Introduction
Epstein’s famous postulate “if you didn’t grow it you didn’t explain it” (Epstein 2006) of the programme of a generative social science captures in a nutshell the explanatory account of agent-based social simulation. Agent-based models enable the generation of macro-social patterns through the local interaction of individual agents (Squazzoni et al. 2014). Classical examples include the segregation of residential patterns in the Schelling model, emergence of equilibrium prices (Epstein and Axtell 1996), or local conformity and global diversity of cultural patterns (Axelrod 1997). Agent-based modelling has been used for a long time in archaeological research for investigating, for instance, spatial population dynamics (Mithen 1994; Kohler and Gumermann 2000; Burg et al. 2016). Likewise there is a growing interest for including culture (Dean et al. 2012; Dignum and Dignum 2013) and qualitative and textual data in agent-based simulation (e.g. Edmonds 2015a). Nevertheless agent-based modelling is less used in ethnographic and cultural studies attempting at uncovering hidden meaning of the phenomenology of action. Typically ethnographic research is done by interpretative research using qualitative methods such as thick description (Geertz 1973) or Grounded Theory (Glaser and Strauss 1967). The objective of this paper is to elaborate a framework for using simulation as a tool in a research process that facilitates interpretation. While applying the generative paradigm the objective is growing artificial culture, i.e. an artificial perspective from within the subjective attribution of meaning to social situations. Thereby a methodology will be described for using simulation research as a means for exploring the horizon of a cultural space.
The paper describes a research process that we developed (Lotzmann et al. 2015) during the recent EU FP 7 GLODERS project (www.gloders.eu). As the project involved stakeholders from the police in a participatory modelling process (Barreteau et al. 2003; Nguyen-Duc and Drogul 2007; Möllenkamp et al. 2010; Le Page 2015) for providing virtual experiences to police officers, the objective of the research process is the cross-fertilization of simulation and interpretation. Thus the example that we use throughout the paper is the investigation of criminal culture. While ethnographic research goes back to classical studies of e.g. Malinowski or Margaret Mead on tribes in the Pacific islands (Malinowski 1922; Mead 1928) it has expanded to studying cultures of various kinds such as youth (Bennett 2000) or business culture (Isabella 1990). Thus it is reasonable to use the field of the criminal world for interpretative investigations. The objective of the research, imposed by the stakeholder interests, was to investigate a specific element of criminal culture, namely modes of conflict regulation in the absence of a state monopoly of violence. For this purpose norms and codes of conduct of the ‘underworld’ needed to be dissected. While individual offenders might pursue their own path of action, as soon as crime is undertaken collectively, i.e. in the domain of organized crime, the necessity for standards that regulate interactions emerges in the criminal world as it does in the legal society. These can be described as social norms (Gibbs 1965; Interis 2011). Thus studying norms and codes of conduct in the criminal world provides a perfect example for studying the emergence of a specific element of culture, namely social norms[1]. However, the focus of this paper is on methodology, namely the research process starting from unstructured textual data ending up in simulation results which can recursively be traced back to the starting point of the research process. Results concerning content are documented elsewhere (Neumann and Lotzmann 2017; Lotzmann and Neumann 2017). For the purpose of this article it is sufficient to emphasize that (specific elements of) criminal culture provides an adequate example to demonstrate the methodology of interpretative simulation. The research process entails two perspectives, an analysis and a modelling perspective, which are recursively related to each other.
- Analysis perspective: The process of recovering information within the empirical data from which certain simulation model elements are derived.
- Modelling perspective: The structured process of the development of a simulation model and the performing of simulation experiments based on the empirical data.
Thus the relation between data, research question and methodology has to be considered carefully. The development of the research process is closely oriented to the modelling process developed in the EU funded OCOPOMO project (Scherer et al. 2013, www.ocopomo.eu) but has been adapted and extended by an interpretative perspective. While for the analysis perspective the process of conceptual modelling, model transformation and implementation of declarative rule-based models has been extended by an initial qualitative data analysis (extending the scenario input proposed in OCOPOMO), for the modelling perspective the traceability concept (Scherer et al. 2013, 2015) is utilized for developing virtual narratives that facilitate interpretative research (i.e. referring back to the initial qualitative analysis). This enables the use of simulation as a means for a qualitative data exploration that reveals how actors make sense of the phenomenology of a situation to finally enable growing criminal culture in the simulation lab.
The rest of the paper is structured as follows: Section 2 provides a brief overview of the concept of thick description as methodology of interpretative research. Next, the research process is described in detail in Sections 3 and 4. This consists of several steps. Section 3 highlights the analysis perspective and Section 4 highlights the modelling perspective. Finally Section 5 discusses how the methodology can provide insights beyond the particular example of criminal culture.
Thick description in cultural studies
The central element of an interpretative approach to the social world is an attempt to comprehend how participants in a social encounter perceive a particular concrete situation from within their worldview. Instead of observing from outside, interpretation attempts to comprehend social interaction from inside the social actors. How this can be achieved has for a long time been the subject of many debates ranging from philosophical speculation, such as Dilthey (1976) who introduced the term ‘understanding’ as a technical terminus in interpretative research, to various methods in qualitative empirical research. A particularly well-known approach to interpretative research is the concept of thick description. Originally coined by Clifford Geertz as a method for participant observation in ethnographic research, the concept quickly traversed to various disciplines such as general sociology, psychology, education research or business science (Denzin 1989; Ponterotto 2006).
Geertz owes the term thick description to the philosopher Gilbert Ryle (1971). Citing Ryle, Geertz considers "two boys rapidly contracting the eyelids of their right eyes. In one, this is an involuntary twitch; in the other, a conspiratorial signal to a friend. The two movements are, as movements, identical; from an I-am-a-camera, ‘phenomenalistic’ observation of them alone, one could not tell which was twitch and which was wink, or indeed whether both or either was twitch or wink. Yet the difference, however unphotographable, between a twitch and a wink is vast ..." (Geertz 1973, p.312). This example describes the step from a phenomenology of a situation to the meaning attributed to it. This move from what Geertz, following Ryle, denotes as a step from ‘thin’ to ‘thick’ description has been influential for an interpretative theory of culture. Geertz provides an example of a drama in the highlands of Morocco in 1912 in which different interpretations of a particular sequence of interactions by various ethnic groups (including Berbers, Jews and French imperial forces) generated a chaotic dissolution of the traditional social order: One man had been hijacked by a Berber clan. In compensation he stole the sheep of the clan. However, subsequently he negotiated that a certain number of sheep was a legitimate compensation for the raid. But when he came back to the town ruled by French forces, they arrested him for theft. The example serves as a demonstration of an interpretative concept of culture. Resembling Wittgenstein’s theory of language games, Geertz argues that culture is public symbolic action. In the example above, the reactions of the different cultural groups failed to be meaningful for the other groups because all parties (in particular the French forces) had a different view of the meaning of the action of the other parties. In consequence, it comes to a “confusion of tongues” (Geertz 1973, p. 322). Winking or stealing sheep have a different meaning in different cultures. Thus meaning is important for social order because, for instance, the reaction to what is perceived as a conspiratorial wink is different from the reaction to an involuntary eye movement, say blinking in strong sunlight. Meaning shapes the space of plausible (and implausible) follow-up actions.
The implication for investigating cultures is that an interpretative theory of culture is an interpretation of interpretations. The task of a cultural analysis is signifying and interpreting the subjects of study, whether criminals, Berbers or Frenchmen, i.e. interpreting how the subjects make sense of the world from the perspective of their worldview (Denzin 1989). This calls for a microscopic diagnosis of specific situations in a manner that enables a reader of a different cultural background to grasp the meaning that the subjects of the investigation attribute to it. Geertz claims that the role of theory in the interpretative account of thick description is to provide a vocabulary to establish conversation across cultures (Geertz 1973, p. 312). Such a vocabulary should “produce for the readers the feeling that they have experienced, or could experience, the events being described in a study” ( Creswell and Miller 2000, p. 129) such as a sense of verisimilitude (Ponterotto 2006). Thus the objective of a thick description is providing narrative storylines of the field (Corbin and Strauss 2008). In the following, we will outline a research process for developing artificial narratives that generate virtual experiences. This enables extending the generative paradigm for growing artificial cultures.
Methodological process to build interpretive simulations: analysis perspective
In fact, agent-based modelling has a number of properties that coincide with the account of a thick description: Agent-based modelling studies the interaction of individual agents on a microscopic level (Squazzoni et al. 2014), in relation between cognition and interaction (Nardin et al. 2016). Likewise qualitative data is increasingly used for the development of agent rules (Fieldhouse et al. 2016; Edmonds 2015a; Ghorbani et al. 2015; Dilaver 2015). Nevertheless, it is rarely the intention of agent-based research to get in conversation with the agents. In the following, we describe a process of deriving agent rules from interpretative empirical research methods. Applying the rules in simulation experiments enables the investigation of socio-cognitive coupling: individuals reasoning about other individuals’ minds, by taking into account a shared social context. That means: the agents attribute meaning to the observed behaviour of other agents.
This is undertaken using the example of the investigation of police files documenting intra-organizational processes within a criminal network, leading to the internal collapse of a criminal organization. Studying intra-organizational norms is faced with the problem of cognitive complexity. For an analysis of intra-organizational norms, detailed information about the motivation and subjective perceptions of the individuals involved in this process is necessary. This calls for tools which support less handling of a quantity of data but rather detailed interpretative research methods. For this purpose first, MAXQDA (www.maxqda.de) has been selected as a tool for Computer assisted qualitative data analysis (CAQDAS). This is a standard tool and others (e.g. ATLAS.ti or NVivo) would have been equally valuable. Next, the interface between interpretative research and development of agent rules in formal modelling draws on the tools that have been developed in the OCOPOMO project, more specifically CCD (Scherer et al. 2013, 2015) and DRAMS (Lotzmann and Meyer 2011), which enable to preserve traceability of agent rules to the empirical evidence base (Lotzmann and Wimmer 2013). These tools are part of the OCOPOMO toolbox developed in previous research to achieve empirically founded simulation results. Here, also, other approaches and tools could have been used, like e.g. flow charts (as inspired by Scheele and Groeben 1989) for the conceptual model and NetLogo for implementing the simulation model, at the price of not having tool-support for semi-automated model-to-code transformation and for generating and visualizing traces. The important criteria for the conceptual modelling is that the (graphical) language used needs to be comprehensible to the stakeholders (i.e. people involved in the participatory modelling), and the programming paradigm and language should facilitate the structure of the conceptual model, i.e. a logic-based programming approach like a declarative rule engine can be considered beneficial. Finally, the results of the simulation are traced back to an interpretative framework for dissecting – in this particular case, criminal – culture.
In our case, the data basis is unstructured textual data from police interrogations of witnesses as well as suspects involved in a violent collapse of the criminal group. It has to be acknowledged that police interrogations are artificial situations and respondents might answer strategically or simply lie. Moreover the interrogation is guided by certain interests of the police. In this case for instance, the police investigations focused on persons related to money laundering and less on drug production. This uncertainty is typical for criminological data (Bley 2014). Nevertheless the data is the best at hand as in-depth interviews are usually problematic in the case of investigating criminals (however, see e.g. Bouchard and Quellet 2011 who interviewed prisoners). Police interrogations differ from court files not least because they are confidential and many of the respondents were witnesses, for instance relatives of victims. This provides certain credibility. Often the interrogation protocols describe testimonials of persons in a stress situation such as being in fear for their life or having experienced the murder of a friend. Therefore police interrogations can be described as approximations of situations of dialogical conversation, allowing for an in-depth analysis of subjective meaning attributed to certain situations, which brings the empirical analysis very close to the subjective perception of the actors. The aim is to infer hypothetical, unobservable cognitive elements from observable actions and statements to analyse cognitive mechanisms that motivate action in very confused and opaque situations (Neumann and Lotzmann 2017). Modelling this cognitive complexity provides a challenge for the foundation of model assumptions. For this reason a procedure has been developed that describes a controlled process from qualitative evidence to agent rules. The research partly draws methodologically upon a Grounded Theory approach (Glaser and Strauss 1967; Corbin and Strauss 2008), partly upon the OCOPOMO process developed in the corresponding project in order to arrive at a thick description of the field. The simulation model is based on a data driven, evidence based model. The overall research process is outlined in Fig. 1
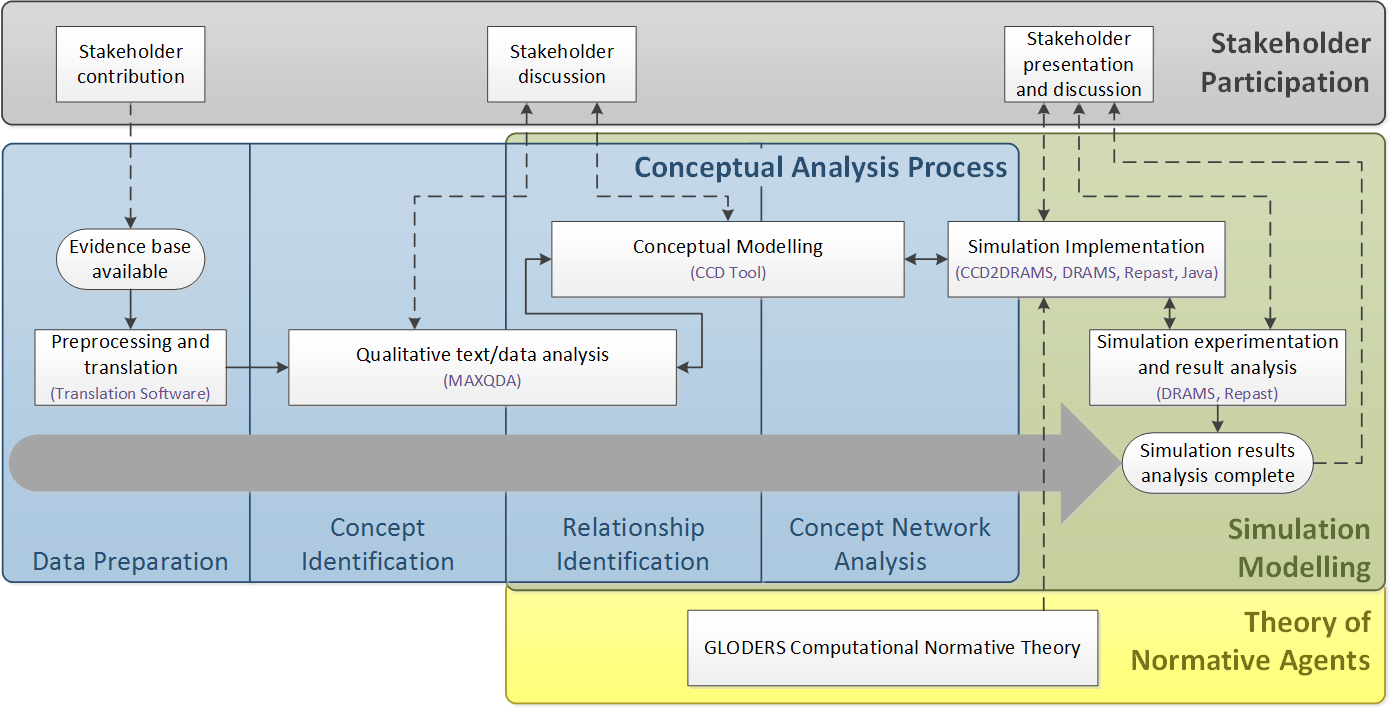
The figure highlights three elements of the analysis process of the data, which provides the foundation for the development of a simulation model. The data analysis is a basically qualitative process which enables to derive detailed model assumptions. The core is the analysis itself, documented in the blue box. The analysis is embedded in a theory of normative agents, developed in prior research in the EMIL project (Conte et al. 2014), and constant stakeholder participation.
The analysis can be grouped into the four process phases depicted in Fig. 2: data preparation, concept identification, concept relation identification and concept network analysis. These process steps include various activities which are supported by different software tools. First, data was provided by the stakeholder. This consists of police interrogations of witnesses and suspects. For an analysis of these documents pre-processing is necessary: data had to be checked for ensuring protection of privacy, the text was translated, and errors and flaws intruded by certain data pre-processing steps (e.g., OCR, translation) needed to be corrected. Data preparation provides the basis for first identifying concepts in the data.
A detailed representation of the qualitative data analysis and conceptual modelling process details is documented in Fig. 2. In this flow chart the relationship identification and the concept network analysis are merged into a single phase, as these two phases are closely interrelated. Hence, this merged phase as well as the preceding concept identification phase are discussed and illustrated in the following two subsections.
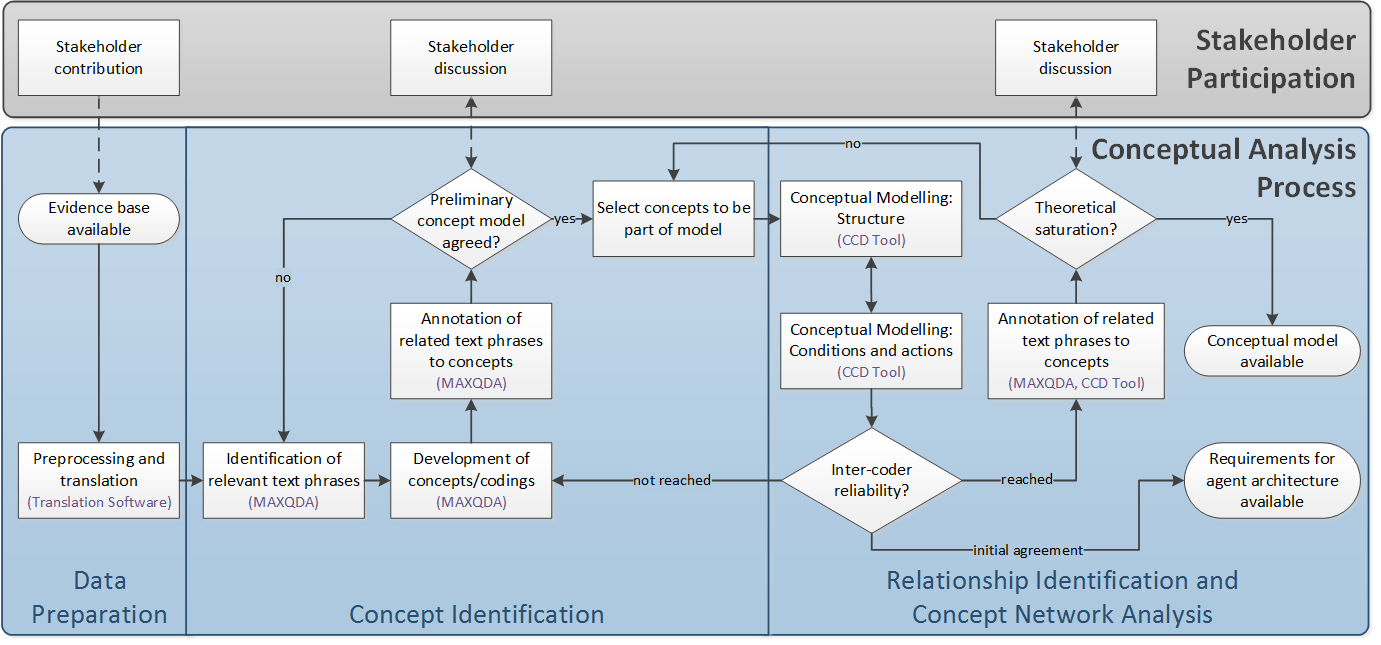
Concept identification: Qualitative text analysis
In a first step of concept identification the data need to be loaded into a tool for qualitative text analysis such as MAXQDA (see Corbin and Strauss 2008). Concepts stand for classes of objects, events or actions which have some major properties in common. Relevant text passages had to be identified which reveal preliminary concepts, documented in a list of codes. These are then used to annotate further text passages which provide additional information about the concept. An example is provided in Fig. 3.
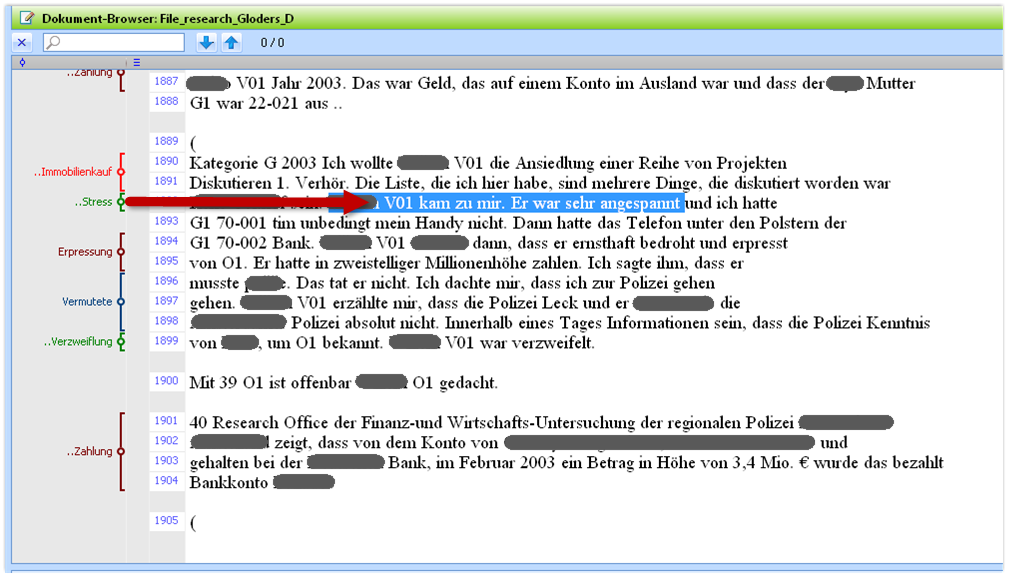
Fig. 3 shows a screenshot of how codes are related to the text. On the right hand side is the textual document, in our case the police file. The left hand side shows the codes that are related to certain text phrases. If one code is selected, the corresponding text is highlighted (relation indicated by the red arrow). For the methodological purpose of the article it is not necessary for the reader to read the text: Rather for empowering the reader to follow the research account only the relation between codes and the textual evidence base shall be highlighted.
As indicated in Fig. 2, coding is an iterative process: further text passages give rise to the development of new codes and the revision of prior preliminary codes until a stage is reached in which the relevant concepts are identified which should be part of a model of the data. This research design follows the open coding approach of Grounded Theory (Corbin and Strauss 2008). Fig. 4 provides an example of the dimensions of certain codes: MAXQDA enables to view the text annotations belonging to a certain code. This enables a proof of consistency and an assessment of the dimensions of the concepts. Following the so-called member checking procedure for ensuring credibility in qualitative research (Lincoln and Guba 1985; Hammersley and Atkinson 1995; Creswell and Miller 2000; Cho and Trent 2006)[2] these results have been presented to the stakeholder in order to ensure the empirical and practical relevance of the concepts. In our case example, the subjects of the investigation had not directly been involved in the research (i.e. criminals), but rather those actors that the research addresses, namely the police, had been part of the participatory research process.
Fig. 4 provides a full view of the coding. On the left is the list of codes. The selected code is highlighted in red. On the bottom of the right side are the text phrases which are annotated by the selected code, whereas on the top of the right side one can find where a particular coding (i.e. a text phrase) is located in the overall document. Again emphasis is not on the content but on the relation between data and analysis.
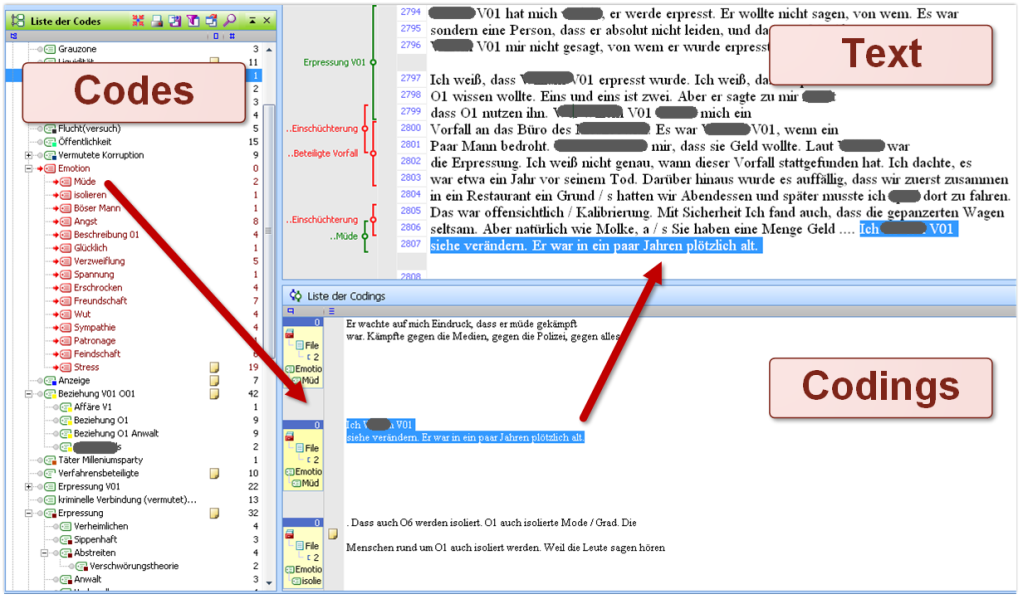
Relationship identification: Conceptual modelling
The first step of the methodology follows Grounded Theory. Already Grounded Theory emphasizes that concepts need to be related, typically undertaken in the research step of axial coding (Corbin and Strauss 2008). However, in order to derive a simulation model from the data, in this second step the research diverges from Grounded Theory accounts. The coding derived with CAQDAS software (here: MAXQDA) serves as the basis for concept relation identification with the CCD tool, which is a piece of software for creating a conceptual model of the processes that can be found by the analysis of the data (Scherer et al. 2013, 2015). The dynamic perspective of conceptual modelling using the CCD approach is loosely related to the concept of flow process charts that have been suggested in qualitative research (e.g. Scheele and Groeben 1989), but with a specific grammar (an alternating appearance of conditions and actions), with a restricted syntax (e.g. there are no explicit conditions), but with more expressive syntax elements (e.g. attributes – following strict schemes – can be attached to conditions and actions). The rationale for this type of graphical language is twofold: On the one hand the “simplistic” concept can help to make the conceptual model of dynamics intuitively more comprehensible, on the other hand a semi-automatic transformation into program code (here: DRAMS rules) can be achieved. In this context, semi-automatic transformation means that so-called rule stubs with information on related data elements can be generated automatically, while the programmer has to implement the actual rule code.
Identifying relations between concepts is a central stage for the process view of a simulation approach. This research step departs from a Grounded Theory approach by making use of an abstract framework of (the above-mentioned) condition-action sequences (Scherer et al. 2013, 2015; Lotzmann and Wimmer 2013). The web of interrelated sequences is denoted as an action diagram. The concept of condition-action sequences is an a-priori methodological device to identify social mechanisms on a micro level of individual (inter-)action. Broadly speaking a mechanism is a relation that transforms an input X into an output Y. A further condition is a certain degree of abstraction, which becomes evident in a certain degree of regularity, i.e., that under similar circumstances a similar input X* yields similar outputs Y*. In the social world this is typically an action which relates X and Y (Hedström and Ylkoski 2010). This is assured by the concept of condition-action sequences. Every process is initiated by a certain condition which triggers a certain action. This action in turn generates a new state of the world which is again a condition for further action. Whereas the data describes individual instantiations, the condition-action sequences represent general event classes. For instance, in our case one condition is denoted as ‘return of investment available’. This triggers an action class denoted as ‘distribute return of investment’. Obviously this condition-action sequence describes classes of events. Return of investment might be rental income as well as purchasing of companies. This methodology enables controlled generalization from the case. The case, however, provides a proof of existence of the inferred mechanisms. Note that the data basis of interrogations allows including cognitive conditions (such as ‘fear for one’s life’) and actions (such as ‘member X interprets aggressive action’). For understanding culture it is essential to retrieve the unobservable meaning attributed to particular situations which are observable at a phenomenological level (Neumann and Lotzmann 2017).
In terms of the research process, data need to be loaded into the CCD tool. An actor-network diagram needs to be compiled which entails relevant actors and objects of the domain. These provide the basis for the development of an action diagram of the condition-action sequences describing the processes in the domain. The development of the action diagram needs to be undertaken in constant comparison with the concepts identified with CAQDAS software (MAXQDA) in the first research step. CCD provides textual annotations for the identified elements like actors, objects, actions and conditions which ensure empirical traceability (comp. Fig. 5, again not the empirical content is relevant but the relation between data and the identified object, actors and relations). These are imported from the annotations to the MAXQDA codes (comp. Fig. 4 with list of annotations to a code). This feature provides a benchmark that all the codes and their relevant dimensions derived with MAXQDA are represented in the condition-action sequences (Neumann and Lotzmann 2014). Note, that this is a recursive process: the action diagram needs to be constantly revised until a situation of theoretical saturation (Corbin and Strauss 2008) is reached. Again the validity needs to be ensured by members checking, i.e. consulting stakeholders.
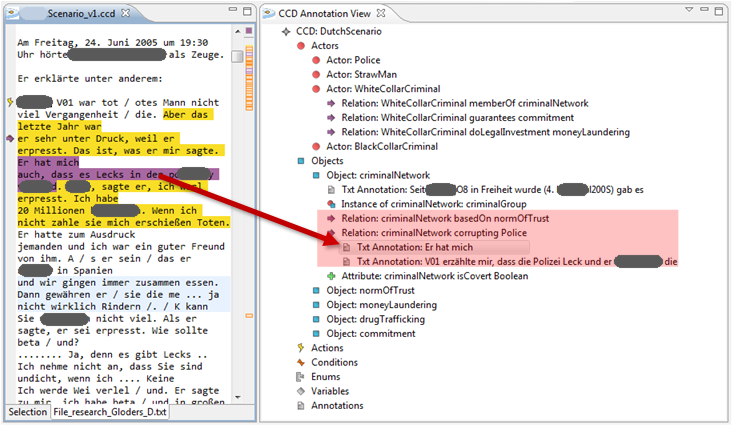
Example: Revealing interpretations
In the following an example of a conceptual model (the example is taken from Neumann and Lotzmann 2014, 2017) will be provided that shows how people under study interpret phenomena. That is how people attribute meaning to the phenomenology of situations. Following Geertz we provide an interpretation of interpretations. Like the example of the sheep raid in the Moroccan highlands discussed by Geertz, the example of our research, in fact, is also an instance of the breakdown of social order. In terms of Geertz, it can be described as a “confusion of tongues” (Geertz 1973 p. 322). For the observer, meaning is most easily transparent in the case when it becomes non-transparent for the participants. However, here we concentrate on the methodological issue to demonstrate how conceptual modelling facilitates dissection of meaning as the core business of interpretative research and abstain from presenting the full confusion (see Neumann and Lotzmann 2014, 2017).
As an example Fig. 6 describes a part of the CCD action diagram. This example, in particular the action “perform aggressive action against member X” will be used in the following to demonstrate the link between annotations as result of the empirical analysis outlined above, and the simulation modelling, experimentation and result analysis.
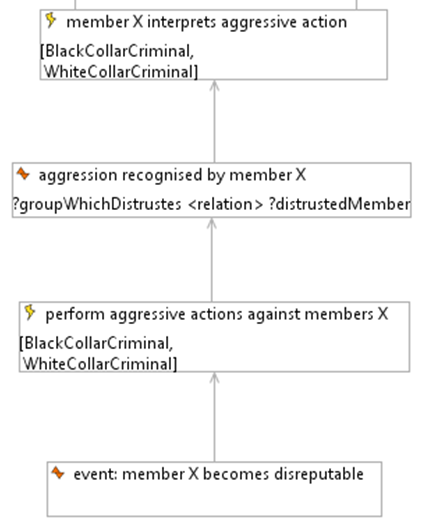
Fig. 6 shows an abstract event-action sequence which is derived from the data analysis. The box with a red flag represents an event. The action is represented by a box with a yellow flag. Moreover, in brackets we see the possible type of agents that can undertake the action. The arrow represents the relation between the event and the action. This is not a deterministic relation. However, the existence of the condition is necessary for triggering the action. Once an action is performed a new situational condition is created which again triggers new actions. In the figure, the process starts with the event that someone becomes suspect (denoted as ‘disreputable’) which triggers the action of performing an act of aggression against this person. When the victim recognizes the aggression, he or she needs to interpret the motivation. This process of interpretation is displayed in Fig. 7.
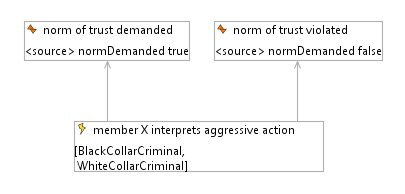
Two options are considered as possible in the conceptual model. In fact, this is our (i.e. the researchers’) interpretation of how the subjects of investigation interpret their experience. However, it is based on a number of instances that had been categorized in the concept identification phase and the credibility has been checked by stakeholder consultation (in terms of validating qualitative research: member checking). Thus Fig. 7 shows a branching point in the interpretation: The perceived aggression can be interpreted either as norm enforcement, denoted as ‘norm of trust demanded’, or as norm deviation, denoted as ‘norm of trust violated’. At this point the agents attribute meaning to the phenomenological experience of being a victim of aggression. As in Geertz’ example of the sheep raid, different interpretations are possible. Dependent on the interpretation different action possibilities are triggered. Again this is an abstract cognitive mechanism. However, we show one example of how these abstract mechanisms can be traced back to the data. The starting point is the event that for some reason (outside of the scope of the investigation) a member of the organization becomes distrusted (see Fig. 6). This initiated a severe aggression as shown in the following annotation[3]:
Annotation (perform aggressive action against member X): “An attack on the life of M.”
It remains unclear who commissioned the assassination and for what reason. It should be noted that it is possible that an attack on the life could be the execution of a death-penalty for deviant behaviour. In fact, some years later M. was killed because he had been accused of stealing drugs. It remains unclear whether this was true or the drugs just got lost for other reasons. However, the murder shows that the death penalty is a realistic option in the interpretation of the attack on his life. However, M. survived this first attack which allowed him to reason about the motivation. No evidence can be found in the data to support this reasoning. However, data on how he reacted can be found.
Annotation (member X decides to betray the criminal organization): Statement of gang member V01: “M. told the newspapers ‘about my role in the network’ because he thought that I wanted to kill him to get the money.”
This example allows a reconstruction of a possible reasoning, i.e. an interpretation of the field data. First, given the evidence that was available to the police it is unlikely that this particular member of the organization (V01) mandated the attack. However, the members of the criminal gang had not the time and resources for a criminal investigation as the police would have undertaken. Nevertheless they had to react quickly in complex situations. In fact, it is not a completely implausible consideration. M. was a drug dealer who invested money in the legal market by consulting a white collar criminal with a good reputation in the legal world. V01 was such a white collar criminal. Thus V01 possessed a considerable amount of drug money which he could have kept for himself if the investor (in this case M.) were dead. This might be a ‘rational’, self-interested incentive for an assassination. Second, it can be noted that M. interpreted the attack on his life not as a penalty (i.e. death-penalty) for deviant behaviour from his side[4]. Instead he concluded that the cause of the attack was based on self-interest (the other criminal ‘wanted his money’). Thus he interpreted the attack as norm deviation rather than enforcement (see Fig. 7). Next, he attributed the aggression to an individual person and started a counter-reaction against this particular person by betraying ‘his role in the network’. This is an example of an interpretation of how participants in the field make sense of an action from the worldview of their culture. Namely, he interpreted the aggression as a violation of his trust in the gang and reacted by betraying the accused norm violating member. Factually this counter-reaction provoked further conflict escalation. However, for the methodological purpose of demonstrating the research process we stop at this point (see further empirical detail in Neumann and Lotzmann 2017) and move on to a documentation of how the conceptual model is transformed into a simulation model.
Simulation modelling and experimentation: Simulation perspective
So far, the presentation of the research process has concentrated on the analysis perspective. Now we come to the simulation modelling perspective, as the process of empirical analysis provides the basis for the development of agent rules. This process encompasses the implementation and verification of the simulation model, with a successive validation phase (involving the stakeholder). With the validated model, productive simulation experiments can be performed, in order to generate results that can be analysed and presented to the stakeholders. Fig. 8 shows this simulation modelling process applied in our research in detail.
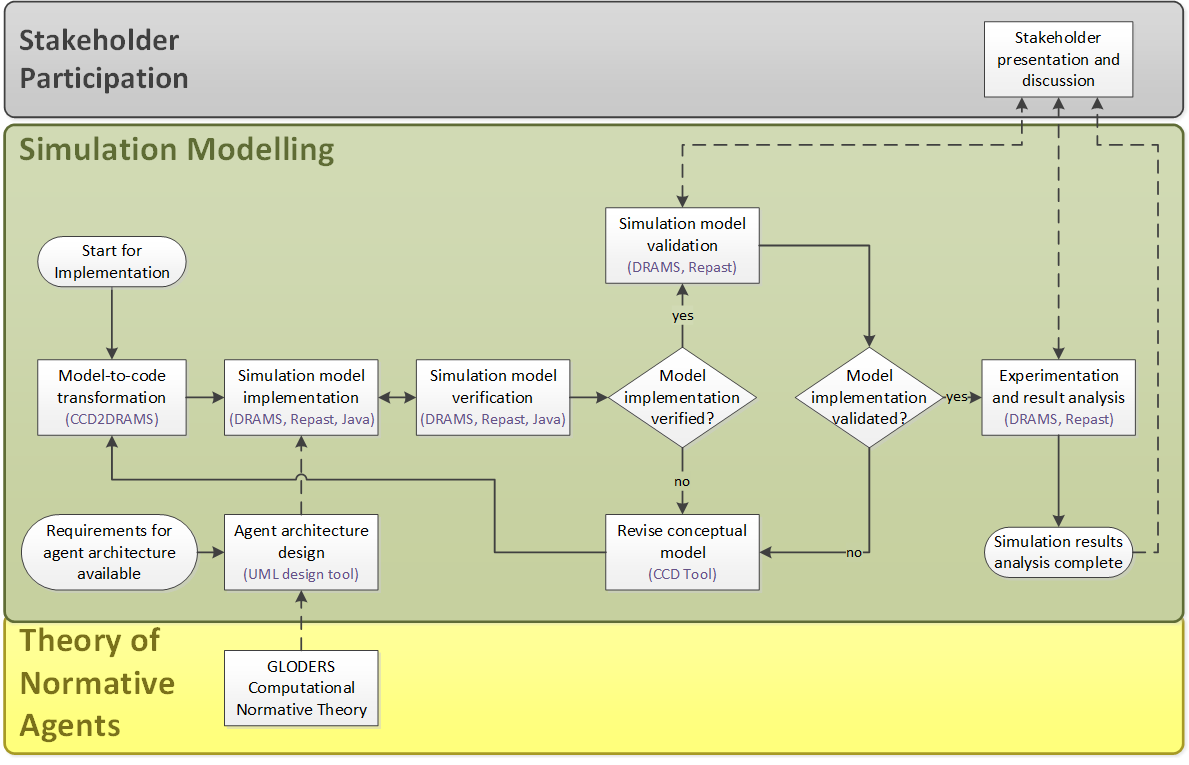
In the diagram two starting points are present: The first is the availability of requirements for the agent architecture that are derived during (quite early stages) the conceptual modelling phase, i.e. as soon as inter-coder reliability is agreed, according to Fig. 2. At this point the implementation-oriented agent architecture can be modelled (using the UML diagram and/or flow charts) which also takes other requirements into account[5].
The second starting point in Fig. 8 is the actual start of the implementation, i.e. when a comprehensive and consistent conceptual model is available. The implementation process is outlined in the next subsection, followed by a subsection on simulation result analysis.
Simulation model implementation
If the conceptual modelling approach and language provide capabilities of model-driven architecture as in this example, then the implementation starts with a transformation step from the conceptual model to program code. With the OCOPOMO toolbox used in our research (as mentioned in Section 3.2), this step is performed by the CCD2DRAMS tool for which the CCD provides an interface that supports a semi-automatic transformation of conceptual model constructs into declarative code for the distributed rule engine DRAMS and Java code for the simulation framework RepastJ 3.1 (North and Collier 2006). Since DRAMS is implemented in Java, it entails the premises for close integration with Java-based simulation frameworks to extend their functionality.
The main objective of using these tools rather than standard tools (e.g. Python, Matlab) or typical simulation software (e.g. NetLogo, Mason) can be seen in the fact that these tools enable using simulation as a means for exploration of qualitative textual data by providing traceability information, an untypical application of social simulation. This is in particular the case since the structure of DRAMS code follows a similar logical approach to the action diagram of the CCD. Conditions in the CCD become facts in DRAMS, and actions become rules. This makes it possible that the formal code can precisely reproduce the conceptual model.
Hence, the code of a simulation model with DRAMS as technological basis consists mainly of declarative rules describing the agent behaviour. From a more technical perspective, these rules are evaluated and processed by a rule engine, according to Lotzmann and Wimmer (2013) a software system that basically consists of:
- Fact bases storing information about the state of the world in the form of facts.
- Rule bases storing rules to describe how to process facts stored in fact bases. A rule consists of a condition part (called left-hand side or abbreviated LHS, with the function to retrieve and evaluate existing facts) and an action part (called right-hand side or abbreviated RHS, with the function to assert new facts). In a nutshell, the RHS ‘fires’ if the condition formulated in the LHS is evaluated with the Boolean result ‘true’.
- An inference engine, an expert system-like software component that is able to draw conclusions from given fact constellations. As a by-product of this inference process the traces from rule outcomes (in effect simulation results) back to elements of the conceptual model are generated. From there the step back to the empirical evidence can easily be taken owing to the annotations included in the CCD.
These features enable decomposing and rearranging the empirical data which will become relevant for the scenario analysis as a means of systematic data exploration (comp. 4.2 and 4.3). The relations between facts and rules can be visualized as a more technical (i.e. including implementation specific details) counterpart to the CCD action diagram, in case of DRAMS by automatically generated so-called data dependency graphs (DDGs). Fig. 9 shows an example, a rule (rectangular node) “member X goes to public” with the actual facts (oval nodes; green for initially existing facts, red for facts generated during simulation) for pre- and post-conditions. The pre-conditions include the membership in the criminal network (initial fact “R_memberOf_criminalNetwork”), the knowledge that a ‘public’ exists (initial fact “public”) and the actual reason for the envisaged betrayal (fact “externalBetrayalInformation”, expressing the consequence of an act of betrayal performed by a White Collar criminal agent earlier in the simulation). The post-conditions of the rule are the information about the decision (fact “aggressionDecided”), a possible normative event as the betrayal violated the norm of trust between the criminals (fact “requestForNormativeEvent”), and the actual fact that the public knows about the criminal network (fact “R_knownBy_public” which becomes effective one tick later, indicated by the edge annotation [1.0]; the annotation [0.0] for the other two signifies an immediate effect of the asserted facts).
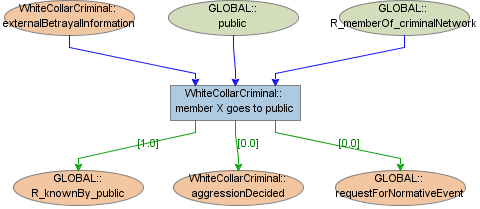
The decision process that might lead to an act of betrayal includes stochastic processes. Calibration of the probabilities in such decision points of the agents refers back to the first phase of a qualitative analysis. In the step of the analysis of the textual data, so-called ‘in-vivo codes’ had been created, i.e. annotations of characteristic brief text-elements. These had then been subsumed to broader categories which provide the building blocks of the conceptual model. Their relative frequency is put in use for specifying probabilities. Certainly these have to be used with caution: first the categorization entails an element of subjective arbitrariness when subsuming a description of a concrete action under a category such as ‘going to public’, etc. Second, the relative frequencies in data might not be very reliable. As they are based on police interrogations, some events might appear more likely to be subject of the interrogation than others. It might well be the case that the respondents did not remember or that the interrogation simply did not approach the issue. Thus, given the problem of estimated number of unreported cases inherent in any criminological research, the relative frequencies provide at least a hint to the empirical likelihood of the different courses of action. Below in Table 1, an example is provided of how the likelihood of agents’ decisions is informed by the evidence base.
Acts of external betrayal | Severity | Cases in evidence | Inferred Probability |
going to police | Modest | 2 | 0.334 |
going to public | High | 4 | 0.666 |
The next step in the simulation modelling process according to Fig. 8 is the verification of the simulation model, i.e. tests whether the concrete implementation reflects the envisaged concept. If the test is negative, then either the bugs in the implementation might be fixed directly (i.e. the implementation is aligned with the specification in the conceptual model), or in cases where gaps or imperfections in the conceptual model are revealed a revision of the conceptual model might become necessary.
The successfully verified model can then be validated. This step is mainly characterized by presentation and discussion of the model and the gained experimentation results with the stakeholders. Unsuccessfully validated aspects of the model can again lead to revisions of the conceptual model. With the validated model, finally experiments can be performed and outcomes for the simulation result analysis generated.
Simulation result analysis
The data dependency preserved during the modelling process ensures traceability of the inference such as simulated facts to the data. This facilitates the analysis of simulation results as means of data exploration. One of the available analysis tools is the Model Explorer tool, a part of the DRAMS software. Fig. 10 shows a screenshot of an actual triggering of the rule “plan a violent action – emotional”, in order to demonstrate a prototypical user interface for analysing simulation results. On the right hand side, the log files of the simulation run can be found. On the left hand side, the empirical, textual data is displayed. Both elements, the simulation and the empirical basis, are related by a visualization of the rules triggered to transform data into simulation results in the log file and the corresponding CCD elements of the conceptual model.
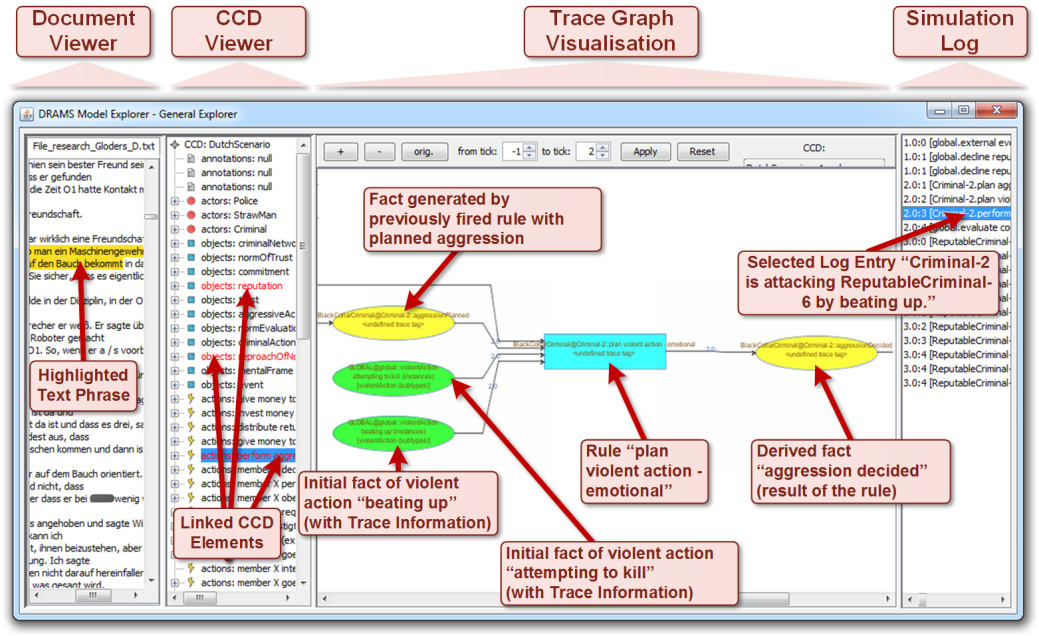
In the following an example of an interpretation of a simulation run will be provided. Again the example is taken from our research to illustrate the process of developing interpretative simulations. Simulation models typically generate outputs such as times series or histograms. Here the output is different: Based on the exploration of the simulation results as displayed in Fig. 10, a simulation run generates a virtual narrative. This final stage of model exploration closes the cycle of qualitative simulation, beginning with a qualitative analysis of the data as a basis for the development of a simulation model and ending with analysing simulation results by means of an interpretative methodology in the development of a narrative of the simulation results. First screenshots of an example of part of a simulation run are provided (six screenshots captured between tick 2 and tick 17 of a simulation run) in the animated Fig. 11. In the next section it is shown how the model explorer enables recourse to the in vivo-codes of textual data generated in the first step of the qualitative data analysis.
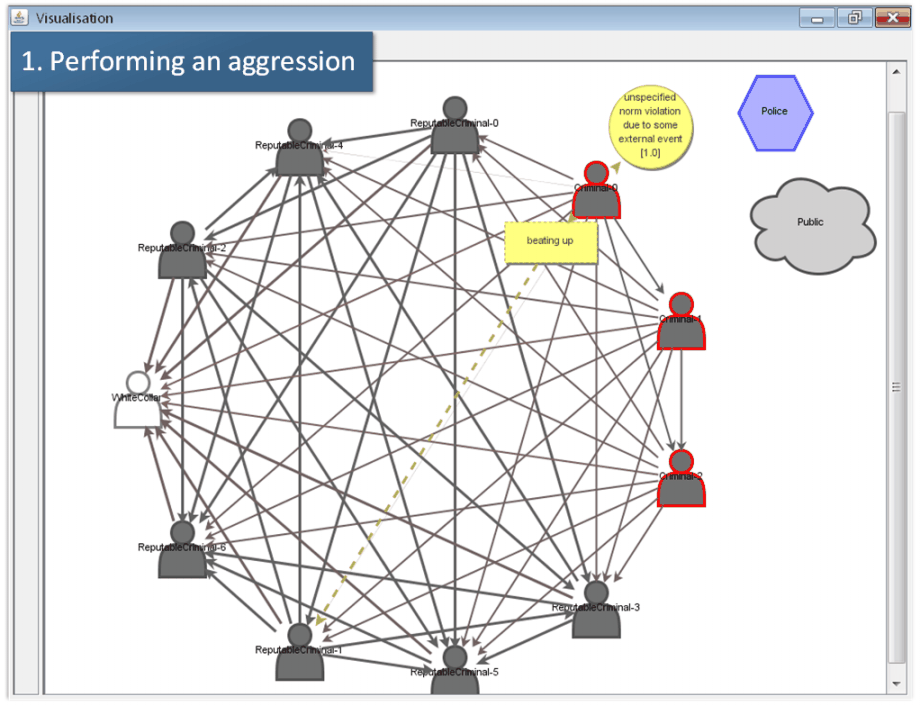
The screenshot shown in Fig. 11-1 (‘Performing an aggression’) displays a scene briefly after the start of the simulation: One randomly selected agent (Criminal 0) became suspect (indicated by the circle) and had been punished (by Reputable Criminal 1). However, interpreting the aggression the agent Criminal 0 does not find a norm violation in its event board, the memory of the agent which stores possible norm violations in the past. For this reason the agent reacts by counter-aggression, namely physically attacking the agent Reputable Criminal 1. This is shown in Fig. 11-1. Fig. 11-2 (‘Reasoning of aggression’) shows the reasoning of this agent on the aggression faced by the agent Criminal 1. He finds that the offender is not reputable and for this reason excludes the possibility that the aggression had been a punishment. Fig. 11-3 (‘Failed assassination’) shows the reaction resulting from the reasoning process: namely an attempted assassination of the agent Criminal 0.
The fact that the agent Criminal 0 is still visible on the visualization interface of the model indicates that the attempt has been unsuccessful. For this reason now the other agent reasons on the aggression as shown in Fig. 11-4 (‘Reasoning on aggression’). This screenshot shows that the agent does not interpret the aggression as a death penalty. Potentially the aggression could have been a candidate norm invocation because the aggressor is a reputable agent. However, the agent finds no norm that might have been invoked in its event base. For this reason he reacts by a further aggression as displayed in Fig. 11-5 (‘Successful assassination’), again an attempt of an assassination. In this case the agent Criminal 0 successfully kills the agent Reputable Criminal 1. This is visualized by the disappearance of this agent from the visualization interface.
However, the fact that somebody has been killed can hardly be concealed. For this reason other agents are also affected by the event of the killing. The screenshot in Fig. 11-6 (‘Panic due to assassination’) illustrates the reaction of the other agents: Some did not observe the event. However, those that observed the death of the agent Reputable Criminal 1 react in panic. Here we stop the further elaboration of the simulation run but rather turn to the development of the narrative of this case.
Interpreting simulation: Growing criminal culture
The simulation runs show how agents act according to rules derived from categories developed by the researchers. This already includes cognitive elements and thus cannot be compared with the ‘I-am-a-camera’ perspective described by Geertz (1973) of a pure phenomenological description of the researcher’s observation. Nevertheless applying the researcher’s categories does not suffice for making sense of a culture from the perspective of the worldview of the participants. In terms of the account of a thick description this still needs to be qualified as a thin description. However, as shown in Fig. 10 the rules that are triggered during the simulation run can be traced back to in-vivo codes of the original textual documents. Following the account of a thick description, the central criterion for sustaining the credibility of a cultural analysis is a sense of verisimilitude (Ponterotto 2006) insofar that the reader gets a feeling that he or she could have experienced the described events (Denzin 1989). In the same vein, Corbin and Strauss (2008) introduce the notion of a storyline that provides a coherent picture of a case as the theoretical insight of a qualitative analysis.
For this reason in the description of the scenarios the rules are now traced back to the original annotations in order to develop narratives of the simulation runs, i.e. the scenarios are a kind of collage of the empirical basis of the agent rules. Thus, text passages of the police interrogations are decomposed and rearranged according to the rules triggered during the simulation run. These are tied together by a verbal description of the rules. In sum, this generates a kind of ‘crime novel’ to get in conversation with a foreign culture (Geertz 1973). Getting in conversation means that the reader would be able to understand interpretations from the perspective of the insiders’ worldview (Donmoyer 2001) and thereby be empowered to be able to react adequately (at least virtually). As the examples of blinking in the sunlight or secretly exchanging signs, stealing sheep, or – as in our example – making sense of a failed assassination indicate: being able to grasp a meaning of the phenomenology of action is essential for comprehending an adequate reaction or comprehending why the participants failed to react adequately. In our case of participatory research, the storyline of the simulations provides an archive of virtual experience for empowering the stakeholders (Creswell and Miller 2000; Cho and Trent 2006). Certainly we do not directly engage in the field for empowering criminals but rather with the police interacting in the field with the subjects under study. Therefore, we provide an example of how a storyline of the simulation described above will look. RC stands for reputable criminal, C for ordinary criminal and WC for white collar criminal, who is responsible for money laundering. Italics in the text indicate paraphrases of in-vivo codes (characteristic text passages of the original data) of the empirical evidence basis of the triggered rules.
“The drama starts with an external event. For unknown reasons C0, who never was very reputable became susceptible. It might be due to an unspecified norm violation, but it may not be so and just some bad talk behind his back. Eventually he stole drugs or they got lost. Then, RC 1 and RC4 decided to respond and agreed that C0 deserved to be severely threatened. The next day RC1 approached C0 and told him that he would be killed if he was not loyal to the group. C0 was really scared as he could not find a reason for this offence. He was convinced that the only way to gain reputation was to demonstrate that he was a real man. So he knocked the head of RC1 against a lamp post and then kicked him when he fell down to the ground. RC1 didn’t know what was happening to him, that such a freak as C0 was beating him up, RC1 one of the most respectable men of the group. There could only be one answer: He pulled his gun and shot. However, while shooting from the ground the bullet missed the body of C0[6]. So he was an easy target for C0. The latter had no other choice than pulling out his gun as well and shooting RC1 to death.
However, this gunfight decisively shaped the fate of the gang. When the news circulated in the group hectic activities broke out: WC bought a bulletproof car and C1 thought about a new life on the other side of the world, in Australia. In panic RC6 wanted to physically attack the offender. While no clear information could be obtained he presumed that C2 must have been the assassinator. So with brute force he assaulted C2 until he was fit for hospital. His head was completely disfigured, his eyes black and swollen. At the same time, RC0 and C2 agreed (wrongly) that it was C1 who killed RC1. While C2 argued that they should kidnap him, the more rational RC0 convinces him that a more modest approach would be wiser. He went to the house of C1 and told him that his family would have a problem if he ever did something similar again. However, when he came back, RC2 was already waiting for him: with a gun in his hand he said that in the early morning he should come to the forest to hand over the money.”
This brief ‘crime novel’ can be described as a ‘virtual experience’. Tracing the simulation runs back to the in-vivo codes of the empirical evidence base enables developing a storyline of a virtual case that provides a coherent picture of a case (Corbin and Strauss 2008). The narrative developed out of the simulation results, including some novel like dramaturgic elements, brings the simulation model back to the interpretative research which has been the starting point of the qualitative analysis in the first step of the research process. It enables to check if the cognitive heuristics implemented in the agent rules reveal observable patterns of behaviour that can be meaningfully interpreted. For this reason, the narrative description suggests to be a story of human actors for exploring the plausibility of the simulated scenarios. The plausibility check consists of an investigation whether the counterfactual composition of single pieces of empirical evidence remains plausible. This means to check if they tell a story that creates a sense of verisimilitude, first of all for the stakeholders, however, as we hope to also to the reader.
Concluding discussion
So far agent-based social simulation has not been used in interpretative research in cultural studies following the ‘understanding’ paradigm in the social sciences. By extending the generative paradigm to growing artificial cultures the paper demonstrates that it can be a useful tool for interpretive research as well. A research process is described to simulate the subjective worldview of participants in the field. Starting from an analysis of qualitative data via the development of a conceptual model to the development of a simulated narrative the research process fosters getting in conversation with foreign cultures. In the following some considerations shall be provided on how the methodology can provide insights and might be useful for further research.
As the particular example involved stakeholder participation during the overall research process, first the potential impact for the stakeholder shall be addressed: Criminal investigators look for evidence that suggests further directions of investigations, e.g. the police can only observe dead bodies on the street but not the motivation of the assassinators. The interpretative simulation provides a further source of (hypothetical) evidence beyond physical signs (such as fingerprints, etc.) on a cognitive level, which provides insights into possible motivations for actions. This can be described as virtual experience. Empirically this research strategy might be useful for investigating all kinds of ambiguous situations: complex situations with a high degree of uncertainty in which many decisions are possible and therefore many different outcomes are possible as well. Examples include, for instance, corruption but certainly also problem fields outside the domain of criminology.
In the field of social simulation, the proposed methodology provides a new contribution for the computational study of culture (Dean et al. 2012; Dignum and Dignum 2013) beyond reducing culture to agents’ attributes (such as colour: e.g. Axelrod 1997) or applying certain theories in the agents’ design by allowing agents to grow culture (i.e. patterns to interpret and react accordingly to courses of actions of other agents) in the course of a simulation run. Moreover, a growing interest in including qualitative data can be observed in the field of social simulation as for instance indicated in a special issue of JASSS on using qualitative evidence to inform the specification of agent models in 2015. The methodology presented here goes one step further by using qualitative (namely: interpretative) methods for the analysis of agent-models. This coincides with current tendencies of cognitively rich agent architectures for understanding social behaviour (e.g. Campenni 2016) as technically the interpretation is generated by a socio-cognitive coupling of an agent’s reasoning on other agents’ state of mind. This feature might provide a direction to explore in future research in developing context sensitive simulation (Edmonds 2015b).
Concerning the impact of the proposed methodology on contemporary qualitative research it has to be admitted that a practical limitation for its application is that the required computational skills are rather demanding. However, as the simulation is intimately interwoven with the data (as the ‘fact base’ of the simulation), the scenarios generated by the simulation enable a systematic exploration of the data with regard to the question of what could be called the horizon of a cultural space. Obviously, simulation results depend on the prior coding in the data analysis phase, as during the simulation run only those ‘facts’ can be executed that have already been coded in the qualitative data analysis. For instance, in the case of our example these concentrate on modes of conflict resolution and aggression. Therefore, the reasoning of the agents about the state of mind of other agents is restricted to such cases. Nevertheless, simulation as a method of data exploration might be of interest for qualitative researchers, namely by rearranging the codings of the qualitative data analysis in a new composition and tracing these back to the in-vivo codes for composing a narrative of a simulated case. Thereby the simulation enables a systematic exploration of qualitative, textual data. This can be described as a horizon of a cultural space, namely what course of action can plausibly be undertaken in a certain cultural context. Note that one criterion for the credibility of qualitative research is the sense of verisimilitude (Ponterotto 2006). For this purpose the generation of narratives by the simulation model is helpful in particular for the counterfactual scenarios. This provides a criterion for ensuring a sense of verisimilitude: checking whether the coding generates a storyline in different combinations (in the modelling phase) increases the credibility of the prior coding in the analysis phase. For this reason we hope to attract the interest also of qualitative researchers.
Acknowledgements
The research leading to these results has received funding from the European Union's Seventh Framework Programme (FP7/2007-2013) under grant agreement n° 315874., GLODERS Project.Notes
- It has to be emphasized that criminal culture cannot be reduced to norms or modes of conflict resolution in particular criminal organizations (see also Bright et al. 2015). These are central to the example used here because of the particular interest of the stakeholders. A fully fledged review of the literature is beyond the scope of this article. Investigations of criminal norms go back at least to the work of Donald Cressey (e.g. Irwin and Cressey 1962, see also Cressey’s investigation of the American Cosa Nostra e.g. in Cressey 1969). Moreover, criminal or deviant culture has been subject of historical studies (e.g. Wiener 1994) as well as the social construction of deviance (Foucault 1977). Often crime is associated with low self-control (Spahr and Allison 2004). A brief overview of the concept of organized crime can also be found in Neumann and Elsenbroich (2017). However, for the methodological purpose one example of an element of culture (in this case: norm enforcement) shall be sufficient to illustrate the research process of growing artificial cultures.
- Member checking denotes a method for ensuring the credibility of qualitative research in which the results are presented to the research subject to examine whether the interpretation of the researcher of how the subjects make sense of a situation from the perspective of their worldview is plausible for the research subjects. In the case of participatory research the subjects are the stakeholders (Cho and Trent 2006).
- To preserve privacy of data, names have been replaced by notations such as M., V01, etc.
- It should be noted that the alternative interpretation can also be found as illustrated in the following in-vivo code: “I paid but I’m alive.”
- For instance, in this example the Computational Normative Theory developed by GLODERS had been taken into account. The normative reasoning is put into action in the simulation by dedicated rules working on a specific part of the agent memory for storing norm-related information, based on the empirical facts of this scenario. Details on this theoretical integration can be found in Neumann and Elsenbroich (2017).
- Note that in the following the description slightly deviates from the story developed in the simulation: In the simulation the agents reason about the aggression. In contrast here, there is an immediate shooting, which might be regarded as ‘ad-hoc’ reasoning. Similar events can be found in descriptions of other cases of fights between criminals, as for instance the Sicilian Cosa Nostra (Arlacchi 1993).
References
ARLACCHI, P. (1993). Mafia von innen – Das Leben des Don Antonino Calderone. Frankfurt a.M.: S. Fischer Verlag.
AXELROD, J. (1997). The dissemination of culture. A model with local convergence and global polarization. Journal of Conflict Resolution 41(2): 203-226. [doi:10.1177/0022002797041002001 ]
BARRETEAU, O. et al. (2003). Our companion modelling approach. Journal of Artificial Societies and Social Simulation 6(2), 1: https://www.jasss.org/6/2/1.html.
BENNETT, A. (2000). Popular music and youth culture: Music, identity, and place. London: Palgrave.
BLEY, R. (2014). Rockerkriminalität. Erste empirische Befunde. Frankfurt a.M.: Verlag für Polizeiwissenschaft.
BOUCHARD, M., & Quellet, F. (2011). Is small beautiful? The link between risk and size in illegal drug markets. Global Crime 12(1): 70 – 86. [doi:10.1080/17440572.2011.548956 ]
BRIGHT, D., Greenhill, C., Ritter, A., & Morselli, C. (2015). Networks within networks: Using multiple link types to examine network structure and identify key actors in a drug trafficking operation. Global Crime 16(3): 219 – 237. [doi:10.1080/17440572.2015.1039164 ]
BURG, M.B., Peeters, H., & Lovis, W.A. (eds.) (2016). Uncertainty and Sensitivity Analysis in Archaeological Computational Modeling. Heidelberg: Springer. [doi:10.1007/978-3-319-27833-9 ]
CAMPENNI, M. (2016). Cognitively Rich Architectures for Agent-Based Models of Social Behaviors and Dynamics: A Multi-Scale Perspective. In: F. Cecconi (ed.), New frontiers in the study of social phenomena. Heidelberg: Springer. [doi:10.1007/978-3-319-23938-5_2 ]
CHO, J., & Trent, A. (2006). Validity in qualitative research revisited. Qualitative Research 6(3), 319 – 340. [doi:10.1177/1468794106065006 ]
CONTE, R., Andrighetto, G., & Campenni, M. (2014). Minding norms: mechanisms and dynamics of social order in agent societies. Oxford: Oxford University Press.
CORBIN, J., & Strauss, A. (2008). Basics of qualitative research. 3rd edition. Thousand Oaks: Sage.
CRESSEY, D. (1969). Theft of the nation: The structure and operations of organized crime in America. New York: Harper & Row.
CRESWELL, J.W., & Miller, D.A. (2000). Determining validity in qualitative inquiry. Theory into Practice 39(3), 124 – 130. [doi:10.1207/s15430421tip3903_2 ]
DEAN, J.S., Gumerman, G. J., Epstein, J. M., Axtell, R. I., Swedlund, A. C., Parker, M. T., & Mccarroll, S. (2012). Understanding Anasazi culture change through agent-based modeling. In Generative Social Science: Studies in Agent-Based Computational Modeling. (pp. 90 – 116). Princeton University Press. [doi:10.1515/9781400842872.90 ]
DENZIN, N.K. (1989) The Research Act: A Theoretical Introduction to Sociological Methods, 3rd Edition. New Jersey: Prentice Hall.
DIGNUM, V., & Dignum, F. (2013) (eds.). Perspectives on culture and agent-based simulations. Heidelberg: Springer.
DILAVER, O. (2015). From Participants to Agents: Grounded Simulation as a Mixed-Method Research Design. Journal of Artificial Societies and Social Simulation 18(1), 15: https://www.jasss.org/18/1/15.html [doi:10.18564/jasss.2724 ]
DILTHEY, W. (1976). Wilhelm Dilthey: Selected writings. Cambridge: Cambridge University Press.
DONMOYER, R. (2001). Paradigm Talk Reconsidered. In: V. Richardson (ed.), Handbook of Research on Teaching, 4th Edition (pp. 174 – 97). Washington, DC: American Educational Research Association.
EDMONDS, B. (2015a). Using Qualitative Evidence to Inform the Specification of Agent-Based Models. Journal of Artificial Societies and Social Simulation 18(1), 18: https://www.jasss.org/18/1/18.html. [doi:10.18564/jasss.2762 ]
EDMONDS, B. (2015b). A Context- and Scope-Sensitive Analysis of Narrative Data to Aid the Specification of Agent Behaviour. Journal of Artificial Societies and Social Simulation 18(1), 17: https://www.jasss.org/18/1/17.html. [doi:10.18564/jasss.2715 ]
EPSTEIN, J. (2006). Generative social science. Studies in agent-based computational modelling. Princeton: Princeton University Press.
EPSTEIN, J. & Axtell, R. (1996). Growing artificial societies. Social science from the bottom-up. Cambridge MA.: MIT Press.
FIELDHOUSE, E., Lessard-Phillips, L., & Edmonds, B. (2016). Cascade or echo chamber? A complex agent-based simulation of voter turnout. Party Politics 22(2), 241 – 256. [doi:10.1177/1354068815605671 ]
FOUCAULT, M. (1977). Discipline and punishment. London: Allan Lane.
GEERTZ, D. (1973). Thick Description: Toward an Interpretive Theory of Culture. In: The Interpretation of Cultures: Selected Essays (pp. 3 – 30). New York: Basic Books.
GHORBANI, A., Dijkema, G., & Schrauwen, N. (2015). Structuring Qualitative Data for Agent-Based Modelling. Journal of Artificial Societies and Social Simulation, 18(1), 2: https://www.jasss.org/18/1/2.html. [doi:10.18564/jasss.2573 ]
GIBBS, J. (1965). Norms: the problem of definition and classification. American Journal of Sociology 70(5): 586–594. [doi:10.1086/223933 ]
GLASER, B., & Strauss, A. (1967). The discovery of Grounded Theory. Strategies for qualitative research. Chicago: Aldine.
HAMMERSLEY, M., & Atkinson, P. (1995). Ethnography: Principles in Practice, 2nd Edition. London: Routledge.
HEDSTRÖM, P., & Ylkoski, P. (2010). Causal mechanisms in the social sciences. Annual Review of Sociology 36, 49 – 67. [doi:10.1146/annurev.soc.012809.102632 ]
INTERIS, M. (2011). On norms: A typology with discussion. American Journal of Economic Sociology 70(2): 424 – 438. [doi:10.1111/j.1536-7150.2011.00778.x ]
IRWIN, J., & Cressey, D. (1962). Thieves, convicts and the inmate culture. Social Problems 10(2): 142 – 155. [doi:10.2307/799047 ]
ISABELLA, L. (1990). Evolving interpretations as change unfolds: How managers construe key organizational events. Academy of Management Journal 33(1): 7 – 41. [doi:10.2307/256350 ]
KOHLER, T., & Gumerman, G. (eds.) (2000). Dynamics in human and primate societies. Oxford: Oxford University Press.
LE PAGE, C., Bobo, K.S., Kamgaing, T.O.W., Ngahane, B.F.N., & Waltert. M. (2015). Interactive Simulations with a Stylized Scale Model to Codesign with Villagers an Agent-Based Model of Bushmeat Hunting in the Periphery of Korup National Park (Cameroon). Journal of Artificial Societies and Social Simulation, 18(1), 8: https://www.jasss.org/18/1/8.html.
LINCOLN, Y.S., & Guba, E.G. (1985). Naturalistic Inquiry. Beverly Hill, CA: Sage.
LOTZMANN, U., Meyer, R. (2011). A Declarative Rule-Based Environment for Agent Modelling Systems. The Seventh Conference of the European Social Simulation Association, ESSA 2011. Montpellier, France.
LOTZMANN, U., Wimmer, M. (2013). Evidence Traces for Multi-agent Declarative Rule-based Policy Simulation. In: Proceedings of the 17th IEEE/ACM International Symposium on Distributed Simulation and Real Time Applications (DS-RT 2013) (pp. 115-122). IEEE Computer Society. [doi:10.1109/ds-rt.2013.20 ]
LOTZMANN, U., Neumann, M., Moehring, M. (2015). From text to agents. Process of developing evidence based simulation models. In: 29th European Conference on Modelling and Simulation, ECMS 2015 (pp. 71-77). European Council for Modeling and Simulation.
LOTZMANN, U., Neumann, M. (2017). A simulation model of intra-organisational conflict regulation in the crime world. In C. Elsenbroich, N. Gilbert, D. Anzola (eds.). Social Dimensions of Organised Crime: Modelling the Dynamics of Extortion Rackets (pp. 177-213). New York: Springer.
MALINOWSKI, B. (1922). Argonauts of the Western Pacific. An Account of Native Enterprise and Adventure in the Archipelagoes of Melanesian New Guinea. New York: Dutton.
MEAD, M. (1928). Coming of age in Samoa. A psychological study of primitive youth for western civilisation. New York: Morrow & Company.
MITHEN, S. (1994). Simulating prehistoric hunter-gatherer societies. In N. Gilbert, J. Doran (eds.), Simulating Societies. London: UCL Press.
MÖLLENKAMP, S., Lamers, M., Huesmann, C., Rotter, S., Pahl-Wostl, C., Speil, K., & Pohl, W. (2010). Informal Participatory Platforms for Adaptive Management. Insights into Niche-finding, Collaborative Design and Outcomes from a Participatory Process in the Rhine Basin. Ecology and Society 15 (4), 41. [doi:10.5751/ES-03588-150441 ]
NARDIN L.G., Székely, Á. & Andrighetto, G. (2016). GLODERS-S: a simulator for agent-based models of criminal organisations. Trends in Organized Crime, online first. [doi:10.1007/s12117-016-9294-z ]
NEUMANN, M., & Elsenbroich, C. (2017). Introduction: The societal dimensions of organized crime. Trends in Organized Crime (online first, open access). [doi:10.7148/2014-0765 ]
NEUMANN, M., Lotzmann, U. (2014). Modelling the Collapse Of A Criminal Network. In: F. Squazzoni, F. Baronio, C. Archetti, M. Castellani (eds.), 28th European Conference on Modelling and Simulation, ECMS 2014 (pp. 765-771). European Council for Modeling and Simulation.
NEUMANN, M., Lotzmann, U. (2017). Text data and computational qualitative analysis. In C. Elsenbroich, N. Gilbert, D. Anzola (eds.), Social Dimensions of Organised Crime: Modelling the Dynamics of Extortion Rackets (pp. 155-176). New York: Springer.
NGUYEN-DUC & Drogul (2007). Using Computational Agents to Design Participatory Social Simulations. Journal of Artificial Societies and Social Simulation, 10(4), 5: https://www.jasss.org/10/4/5.html.
NORTH, M.J., Collier, N.T., & Vos, J.R. (2006). Experiences Creating Three Implementations of the Repast Agent Modeling Toolkit. ACM Transactions on Modeling and Computer Simulation 16(1) (Jan.), 1-25.
PONTEROTTO, J.G. (2006). Brief note on the origins, evolution, and meaning of the qualitative research concept thick description. The Qualitative Report 11(3), 538 – 549.
RYLE, G. (1971). Collected papers. Volume II collected essays, 1929-1968. London: Hutchinson. [doi:10.1007/s10506-013-9142-2 ]
SCHEELE, B., & Groeben, N. (1989). Dialog-Konsens-Methoden. Zur Rekonstruktion subjektiver Theorien. Tuebingen: Franche Verlag. [doi:10.18564/jasss.2834 ]
SCHERER, S., Wimmer, M., & Markisic, S. (2013). Bridging narrative scenario texts and formal policy modeling through conceptual policy modeling. AI and Law 21(4), 455 – 484.
SCHERER, S., Wimmer M., Lotzmann, U., Moss, S., & Pinotti, D. (2015). An evidence-based and conceptual model-driven approach for agent-based policy modelling. Journal of Artificial Societies and Social Simulation 18(3), 14: https://www.jasss.org/18/3/14.html.
SPAHR, L., & Allison L. (2004). US savings and loan fraud: Implications for general and criminal culture theories of crime. Crime, Law, and Social Change 41: 95 – 106.
SQUAZZONI, F., Jager, W., & Edmonds, B. (2014). Social simulation in the social sciences. A brief overview. Social Science Computer Review 32(3), 279 – 294. [doi.org/10.1177/0894439313512975 ]
WIENER, M. (1994). Reconstructing the criminal: Culture, law, and policy in England, 1830-1914. Cambridge: Cambridge University Press.