Introduction
Natural disasters (earthquakes, tsunamis, snow storms, bushfires, floods, etc) cause many victims every year. It is particularly important, in order to decrease the number of victims, to prepare the population and the decision-makers in advance so that they can react appropriately when a crisis occurs. In this paper we are interested in the bushfires in the state of Victoria in Australia. The state policy is "Prepare, stay and defend, or leave early", so the population is given a choice between: evacuating early, before fire reaches their area of residence, because "many people have died trying to leave at the last minute" (Country Fire Authority 2014); or stay and defend their house, only if very well physically and mentally prepared. In both cases, the decision must be made and a plan prepared well in advance. But in the summer 2009, serious bushfires devastated a part of Victoria, culminating on the Black Saturday 7th February when 173 people died, despite all efforts at raising awareness. Several reports (Teague et al. 2009a; McLennan & Elliott 2011) have tried to explain the reasons for this heavy death toll and have identified inconsistencies in behaviour (the population does not react as expected by decision-makers), in information (received information is not always considered as relevant by the population), and in communication means (inefficient, specifically information broadcast).
We focus on the discrepancy between the population behaviour as expected by the decision-makers (that determines what information is sent) and their actual behaviour (that determines what information they would need or find relevant). We propose to explain this mismatch by a gap between objective and subjective values of two aspects: risk, and ability to control it. Decision makers tend to predict behaviour based on objective values, while each resident behaves based on their own, necessarily biased, subjective values.
Our approach is agent-based modelling and simulation (ABMS), where autonomous entities (agents) represent the human residents. ABMS is the classical approach when focusing on individual behaviours, or heterogeneous capabilities and motivations among the population. But the originality of our approach is to have identified the main behavioural patterns from actual survivors' testimonies (Teague et al. 2009a). This is a very important point because we want to simulate actual behaviours as opposed to expected, best or prescribed behaviours.
The paper is structured as follows: we first describe our analysis of witness statements (Section 2), and how we used those to design our conceptual model of population behaviour, that we show to be consistent with psychology (Section 3). We then describe the implementation of our model on the GAMA simulation platform (Section 4) and its evaluation against behaviour data (Section 5). We conclude (Section 6) with a discussion of these results, comparison with related works, and future directions of research.
Analysis of behaviour data
Methodology
There are 2 main difficulties in building a realistic agent-based model of human behaviour: finding the right balance between model descriptivity (realistic enough to accurately describe and generate believable human behaviour for each agent) and complexity (yet simple enough for its results to be easily understandable) (Edmonds & Moss 2005;Adam & Gaudou 2016); and finding and exploiting data to inform the model.
To solve the problem of data, we chose the 2009 Victorian bushfires as a case study because of the huge amount of available data about the population behaviours: 100 witness statements (Teague et al. 2009b), 86 police hearings about circumstances of deaths (Teague et al. 2009d), and extensive statistics about the fatalities (Teague et al. 2009c). As a compromise between descriptivity and complexity, we chose to model the agents with a finite state architecture (their behaviour depends on which state they are currently in, and transitions between states depend on their attributes). This appears to fit well with the testimonies that we analysed to design our model.
In the absence of appropriate tools and methodologies to handle such unformatted data, we manually analysed the interviews to extract relevant information of several types. First, we listed the domain actions and behavioural states mentioned by the interviewees, to design the finite state machine. Second, we focused on extracting factors that could explain seemingly irrational behaviour, and short-listed those that appeared most frequently. Third, we hypothesised empirical behaviour rules to explain the precise impact of those factors, by matching them with known theories from the psychological literature. For space reasons, below we only list some extracts that best illustrate each possible explanation found, and discuss in more details how we exploited them to design and inform our model.
Witness statements and statistics
We started from interviews gathered by the 2009 Victorian Bushfires Royal Commission (VBRC) after the Black Saturday bushfires, among 100 residents of the bushfire affected areas (Teague et al. 2009b). We discuss in this section some particularly relevant extracts that illustrate various types of discrepancy between expected and observed behaviour: under-estimation of danger, passivity, denial, over-estimation of capabilities, etc. All the quotes below are taken from this report (Teague et al. 2009b).
Under-estimation of danger
Reports state that "among those who died, some misinterpreted the information they had received, not realising how little time they had to respond or how soon the fire would reach them". As a result they did not have enough time to implement their fire plans. Even people who did not plan to defend still found themselves forced to when surprised by the fire. For instance, this father reports how he evaluated danger while preparing his property with his son, intending and expecting to leave safely well before the fire reached it.
"When we headed up to [the] property, there was just a little fire at the bottom of the hills and I didn't think there were any major dramas. [...] We could see the smoke coming up over that ridge. I wasn't too worried at that stage as the smoke was still two valleys away. [...] We watched the fire come up to the communications tower. We could see that they were fighting the fire really hard up there to stop it getting to the tower. We could see helicopters and bulldozers. [...] When I saw them knock the fire off the top of the hill I was quietly confident that we were going to be okay then. [...] As soon as I saw the smoke I decided it would be too dangerous to drive the 5km bush track out. I then prepared to face the fire."Another reason for a wrong evaluation of danger comes from sudden and unexpected wind changes, a "major cause of the deaths that resulted from the 7 February bushfires".
Over-estimation of capabilities
Many residents had over-estimated their ability to face the fires, and were unaware and unprepared.
"Although I had never really given it much consideration, I suppose that my fire plan always consisted of staying and defending my property. [...] Nothing prepared me for this bushfire. Although I never thought about leaving, I now know that my decision to stay and defend was not a well-thought-out decision and that I was very underprepared. In particular, I was psychologically unprepared to fight a bushfire. I simply did not have any idea what the reality of facing a fire would be. [...] Most people, including myself, just did not understand what it would really be like, facing a fire coming straight at you."Some were well-prepared physically but ignored the psychological preparation also needed to face such a strong fire.
"Despite saving our lives and our house, I don't think I would ever stay and defend again. We were physically very well prepared [...] but I don't think anything can prepare you for the psychological impact of facing the inferno that hit us on Black Saturday."Others did not update their perceived ability with their current condition (age, illness), like this woman reporting how her husband insisted on defending their house despite now being old and unable to do so anymore.
"[He] was in Marysville during the 1939 bushfires and fought the fire from the roof of our old house and helped to successfully save that house. [He] also spent many years working for the Forestry Commission and one of his jobs there was to go to different places to fight fires. During his younger years, [he] was a very fit and strong man. [...] During the last few years of his life, [his] health deteriorated, [...] he had a degenerative spinal disease which affected his mobility. [He] was a proud man and [...] having been such a good sportsman, he found his loss of mobility very difficult to accept."Reports confirm that "most of those who died did not, and often could not, respond appropriately to the risk that the bushfires presented for them on 7 February"; in particular 30% of fatalities occurred in undefendable properties.
Passivity
Many people stayed passive in front of fire until it was too late, therefore feeling as if everything went too fast. It was reported (Teague et al. 2009c) that 69% of the fatalities were "passively sheltering" when they died.
For instance this woman reports how she was fascinated by the fire and could not stop watching it.
"This was not a conscious decision, but I was standing just outside the house watching for flames and when they came, they came so suddenly that I just didn't have time to do anything or than stand and watch. It feels almost sacrilegious to say this, but I found the fire fascinating and strangely beautiful. The contrast of the black and red was stunning."
Individual differences
Different individuals can have different perceptions of risk and motivations, resulting in various emotions, and a negotiation of the decision. On average, men prefer to defend their house, while women want to leave but often end up staying with their partners because they are reluctant to leave them behind (Teague et al. 2009c). Witness statements illustrate the impact of relationships, and the resulting negotiation and emotions.
For instance, all four members of a family died in their house because they could not agree on leaving.
"She was determined to leave, but [her husband] wouldn't leave his parents. [His father] wouldn't go, [his mother] wouldn't leave [his father], [her husband] wouldn't leave his parents."In another statement, the wife ended up escaping alone to safety because she could not convince her husband to leave (he died in their house).
"I continued to talk to [my husband] and tried several times to persuade him to leave, but he would not budge. I could smell the fire, I could hear the fire and I could see it with my own eyes. But I couldn't get my husband to accept that it was coming. He just sat and sat and sat. [...] I decided that I could not stand it any longer. I was feeling very anxious and angry with [my husband]. I kept saying "hurry up, come on we've got to go". The fire seemed to be coming closer. I could hear the fire crackling. After too much of that I thought "I'm not staying here to burn". So I walked out the gate and I left the house on foot. [...] When I left the house, I had no idea where I was going – the only thing I can remember is that I wanted to get out. I was not thinking clearly because I was so annoyed with [him] and I was also feeling terribly guilty about leaving him. I still have this guilty feeling because I survived and he didn't. Should I have stayed and if I had, what could I have done?"This last statement also illustrates the well-known survivor's guilt, which we do not address yet.
External motivations to defend
People can have additional motivation to defend their house, for instance for financial or emotional reasons. Some residents report having stored expensive equipment or personal belongings.
"I also had many belongings stored on [the] property in four sheds. I am a hoarder by nature [...]. My belongings included the fit out of a cafe that I used to own, $50,000 - $60,000 worth of tools [...]. I also stored family photos and personal papers in the sheds."Others report drawing additional income from cattle or plantations.
"[We] are both retired teachers. The olive grove had been planted in 1999 and the trees were at full production. Both the cattle and the olive grove provided additional income for our retirement."In both cases they tried to protect their belongings from the fire.
Summary
In conclusion, the manual analysis of these statements highlighted the essential role of some human factors in decision making, that should therefore be included in our model. These factors are: a discrepancy between actual danger and perception of risks; a discrepancy between actual abilities and confidence in one's abilities; inter-individual differences in initial motivations for defense (e.g financial) or escape (e.g risk aversion); inter-individual differences in awareness of and knowledge about fires. It also showed that residents go through different stages based on these factors (unaware, passive, preparing, defending, escaping...); they can stay in each stage for a varying amount of time, with many residents surprised by the fire while still indecisive or passive (58% of fatalities had made no preparation at all, neither for leaving nor for staying); and they can die in any of those stages.
Conceptual model of population behavior
Behaviour model: finite state machine
The manual analysis of these interviews clearly showed that the residents base their decisions on subjective (and possibly biased) representations of their environment and of themselves: a subjective perception of risk (that can be wrong, biased, under-estimated), and a subjective estimation of their abilities to deal with it or escape it (that can be wrong, biased, over-estimated). Residents can also be too attached to a predefined plan. All these factors lead to a more risky behaviour than the rational one as expected by the authorities, that would be based on an objective perception of risk and self abilities.
In order to highlight the role of these subjective, irrational determinants of the decisions and behaviours of each resident, we need a model descriptive enough to capture these factors, but not so complex that the results will not be understandable. Our choice is to model the population as heterogeneous agents, each having their own values of attributes to trigger possibly different behaviours in a similar situation. We chose a finite-state-machine architecture to capture the different stages observed in the interviews (unaware of the fires, indecisive, preparing, etc) and the transitions between them.
Residents attributes and actions
In order to provide a conceptual model, we had to make some simplifications and abstract a limited number of relevant attributes and actions to be represented.
Residents are represented by agents with their own individual attributes, as identified from the interviews. They have in particular: physical attributes (health, injuries, objective danger, objective abilities), and cognitive attributes (subjective evaluation of their capabilities and of danger, motivations).
Agents can perform various actions as stated by the interviewees: observe the fires, prepare their house and themselves in various ways, fight the fire, escape their house towards a safe shelter. Their choice of action depends on their perceived and appraised (a.k.a. subjective) danger and abilities, and on their motivation. For instance, a resident who is motivated to defend their house, and believes they are capable of doing so, will probably choose to stay and defend. However, their success when performing actions depends on their actual ability (a.k.a. objective ability) and on the actual danger (a.k.a objective danger). For instance, a resident who believes he is capable of defending might be over-estimating his ability, and the success or failure of his defense depends on his actual ability rather than on his estimation of it.
States and transitions of the FSM
A finite-state machine (FSM) architecture describes the behaviour of an agent in terms of different possible states; the agent starts in the initial state, and has one single current state at each time. Each state is associated with: the actions performed by the agent while in this state; and the transition conditions to move towards other states.
The architecture of the residents agents in our model is a finite-state machine (cf Figure 1). Its states and transitions are inspired by the stages observed in the interviews, and are as follows:
- \(\texttt{Unaware}\): initially the agent is (rightly or wrongly) unaware of any danger, and does nothing; agents can become aware (transition to \(\texttt{Aware Indecisive}\)) by spotting fires around them (see flames, smell smoke...);
- \(\texttt{Aware Indecisive}\): the agent is aware of some fires but has not yet made a decision about how to react; agents stay indecisive for a varying amount of time, before choosing to either defend their house (transition to \(\texttt{Preparing to defend}\)) or escape (transition to \(\texttt{Preparing to escape}\));
- \(\texttt{Preparing to escape}\): the agent has decided to leave and starts preparing, until either ready (transition to \(\texttt{Escaping}\), or surprised by the fire and forced to escape before being ready (transition to \(\texttt{Escaping}\) as well), or blocked by the fire and forced to stay (transition to \(\texttt{Preparing to defend}\));
- \(\texttt{Escaping}\): the agent is evacuating towards the closest shelter; travel efficiency depends on objective abilities; injuries can be received from fires on the way. Unless it dies during travel due to fire burns (transition to \(\texttt{Dead}\)), its next state will be \(\texttt{Safe}\) when reaching the shelter;
- \(\texttt{Preparing to defend}\): the agent has either decided to defend, or was forced to stay because the fire blocks escape; it prepares its house and itself until the fire is close enough to be fought, which triggers the transition to \(\texttt{Defending}\);
- \(\texttt{Defending}\): the agent is actively fighting the fire around its house; when that fire is extinguished, the agent transitions back to \(\texttt{Preparing to defend}\) until another fire comes; if fighting the fire makes it reevaluate the danger or its own capabilities and that evacuation becomes more urgent, the agent transitions directly to \(\texttt{Escaping}\);
- \(\texttt{Safe}\): the agent is (and will stay) in a shelter, it cannot leave it, and cannot be injured anymore.
Two more states are particular as they can be reached from all the other states:
- \(\texttt{Dead}\): final state of agents which have been killed by the fire (from whatever other state);
- \(\texttt{Survivor}\): final state of all agents that did not die during the fires (e.g successful defenders, lucky passive, and all sheltered residents), reached at the end of the simulation.
Evaluation of the conceptual model
This simple conceptual model is sufficient to capture the discrepancies highlighted by the data. Indeed the objective value of danger influences injuries and damage, and the objective value of capability influences the success of actions. But these objective values are inaccessible to the agents, whose decisions are based on their subjective values of danger and abilities, and on their motivations. This therefore explains the observed difference between expected rational behaviour (based on objective danger and ability) and actual behaviour (based on subjective danger and ability). Before implementing our conceptual model, we evaluate in the next paragraph its consistency with psychological theories of human behaviour.
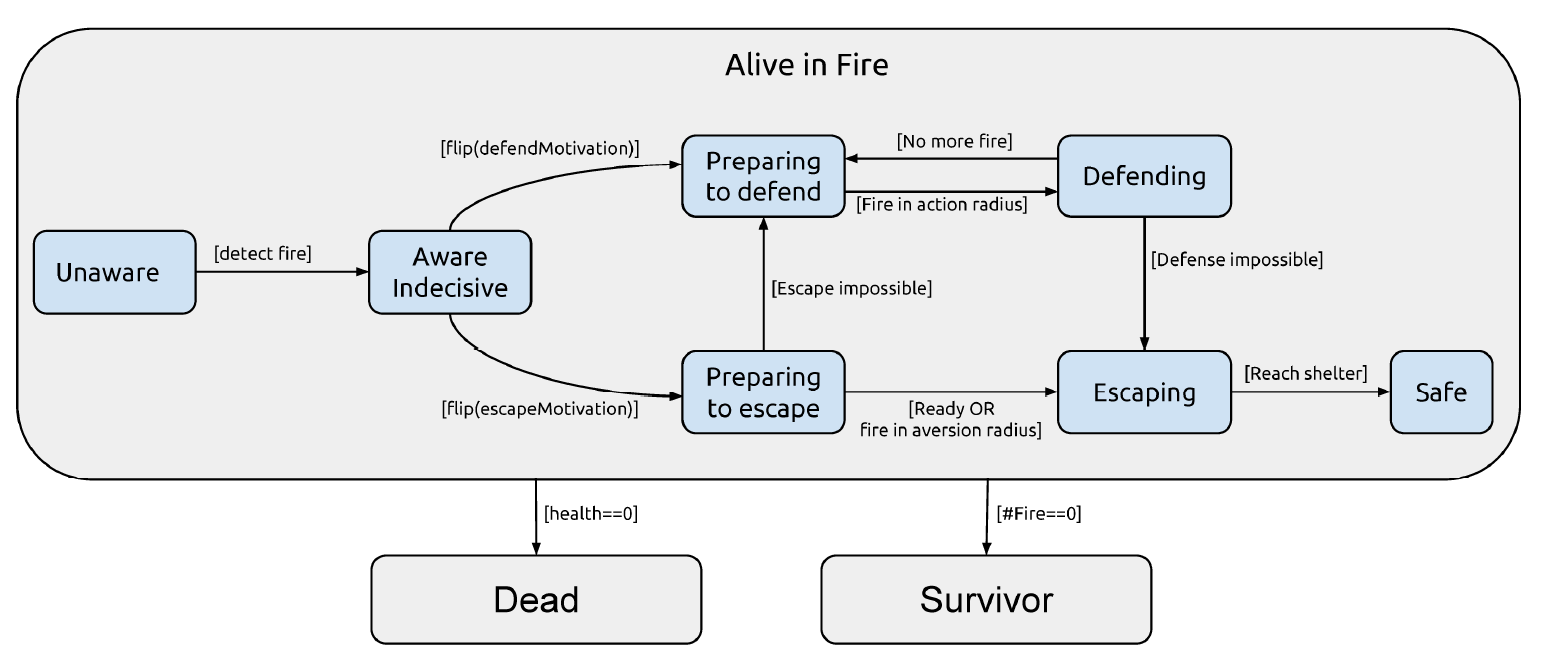
Comparison with the psychology of stress
Our model is in agreement with Lazarus' theory of stress (Lazarus 1991; Lazarus & Folkman 1984), making a distinction between two simultaneous processes:
- Primary (or demands) appraisalconsists in evaluating the significance of the situation for the individual (good, stressing, or irrelevant); this demands appraisal matches our subjective danger, which is an evaluation of the dangerousness of the situation;
- Secondary (or resources) appraisal consists in evaluating the individual's capability (skills, social and material support, resources) to cope with the stressor; this resources appraisal matches our subjective ability, which is an evaluation of the ability to deal with the fire.
Comparison with cognitive bias theories
Behaviours reported in the statements are consistent with known cognitive bias (Yudkowsky 2008; Kinateder et al. 2014), in particular the confirmation bias, a tendency to give more credit to information consistent with one's beliefs or motivation, and to discard inconsistent cues (e.g interpreting the presence of firemen as a sign that everything is safe, or denying the reality of the fire when wanting to stay). In other words, motivation tends to bias interpretation: people focus more on cues that support their current motivation, and less on cues that would contradict it, the extreme case being denial as illustrated above. Our model allows to capture this confirmation bias, because the motivation attributes play a role in the computation of subjective danger and subjective ability.
Many other biases were also found in the interviews, which shows that modelling them is particularly relevant for simulating human behaviour in crisis situations. For instance:
- the anchoring effect: an excessive focus on the first information received, preventing residents from changing their mind after making an initial decision;
- the hindsight bias, making people believe afterwards that they could have predicted the events (and that they will next time), and making them feel guilty for not having predicted them;
- the bandwagon effect: doing and believing the same as others around;
- the planning fallacy: underestimating the time something will take, for instance preparing;
- the sunk cost fallacy: refusing to abandon an action because of having already invested in it (making the goal less likely to change as more actions have been performed to reach it).
Implemented model of behaviour in bushfires
We implemented our conceptual model[1] in version 1.6.1 of the GAMA simulation platform (Grignard. et al. 2013).
Model of environment
For the sake of simplicity, the environment is a grid containing the different types of agents (houses, shelters, fires, and residents). This simplistic environment is not realistic but is sufficient to simulate the residents' decision-making in reaction to fires.
Fire
Very complex and detailed models of fire spreading already exist (Duff et al. 2013), but realistic fire behaviour is not the focus here. Still with the goal of not adding unneeded complexity, we have designed a very simplistic model of fire that is sufficient to trigger and visualise the reactions of the population that we are interested in here. The fire is composed of fire agents (each with a location and an intensity representing its radius of action), having a reflex architecture, i.e.the following reflexes are triggered at each step of the simulation:
- Increase or decrease intensity: probabilities are parameters.
- Propagate to a non-burning neighbour cell, creating a new fire agent. Probability of propagating, and starting intensity of new fires, are parameters.
- Deal damage to buildings in its radius of action (based on its intensity): the amount of damage is picked randomly between 0 and a maximum value, function of intensity and a "damage factor" parameter.
- Deal injuries to residents in its radius of action, also random amount between 0 and the maximum value based on its intensity and an ''injury factor'' parameter. If the person is in their house, the injury is moderated by its resistance weighed by a ''protection factor'' parameter.
- Disappear when its intensity is null.
Houses
The environment initially contains a number (parameter) of houses each inhabited by exactly 1 resident (in future work we plan to consider families and their relationships). Each house is an agent with the following attributes:
- Owner: the resident of that house.
- Resistance: random initial value between 100 and 200 to simulate different solidity, will be increased by preparing, or decreased by fire damage, and offers some protection from fire injuries to its resident.
- Damage: the damage received from fire.
The houses collapse from fire damage when their resistance drops to 0. They then cease to offer protection, and the resident's motivation to defend them also disappears. They stay in the environment as ruins for final visualisation.
Shelters
Shelters are safe places whose location is known by all residents[2]. They offer a total protection from fires (no injuries can be received while in a shelter). Once a resident has reached a shelter, he stays inside until the end of the simulation.
Model of residents
Architecture
We used GAMA finite-state-machine (FSM) architecture for the residents, with the states specified in our conceptual model above: initial state \(\texttt{Unaware}\); states during the fires: \(\texttt{Aware Indecisive}\), \(\texttt{Preparing to defend}\), \(\texttt{Defending}\), \(\texttt{Preparing to escape}\), \(\texttt{Escaping}\), \(\texttt{Dead}\), \(\texttt{Safe}\); final state \(\texttt{Survivor}\), only reached by agents still alive when all fires are stopped.
Attributes
The agents have various attributes that influence their behaviour depending on their value for each individual. There are two main types of attributes: physical and cognitive.
Physical attributes include:
- Current state (initially \(\texttt{Unaware}\), then following the fsm, see (Figure 1).
- House id (each agent is initially in a house, and there is only one agent per house).
- Health: random initial value (between 100 and 200), increased by preparing for fire, decreased when receiving injuries. Agents whose health drops to 0 die[3].
- Injuries: total injuries received from fires, decrease health level and influence decision to escape.
- Location on the grid. This attribute is used to compute and update the distance to the closest fire as the situation evolves.
- Objective danger: dynamic float value (normalised between 0 and 1) computed as a function of the intensity of and distance to the different fires present in the environment; its value is 0 in a shelter.
- Objective defense ability: random static value (float between 0 and 1) impacting the chance to perceive fires in perception radius, and the effect of preparation and defense actions.
- Objective escape ability: random static value (e.g driving vs walking, fitness), impacting accuracy and speed of evacuation actions. The possibility to escape is evaluated by observing the environment and checking if a safe path exists to a shelter.
- Awareness: boolean indicating if the resident is aware (i.e. has perceived at least one fire). The resident also maintains a list of known fires.
- Subjective danger (normalised between 0 and 1) is computed as a function of intensity of and distance to only the known fires. It is biased by motivations (someone motivated to escape will tend to over-estimate danger, while someone motivated to stay will under-estimate it).
- Subjective ability (random float between 0 and 1) is updated during the simulation with the feedback from the resident's actions (time spent defending, effect on fires, number of fires extinguished): success increases subjective ability while failure decreases it. It is also biased by motivation to defend (the more one wants to defend, the more capable they feel).
- Subjective defense ability: initialised by applying a (under- or over-estimation) bias (based on confidence parameter and defense motivation) on objective ability, then updated (rate is a parameter) by observing performance (success or failure of defense actions); influences defense motivation.
- Motivation to escape: float value between 0 and 1 representing a summary of all motivations to (not) escape (risk aversion, protecting children, laziness to leave if not needed, etc). It has a random initial value, and is then updated based on subjective danger, health, and resistance of the house (offering protection). It influences the decision to escape.
- Motivation to defend: float value between 0 and 1 representing a summary of all different motivations to defend (protect one's livelihood, emotional attachment to house, etc). It has a random initial value (e.g. financial reasons, previous experience), and is then dynamically updated based on subjective evaluation of danger and fighting capabilities (feedback from defense actions), health and injuries received, and house status (the more capable and healthy, the more motivated a resident will be). It influences the decision to stay and defend.
Actions
Residents agents can perform the following actions depending on their state:
- Observe fires: action performed by all agents as a reflex at each cycle of the simulation. Their chance of actually detecting a (new) fire depends on their objective ability. Detected fires are added to the resident's list of known fires, and motivations are subsequently updated.
- Prepare for fire: action performed while in \(\texttt{Preparing to defend}\) or \(\texttt{Preparing to escape}\) state. It consists in raising the resistance of the house (to simulate various actions such as watering, weeding, etc) and the agent's health (to simulate the effect of wearing appropriate clothing, etc). The value of the increment is computed based on the agent's objective ability and on a parameter of the resident agents. Success or failure of this action in monitored by the agent and influences its subjective ability.
- Fight fire: action performed while in \(\texttt{Defending}\) state. Its effect is to decrease the intensity of nearby fires by a value based on objective ability and on another resident parameter. The agent monitors success (number of fires extinguish, total intensity decrement) to update its subjective ability, thus reconsidering its motivation over time.
- Escape: action performed while in the \(\texttt{Escaping}\) state. Its effect is to compute and follow a path towards the nearest shelter. The resident's evacuation speed and accuracy depend on its objective escape ability: an agent with a low ability has more chances to take a longer path (to simulate getting lost or not taking the best route), and it moves more slowly along that path (e.g. disabled or injured people). Agents might get injured while escaping if they travel too close to the fire.
User interactivity
With the eventual goal of turning this simulation into a serious game, we have already inserted some level of user interactivity to help them observe the desired behaviours. Our model allows two kinds of interaction with the simulation: direct actions (that modify the state of the simulation at runtime), and setting simulation parameters. Available actions implemented so far in our model are limited to starting new fires (at random locations) and stopping all fires (and thus the simulation).
There are two types of parameters: initialisation parameters (requiring a re-launch to be taken into account), and runtime parameters (whose effects immediately modify the simulation behaviour). The parameters of our simulation allow the user to: initialise the environment as desired (initial number of fires and buildings, etc), make the fire more or less dangerous (growing and propagating quicker, dealing more damage and injuries, etc), and set properties shared by all residents (probability to detect fires in perception radius, bias in updating beliefs, etc).Results and evaluation of the model
In this section we describe the experiments we ran to evaluate our model.
Initialisation of the simulation
The environment is a 50x50 non-torus grid (cf Figure 2), initially containing 2 shelters in the NE and SW corners (blue-green circles) and 200 houses (grey squares) inhabited by 200 residents. Attributes are randomly initialised (health and resistance between 100 and 200; capabilities and motivations between 0 and 1). The simulation starts with 20 medium fires (orange triangles) that then grow and propagate. Burning cells are in red, surrounded by yellow cells (radiant heat, radius equal to the fire intensity); green cells are safe. At runtime[4] the user of the simulation can start random new fires or stop all fires, and can specify 3 categories of parameters concerning: fires (probability to grow or propagate, initial intensity, damage factor, etc); buildings (resistance, protection factor, etc); and residents (confidence bias, perception and action radius, etc).
The residents have 2 colours to visualise their current (outside colour) and previous (centre colour) state in the fsm: dark blue \(\texttt{unaware}\), pink \(\texttt{indecisive}\), orange \(\texttt{Preparing to defend}\), red \(\texttt{defending}\), yellow \(\texttt{Preparing to escape}\), light blue \(\texttt{escaping}\), with an additional nuance of blue grey if escaping before they are ready), dark green \(\texttt{safe}\), black \(\texttt{dead}\). There is no specific colour for the \(\texttt{survivor}\) state as it is reached by all agents still alive when the fire stops.
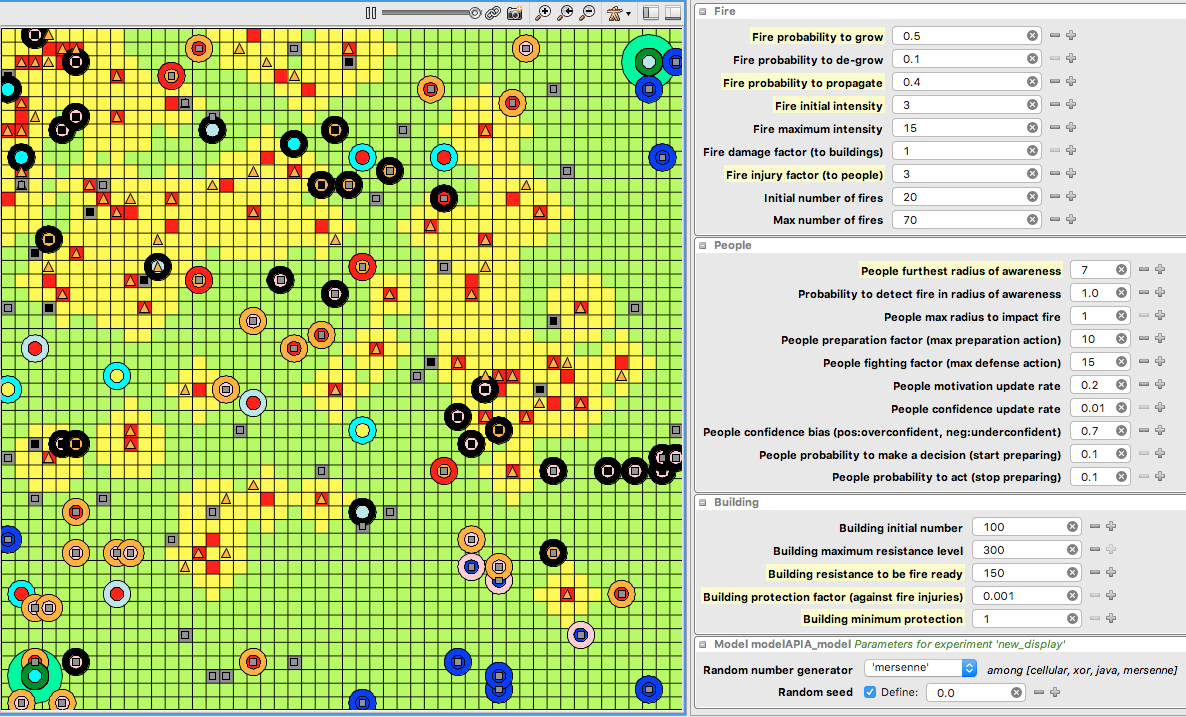
Evaluation methodology
Our model is aimed at the reproduction of realistic human behaviours (and not at realistic initial situation or fire spreading), and should therefore not be evaluated as a whole. This is why we focus here on evaluating the generated human behaviour. We have implemented a tool to track and log the agents' states trajectories: what states they went through, at what cycle, and what were the values of their attributes when making the transition. This allows us to study and explain what happens in the simulation. Thanks to this tool, we can evaluate our model on two axis: correctness, by comparing the generated trajectories with those observed in the real population (profiles of behaviours), and in particular comparing the causes of deaths; and explicative value, by showing the importance of the subjective-objective discrepancy, thus proving the potential of our model to raise decision-makers' awareness of this gap.
Evaluation of the correctness of model
Consistency with behaviour profiles
A report (Rhodes 2014) has established 6 profiles of behaviours in the residents of fire-affected areas: can-do defenders (most determined, experienced, self-confident and skilled, determined to defend); considered defenders (strongly committed to defend, aware of risks, well prepared and trained); livelihood defenders (committed to defend their livelihood, well prepared); threat monitors (not intending to stay in front of a serious threat, nor to leave until necessary, wait and see); threat avoiders (aware of risks an dvulnerability, plan to leave early before any real threat); unaware reactors(unaware of risk, feel unconcerned, no knowledge, preparation or training).
We were able to observe the same profiles of behaviours in our simulation, and to categorise the agents in these profiles based on their logged trajectories. For instance a typical trajectory for a can-do defender is an early perception of the fire (transition to \(\texttt{Indecisive}\)) followed by an immediate decision (transition to \(\texttt{Preparing to defend}\)), an efficient preparation (strong improvement of health and resistance in that state) and defense (big decrease of fire intensity). Also they have a good perception of risks and are able to reconsider their intention (transition to \(\texttt{Escaping}\) when health decreases too much), unlike livelihood defenders who tend to stay on their property no matter what happens. We have then computed average values of the attributes in each category of agents and compared them with the global value on all agents. For instance, we found that can-do defenders have a significantly higher self-confidence, which is in agreement with the definition of the profile.
Consistency with death causes statistics
The VBRC report also provides statistics about the circumstances of the 173 deaths drawn from police hearings and experts reports. In particular they found that 14% died while fleeing (4% in cars, 10% on foot); 69% while "passively sheltering" (as opposed to "actively defending"), possibly after having tried to defend; some died while defending, even when well prepared. In total 30% were taken by surprise by the fire. Figure 3 shows the percentage of deaths from each state in our simulation. With a first manual calibration of our parameters, we obtained the following distribution of causes of death: 47% of the agents died while still passive (indecisive); 19% die while escaping; the others died while preparing to defend (28%) or defending (6%), taken by surprise before they could evacuate. Of course, different models can lay the same results with different underlying mechanisms (this is known as the equifinality problem). However, our conceptual model was designed from qualitative behaviour data and evaluated against psychological theories (see Section 3.8), which helps in ensuring that we get the right distribution of death causes for the right reason, and not only as a result of calibration. In future works we will do a sensitivity analysis to reduce the number of parameters, and do an automatic calibration of our model.
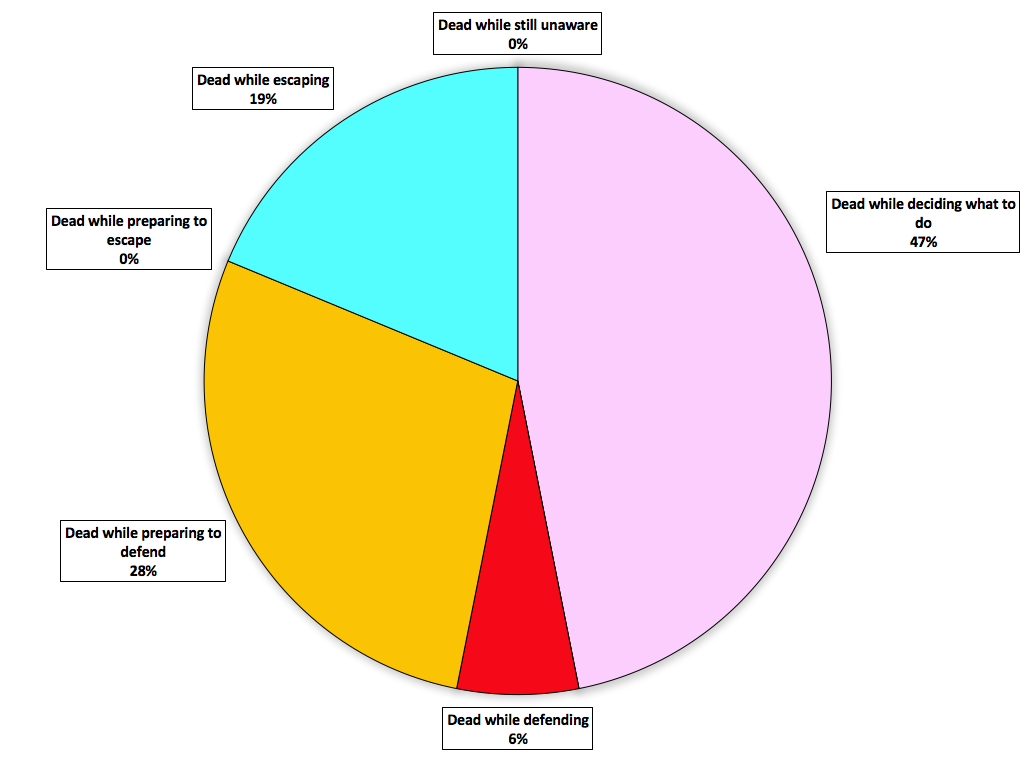
Figure 4 compares the death causes in our simulation with those reported in the 2009 bushfires. The problem is that the categories of death causes considered in the available statistics do not exactly match the states in our simulation, apart from the escaping category (where the numbers are quite close). In particular, 60% of the victims in the real population died while passively sheltering, whether they had made some preparations or not. In our simulation we do not have a ''passive sheltering'' state, so an agent that started actively preparing cannot return to a passive state. As a result, we find less passive victims (47% vs 69%) but these are only the agents that stayed passive all along. On the contrary, we have more active victims because we included in this category both the agents dead while preparing (28%) and those dead while defending (6%). The statistics about the 2009 bushfires only mention ''some'' victims dying while defending (in Figure 4 we extrapolated to show the 17% who died neither while escaping nor while passively sheltering, as being the ones who died while defending). With this difference in categories in mind, the death causes in our simulation are pretty close to the real death causes observed in the 2009 bushfires.
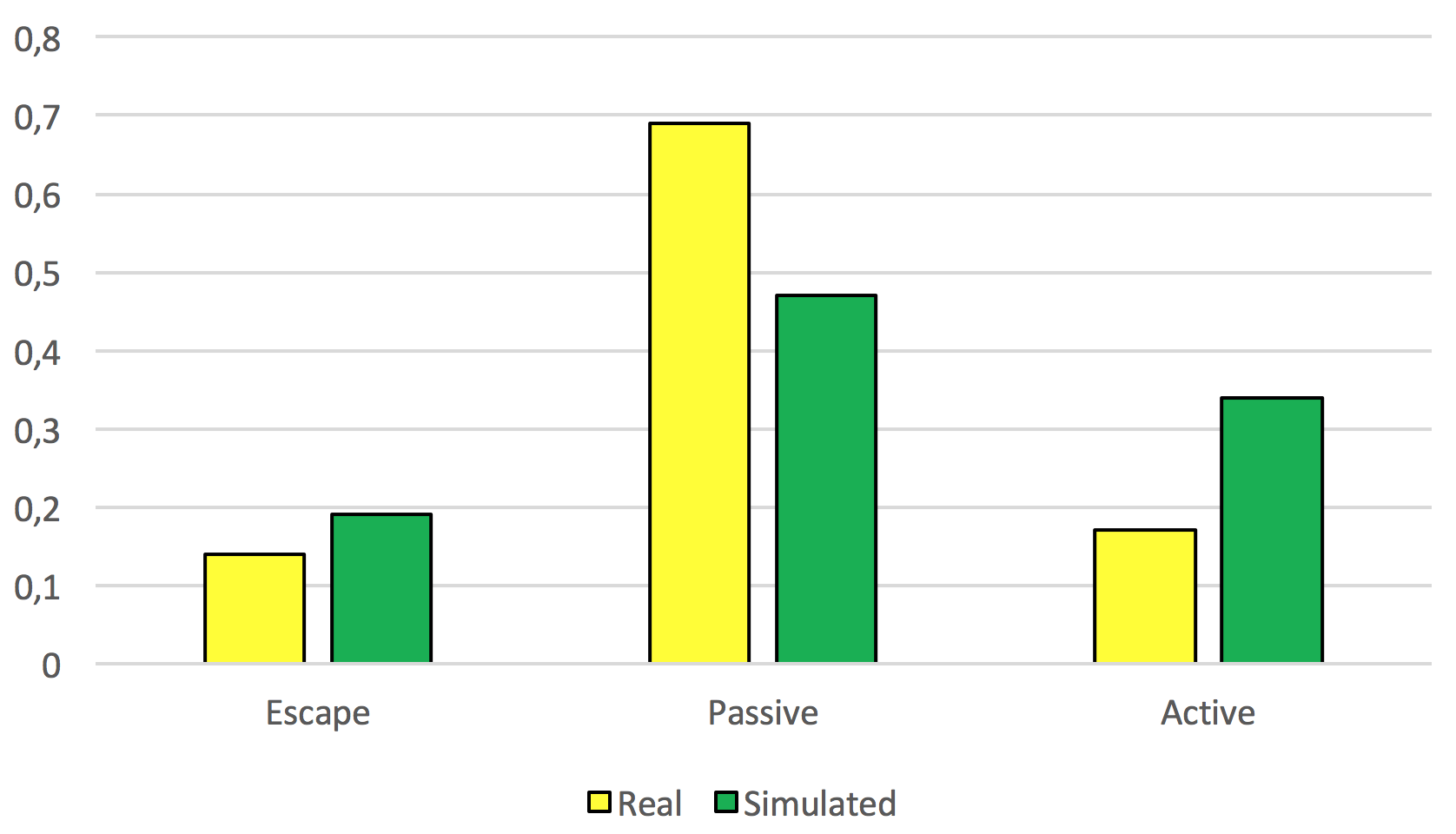
Explicative value of our model
The goal of our model is to raise decision-makers' awareness of the factors determining real population behaviour, as opposed to expected behaviour. Indeed, the statements clearly show that decision-makers expect a "rational" behaviour based on objective values, while people behaved based on their subjective (possibly wrong) values. Our hypothesis was that the discrepancy between these objective and subjective values of danger and capabilities could explain deaths, and should therefore be taken into account. If we are right, we should observe stronger discrepancy in agents who die than in agents who survive.
Figure 5 shows the evolution over time of the average discrepancy between objective and subjective values of danger and capabilities, for alive agents and for dead agents. Note that once dead, agents do not update their values, so the evolution only comes from new agents dying over time.
- Danger discrepancy for dead agents (red) starts at 0 (no death yet) then jumps to a very high value as the first agents die while unaware of (yet real) danger. It then tends to decrease as the agents dying later in the simulation are those who have a lower underestimation of danger.
- Danger discrepancy for alive agents (orange) is always much lower than in dead agents; it also continuously decreases for two reasons: agents with a higher discrepancy die, and those that survive update their perception of danger to tend towards the objective value.
- Ability discrepancy for dead agents (blue) also starts (and stays) higher than for alive agents. It decreases quickly at the start (as agents with higher discrepancy died early), then stays mostly stable.
- Ability discrepancy in alive agents (green) keeps going down, until the last survivors tend to actually underestimate their abilities (this is due to them updating their subjective abilities based on their performance at fighting the fire, which gets worse as the fire keeps intensifying).
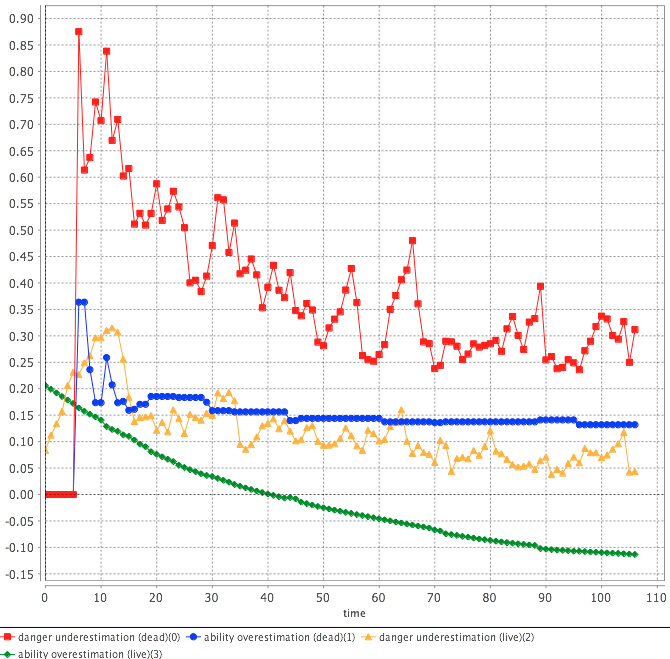
Discussion and conclusion
In this paper we therefore provided a realistic model of population behaviour, based on qualitative data from interviews, consistent with statistics of causes of deaths, and with a high explicative value. It is important to notice that despite being designed to simulate the behaviour of the Australian population in bushfires, this model is relatively generic and could be applied to other contexts. Some aspects are not generalisable because they depend on the particular culture or type of disaster. For instance, the norms at play differ depending on the country (in Australia, people are given a choice to evacuate or stay and defend; in France they are requested to shelter in their house; in other countries they are required to evacuate). The appropriate behaviour in a fire (possibility to save your house from the flames) may not be appropriate in another type of disaster (defending your house can be a valid choice in a hurricane or a flood, but not in a tsunami or earthquake). However, the main aspects highlighted in this work, i.e. the role of cognitive factors in explaining seemingly irrational behaviour, are generalisable to any type of crisis situation. In particular, cognitive biases and a subjective perception of risk often impact decisions in such time-pressured situations Kinateder et al. (2014).
Comparison with related works
Some existing simulations focus on realistic fire behaviour and spreading (e.g Phoenix, Duff et al. 2013), easier to understand in physical terms. On the contrary as far as we know no model provide the same degree of realism for human behaviour. Indeed, many agent-based simulations focus on crowd evacuation in building fires, with often homogeneous reactive agents (e.g social force model (Helbing & Molnar 1995). More complex models have then appeared, integrating emotions (Le et al. 2010; Nguyen et al. 2014 or social relationships (Chu et al. 2014) of agents, but they are usually based on psychological theories. An exception is the work of (Shendarkar et al. 2008) who simulated evacuation under terrorist bombing attacks with BDI agents, and used participative modelling to capture behaviours in a Virtual Reality laboratory. Our work also differs from this main trend as we rely not on theories but on witness statements providing a precise description of people actual behaviour.
Existing simulations often focus on the evacuation of public buildings (e.g airport, Tsai et al. 2011, stadium, or buildings, Rogsch et al. 2014; Ronchi & Nilsson 2013) to inform their design and prevent typical problems (e.g crowding at doors or in stairs), which are not relevant in our case study of bushfires in wide and scarcely populated outdoor areas.
Indeed we can make (in line with Le 2016) a distinction between indoor (e.g stadium, airport, train station...) and outdoor (e.g beach in case of a Tsunami, Nurdin & Yuliana 2012; Nguyen et al. 2012) evacuations. Both are focused on simulating individuals trying to flee from a hazard. But the main specificity of indoor evacuations is that the high density of people and some features of the environment (e.g walls and exit doors size) constrain movement, induce severe congestion issues, decrease evacuation rate and tend to increase the number of victims. As a result, these studies are generally interested in how to improve building layout.
On the contrary, outdoor evacuations are less influenced by environment constraints, as the space for people to move is wider (outdoor evacuations are often at the scale of a district or entire town) (Nguyen et al. 2012). However, in the absence of signals helping people indoors to find their way to the exits, the main issue for people outdoors is to find a way to shelters (Le et al. 2015). Congestion can happen but in different forms: people tend to evacuate thanks to transportation means such as cars (Dawson et al. 2012; Mas et al. 2012), leading to traffic jams. Our study is thus closer to (Le et al. 2015) work, as we consider a scarcely populated environment which avoids cases of congestion.
Another difference is that due to this different scale, outdoor evacuation simulations focus less on complex individual behaviour and more on crowd behaviour (Beck et al. 2014). For instance, Le et al. (2015) attempt to optimize the location of evacuation signs to evacuate a beach during a tsunami. On the contrary, many indoor simulations try to better understand the cognitive factors influencing people evacuation. The most studied factors are emotions (Minh et al. 2010; Johnson 1987) and social bonds (Ta et al. 2015; Chu et al. 2015, 2014). However, we focus on the cognitive factors influencing people evacuation from their home: subjective appraisal of danger and abilities, motivations, cognitive bias. In future work we plan to also add emotions, which are very important in such situations, as shown by the interviews (home attachment, fear, survivor's guilt, etc).
Besides, most of these simulations tend to focus on the evacuation itself, neglecting the pre-evacuation time which is at least as important (Kinateder et al. 2014). On the contrary we did model this decision-making phase, which is even more important in bushfires where people are not evacuating from a public building (where their only motivation is to save their life) but have to abandon their own house to the flames (with an additional contradictory motivation to also save it).
So we differ from the state of the art by providing a simulation of the population behaviour in outdoor bushfires, focusing on individual cognition, and taking into account both pre-evacuation decision-making with contradictory motivations (evacuate or protect house) and evacuation itself.
Future work
Our model relies on simplifying hypotheses and will need to be extended in the future. In particular, the initial situation has exactly one person per house, while statements and reports show the importance of family relationships (several people in the same house making a decision together), and of tourists or visitors who (in addition to their lack of knowledge) have nowhere to go when the fire surprises them outside. Also, we need to model how different sources of information (observation of fire, information on the radio, visits from authorities, phone calls from relatives, etc) with different levels of trustworthiness are combined to evaluate risk and make a decision. Emotions should also be added, in line with the interviews that show their key role in many behaviours.
Our agent architecture is also very simple, tailored to prove our point about the discrepancy between objective and subjective values of danger and ability. In future work we plan to design a BDI (belief, desire, intention) model of behaviour, improving its descriptivity but also its explaining power, since mental attitudes are people's preferred level of abstraction to explain behaviour (Adam & Gaudou 2016). The GAMA platform provides a plugin for implementing BDI agents Taillandier et al. (2016) and emotions Bourgais et al. (2016) based on a BDI formalisation Adam et al. (2009).
Thanks to this high explicative value, this model can serve two complementary purposes. First, we intend to transform it into a serious game which will be a valuable tool for decision-makers to test response and communication strategies on a simulated population, in order to better handle future bushfires; this requires a very realistic human behaviour model to lay valid results. Second, this model will raise awareness about the factors explaining real human behaviour, and therefore encourage modellers to improve their models by considering these factors; this will probably also require the development of new methodologies and tools, advancing the field of agent-based modelling as a result.
Acknowledgements
This work was funded by the Rhône-Alpes region, Grenoble, France under project AGIR SWIFT (Simulations With Intelligence for Fire Training).Notes
- Our GAMA models can be downloaded from OpenABM at https://www.openabm.org/model/5430/version/1/view and from the SWIFT project webpage at https://goo.gl/HvrSls.
- In future works we want each resident to have a different (partial, and possibly wrong) knowledge of the position of existing shelters, to simulate varying degrees of preparation, but this adds a lot of computational complexity to the model.
- Dead agents stay in the grid for visualisation purposes, but cannot act anymore.
- Videos of several example runs can be found at https://goo.gl/MTojB3.
References
ADAM, C. & Gaudou, B. (2016). BDI agents in social simulations: A survey. Knowledge Engineering Review, 31, 207–238 [doi:10.1017/S0269888916000096]
ADAM, C., Herzig, A. & Longin, D. (2009). A logical formalization of the OCC theory of emotions. Synthese, 168(2), 201–248 [doi:10.1007/s11229-009-9460-9]
BECK, E., Dugdale, J., Van Truong, H., Adam, C. & Colbeau-Justin, L. (2014). Crisis mobility of pedestrians: from survey to modelling, lessons from Lebanon and Argentina. In International Conference on Information Systems for Crisis Response and Management in Mediterranean Countries, (pp. 57–70). Springer [doi:10.1007/978-3-319-11818-5_6]
BOURGAIS, M., Taillandier, P. & Vercouter, L. (2016). An agent architecture coupling cognition and emotions for simulation of complex systems. In Social Simulation Conference
CHU, M. L., Parigi, P., Latombe, J.-C. & Law, K. H. (2015). Simulating effects of signage, groups, and crowds on emergent evacuation patterns. AI & SOCIETY, 30(4), 493–507 [doi:10.1007/s00146-014-0557-4]
CHU, M. L., Parigi, P., Law, K. & Latombe, J.-C. (2014). SAFEgress: a flexible platform to study the effect of human and social behaviors on egress performance. In Symposium on Simulation for Architecture & Urban Design, (p. 4). Society for Computer Simulation International
COUNTRY FIRE AUTHORITY (2014). Your guide to survival: http://goo.gl/7UzH7g.
DAWSON, R. J., Peppe, R. & Wang, M. (2011). An agent-based model for risk-based flood incident management.Natural Hazards, 59(1), 167–189 [doi:10.1007/s11069-011-9745-4]
DUFF, T. J., Chong, D. M. & Tolhurst, K. G. (2013). Quantifying spatio-temporal differences between fire shapes: Estimating fire travel paths for the improvement of dynamic spread models. Environmental Modelling and Software, 46, 33–43 [doi:10.1016/j.envsoft.2013.02.005]
EDMONDS, B. & Moss, S. (2005). From KISS to KIDS: an anti-simplistic modelling approach. In P. Davidsson (Ed.), MABS, vol. 3415 of LNAI, (pp. 130–144). Springer [doi:10.1007/978-3-540-32243-6_11]
GRIGNARD, A., Taillandier, P., Gaudou, B., Huynh, N., Vo, D.-A. & Drogoul, A. (2013). Gama v. 1.6: Advancing the art of complex agent-based modeling and simulation. In PRIMA, (pp. 117–131)
HELBING, D. & Molnar, P. (1995). Social force model for pedestrian dynamics. Physical review E, 51(5), 4282 [doi:10.1103/PhysRevE.51.4282]
JOHNSON, N. R. (1987). Panic and the breakdown of social order: Popular myth, social theory, empirical evidence. Sociological focus, 20(3), 171–183 [doi:10.1080/00380237.1987.10570950]
KINATEDER, M. T., Kuligowski, E. D., Reneke, P. A. & Peacock, R. D. (2014). A review of risk perception in building fire evacuation. Tech. Rep. 1840, National Institute of Standards and Technology (NIST), USA [doi:10.6028/nist.tn.1840]
LAZARUS, R. S. (1991). Emotion and adaptation. London: Oxford University Press
LAZARUS, R. S. & Folkman, S. (1984) Stress, appraisal, and coping. New York: Springer
LE, V. M. (2016). Machine Learning methods for optimization in Multi-Agent Decisions Support System: application to Sign Placement for Tsunami Evacuation. Ph.D. thesis, Université Paris Nord
LE, V. M., Adam, C., Canal, R., Gaudou, B., Vinh, H. T., Taillandier, P. et al. (2010). Simulation of the emotion dynamics in a group of agents in an evacuation situation. In Principles and Practice of MAS, (pp. 604–619). Springer
LE, V. M., Chevaleyre, Y., Hô, T. V.& Zucker, J. (2015). Hybrid of linear programming and genetic algorithm for optimizing agent-based simulation. application to optimization of sign placement for Tsunami evacuation. In The 2015 IEEE RIVF International Conference on Computing & Communication Technologies - Research, Innovation, and Vision for Future, RIVF 2015, Can Tho, Vietnam, January 25-28, 2015, (pp. 138–143)
LE, V. M., Chevaleyre, Y., Hô, T. V.&Zucker, J. (2015). Hybrid of linear programming and genetic algorithm for optimizing agent-based simulation. application to optimization of sign placement for tsunami evacuation. In The 2015 IEEE RIVF International Conference on Computing & Communication Technologies - Research, Innovation, and Vision for Future, RIVF 2015, Can Tho, Vietnam, January 25-28, 2015, (pp. 138–143)
MAS, E., Imamura, F. & Koshimura, S. (2012). An agent based model for the Tsunami evacuation simulation. A case study of the 2011 great East Japan Tsunami in Arahama Town. In 9th International Conference on Urban Earthquake Engineering, (pp. 1957–1964). Citeseer
MCLENNAN, J. & Elliott, G. (2011). Community members decision making under the stress of imminent bushfire threat - murrindindi fire. Tech. rep., Bushfire CRC Extension, School of Psychol. Science, La Trobe Univ. http://goo.gl/cyVgY3
MINH, L. V., Adam, C., Canal, R., Gaudou, B., Ho, T. V. & Taillandier, P. (2010). Simulation of the emotion dynamics in a group of agents in an evacuation situation. In Principles and Practice of Multi-Agent Systems- 13th International Conference, PRIMA 2010, Kolkata, India, November 12-15, 2010, Revised Selected Papers, (pp. 604–619)
NGUYEN, T. N. A., Zucker, J. D., Nguyen, M. H., Drogoul, A. & Nguyen, H. P. (2012). Simulation of emergency evacuation of pedestrians along the road networks in Nhatrang city. In Computing and Communication Technologies, Research, Innovation, and Vision for the Future (RIVF), 2012 IEEE RIVF International Conference on, (pp. 1–6). IEEE
NGUYEN, V. T., Longin, D., Ho, T. V. & Gaudou, B. (2014). Integration of emotion in evacuation simulation. In ISCRAM-Med, (pp. 192–205). Springer [doi:10.1007/978-3-319-11818-5_17]
NURDIN, Y. & Yuliana, D. K. (2012). Tsunami evacuation simulation for disaster education and city planning. Journal of Disaster Research, 7(1), 92–101 [doi:10.20965/jdr.2012.p0092]
RHODES, A. (2014). Why don’t they do what we think they should? In AFAC. Emergency Management Victoria.
ROGSCH, C., Galster, R., Luthardt, T. & Mohr, D. (2014). The effect of pedestrian placement and pre-movement times on evacuation simulation. Transportation Research Procedia, 2, 291–299 [doi:10.1016/j.trpro.2014.09.055]
RONCHI, E. & Nilsson, D. (2013). Fire evacuation in high-rise buildings: a review of human behaviour and modelling research. Fire science reviews, 2(1), 1 [doi:10.1186/2193-0414-2-7]
SHENDARKAR, A., Vasudevan, K., Lee, S. & Son, Y.-J. (2008). Crowd simulation for emergency response using bdi agents based on immersive virtual reality. Simulation Modelling Practice and Theory, 16(9), 1415–1429 [doi:10.1016/j.simpat.2008.07.004]
TA, X. H., Longin, D., Gaudou, B. & Ho, T. V. (2015). Impact of group on the evacuation process: theory and simulation. In Proceedings of the Sixth International Symposium on Information and Communication Technology, (pp. 350–357). ACM [doi:10.1145/2833258.2833265]
TAILLANDIER, P., Bourgais, M., Caillou, P., Adam, C. & Gaudou, B. (2016). A situated BDI agent architecture for the GAMA modelling and simulation platform. In International Workshop on Multi-Agent-Based Simulation (MABS), Singapore. Springer
TEAGUE, B., McLeod, R. & Pascoe, S. (2009a). Final report. Tech. rep., Victorian Bushfires Royal Commission. Summary at http://goo.gl/ulCt4U
TEAGUE, B., McLeod, R. & Pascoe, S. (2009b). Final report - volume 4 - the statements of lay witnesses. Tech. rep., Victorian Bushfires Royal Commission URL http://goo.gl/3136pw
TEAGUE, B., McLeod, R. & Pascoe, S. (2009c). Final Report, Volume I, Part 2: The people who died. Chapter 21: lessons learnt. Tech. rep., Victorian Bushfires Royal Commission: http://goo.gl/0xcUFG
TEAGUE, B., McLeod, R. & Pascoe, S. (2009d). Final report, volume i: The fires and the fire-related deaths. Tech. rep., Victorian Bushfires Royal Commission. http://goo.gl/TW1N9b
TSAI, J., Fridman, N., Bowring, E., Brown, M., Epstein, S., Kaminka, G., Marsella, S., Ogden, A., Rika, I., Sheel, A., Taylor, M.,Wang, X., Zilka, A. & Tambe, M. (2011). ESCAPES - Evacuation Simulation with Children, Authorities, Parents, Emotions, and Social comparison. In S. Tumer, Yolum & Stone (Eds.), AAMAS Innovative Applications Track. Taipei, Taiwan
YUDUDKOWSKY, E. (2008). Cognitive biases potentially affecting judgment of global risks. In Global Catastrophic Risks, (pp. 91–119). New York: Oxford University Press