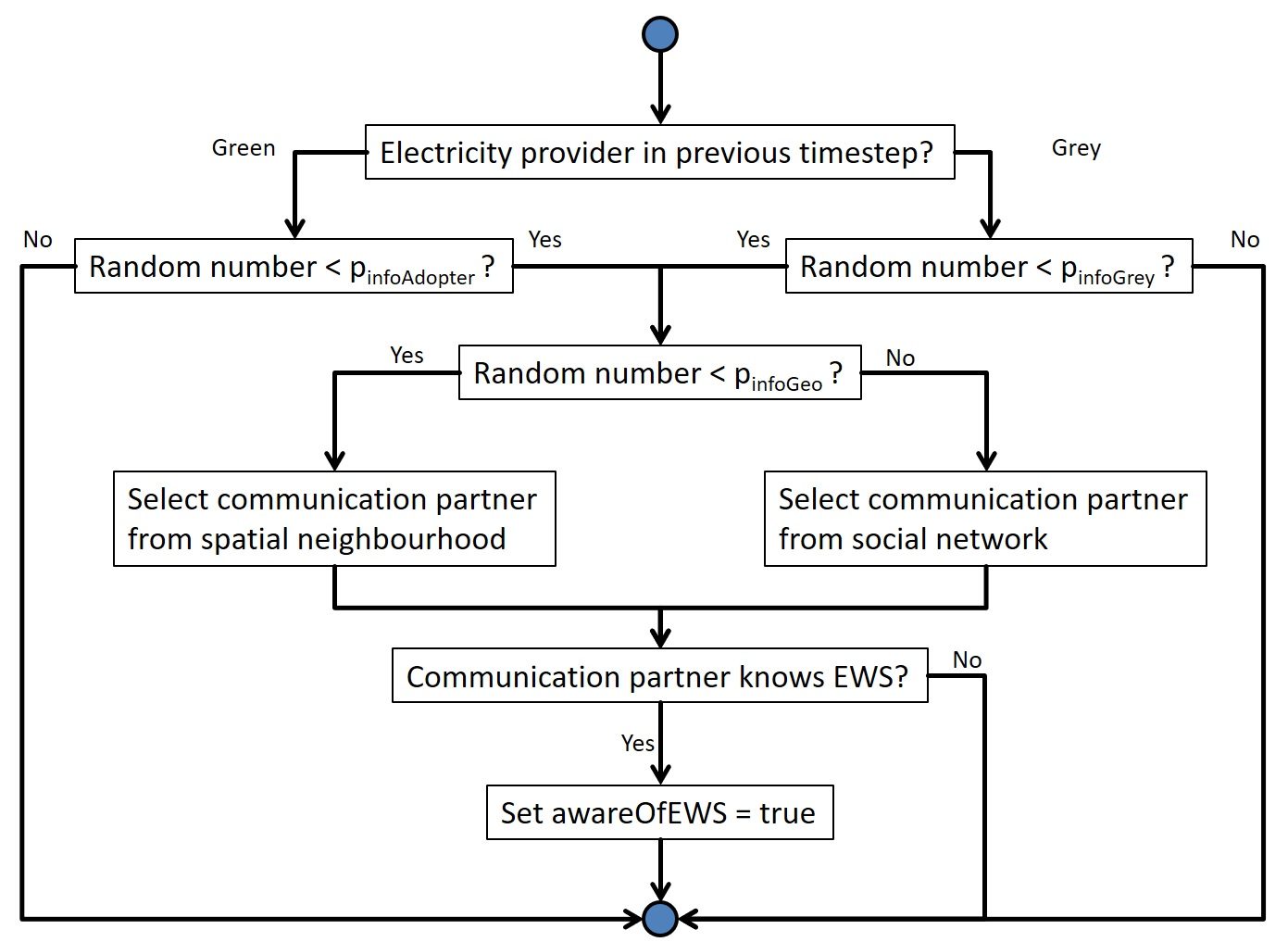
Simulation results
This section presents simulation results obtained from various runs of the described model. The focus of analysis is on the model’s retrodictive validity under the different parameter settings. Therefore, we systematically assess the model’s ability to reproduce temporal and spatial patterns found in historical data of the green electricity market in Germany for the time range from the empirical model initialisation (see section 2.18) to December 2011. The methods employed belong to the domain of parameter exploration and sensitivity analysis (Lee et al. 2015, 3.1ff) and spatial output analysis (Lee et al. 2015, 4.14ff). Following this analysis, we report on selected results from the calibrated model with particular focus on different patterns of diffusion emerging from the model.
We start by introducing validation measures which quantify the similarity of empirically observed indicators of the diffusion process and the respective indicators calculated from simulation results. Following this, we apply the validation measures to assess different model parameterisations and to identify suitable parameter settings by a model calibration exercise. Finally, a deeper discussion of individual simulation runs of the calibrated model is presented.
Validation measures
Model state variables and their mapping to empirical data
The presented validation measures focus on two agent-level state variables of the outlined ABM. The first variable is the electricity supplier selected by the respective agent in the model. This variable can take three states: Green electricity from EWS, green electricity from a supplier other than EWS, or grey electricity. The second model variable investigated can take two states and represents whether an agent is aware of EWS as an energy brand. The two model variables are indicative of the outcome of two different interacting agent-level processes in the model namely electricity tariff choice and information diffusion by agent-to-agent communication. During model initialisation, the two agent state variables are set up according to the December 2004 time slice of empirical time series data.
The purpose of a retrodictive validation is to make statements on the relation of model-generated time series starting from January 2005 to the respective observed historical time series at hand. For the market share of the different electricity suppliers, validation data sets are given by the spatially explicit EWS customer data and by the spatially-aggregated customer numbers of the three major suppliers of green energy in Germany from 2004 to 2011. For the market awareness of EWS no direct empirical validation data are available. Therefore, brand awareness of EWS was estimated depending on the brand’s market share. To do so, a regression analysis of existing survey data on market share and customer awareness of various electricity brands was performed. Then, EWS brand awareness was estimated based on the described spatially and temporally explicit EWS customer data. The estimator used is shown in Figure 3. Details are found in appendix 6.1.
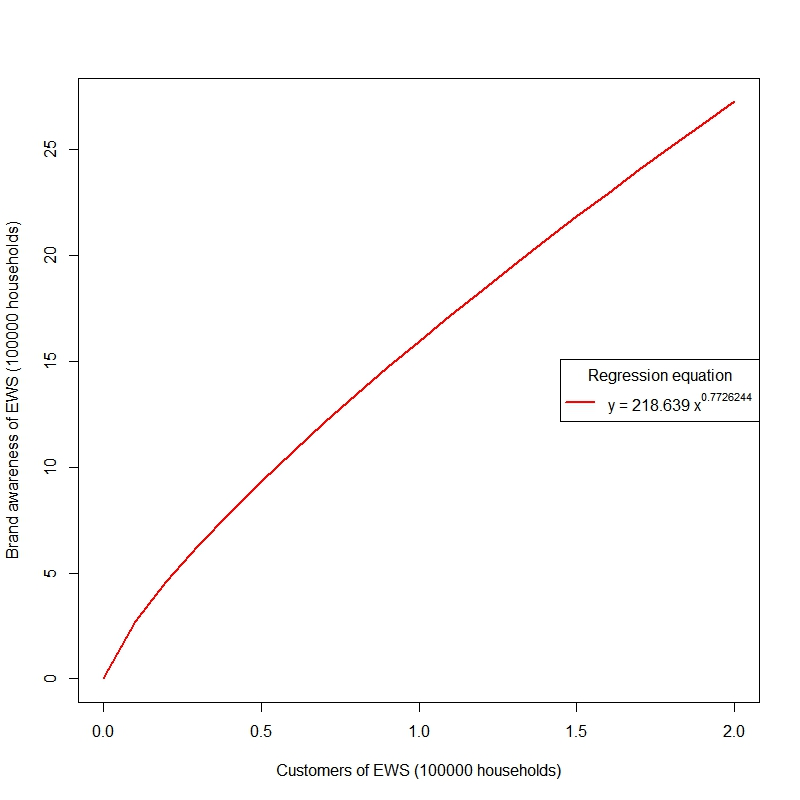
Measures of model fit
The calibration exercise reported in the following section investigates two measures of model fit which capture different aspects of the diffusion process. The first measure evaluates time series of spatial aggregated model state variables x(t) with respect to spatially-aggregated historical data y(t) for the considered time range from January 2005 to December 2011 on a monthly resolution. The most common approach to quantify the fit between two time series is to calculate the respective differences between observed and modelled data points and to evaluate the residuals. While numerous residual methods exist (see e.g. Bennett et al. 2013), mean square error measures are “good initial candidates” (Bennett et al. 2013, p. 16–16). We use the mean square error of the relative differences between simulation and real-world data over all time steps of the considered time range (MSRE).
The second measure looks at spatiotemporal patterns in the EWS customer data. To do so, we calculate the average increase of the market share of EWS from 2005 to 2011 at a spatial resolution of 50km for Germany (total of 169 grid cells). For each cell on the grid we determine an indicator of innovation spread by dividing the mean number of agents / households becoming EWS customers during 2005 to 2011 by the total number of agents / households located in the cell. Then we classify the data into four categories given by the quartile ranges of the indicator for the 169 cells based on the empirical data. Based on this indicator, an objective quantification of the spatial fit of model data and empirical data may be obtained by comparing the observed and modelled data in the form of an error matrix (Congalton 1991). For this technique a contingency table in constructed where rows represent categories assigned to cells by the simulation model and columns represent categories of the cells based on empirical data. In order to further substantiate the observed differences it is common to calculate specific error measures based on the matrices (see Congalton 1991, p. 36–36; Bennett et al. 2013, p. 7–7). Here, we use the Kappa index of agreement (KIA, Cohen 1960) which was previously used in the validation of spatial map outputs of ABMs (Manson 2005). The KIA relates the success rate of the model in assigning cells to categories to random assignment. See Table 6 for an overview of the measures and the respective formulas.
Indicator | Measure definition | Description |
MSRE | \(\frac{\sum_{t=1}^N \Bigl(\frac{x(t)-y(t)}{y(t)}\Bigr)}{N}^2\) | Mean square error of the relative differences between simulation and real-world data over all time steps t from 1 to N=84 in months. |
KIA | (E1 - E2)/E1 where E1 (= 1 - 1/number of quantiles) is the expected error rate if cells are randomly assigned to categories. E2 is the error rate of the simulation, i.e. the number of cells assigned to wrong quantiles divided by the total number of cells. | Kappa index of agreement, i.e. the improvement in “predicting” the historical data that can be attributed to the simulation results compared to a “random model”. |
It has to be noted that the KIA measure is based on cell-by-cell comparisons and might omit other spatial regularities. Future work could include alternative quantitative measures of spatial fit (Lee et al. 2015, 4.14ff) and in particular indices of spatial dissimilarity which were previously used to quantify spatial properties of urban patterns in ABM (da Fonseca Feitossa 2010, 29ff).
Model calibration
Calibration parameters and procedure
The presented simulations are identical regarding the empirical initialisation but differ in the parameterisation of the two submodels outlined in the ODD description. The submodels state a total of five parameters which cannot be directly set from empirical data. In a first step we determine Cecon such that the utility calculations for cost minimisation and social conformity operate on the same scale. To do so, we use the historical price development of green and grey electricity and set Ceconsuch that the minimum partial utility of green electricity with respect to cost minimisation is -1 during the time period (which implies that the maximum value of the partial utility is 0.15).
The remaining parameters are analysed in the calibration exercise. Parameter Cecol defines the ecological utility value of green electricity as well as the negative ecological utility –Cecol of grey electricity (see Table 7). The parameter allows scaling the relation between ecological orientation and the other two goals in the utility calculations. The remaining three parameters define the characteristics of the modelled communication processes regarding the market awareness of EWS, namely the respective communication probabilities of agents representing adopters of green electricity and grey electricity customers (pinfoAdopters and pinfoGrey), and the spatial bias of the communication (pinfoGeo). Table 5 gives an overview of the parameters and the values investigated.
Parameter | Investigated value range | Description |
Cecon | 6.815 (determined based on electricity market data) | Magnitude of the partial utility of the two electricity types with respect to cost minimisation |
Cecol | 0.4 to 0.8 in steps of 0.1 | Magnitude of the partial utility of the two electricity types with respect to ecological orientation |
pinfoGrey | 0.02 | Communication probability of agents representing grey electricity customers |
pinfoAdopters | 0.02, 0.1, 0.5 | Communication probability of agents representing green electricity adopters |
pinfoGeo | 0.0 to 1.0 in steps of 0.125 | Proportion of communication partners selected from spatial neighbourhood |
The computation time of 3 hours for a model run restricts the design of the model analysis. Therefore the calibration exercise does not systematically focus on parameter interactions but follows a “one-parameter-at-a-time” design (Lee et al. 2015, 3.9). Yet, due to the small number of parameters investigated we will qualitatively address potential interaction effects as we discuss the simulation results. In addition, we run only five model replications for each parameter setting with differ in the seed of the model’s random number generator and do not systematically determine the statistically required minimum number of replications (Lee et al. 2015, 2.3). Finally, it has to be noted that the proposed full factorial sampling of the parameter space is limited to discrete values and potentially misses interesting combinations between the selected parameter values. The problem can be avoided by applying Latin hypercube sampling which was previously applied in ABM (Collins et al. 2013). We leave this as future work.
Market development of green electricity
This section investigates the simulated overall development of the German retail green electricity market independent of a particular green electricity supplier. The empirical reference data comprise the yearly customer numbers of the three major suppliers of green energy in Germany and the respective EWS customer data on a spatial aggregation for Germany by December of the respective year. The corresponding model indicator is given by the total number of green electricity customer agents in a given month of the simulation. Note that this model output is independent of a particular parameterisation of the information diffusion. Therefore, the simulated customer development solely depends on the maximum ecological utility Cecol which agents may derive from selecting green electricity.
Figure 4 displays the simulated development of the overall green electricity market depending on the setting of Cecol where the dashed time series shows the historical market data. In the diagram, vertical reference lines mark price change events which are perceived by the agents and which temporally increase the tendency of an agent to deliberate about its electricity supplier. The colour of the reference lines indicates price changes of both electricity types (black) and only one of the types (green or grey).
In accordance with the utility definitions given in section 2.28 we see that simulated green electricity customer numbers are higher during the considered time span when Cecol increases. Furthermore, the gradient of the curves shows some sensitivity to the price signals; in particular with respect to the price signal regarding only grey electricity in 2007 and the one pertaining to green electricity after 2010. The steep increase in the historical customer data in 2011 is not reproduced by the simulations because the effect was most likely caused by the Fukushima hazard in April 2011 which is not included in the model version presented here.
During the first two years, the interplay between cost minimization and ecological orientation appears to depend critically on Cecol. The price advantage of grey electricity during the first years inhibits a growth of the green electricity market share up to a threshold value for Cecol somewhere between 0.5 and 0.6. Above the threshold, ecological utility can effectively compensate negative economic utility even during the initial years.
After 2009 we observe a similarly critical interaction between ecological utility and social conformity: Comparing the simulations with the two highest values of Cecol there is a transition between a dampened increase of green electricity market share for on Cecol=0.7 and a steep increase of customer numbers for Cecol=0.8. The latter case resembles an “avalanche effect” propagated through social networks which is caused by a dominance of the social conformity and ecological orientation partial utilities in individual decision-making for Cecol=0.8. In contrast, for the lower setting of Cecol the development clearly reflects the price increase of green electricity after 2010 which indicates a relatively high importance of the economic partial utility during decision-making.
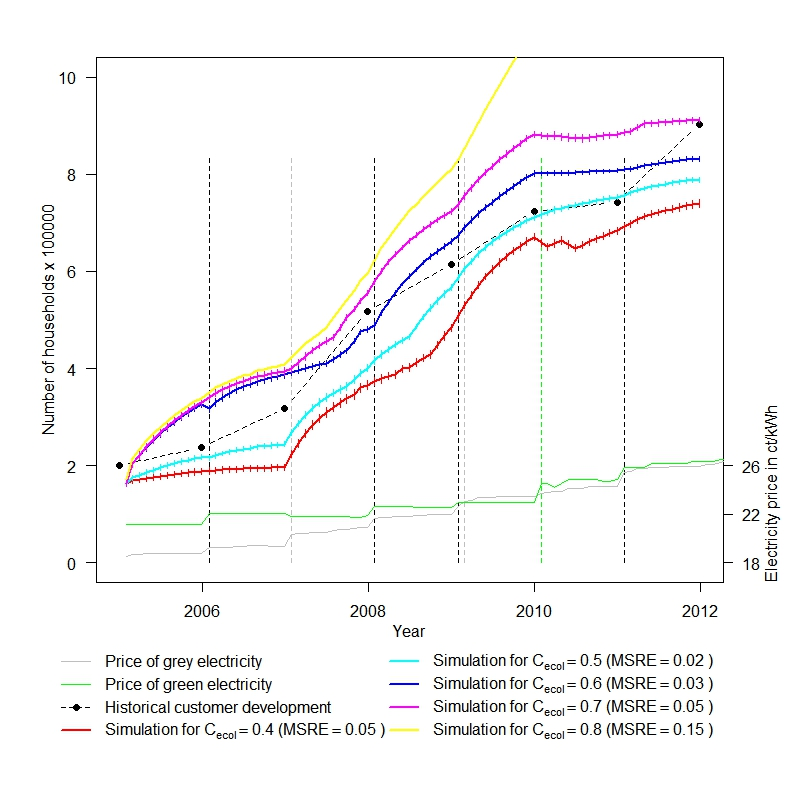
Comparing the overall fit of the five simulations to the historical data we see that the MSRE is quite small for all values of Cecol except for Cecol=0.8. The runs with lowest two settings of the parameter mostly underestimate and the other runs rather overestimate the customer development. For the investigations in the following sections we restrict the settings for Cecol to the values 0.4, 0.5, 0.6 and 0.7.
Information diffusion
As a next step we investigate the modelled market awareness of EWS. The empirical reference is given by the estimated awareness based on the EWS customer numbers. For the model analysis we vary the spatial bias of the information diffusion (parameter pinfoGeo) and the communication probabilities (parameters pinfoGrey and pinfoAdopters) according to Table 5 with the exception that parameter Cecol is only varied from 0.4 to 0.7. For each parameter combination we run independent model replications from 2005 to 2011 and calculate the mean of the MSRE measure for the runs.
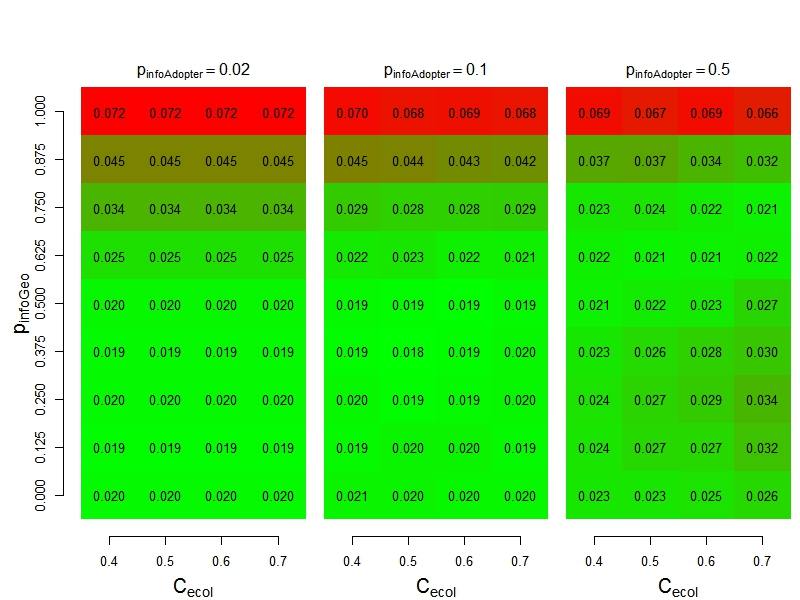
Figure 5 visualises the simulation results. As a general trend the model fits the reference data well (with only slight variations) as long as the spatial bias of the information diffusion is below 1.0. When communication partners are exclusively selected from a spatial neighbourhood (pinfoGeo=1.0) the MSRE increases significantly. This shows that the inclusion of social networks in the model is essential in order to explain the information diffusion. However, the lowest values of MSRE are found for pinfoGeo=0.50, i.e. for a balanced combination between social network and local communication.
Considering the first block of simulations (pinfoAdopters=0.02) the MSRE is independent of the setting for Cecol . This is consistent with our model definition because under these settings holds pinfoAdopters=pinfoGrey, which means that the expected overall number of communication events is independent of the respective number of green energy adopters. In contrast, for the other two blocks of simulations the expected total number of communications increases as the total number of green energy customers rises depending on the setting of for Cecol (see Figure 4). In turn, the MSRE increases slightly in particular in the right hand panel of Figure 5. Still, the magnitude of the increase remains marginal because of the generally small market share of green electricity (see below).
EWS customer development
The next analysis looks into the combined effect of the overall green electricity market development and information diffusion regarding the supplier EWS. Here, the empirical reference data is given by the spatiotemporal EWS customer data at hand. Like in the previous section we explore different model parameterisations and run independent model replications from 2005 to 2011 for each parameter combination. In addition to the MSRE we include the KIA measure in the assessments. Figure 6 shows the assessment of the spatially-aggregated temporal development of the EWS customer numbers. Three general patterns may be observed in the evaluations:
- First, the MSRE decreases as Cecol increases, i.e. the modelled overall market share of green electricity has to be sufficiently high in order to achieve a good fit of the EWS customer development.
- Second, simulations get better when the communication probability of adopter agents pinfoAdopters is assumed higher. Clearly, on a population level, higher settings for this parameter selectively accelerate the information diffusion to agents which were adopters at some point in time. When these agents reconsider their electricity supplier, they are more likely than others to adopt green electricity again but now being aware of EWS.
- Finally, in line with the results regarding information diffusion the assessments in Figure 6 indicate that the MSRE is smallest for medium settings of the spatial bias of information diffusion pinfoGeo. The finding suggests that the explanation of the EWS customer development involves combined spatial and social network considerations on the agent level.
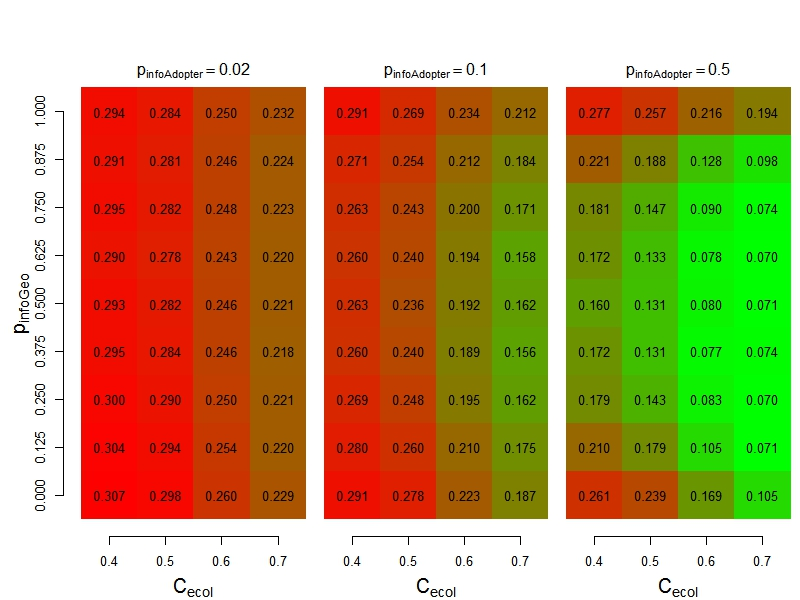
The final calibration assessment investigates the spatial fit of the simulation data to the empirical customer data. Figure 7 shows the introduced KIA measure for the different model parameterisations. The general trend is mostly in line with the observations regarding the MSRE measure: The model fit is best for rather high communication probabilities of adopter agents along with medium settings for the spatial bias in agent communication. Still, the magnitude of the fit remains low: The average KIA over model replications does not exceed 0.209 which would indicate a 21% improvement in assigning spatial grid cells to quartile categories compared to random allocation. Yet, the relative trend of the KIA in particular regarding the setting of Cecol is stable.
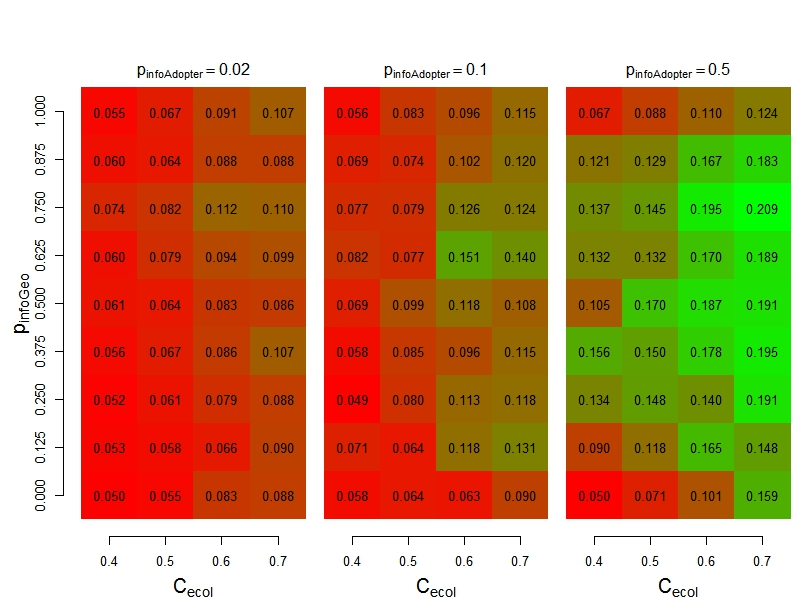
Combined assessments
The model analysis presented in the previous subsections comprises a total of four assessment indicators, namely the MSRE of the modelled overall green electricity market, the MSRE of the modelled market awareness of EWS, the MSRE of the modelled EWS customer development, and the KIA measure of the spatial spread of EWS customers. In order to achieve a consistent model assessment, all of the indicator dimensions have to be considered in combination. Assuming that all four assessment dimensions are equally important we can define a rank-based score measure for individual model runs as follows: For each model simulation run including replications we determine the four assessment indicators. Then we determine the ranks of the simulation runs with respect to each indicator dimension and perform a linear mapping of ranks on each dimension to a score value ranging from 0 for the lowest score on the dimension to 1 for the highest score. Finally, we calculate the total score for a model run as the (unweighted) average of the run’s scores on all of the indicator dimensions. The results of this combined assessment are shown in Figure 8.
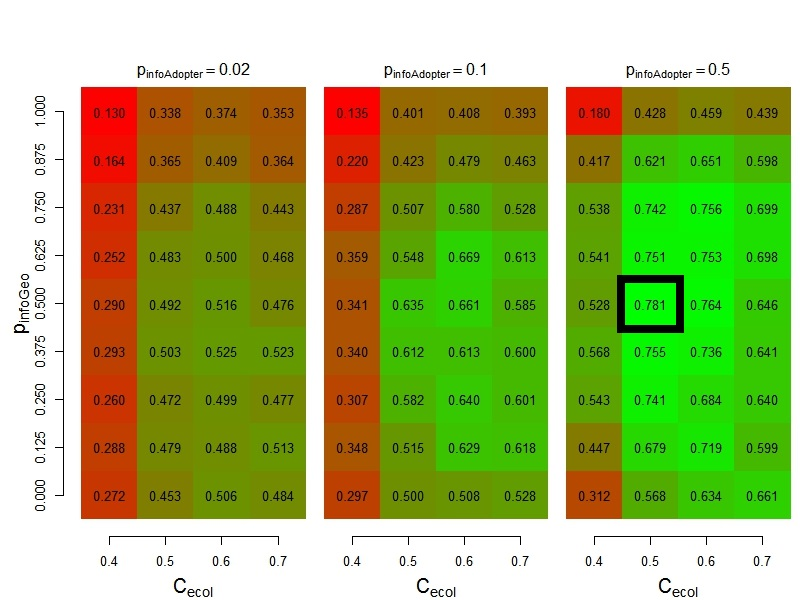
Figure 8 shows that the highest overall model scores are found in the right hand panel for pinfoAdopter=0.5 where the best six parameterisations reach scores of at least 0.75. For the more detailed discussion of simulation results in the following subsection we use the parameterisation with the best overall score, i.e. Cecol=0.5, pinfoAdopter=0.5, and pinfoGeo=0.5.
Selected simulation results
This section reports simulation results for the best scoring model parameterisation. Figure 9 shows four different views on spatially-aggregated data for Germany. As a reference, the first diagram in Figure 9 displays the total number of green electricity customers for the selected parameterisation as discussed above (cf. Figure 4). The top right diagram adds a different perspective and shows the lifestyle affiliations of the green energy customer agents. Starting from model initialisation when customer agents are assigned exclusively to postmaterialist and hedonistic agents the innovation spreads to agents of the other lifestyles during the course of the simulation. In turn, the social composition of the green electricity customer group changes over the simulation time. This social diffusion process shows a distinct temporal sequence: While agents representing leading lifestyles start buying green electricity from the beginning of the simulation, mainstream and traditional agents increasingly adopt from the end of 2007. The micro-level grounding of this effect is found in the agent preference sets for the lifestyles (see Table 1) which influence the utility calculations during deliberative decision-making: Agents of the three early adopting lifestyles have relatively high preference weights on the goal of ecological orientation. Therefore, for theses agent types the partial utility with respect to ecology compensates low utility regarding cost minimisation during the initial years when green electricity is comparatively expensive. On the other hand, traditional and mainstream agents have relatively high preference weights on the goal of cost minimisation which triggers them to adopt when the price difference between grey and green electricity decreases towards the end of 2007. Furthermore, agents of these types have high preference weights for social conformity which makes their utility calculation more sensitive to increasing numbers of adopters in their social networks. The latter effect gains additional weight as the total number of green electricity adopter agents rises after 2008.
The bottom two diagrams in Figure 9 show simulation results regarding EWS. The left diagram shows the simulated development of the brand awareness of EWS and the estimated historical awareness of EWS (dashed). Qualitatively the simulation gets close to the empirically estimated data and, as might be expected, some path dependency due to random factors becomes visible in the error bars over the model replications. Still, the shape of the simulated awareness curve remains simplistic due to the minimal assumptions on the modelled process of information diffusion. Likewise, the simulated EWS customer development in the bottom right diagram qualitatively reproduces the trend in the empirical data (dashed). Yet, the bend in the empirical customer data around 2008 and the decrease in 2010 are not covered by the model. These observations indicate the presence of additional external events influencing customer decisions which are not represented in the model structure.
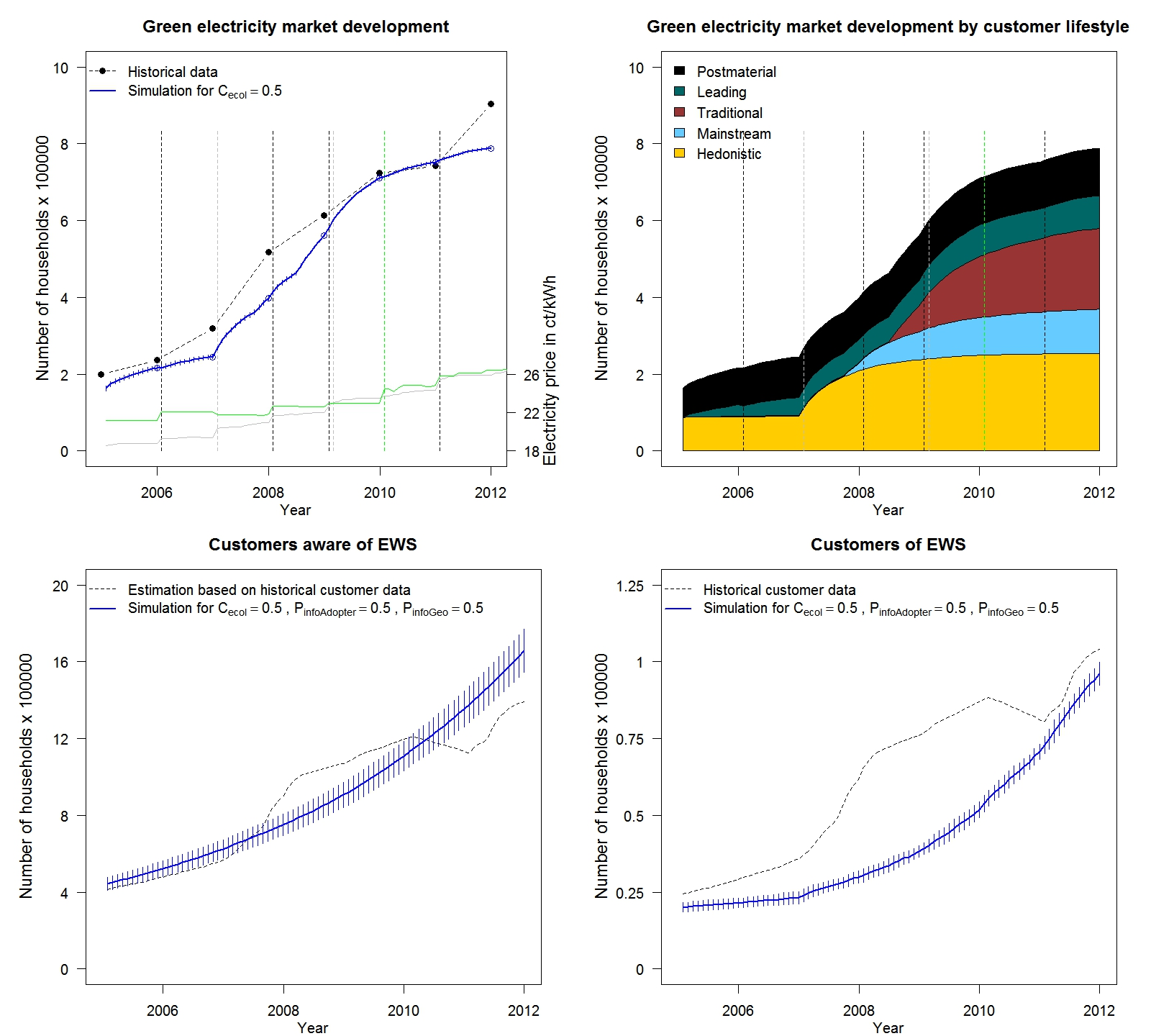
Finally, we investigate patterns in the spatiotemporal spread of EWS customers. To do so, we show maps of the average increase in market share from 2005 to 2011 at a spatial resolution of 50km for Germany. In Figure 10, the respective quartile categories of the cells (as used for the calculation of the KIA measure used in calibration) are shown in shades of blue. The simulation roughly captures some of the spatial patterns in the historical data: a relatively high increase in market share located in a contagious area in the southwest of Germany and around three larger cities starting from the North Hamburg, Berlin and Dresden. In contrast to this characteristic pattern of high diffusion speed, regions with low increase of market share are not well captured by the simulation. This is indicated by the comparatively large grey areas on the simulation map.
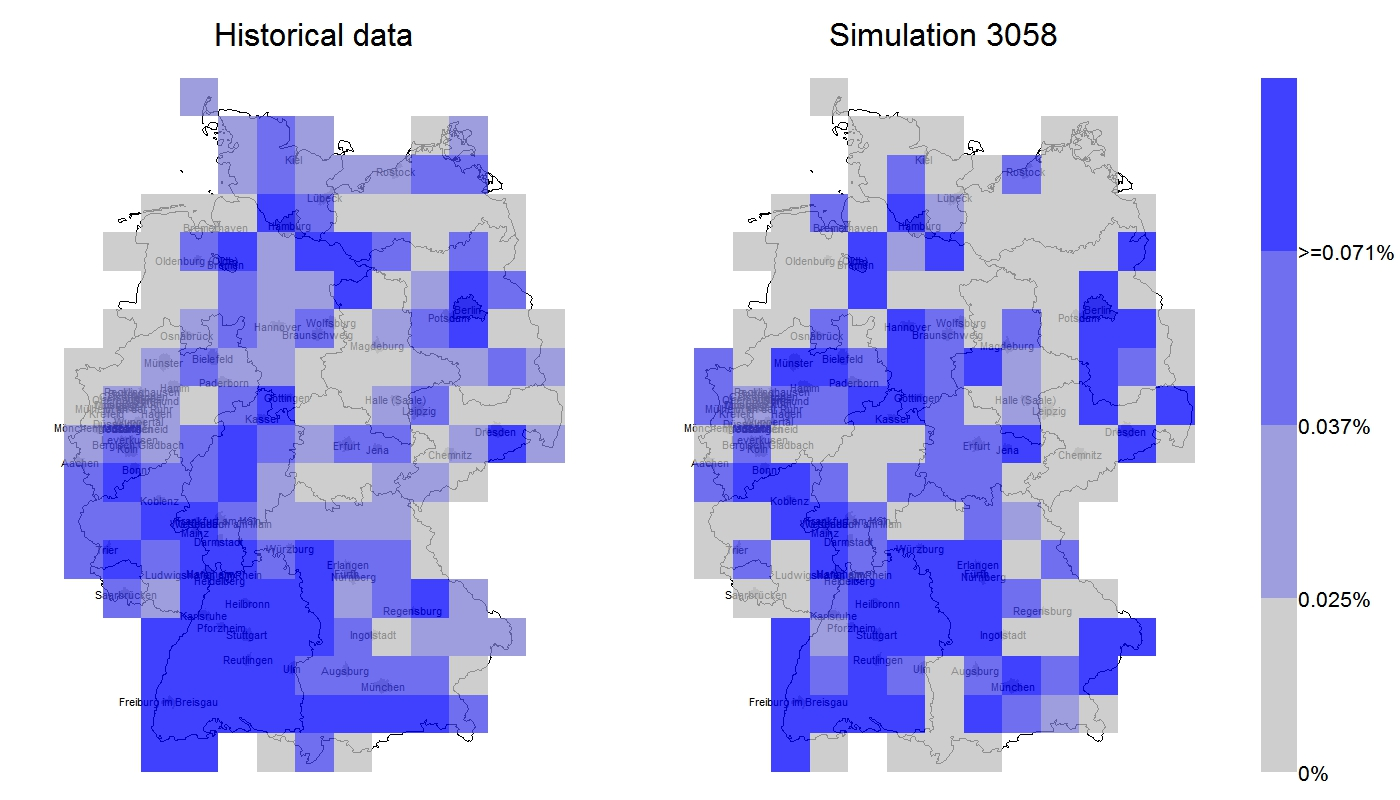
Discussion and conclusions
Rich empirical datasets become increasingly available and pose novel challenges to the empirical grounding and validation of spatially explicit agent-based diffusion models. The common argument for ABMs is their potential to capture structural properties of a target system in order to propose explanations of collective phenomena. However, once real-world geography is included in the simulations, the credibility of ABMs is significantly increased if the degree of spatiotemporal fit of simulation results and empirical data can be stated, in particular when offering decision support in policy contexts. The ABM exercise presented in this paper was initially motivated by the availability of high resolution household data on the spread of green electricity supply in Germany. The underlying research question is as old as social simulation: “Can you grow it?” (Epstein & Axtell 1996, p. 19–19) - Can we define a spatially explicit ABM that reproduces the spatiotemporal diffusion process found in the empirical data?
Our approach of modelling household decision-making on an electricity supplier in agents is parsimonious but psychologically sound regarding the represented micro-level concepts and theories. On one hand the embedding of agent properties in psychological theory ensures structural model validity on the micro-level. On the other hand the included psychological concepts may be “parameterised” by results from psychological surveys. We utilised this link by introducing five differently parameterised agent types representing household types according to the Sinus Milieus® for Germany. The properties of the agent types were derived from the general characterisations of the Sinus Milieus® and from domain specific survey results. To address our research question we set up a representative population of household agents which is grounded in a detailed dataset on the spatial distribution of the Sinus Milieus® in Germany. The design of the presented simulation experiments followed the typical workflow of an ABM exercise: In a first step the model was calibrated and validated. Then we investigated simulation results for one selected parameterisation in more detail.
For the experimental setup of the retrodictive validation and calibration procedure we used one time slice of historical data to initialise the customer side of the green electricity market in the model, ran simulations to some end point in time and compared the simulated spatiotemporal development to the historical market development for the respective time range. For the latter kind of ABM output analysis a variety of established methods exist (Lee et al. 2015) but they have rarely been consistently used in the domain in innovation diffusion. This is likely due to a lack of sufficiently comprehensive social science data which marks a well-known problem of empirical validation of ABM (e.g. Troitzsch 2004). In contrast, the presented ABM analysis draws on existing data of the German green electricity market including detailed customer data of one of the major green electricity supplier on spatial and temporal resolution. In order to assess the similarity of the simulation results to historical observations we introduced two validation measures which capture different aspects of the green electricity diffusion. One measure is based on the residuals of spatially-aggregated time series of model indicators and the other measure considers a temporally aggregated but spatially disaggregated indicator of spatial spread. The measures were applied to assess the sensitivity of the simulation results to the model assumptions on the personal benefit individuals associate with choosing green electricity on one hand and on the characteristics of the information diffusion of a particular supplier of green electricity on the other. We concluded by selecting a model parameterisation for further analysis based on the obtained scores according to the validation measures.
The investigation of the simulation results for the selected model parameterisation provided a view on two different sources of heterogeneity “underneath” the diffusion curve of green electricity in Germany. The first source of heterogeneity pertains to the social composition of the green electricity adopters and its development over time. We showed how individual-level subjective balancing between possibly conflicting behavioural goals can yield plausible collective dynamics when embedded in a dynamic economic context and in networks of social influence. The presented simulations commenced at a point in time where the green electricity diffusion is restricted to the most innovative lifestyles, i.e. typical early adopters in the sense of Rogers. The investigation of the course of the simulation after initialisation revealed how the green electricity innovation might spread to the other lifestyles as the result of a complex mix of inter-individual social dynamics, subjective individual preferences and external economic dynamics. The temporal sequence in the diffusion process found in the simulation results is qualitatively in line with empirical results (Gellrich 2016, p. 237–237). Yet, the proportion of traditionals in the simulated adopter group is significantly too high compared to empirical results.
The second investigation of the selected model parameterisation considered the spatial heterogeneity of green electricity adoption rates. In contrast to the assessments of social heterogeneity we could use the detailed EWS customer data as a fine grained reference for visual comparison. In addition to the KIA measure evaluations used in the calibration exercise the maps allow scrutinising spatial arrangement of the grid cells. The visual similarity of the simulated diffusion patterns and those of the empirical data hints at the structural validity of the model with respect to core characteristics of the spatial diffusion process. Further investigations could take an explanatory direction and scrutinise local population characteristics which drive or inhibit diffusion. One hypothesis is that the high spread in the southwest of Germany where the initial customers and the company headquarters are located has different drivers than the high spread in more distant metropolitan areas. While the former is likely driven by local circumstances (e.g. life style composition of the local population), the latter is probably more depending on social network exchange over weak links (Granovetter 1973). The respective mechanisms are descriptively represented in the model and could be probed and assessed on agent-level.
A point has to be made on the implications arising from the “mental calculations” at the heart of agent decision-making. The involved notions of utility are well grounded in psychological theories of decision-making which were independently validated in many empirical contexts. This holds in particular for the Theory of Planned Behaviour. Still, it may be argued that the utility approach has drawbacks in its compatibility with the paradigm of bounded rationality. However, this argument is substantially weakened when applied in an ABM context that naturally accounts for various forms of subjective and limited information processing on the micro-level of agents. A limitation of the presented approach may be seen in the introduced preference weights which are set to constant agent-type-specific values. It has to be acknowledged that preferences are subjective in nature and that within one milieu group a range of weights per goal should be expected. A possible approach is to use empirically motivated goal weights as desired mean values for a particular agent type and include random variance when initializing individual agents while preserving the differences between the agent types. Future model versions could explore the effect of such intra-lifestyle heterogeneity on the simulation results.
Finally, some methodological comments have to be made on the proposed retrodictive calibration and validation procedure. In general, the employed “history-friendly” approach (Windrum et al. 2007) bears the problem that a model which is fitted against one particular historical account might lack the ability to provide any generalisations in terms of predictions of future developments beyond the historical data used for calibration or concerning the applicability of the model to other similar empirical cases (Windrum et al. 2007, 4.25). For the presented model I would react on such concerns in several ways: On the micro-level the model is grounded in general psychological theories which have typically been independently validated in numerous empirical contexts. As outlined above this grounding contributes to the validity of the model’s structure and therefore enables the model to propose explanations which is a virtue per se and does not imply or require the model’s ability to predict (Epstein 2008, 1.9). Finally, the theoretical grounding of the model limits the target of the calibration exercise to a few descriptive and transparent parameters which may not directly be set from available empirical data. In other words, the calibrated model parameterisation defines the most plausible boundary conditions for further explanatory research based on the detailed descriptive micro-level data generated by the simulations.
Acknowledgements
The research presented in this article was partly funded by the German Ministry for Education and Research (BMBF) under contract no 01UV1003A, “Scenarios of Perception and Reaction to Adaptation (SPREAD)”. Early versions of the paper were presented and discussed at the Eleventh Artificial Economics Conference in Porto 2015 (Krebs 2015) and at the Social Simulation Conference 2015 in Groningen (Krebs & Ernst 2017). Special thanks go to Angelika Gellrich for carrying through parts of the empirical research reported here. I also greatly appreciate the inputs of Andreas Ernst, Sascha Holzhauer and Klaus Troitzsch. Finally, I would like to thank Tim Verwaart and Pedro Campos for editing the special section for JASSS and the anonymous referees for many helpful comments.Appendix: Estimation of brand awareness based on customer numbers
The historical market awareness of EWS had to be estimated based on the brand’s market share data at hand. To do so, we draw on existing survey data on market share (Bundesverband der deutschen Energie- und Wasserwirtschaft e.V. (BDEW) 2013) and on customer awareness (Serviceplan 2010) of eight major electricity brands for 2009. In order to obtain an estimator function for the brand awareness of an electricity supplier based on its customer numbers a regression analysis of was performed. Figure 11 shows the results of the regression including the estimator function used. Details on the regression approach are reported elsewhere (Heister 2014).
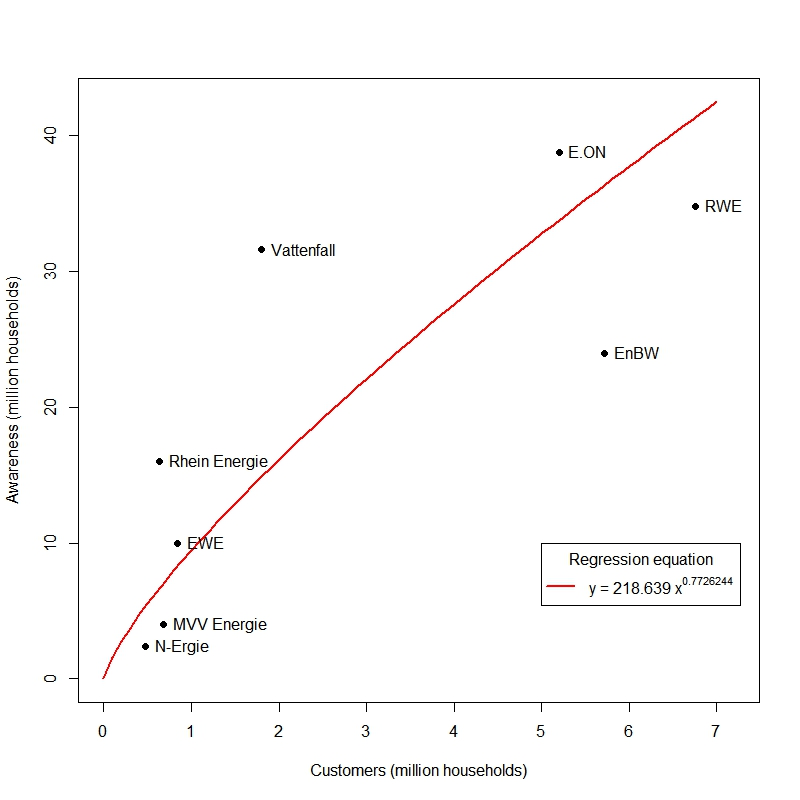
References
AJZEN, I. (1985). 'From intentions to actions: A theory of planned behavior'. In J. Kuhl & J. Beckman (Eds.), Action-control: From cognition to behavior (pp. 11–39). Heidelberg, Germany: Springer. [doi:10.1007/978-3-642-69746-3_2]
AJZEN, I. (1991). The theory of planned behavior. Organizational Behavior and Human Decision Processes, 50(2), 179–211.
AMBLARD, F., Bommel, P., & Rouchier, J. (2007). 'Assessment and validation of multi-agent models'. In D. Phan (Ed.), GEMAS studies in social analysis. Agent-based modelling and simulation in the social and human sciences (pp. 93–114). Oxford: Bardwell Press.
BARON, J. (2007). Thinking and deciding (3. ed, 7. print.). Cambridge, UK: Cambridge Univ. Press.
BASS, F. M. (1969). A New Product Growth for Model Consumer Durables. Management Science, 15(5), 215–227. [doi:10.1287/mnsc.15.5.215]
BENNETT, N. D., Croke, B. F., Guariso, G., Guillaume, J. H., Hamilton, S. H., Jakeman, A. J., et al. (2013). Characterising performance of environmental models. Environmental Modelling & Software, 40, 1–20.
BOURDIEU, P. (1984). Distinction: A social critique of the judgement of taste. Cambridge, MA: Harvard University Press.
BRAVO, G., Vallino, E., Cerutti, A. K., & Pairotti, M. B. (2013). Alternative scenarios of green consumption in Italy: An empirically grounded model. Environmental Modelling & Software, 47, 225–234.
BRIEGEL, R., Ernst, A., Holzhauer, S., Klemm, D., Krebs, F., & Martínez Piñánez, A. (2012). 'Social-ecological modelling with LARA: A psychologically well-founded lightweight agent architecture'. In R. Seppelt, A. A. Voinov, S. Lange, & D. Bankamp (Eds.), International Congress on Environmental Modelling and Software. Managing Resources of a Limited Planet. Sixth Biennial Meeting. Leipzig, Germany.
BUNDESVERBAND DER DEUTSCHEN ENERGIE- UND WASSERWIRTSCHAFT E.V. (BDEW) (2013). Die 10 größten deutschen Stromversorger 2003 bis 2010, archived at: http://www.webcitation.org/6orIjo0p2.
BUURMA, J., Hennen, W., & Verwaart, T. (2017). How Social Unrest Started Innovations in a Food Supply Chain. Journal of Artificial Societies and Social Simulation, 20(1). 8: https://www.jasss.org/20/1/8.html. [doi:10.18564/jasss.3350]
CACIOPPO, J. T., & Petty, R. E. (1982). The need for cognition. Journal of Personality and Social Psychology, 42(1), 116–131.
CIALDINI, R. B., Kallgren, C. A., & Reno, R. R. (1991). 'A Focus Theory of Normative Conduct: A Theoretical Refinement and Reevaluation of the Role of Norms in Human Behavior'. In Mark P. Zanna (Ed.), Advances in Experimental Social Psychology (pp. 201–234). Academic Press.
COHEN, J. (1960). A Coefficient of Agreement for Nominal Scales. Educational and Psychological Measurement, 20(1), 37–46.
COLLINS, A. J., Seiler, M. J., Gangel, M., & Croll, M. (2013). Applying Latin hyper-cube sampling to agent-based models. International Journal of Housing Markets and Analysis, 6(4), 422–437. [doi:10.1108/IJHMA-Jul-2012-0027]
CONGALTON, R. G. (1991). A review of assessing the accuracy of classifications of remotely sensed data. Remote Sensing of Environment, 37(1), 35–46.
DA FONSECA FEITOSSA, F. (2010). Urban segregation as a complex system - an agent-based simulation approach. Bonn, Germany.
DUNN, A. G., & Gallego, B. (2010). Diffusion of Competing Innovations: The Effects of Network Structure on the Provision of Healthcare. Journal of Artificial Societies and Social Simulation, 13(4), 8: https://www.jasss.org/13/4/8.html.
ELBERS, M., Ernst, A., Krebs, F., Holzhauer, S., & Klemm, D. (2009). LARA: A Lightweight Architecture for boundedly Rational Citizen Agents. In B. Edmonds, N. Gilbert (eds.), European social simulation association (Eds.), Proceedings of ESSA'09. Guildford.
ELEKTRIZITÄTSWERKE Schönau (EWS) (2013). Anonymized customer data time series.
EPSTEIN, J. M. (2008). Why Model? Journal of Artificial Societies and Social Simulation, 11(4), 12: https://www.jasss.org/11/4/12.html.
EPSTEIN, J. M., & Axtell, R. (1996). Growing artificial societies: Social science from the bottom up. Complex adaptive systems. Washington, DC: Brookings Institute.
ERNST, A. (2014). 'Using Spatially Explicit Marketing Data to Build Social simulations'. In A. Smajgl & O. Barreteau (Eds.), Empirical Agent-Based Modelling - Challenges and Solutions (pp. 85–103). New York, NY: Springer New York. [doi:10.1007/978-1-4614-6134-0_5]
ERNST, A., & Briegel, R. (2016). A dynamic and spatially explicit psychological model of the diffusion of green electricity across Germany. Journal of Environmental Psychology.
ERNST, A., & Spada, H. (1993). 'Modeling Actors in a Resource Dilemma: A Computerized Social Learning Environment'. In D. Towne, T. de Jong, & H. Spada (Eds.), NATO ASI Series. Simulation-Based Experiential Learning (pp. 105–120). Springer Berlin Heidelberg. [doi:10.1007/978-3-642-78539-9_8]
FRIEGE, J., Holtz, G., & Chappin, É. J. (2016). Exploring Homeowners’ Insulation Activity. Journal of Artificial Societies and Social Simulation, 19(1), 4: https://www.jasss.org/19/1/4.html.
GELLRICH, A. (2016). Von der Minderheit zur Mehrheit? Psycho-soziale Ein-flüsse bei der Verbreitung klimaschonender Innovationen. Unpublished dissertation. Kassel: University of Kassel.
GÖCKERITZ, S., Schultz, P. W., Rendón, T., Cialdini, R. B., Goldstein, N. J., & Griskevicius, V. (2010). Descriptive normative beliefs and conservation behavior: The moderating roles of personal involvement and injunctive normative beliefs. European Journal of Social Psychology, 40(3), 514–523.
GRANOVETTER, M. S. (1973). The Strength of Weak Ties. American Journal of Sociology, 78(6), 1360. [doi:10.1086/225469]
GREENPEACE ENERGY eG (2013). Geschäftsbericht 2012, from http://www.greenpeace-energy.de/fileadmin/docs/geschaeftsberichte/Greenpeace_Energy_Geschaeftsbericht2012.pdf.
GRIMM, V., Berger, U., Bastiansen, F., Eliassen, S., Ginot, V., Giske, J., et al. (2006). A standard protocol for describing individual-based and agent-based models. Ecological Modelling, 198(1–2), 115–126. [doi:10.1016/j.ecolmodel.2006.04.023]
GRIMM, V., Berger, U., DeAngelis, D. L., Polhill, J. G., Giske, J., & Railsback, S. F. (2010). The ODD protocol: A review and first update. Ecological Modelling, 221(23), 2760–2768.
GRÖGER, M. (2011). Lifestyles and Their Impact on Energy-Related Investment Decisions. Low Carbon Economy, 02(02), 107–114. [doi:10.4236/lce.2011.22014]
HEISTER, J. (2014). Agentenbasierte Analyse der Informationsdiffusion in empirisch fundierten sozialen Netzwerken. Unpublished Bachelor Thesis. Kassel: Center for Environmental Systems Research, University of Kassel.
HOLZHAUER, S. (2016). Dynamic social networks in agent-based modelling - analysing increasingly detailed approaches of network initialisation and network dynamics. Unpublished dissertation. Kassel: University of Kassel.
HOLZHAUER, S., Krebs, F., & Ernst, A. (2013). Considering baseline homophily when generating spatial social networks for agent-based modelling. Computational and Mathematical Organization Theory, 19(2), 128–150.
HOLZHAUER, S. (2015). LARA - Lightweight Architecture for boundedly Rational Agents - LARA. Retrieved March 10, 2017, from http://lara-framework.sourceforge.net/.
JANSSEN, M. A., & Ahn, T. K. (2006). Learning, signaling, and social preferences in public-good games. Ecology and Society, 11(2).
KIESLING, E., Günther, M., Stummer, C., & Wakolbinger, L. (2012). Agent-based simulation of innovation diffusion: a review. Central European Journal of Operations Research, 20(2), 183–230. [doi:10.1007/s10100-011-0210-y]
KREBS, F. (2015). Towards an empirical validation of spatial patterns of simulated innovation diffusion. Proceedings of the Eleventh Artificial Economics Conference, Porto, 2015.
KREBS, F. (2016). Heterogeneity in individual adaptation action: Modelling the provision of a climate adaptation public good in an empirically grounded synthetic population. Journal of Environmental Psychology. [doi:10.1016/j.jenvp.2016.03.006]
KREBS, F., & Ernst, A. (2017). A spatially explicit agent-based model of the diffusion of green electricity: Model setup and retrodictive validation. In Jager, W., Verbrugge, R., Flache, A., de Roo, G., Hoogduin, L. & Hemelrijk, C. (eds.): Advances in Social Simulation 2015, Cham: Springer International Publishing, S. 217–230.
KREBS, F., Holzhauer, S., & Ernst, A. (2013). Modelling the Role of Neighbourhood Support in Regional Climate Change Adaptation. Applied Spatial Analysis and Policy, (6), 305–331. [doi:10.1007/s12061-013-9085-8]
LEE, J.-S., Filatova, T., Ligmann-Zielinska, A., Hassani-Mahmooei, B., Stonedahl, F., Lorscheid, I., et al. (2015). The Complexities of Agent-Based Modeling Output Analysis. Journal of Artificial Societies and Social Simulation, 18(4), 4: https://www.jasss.org/18/4/4.html.
LICHTBLICK SE (2014, May 23). Yearly customer numbers of Lichtblick for Germany from 2004-2013 (Email).
LINDENBERG, S., & Steg, L. (2007). Normative, Gain and Hedonic Goal Frames Guiding Environmental Behavior. Journal of Social Issues, 63(1), 117–137.
MAHAJAN, V., Muller, E., & Bass, F. M. (1990). New Product Diffusion Models in Marketing: A Review and Directions for Research. Journal of Marketing, 54(1), 1–26. [doi:10.2307/1252170]
MAHAJAN,, V., & Peterson, R. A. (1979). Integrating time and space in technological substitution models. Technological Forecasting and Social Change, 14(3), 231–241.
MANSON, S. M. (2005). Agent-based modeling and genetic programming for modeling land change in the Southern Yucatán Peninsular Region of Mexico. Agriculture, Eco-systems & Environment, 111(1–4), 47–62. [doi:10.1016/j.agee.2005.04.024]
MESSICK, D. M., & Brewer, M. B. (1983). Solving social dilemmas: a review. In L. Wheeler & P. Shaver (Eds.), Personality and social psychology review (pp. 11–44). Beverly Hills, CA: Sage Publications Inc.
MICROM (2015). Microm Consumer Marketing. Retrieved March 10, 2015, from http://www.microm-online.de/zielgruppe/strategische-zielgruppen/microm-geo-milieusr/.
NATURFREUNDE DEUTSCHLANDS (2016). Atomausstieg selber machen | Archiv | Wechseln Sie weiter! | NaturFreunde Deutschlands | Verband für Umweltschutz, sanften Tourismus, Sport und Kultur. Retrieved July 01, 2016, from http://www.naturfreunde.de/archiv-atomausstieg-selber-machen, Archived at: http://www.webcitation.org/6orLOa2md.
Naturstrom AG (2014, April 15). Yearly customer numbers of Naturstrom for Germany from 2004-2013 (Email).
NOLAN, J. M., Schultz, P. W., Cialdini, R. B., Goldstein, N. J., & Griskevicius, V. (2008). Normative Social Influence is Underdetected. Personality and Social Psychology Bulletin, 34(7), 913–923.
NORTH, M. J., Collier, N. T., Ozik, J., Tatara, E. R., Macal, C. M., Bragen, M., & Sydelko, P. (2013). Complex adaptive systems modeling with Repast Simphony. Complex Adaptive Systems Modeling, 1(1), 3. [doi:10.1186/2194-3206-1-3]
ORMEROD, P., & Rosewell, B. (2009). 'Validation and Verification of Agent-Based Models in the Social Sciences'. In F. Squazzoni (Ed.), Epistemological Aspects of Computer Simulation in the Social Sciences, Second International Workshop, EPOS 2006, Brescia, Italy, October 5-6, 2006, Revised Selected and Invited Papers. Lecture Notes in Computer Science. (pp. 130–140). Springer Berlin / Heidelberg.
RAI, V., & Henry, A. D. (2016). Agent-based modelling of consumer energy choices. Nature Climate Change, 6(6), 556–562. [doi:10.1038/nclimate2967]
ROBIN WOOD (2013). Robin Wood Ökostrom-Wechsel Jetzt. Retrieved July 01, 2016, from http://www.robinwood.de/Oekostrom.55.0.html, archived at: http://www.webcitation.org/6orLnOFj9.
ROGERS, E. M. (1962). Diffusion of innovations. New York, NY: Free Press.
RYAN, B. & Gross, N. (1943). The diffusion of hybrid seed corn in two Iowa communities. Rural Sociology, 8(1), 15–24.
SCHWARZ, N., & Ernst, A. (2009). Agent-based modeling of the diffusion of environmental innovations - An empirical approach. Technological Forecasting and Social Change, 76(4, Sp. Iss. SI), 497–511. [doi:10.1016/j.techfore.2008.03.024]
SERVICEPLAN (2010). Stromanbieter - Bekanntheit 2010 | Umfrage. Retrieved February 16, 2017, from https://de.statista.com/statistik/daten/studie/77048/umfrage/bekanntheit-der-stromanbieter-in-2010/, archived at: http://www.webcitation.org/6orMEom3J.
SINUS SOCIOVISION GmbH (2007). Die Sinus-Milieus® in Deutschland 2007. Retrieved April 09, 2009, from http://www.sinus-sociovision.de/2/2-3-1-1.html.
SOBOLL, A., Elbers, M., Barthel, R., Schmude, J., Ernst, A., & Ziller, R. (2011). Integrated regional modelling and scenario development to evaluate future water demand under global change conditions. Mitigation and Adaptation Strategies for Global Change, 16(4), 477–498.
SOPHA, B. M., Klöckner, C. A., & Hertwich, E. G. (2013). Adoption and diffusion of heating systems in Norway: Coupling agent-based modeling with empirical research. Environmental Innovation and Societal Transitions, 8(0), 42–61. [doi:10.1016/j.eist.2013.06.001]
SORDA, G., Sunak, Y., & Madlener, R. (2013). An agent-based spatial simulation to evaluate the promotion of electricity from agricultural biogas plants in Germany. Ecological Economics, 89, 43–60.
STIFTUNG WARENTEST (2011). Ökostrom - Alles Öko oder was? - Special - Stiftung Warentest. Retrieved July 01, 2016, from Stiftung Warentest: https://www.test.de/Strom-Der-Wechsel-lohnt-1132700-1132740/.
TROITZSCH, K. G. (2004). 'Validating Simulation Models'. In G. Horton (Ed.), Networked simulations and simulated networks. 18th European Simulation Multiconference, June 13th - 16th, 2004 (pp. 265–270). Erlangen: SCS Publ. House.
VERIVOX (2013). Comparison of German electricity prices. Retrieved March 21, 2013, from http://www.verivox.de/.
WASSERMAN, S., & Faust, K. (2009). Social network analysis: Methods and applications (19th ed.). Structural analysis in the social sciences: Vol. 8. Cambridge: Cambridge Univ. Press.
WATTS, C., & Gilbert, N. (2014). Simulating Innovation: Computer-based Tools for Rethinking Innovation. Cheltenham: Edward Elgar. [doi:10.4337/9781783472536]
WINDRUM, P., Fagiolo, G., & Moneta, A. (2007). Empirical Validation of Agent-Based Models: Alternatives and Prospects. Journal of Artificial Societies and Social Simulation, 10(2), 8: https://www.jasss.org/10/2/8.html.
Zhang, T., & Nuttall, W. J. (2011). Evaluating Government's Policies on Promoting Smart Metering Diffusion in Retail Electricity Markets via Agent-Based Simulation. Journal of Product Innovation Management, 28(2), 169–186. [doi:10.1111/j.1540-5885.2011.00790.x]