Introduction
The evolution and persistence of social norms is one of the core topics of social theory. Social norms form the core of grand sociological theories such as Parsonian systems theory (cf. Joas & Knöbl 2013, chapter 2-4), and more recently, even proponents of rational choice (Gintis 2009) and analytical sociology (Kroneberg 2014) emphasize the explanatory importance of social norms. The meaning of the term social norm is thereby fairly broad: In general, it describes a complex of behavioural patterns, expectations and potential sanctions.
Traditionally, social norms have mostly been considered as solutions to problems of cooperation and coordination in social groups (Raub et al. 2015). Rational choice theory in particular often provides descriptions that make successful cooperation in human societies seem almost miraculous. Since the seminal work of Axelrod (1984), various models, in particular, computational models, have been developed to explain the evolution and persistence of social norms and cooperation in a world of limited resources and egoism (for a recent example, see Skyrms 2004, 2014).
However, beyond this world of overall beneficial norms that allow their subjects to cooperate in complex social settings, there are also norms that appear to be largely detrimental to their subjects: So-called unpopular norms. These are norms that hurt the interests of the majority or even all its subjects, without being coerced onto the population from outside; for example, an enforced code of conduct in a prison does not constitute an unpopular norm, no matter how unpopular it is among the prisoners. If, on the other hand, the prison guards perceive a norm of strictness and hostility against the prisoner and align their behaviour with that norm against their actual private preferences, an unpopular norm has been constituted. In fact, this example is not far-fetched, since social psychologists discovered such a pattern in the U.S. penitentiary system (Kauffman 1981).
How do such norms arise, and how are they maintained? In some cases, the answer is relatively simple. For example, in a corrupt political system, bribery might be the established social norm. Such a situation is easily modelled as an instance of a Prisoner’s Dilemma: If firm A stops bribing while firm B goes on, firm A will lose valuable contracts and eventually run out of business; but – at least potentially – both firms would prefer a situation where neither A nor B would bribe (defect, in terms of the Prisoner’s Dilemma). One can understand such ambiguous cases in various ways. One option is, unlike Bicchieri & Fukui (1999), to argue that such cases should not be classified as norms at all. The expectations of the members of the relevant population might not be sufficient to constitute a social norm. To clarify this, it has to be laid out in more detail what exactly constitutes a social norm[1]. An alternative stance is to explain some unpopular norms in terms of rational choice, while others require a more subtle psychological and sociological explanation, as pluralistic ignorance. While such contentious cases do not offer a solid grounding for a theoretical account of the latter kind of explanation, there are also genuinely puzzling cases.
Such puzzlement is effected most strongly in cases where unilateral deviation appears to be advantageous to at least some of the agents. In fact, social psychologists have suggested norms of alcohol consumption of a college campus as a case study (Prentice & Miller 1993). Their data supports the hypothesis that students systematically misperceive the preferences of their fellow students with respect to the excessive consumption of alcohol. Similar patterns have also been reported in cases of sexual promiscuity (Lambert et al. 2003). In both cases, the behavioural expectation leads students to behave in potentially harmful ways against their own actual preferences. These cases call for an explanation in its own right[2].
Bicchieri & Fukui (1999), among others, have argued that a major source of unpopular norms is pluralistic ignorance. In a social group, behaviour is often coordinated by perceiving behaviour and inferring a preference to coordinate with from the observations. This process of social influence appears to be evolutionary successful, establishing widely accepted behavioural expectations with limited resources. However, limited information or misinterpreted behaviour can also start a cascade of misrepresentation and failed communication of true preferences, resulting in an unpopular norm.
Once the norm is thereby established, it reproduces the suboptimal behaviour and maintains a system of misrepresentation of preferences. If a strict system of sanctions is implemented to protect the norm, it can survive indefinitely, as it appears to be the case with certain anachronistic religious norms. However, if sanctions are weak or powerful external events shake up the social system, an unpopular norm is expected to be very fragile. Once successful communication is enabled, the norm’s subjects have strong incentive to deviate and dethrone the norm.
The open question is how to formalize this informal theoretical account of the growth of unpopular norms. In the spirit of generative social science (cf. Epstein 1999, p. 43): If you didn’t grow it, you didn’t explain the phenomenon. Agent-based modelling (ABM) provides an extremely useful tool to develop a model from informal theory. The main contributions of this paper are thus:
- The development of an agent-based model providing a potential explanation of the evolution, maintenance and decline of unpopular norms
- An analysis of the dynamics underlying the end result of an unpopular norm
- Qualitative comparisons with empirical results from both survey and experimental research
- The provision of an easily extensible baseline model, as shown by the addition of central influences
The paper will proceed in the following way: First, the empirical literature on pluralistic ignorance is reviewed to extract a qualitative benchmark for the model, followed by a critical examination of an existing theoretical model and the concept of social norms assumed for the analysis. Next, a network-based ABM is introduced and analysed in various simulation experiments. The paper concludes with a consideration of the model’s limitations and a short summary and a review of open questions for future research.
Related Work
Empirical Background
Pluralistic ignorance has been shown by social psychologists in a variety of contexts. Students of U.S. universities misperceive the expectations and preferences of fellow students with respect to excessive alcohol consumption (Prentice & Miller 1993) and so-called hooking-up behaviour (Lambert et al. 2003). In the study by Prentice and Miller, the actual preferences on alcohol consumption where approximately uniformly distributed on their scale. The perceived preference of other students, however, turned out to be normally distributed with a significantly higher mean than the preference distribution – where the preference of the other students is also interpreted by the study’s subjects as their expectation towards them[3]. The transformation of the uniform to a normal distribution can be explained as the students trying to coordinate on a common norm expectation. The shift in the mean then shows the failure of the social influence mechanism to find the optimum.
Similar patterns are also found outside college campuses. Prison guards in the United States turned out to misperceive their colleagues as way less liberal than themselves and than they actually were (Kauffman 1981). The perception of the support for segregation among white men is another case where the members of a group failed to recognize the actual distribution of preferences (O’Gorman 1975). Bicchieri & Fukui (1999) argue that even norms of corruption can be the product of a social failure to reveal preferences.
Importantly, not all cases of pluralistic ignorance lead to the establishment of an unpopular norm. Bystander scenarios (Darley & Latane 1968) can be explained by pluralistic ignorance, i.e., the failure to correctly infer another person’s mental states from behaviour, but the problem is limited to a specific situation. Pluralistic ignorance is a mechanism that potentially creates an unpopular norm, if it is sustained in a population over time. The studies cited above focus mostly on measuring the deviation between private preference and behavioural expectation. While this deviation is a valid operationalization of the construct of an unpopular norm, it does not help much in understanding the underlying dynamics of pluralistic ignorance which are supposed to explain the pattern of expectations.
The study by Shamir & Shamir (1997) provides such a dynamic picture. They investigated the development of the political climate in Israel prior to the election of 1992. They show that private opinion became more and more friendly to the idea of returning territory, but the perceived political climate remained almost stable. Their explanation relies on the limited information on which Israeli citizens formed their opinions, in particular the strong influence of the incumbent conservative government. The spell was broken by the actual outcomes of the election that led to government turnover, therefore revealing the private preferences in a secret election.
Coming from a very different methodological angle, Gërxhani & Bruggeman (2015) observed such a lag in an experimental setting. Constraints on communication allow a certain perception of social expectation to last when its actual support is decreasing. In both settings, the perceived shared belief is adapting eventually; but in the political climate case at least, a significant change took place only after an important external event. Thus, at least in some cases, an unpopular norm can survive almost indefinitely if unchallenged[4].
It is also worth noting that according to Shamir & Shamir (1997), there are two kinds of pluralistic ignorance: absolute and relative. In a case of absolute pluralistic ignorance, the population chooses the option that is least preferred by the majority. Politics offer a simple example: When the party despised by the majority successfully is elected in a two-party system that constitutes a case of absolute pluralistic ignorance. If a population of students perceives the mean preference for alcohol consumption by 2 points on an 11-point scale, it is in a state of relative pluralistic ignorance. Theoretically, relative pluralistic ignorance is the more fundamental phenomenon, while absolute pluralistic ignorance is rather a product of binary choices imposed on a population. It is easy to imagine how a relative misperception of the political climate could be transformed into a case of absolute pluralistic ignorance via binary choice: The misperception just has to shift the behavioural expectation across a party divide.
From these empirical insights, one can derive some important qualitative benchmarks for a simulation model that tries to explain unpopular norms by pluralistic ignorance:
- It must be able to reproduce the basic pattern of a deviation between the mean of the preference distribution and the distribution of norm perception.
- It should include variable constraints on the available information to constitute a model of pluralistic ignorance.
- Ideally, it reproduces the dynamical behaviour qualitatively, i. e. it exhibits the above mentioned lag of the dynamic of the norm expectation distribution behind the preference distribution.
There is one feature of all these empirical studies that has not yet been mentioned, since it does not concern their descriptive content or explanatory value. The issues investigated all seem to be of high normative relevance. Excessive alcohol consumption, sexual conduct, the penitentiary system and Israel’s territorial policy can all be considered ethical problems, too. While the authors do not explicitly claim anything normative, they can consistently be interpreted as ascribing collective irrationality to a group following an unpopular norm. If that reading were appropriate, it would imply that seemingly ethical problems are in fact failures of rationality and could be resolved by improving collective judgment. These normative implications are not the main concern of this paper, but the discussion of the simulation results will return to this important issue briefly.
Theoretical Models
The landscape of theoretical models of the evolution of unpopular norms is rather sparsely populated compared to its empirical counterpart. In fact, there are only two attempts to model the process formally. The first one is a model by Centola et al. (2005) that relies on false enforcement as its main mechanism. False enforcement denotes the phenomenon that agents might enforce a norm they privately disagree with to prove their allegiance to the rest of the social group. While false enforcement is a potential explanation for unpopular norms, the mechanism is very specific and hard to detect empirically. None of the above-cited empirical research offers any evidence of false enforcement, making it relatively hard to validate or invalidate this particular model.
The second model has been suggested by Bicchieri (2005, chap. 6). This model, referred to as BM from here on, relies on pluralistic ignorance in a binary choice situation only. For example, the agents represented in BM might have to decide to litter the street or keep it clean, both of which could be the norm in a given neighbourhood. The chosen action of an agent \(i\) is denoted by \(x_i \in \{x_1, x_2\}\). In addition, the agent has a private preference \(y\) and a degree of nonconformism \(\beta\). There is a “true majority” with respect to preference: Any agent is a member of that majority with probability \(p\). There are two kinds of agents: Conformists (\(\beta=0\)) and trendsetters \(\beta=1\)). In addition, trendsetters lose \(\theta\) if they suspend their choice. The overall utility of an agent’s choice is described by the function
$$ U_i = -(x_i - \hat x)^2 -\frac{\beta}{2}(x_i - y)^2 - \theta $$ | (1) |
Bicchieri has shown that informational cascades take place in this model, both positive, i.e., revealing the true majority, and negative, leaving the population in a state of absolute pluralistic ignorance. In addition, it is possible that the trendsetters do not provide a clear signal to the conformists. In such a case, the conformists fall back on a coin flip, resulting in a partial cascade. While the model offers a first impression as to how pluralistic ignorance can be formalised to grow an unpopular norm, it has some significant shortcomings. The model does not meet the first of the above benchmarks, since it cannot reproduce a pattern of relative pluralistic ignorance. The second benchmark is met, since informational constraints are successfully endogenised in its utility functions. However, such a conceptualization makes it difficult to model a structurally more complex communication environment. It would be desirable to decompose what is compounded in the utility function in BM, namely structural features of the informational environment, psychological features of the agents, and variables that are to be understood purely in terms of rational reconstruction. Finally, the model does not exhibit any real dynamics. There is only a two step process that does not allow for a more fine grained analysis of the dynamics of the system. Finally, it is too restrictive to assume trendsetters that are psychologically different. The role of an agent is often determined by structural features of the social environment rather than individual psychological features. That is not to say that individual differences are not potentially important for the process, but they should not be a necessary condition for pluralistic ignorance. Nevertheless, BM provides a starting point to develop a more sophisticated model of the process in question, a task at which the model proposed here is aimed. But before the discussion can proceed in that direction, it needs to be stated explicitly how the concept of a social norm is understood.
Social Norms
Given the reliance on Bicchieri’s model of pluralistic ignorance, her definition of social norms is a natural starting point. According to that account (cf. Bicchieri 2005, p. 11), a social norm is a behavioural rule \(R\) applicable to a situation \(S\) representable as a mixed-motive game. It exists in a population \(P\), if there is a sufficiently large number \(P_{cf} \subset P\) exists, such that for each \(i \in P_{cf}\), the following conditions hold:
- \(i\) knows about \(R\) and its applicability to \(S\).
- \(i\) prefers to act according to \(R\) if \(i\) beliefs there is a sufficiently large set of agents who follow \(R\) in situations of type \(S\) and an analogous (not necessarily identical) subset of \(P\) exists, which expects \(i\) to conform to \(R\) in situations of type \(S\), potentially also willing to punish \(i\) for violating the norm.
This definition certainly encompasses some of the important features of social norms also noted by Elster (1989): They are socially shared, i.e., not simply individual habits, they come with normative expectations unlike simple collective patterns of behaviour, and they do not require codification. In fact, the expectation of sanction is not a necessary condition for a social norm to exist. Note that, according to this definition, a norm can exist but not be followed, since the preference is conditional. While this definition is prima facie quite plausible, there are two major points in need of closer inspection.
First, it offers a rational reconstruction, which, according to Bicchieri (2005, p. 11f.) implies that the beliefs and preferences mentioned in the definition are not necessarily identifiable with beliefs and preferences within the agents. At a first glance, that is an important concession to remain plausible, since Elster (1990) argued extensively that some norms are not plausibly explained rationally, in particular certain norms of revenge[5]. However, this concession also raises the question about how to explain in any given concrete case the existence of a norm, and the motivation to follow it. This is not a principled problem, but it highlights an issue potentially concealed by the rational reconstruction: There are likely numerous different explanations for various cases of social norms, and to offer an actual explanation, one has to specify what takes the place of the rational implementation of preferences in the rational reconstruction[6].
Second, the assumption that norms only govern phenomena that are faithfully represented by mixed-motive games is too restrictive. Gender-role norms, for example, are often to be represented by asymmetric coordination games[7]. A lot of norm-governed behaviour might also be better described by a decision under risk or uncertainty without a strategic element, where the social component enters only through an additional norm requiring an agent to punish. This idea of norm that requires an agent to sanction is not dissimilar to that of a meta-norm (Axelrod 1986) requiring agents to punish agents who fail to sanction norm violations, since it is logically independent of the actual norm[8]. This implies the possibility of a scenario, where the social norm on the non-interactive behaviour is in place, but the punishment norm, which would allow to interpret the whole as a mixed-motive game, does not exist in the population. For example, consider norms against masturbation in a world where no one is expected to actually sanction it; One would have to construct a seriously convoluted argument to represent the norm-governed situation as a strategic interaction[9].
Elster (1989) avoids such problems by giving a negative definition in terms of what is not a social norm – i.e., legal norms, habits, moral norms – and providing some examples – norms against cannibalism or incest – to offer positive guidance of what is to be understood by the talk of social norms. Where does that leave the modeller, intending to explain the emergence of a particular kind of norm? While there is no convincing philosophical definition stating the necessary and sufficient conditions available, one can build on Bicchieri’s account by relaxing the problematic components and adding the caveat that it might not offer a perfect demarcation criterion for the class of phenomena accurately referred to as social norms.
Therefore, for the following, a social norm is a behavioural rule \(R\) for situations of type \(S\), for which the second condition in the above definition is fulfilled. In addition, the rule can allow for some “slack”: Even if a situation is classified as an instance of \(S\), there can be a room to behave slightly deviant without violating the norm. This is a useful clarification for cases of fuzzy norms. For example, norms of fairness often do not exactly specify what a fair split is, as experiments based on the ultimatum game suggest (cf. Roth et al. 1991). This fuzziness is likely a tribute to the limitations of human norm-followers, both with respect to their epistemic powers and the control over their actions. A final remark on this notion of a social norm: It is, for any practical case, difficult to establish the existence of a social norm, since it is impossible to spell out the meaning of “sufficiently large subpopulation” unambiguously. However, the ambiguity seems unavoidable given that, similarly to the concept of a heap, establishing the amount of deviation marking the applicability of the concept of a social norm is arbitrary. However, for the purpose of explaining clear-cut cases of unpopular norms, potentially contentious borderline cases do not pose a problem. Hence, the discussion can now turn to the model.
Model
The basic process of the model consists of agents entering a social network and choosing their observable behaviour from a range of options. They know the behaviour of their neighbours on the network and their own private preference, but neither the global distribution of choices nor anyone else’s private preferences. The theoretical assumption behind this model outline is the bounded rationality of the agents: They have limited information and limited capability to make their decision, and therefore rely on a simple behavioural heuristic to consider their preferences and the cues provided by a small number of other agents.
The model description therefore has two major parts: The decision rule for the agents and the network growth algorithm determining the evolution of the social structure.
Decision Under Social Influence
Every agent has two properties: Their private preference, which is assigned on initialization, and their chosen behavioural norm, which is visible to other agents. Which agents actually perceive the norm choice is defined by the network structure. Since the model is meant for relative pluralistic ignorance, the preference is chosen uniformly at random from \([1, \dots, 11]\). This kind of scale is able to represent the commonly used Likert-scales from research on pluralistic ignorance and unpopular norms. When agent enter the network, they have to choose a behavioural norm, again from \([1, \dots, 11]\). The goal of the choosing agent is to accord with the prevailing norm. Given that agents are part of the population, their own preferences will also figure in the decision. The rationale is that the agent tries to fulfil the social expectations based on other agents' preferences. They cannot access them directly and so use their behaviour as a proxy. With respect to themselves, however, the actual preference is known.
This leads to the stipulation of the following payoff scheme: For an agent \(i\) with the preference \(p_i \in [1, \dots, 11]\), the behavioural norm choice \(c_i \in [1, \dots, 11]\) and the accessible set of agents \(N\), the goal function to maximize is defined as
$$G_i(c_i) = - |\frac{1}{|N|+1} (w_i(i) p_i+\sum_{j \in N} w_i(j) c_j)-c_i| $$ | (2) |
Within the model, some constraints on Equation 2 are necessary. First of all, it will be assumed that all weights are equal, representing that agents have no particular reason to give a particular agent more weight. If an environment exists that has actual power differentials, this would be an invalid assumption, but in our case, this is a reasonable idealization. Second, agent information is limited to network neighbours. This constraint is in fact a crucial component of the model, and leads to the localised version of conformism that agents are actually able to follow,
$$G_i(c_i) = - |\frac{1}{|\Gamma_i|+1} (p_i+\sum_{j \in \Gamma_i} c_j)-c_i|$$ | (3) |
$$G_i(c_i) = - |\frac{|p_i - c_i| + \sum_{j \in \Gamma_i} |c_j - c_i|}{|\Gamma_i| + 1}| $$ | (4) |
Note that agents without a reference group will always behave on the basis of their own preference. Therefore, the decision rule is homogeneous across the population, including an initial set of unconnected agents. This avoids the assumption made in BM that trendsetters are psychologically special; instead, the difference is explained by social structure alone. Both pure explanations are likely incomplete, but since it is one of the main motivations for the current model to include social structure, the design focuses on this aspect omitted in BM.
It is not necessary to imagine the decision as conscious, although the concepts of computation and choice are involved. The model is supposed to be instrumentally valid, but the choice procedure can also be interpreted realistically in various ways. Given the interpretation of \(c_i\) as the choice of a behavioural pattern potentially sanctioned by a social norm, the decision procedure can be interpreted at least in the following ways: First, it can represent an agent's anticipation of sanction for deviation. This anticipation creates an incentive to conform as closely to the perceived norm as possible, given the agent's limited information. Less rationalistically, agents could also have a metapreference to conform to their neighbours for the sake of coordination itself. Finally, agents could also assume that other agents are onto something in that their choices are more beneficial. This interpretation implies that agent preferences cannot help to judge the efficiency of the established norm, which can be true in some cases but should not be generally assumed.
Network Growth
There are various different algorithms to construct networks with certain statistical properties. A problem with classical random graph models (Erdös & Rényi 1960) or small-world networks (Watts & Strogatz 1998) is that they are not designed to grow dynamically. While it is possible to reformulate them in a way to address this issue, there is also an elegant and likely more realistic alternative in the preferential attachment model (Barabási & Albert 1999).
The preferential attachment algorithm starts with a small initial network of \(m_0\) nodes. Additional nodes then become iteratively attached to the network by randomly generating \(m\) edges with existing nodes. The new edges are not chosen uniformly at random, but the probability depends on the current network structure. More precisely, the more network links an old node \(i\) already has, the more likely it is that a new node will attach itself to that node. Formally, the probability that a freshly created link includes \(i\) is defined as
$$p_i = \frac{k_i}{\sum_j k_j}$$ | (4) |
- Its statistical properties are quite well understood. For example, Barabási & Albert (1999) have shown that the degree distribution approaches \(P(k) \propto k^{-\gamma}\) with the parameter dependent constant \(\gamma\) for large numbers of nodes.
- It has been shown that the degree distribution generated by this model fits well to empirical data on real-world social networks (Barabási & Albert 1999; Albert & Barabási 2002).
- There exist useful generalizations of this model that make it possible to adapt the growth algorithm to different applications with special requirements (Albert & Barabási 2002).
As the decision rule under social influence, the preferential attachment procedure lends itself to various behavioural interpretations. One can just understand it instrumentally, but one of its advantages is the possibility to provide multiple potentially realistic interpretations. The two most relevant interpretations for our purposes are a more rationalistic and a psychological version. From the perspective of rational choice, it can be an efficient heuristic to acquire information to only inquire the choices of the most well-connected members of the network. The assumption is that (a) creating links is costly and (b) well-connected nodes are statistically more representative with respect to the relevant information. These assumptions are not explicitly implemented in the overall model, but they justify preferential attachment as a potentially efficient heuristic.
An alternative interpretation assumes that human agents tend to prefer to connect to well-connected individuals. Being central in one’s social network often represents high status, power or charisma, making it desirable to be socially connected to a highly connected individual. For modelling, it is sufficient to note that there are multiple plausible interpretations of the behavioural rules employed. Any real-world system following preferential attachment will likely include a mixture of social and psychological mechanisms implementing preferential attachment without challenging the validity of the model.
Simulation Algorithm
At this point, the parts of the model can be assembled: It is initialized with a set of agents which decide on their behaviour purely on the basis of their preferences. They become connected afterward to make the probabilities for the preferential attachment algorithm well-defined. Note again that the agents are not heterogeneous with respect to their decision functions, only using different information. After the initialization step, agents are successively attached to the network and make their decisions according to the information they acquire from their initial neighbourhood. The following pseudocode specifies the model’s behaviour:
- Initialize \(m_0\) agents’ preferences by drawing them uniformly at random out of \([1, \dots, 11]\), and choose the preference as the observable behaviour.
- Connect the initial set of agents.
- Repeat:
- Create a new node \(i\), with its preference \(p_i\) chosen uniformly at random.
- Add i to the graph by preferential attachment.
- Compute the median behaviour choice in \(i\)’s neighbourhood to determine \(c_i\).
A few remarks on the simulation model are appropriate before the analysis turns to its surprisingly complex behaviour. The variables that a simulation experiment can directly intervene on are \(m_0\) and \(m\), thereby to some extent controlling the quality and amount of information available to the agents that enter the model later on. However, the stochastic elements of the model are influential, requiring the simulator to run large numbers of simulations to identify interesting cases; to a significant extent, the explanatory factors are to be found in the early dynamics of a model run rather than the model parameters. Therefore, the analysis will start out with a number of single-run analyses to exhibit the relevant qualitative behaviour, followed by a thorough exploration of the parameter space and the crucial factors leading to an unpopular norm.
The results in the following section are based on a Python implementation of the algorithm above which can be accessed at https://www.openabm.org/model/5289/version/1/view.
Results and Discussion
The Emergence of an Unpopular Norm
The first question to be answered is if an unpopular norm can actually emerge in the model. The pattern that serves as a qualitative benchmark for an unpopular norm due to pluralistic ignorance is provided by the alcohol consumption norms reported by Prentice & Miller (1993): There should emerge an approximately normal distribution of behavioural expectations and therefore choices from an approximately uniform distribution of preferences, but with different means.
Figure 1 depicts the result of a simulation run exhibiting the described pattern. The parameter configuration is \(m_0 = 40\), \(m = 10\) and the simulation is run for about \(10^3\) timesteps, thereby increasing the population size to 1000 agents. The distribution of behavioural choices has the form of a steep binomial distribution that has the normal distribution as its limit. The steepness signifies a high degree of coordination and the difference of 0.73 between the means of the two distributions represents the unpopularity of the norm the population coordinated on.
More theoretically, let us reconsider the concept of a social norm that has been assumed and match the components of the definition with the results. The possible choices should be understood as various options for actions, available to become rules for behaviour. The conditional preference for norm-following is assumed in the agents decision rule: They try to estimate a rule which factually has the most followers, and by their decision rule they assume others to expect to follow and are therefore motivated to follow the rule themselves, as expressed by their particular choice. The final piece, and what is really the result of the simulation, is to show that there is a sufficiently large part of the population sharing the same beliefs on actual followership and expectation. The distribution of choices in Figure 1 exhibits this high degree of shared belief, and thus clearly is not one of the potentially problematic borderline cases for the assumed definition of a social norm. If, in addition, the norm is allowed to be slightly fuzzy, the agents only one by one in the spectrum of potential options can even be counted in the subpopulation of norm-followers, making the case even more clear-cut.
The results of a single run are useful to showcase the model’s behaviour, but a more detailed exploration of the distribution of outcomes will be provided in the sensitivity analysis. But before the discussion turns to a more detailed analysis for the dynamics of unpopular norms and the overall behaviour of the model, a few remarks on the interpretation of this first result are in place.
First, it is now easy to see how a binary choice imposed on the simulated population could generate absolute pluralistic ignorance. Imagine that the scale of choices and preferences represents a simple political spectrum from left to right. However, whereas political preference is diverse, the political system supports only two parties. Now assume that everyone votes for the party that is closest not to his or her preference, but to their behavioural expectation for the population, for example because the election is not secret. Furthermore, assume that party \(A\)’s program exactly mirrors the mean of the preference distribution (6), but party \(B\) is more radical and positions itself at 7.4. In this scenario, the majority of agents would vote for \(B\), therefore creating a choice that exhibits absolute pluralistic ignorance. A more extreme case of pluralistic ignorance could lead to an even more extreme situation, and is exacerbated by the assumption that everyone votes for the closest party – an assumption that is not obviously true, but quite standard in voting models (Black 1948).
Second, the level of coordination achieved in the simulation is quite surprising. There is no belief revision involved, and there is a steady inflow of conflicting private preferences. The communication structure of the model seems to enable such a high degree of coordination with very small effort. This relationship between network structure and outcome will be explored more closely below, but it is crucial to understand how a social process can become established that can produce highly suboptimal results: It does not deterministically produce inefficient outcomes, but allows for sometimes successful coordination using a minimum of both cognitive and communicative resources.
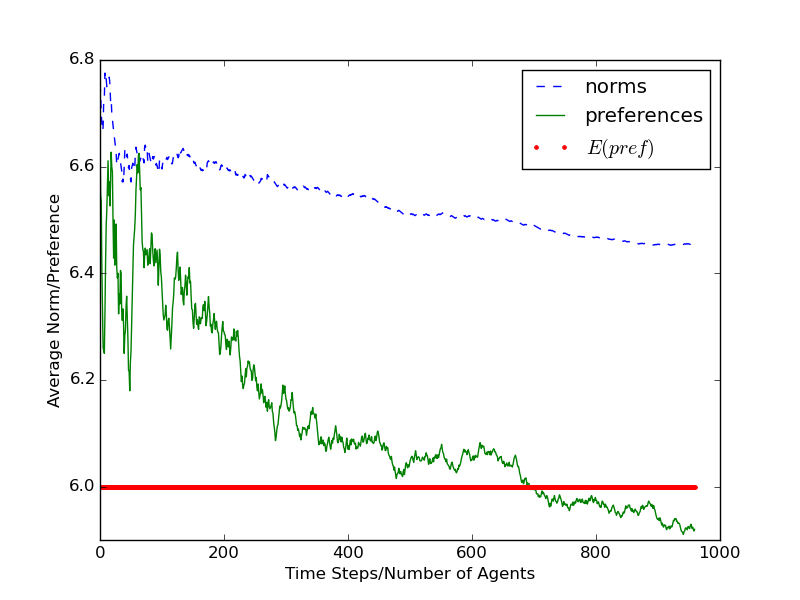
The Dynamics of Pluralistic Ignorance
One of the advantages of a simulation model that successively emulates a process is the access to the dynamic features of the process. These features can be compared to known empirical properties of the process to increase the model’s credibility. The empirical finding referred to is the lag of change in norm perception and adaption behind changes of the distribution of preferences. The model has no mechanism for change in attitudes or preferences, but the actual distribution of preferences approaches the distribution defined by the underlying generating process over time, due to the growth of the network. Likewise, the distribution of norm expectations evolves dynamically with the addition of new agents. If pluralistic ignorance occurs in the model and potentially leads to an unpopular norm, a lag as described by Shamir & Shamir (1997) and Gërxhani & Bruggeman (2015) should be observable. This is indeed the case, as Figure 2 shows.
The evolution shows the characteristic lag of the perceived norm behind the preferences. The mean of preferences over time approaches the mean of the underlying uniform distribution[10]. The perceived norm, however lags behind this development and is stuck on a suboptimal option. After a highly volatile initial phase, the lag becomes visible: The mean of the actual preferences starts to drop (modulo random variation) towards the underlying distribution’s mean. At this point, the average of choices also starts to drop, but way slower, remaining at a significantly higher value for the rest of the observed time interval, while steadily decreasing in the direction of the preference distribution’s mean. This fits very well the observations reported by the longitudinal empirical research. In the example of the political climate in Israel, private preferences evolved towards what became the actual election results and came close, while the perceived political climate did not change significantly until the election results became public. Since such an external event does not occur in the model system, it reproduces the process until the election, where the preference distribution changes without an appropriate change in the perception of the situation.
The source of this time lag in the model is the combination of conformism with a lack of belief revision. As Gërxhani & Bruggeman (2015) argue, belief revision in the real world is delayed by uncertainty. This is very much in line with both the observation by Shamir & Shamir and the simulation outcomes, and gives an overall justification of the assumption that there is no belief revision on the simulation’s time scale. The process starts out with a non-representative initial agent population with respect to their preferences. These form a norm that fits their distribution of preferences. The agents that enter the group later on adapt to their norm due to social influence. Then, over time, the preference distribution approaches the underlying uniform distribution. Notably, the distribution of norm expectation evolves in the general direction of the preference distribution but is inhibited by the implicit conservatism of the underlying mechanism.
The model is also able to reproduce other scenarios than the emergence of unpopular norms. Two instances of convergence of the norm to the underlying preference distribution are depicted in Figure 3. It is interesting to note the differences between these scenarios. In the run depicted in the upper graph, an initial misrepresentation of the population-wide preference distribution creates a deviation of the behavioural expectation, and therefore a suboptimal norm. However, the mean of the instantiated preferences then drops sharply, and pulls the norm towards the mean of the underlying preference distribution. Thus, in this case, the shift from one misrepresentation to the other allows the mean of normative expectations to converge comparatively quickly to the population-wide mean of preferences. It is important to note, however, that the deviation started out relatively small, making the initial situation of ignorance easier to overcome. More surprising is the case depicted in the lower graph of Figure 3, where the convergence of the mean of preferences to that of the underlying distribution lags behind the norm as the mean of norm expectations is approaching the underlying preference mean. This can be understood as inverse pluralistic ignorance, since the initial nodes of the network are more representative of the underlying distribution of preferences than the subpopulation that exists after a few dozen time steps. This suggests the intermediate normative result that the social process described by the model is not in and for itself collectively irrational as it might appear. For some configurations, the results are maybe even surprisingly close to the stipulated optimum, in particular given the uncertainty. The discussion will return to this normative aspect.
The capability of the model to generate a variety of phenomena surrounding social norms very different from unpopular norms raises of course the question under which conditions the process runs into a problematic, since unpopular, norm and when it generates a reasonable or even favourable outcome given the assumed preference distribution. To address this issue, it has to be investigated which variables within the simulation model can explain the pluralistic ignorance and thereby the evolution of an unpopular norm. This task will be undertaken in the next section, but the candidate hypothesis that will be tested there could be stated the following way: Given an initial population not representative of the underlying preference distribution and a network evolution that facilitates the influence of these initial nodes providing misleading cues, an unpopular norm emerges[11]. Besides testing this hypothesis, the sensitivity analysis has to explore the impact of variation in the parameters \(m\) and \(m_0\), which control the network growth algorithm.
A side remark on the stochasticity of the model and potential correlations in the target system: Random elements of the model are not necessarily related to actual random, unknown or unpredictable factors. For example, there might be patterns relating political radicalism and influence, positive or negative. The model’s randomness is meant to allow a variety of different social settings, generating quite different patterns and exploring their consequences.
Sensitivity Analysis
The Importance of Network Structure
A first important question is how relevant the network structure is for the model’s behaviour. The preferential attachment mechanism creates networks with a relatively small number of highly connected nodes, so-called hubs, while most agents have a degree close to the minimum set by \(m\). While the model configuration only provides an approximation of the limit degree distribution for relatively small \(k\), it already assures the general pattern of a small number of hubs and a large number of peripheral nodes. Up to this point, it has been tacitly assumed that the informational constraints posed by this network structure are vital for the model’s behaviour. The assumption can be operationalized for testing by the following hypothesis:
[\(H_{Network}\)] The perceived norm in a given network is positively correlated with the preferences of a small number of hub nodes.The rationale behind this hypothesis is that most agents make their judgment on the basis of perceiving hub nodes. They do not only perceive hub nodes – at least they perceive themselves – but hub nodes’ preferences are overrepresented. Figure 4 depicts the relationship between the average population norm and the preferences of the top 5% of agents with regard to their degree in the network.
A simple linear regression for the influence of the hubs’ preferences gives us approximately a slope 0.8 with an r-squared of 0.52 and a standard error statistics of 0.05, with \(p \approx 5.5e^{-33}\). This makes the average preference of the network’s hubs a good predictor of the resulting population-level norm. To better frame this result, a second regression relating the average population preference to the average norm has been fitted. The plot exhibits a large amount of variance, with the following regression statistics: The slope of the regression line is 2.1, with a value of 0.08 for r-squared and a standard error of 0.52. Comparing these two statistical models confirms the assumed importance of central nodes for the resulting norm in a given simulation run. The actual preference distribution’s influence is strongly perturbed, making it effectively useless to explain the variation.
To summarize, well-positioned nodes are crucial to the evolution of norms in a world of restricted information and the need to adapt behaviour to perceived norm expectations. With respect to real-world phenomena, network hubs can be interpreted differently to account for different application domains. For example, in the analysis of the political climate in Israel, Shamir & Shamir point to the important role of the incumbent government with its superior ability to communicate a certain opinion as the norm. Switching contexts, while Prentice & Miller (1993) do not give this explanation explicitly, they mention the influence of fraternities on the perception of students. Again, an established institution allows its leading members to overrepresent a certain behavioural norm to the overall population. In the case of fraternities on a campus, they might also have additional influence on the perception of students in the general public, creating an additional indirect effect on prospective students’ expectation and perception of campus norms. The discussion will return to this issue when exploring the effect of explicitly modelled central influence.
However, even without the assumption of institutionalized influence, the example of a college campus can be framed in the model’s terms to understand the application of preferential attachment in a practical example: When a new batch of freshmen enter the school, they have approximately no social ties. When entering the social network at the campus, it makes sense for them to orient towards the most visible senior students, since those already know the social rules in place, which the freshmen have an interest in learning. While in practice, many links are probably formed simultaneously, and the probability to connect to fellow freshmen is higher than assumed in the preferential attachment model, a process of continuous addition of nodes to the social network with the probability of new links skewed towards the incumbent population is a reasonable model of the stylized description.
Parameter Space Exploration
What remains to be analysed is the model’s behaviour across a fine-grained variation of the parameters. Especially the potential interplay of \(m\) and \(m_0\) has to be taken into account. These parameters control network growth, and therefore the available information. Larger \(m\) improves the access of new agents to the existing network, while \(m_0\) controls the likelihood that the initial population is representative: The larger the initial sample, the more likely it is representative of the generating distribution. Figure 5 depicts an exploration of a larger part of the parameter space. The parameters \(m\) and \(m_0\) are varied between 1 and 2 respectively to 50. The upper graphic shows the maximum difference between the mean norm and the mean preference over 5 runs, the lower one depicts the average of that difference. This difference provides a rough measure for the failure to identify the optimal norm in a given simulation run.
Descriptively, there are two main features shown in the graphics: The lowest values of pluralistic ignorance, signified by the lighter blue, are concentrated in the domain of low \(m\) and large \(m_0\). There is a strong increase (signified by orange and red) towards smaller \(m_0\), and a smaller, but clear increase when both \(m\) and \(m_0\) grow larger. It is also relevant to observe that cases of stronger pluralistic ignorance are scattered within areas of weaker ignorance, pointing to the volatility of the underlying process.
To interpret this, one should start by noting that pluralistic ignorance occurs consistently over a wide variety of parameter configurations. While being mostly a negative result, it is important to show the robustness of the process to justify the use of single run analysis. However, the extent of ignorance and its variability still differ across the parameter space. Configurations with very small \(m\) are less prone to pluralistic ignorance, but the most extreme scenarios seem to appear more often for relatively small values of both parameters more. It appears prima facie counterintuitive that smaller \(m\) improve the performance when \(m_0\) is large, since larger values of \(m\) seem to represent more information for the agent. However, in the case of a small initial population that is misrepresentative of the actual preference distribution, it just reduces the weight of the preferences of new agents compared to the incumbent population, thereby cementing the unrepresentative distribution of the first \(m_0\) agents. The logic behind that is built into the decision rule, since larger \(m\) automatically reduce the weight agents assign to themselves and increase the relative importance of the current agent population. There are three important takeaways from the sensitivity analysis for the general validity of the model:
- Pluralistic ignorance occurs under a variety of different structural conditions imposed on the network. This justifies the generalization from the behaviour under a specific parameter configuration to the properties of the simulation model more generally.
- It shows that the skewed degree distribution maintains pluralistic ignorance when connectivity is increased. This complements the above experiment on the relevance of hubs: Since the number of hubs does not increase due to the scale-free character of the degree distribution, the influence of hubs is sustained even in scenarios with a larger total number of sources of information.
- The whole process is overall fairly volatile relative to the network structure. This also implies that to potentially evaluate the risk of the emergence of an unpopular norm, a researcher would have to know not only the network structure and the distribution of preferences, but also how the preferences map to the structurally important nodes in the network.
Central Information
The influence of centralized agencies like government media, or more general, visible government action, seems to have an important impact on the emergence and sustenance of a state of pluralistic ignorance and, in turn, unpopular norms. Other examples where a central agent or agency is able to communicate a behavioural norm to a whole population are centralized churches and media empires holding a quasi-monopoly on a certain widely consumed medium. In the basic model, this kind of influence does not exist. It is decentralized, and the initial nodes, which are most likely to become important hubs in the network, are independent in their preferences. This is once again similar to many models of opinion formation (as the basic version of the Hegselmann-Krause-model, see Hegselmann & Krause 2002), but in either case an unrealistic assumption. In particular, there exist extensions to the Hegselmann-Krause-model of opinion formation to include various central sources of information (Hegselmann et al. 2006; Hegselmann & Krause 2015).
Therefore it is an important step to amend the model to represent central influences, which can be achieved by adding a single node to the network which is visible to everyone from the beginning on. Figure 6 shows the outcomes of simulation runs, again with \(m=10\) and \(m_0=40\), one with a central influence \(I=6.0\), which is identical to the mean of the distribution from which agents’ preferences are drawn, and a second one with \(I = 8.0\), representing a perturbing central influence.
These outcomes can also be compared to the case without any central influence shown in Figure 4. The outcomes are significantly more clustered around the mean of the preference distribution in the case of representative central information, as one would expect. In the case of a misleading central agent, again unsurprisingly, the perceived norm shifts towards this agent’s behaviour. In addition, choices are clustered around integer values due to the agents’ adherence to the median perceived norm that is necessarily discrete on an integer scale. It is interesting to note that these effects are pronounced despite the fact that the central information is only 1 in 11 neighbours in the given configuration. This explains the variation in the outcomes, but it also confirms the immense influence of a central agent in a scale-free network.
An interesting feature of this model of centralized sources of information is that it also generates an indirect effect. When a new agent is added to the network and gathers information about the current norm, it is directly influenced by the central media node. However, there is also an indirect effect, since all the neighbours it retrieves information from have been influenced by the central agency before. Therefore, the cumulative effect of such an agency goes beyond giving information, it also creates a certain informational background across the agent population it influences. In this manner, centralized agencies can be highly influential in creating a certain political or cultural climate, without even being perceived as a more important source of information than normal agents close in the social network.
A Normative Perspective
Up to this point, normative judgment is only implicit in the analysis. However, evaluative statements are clearly pervasive in the analysis of unpopular norms. By definition they are norms that are not in the interest of the majority of their subjects – a point that is also mostly implicitly present in the empirical literature. The selection of examples includes norms of corruption, alcohol abuse and racial segregation, which are often not only detrimental to the subjects but also considered social problems externally. On the other hand, in some cases, they might even be considered ethical problems. Since the model does not endogenize a standard provided by the society external to the modelled agents or ethical theory, the analysis will focus on criteria that can be evaluated within the model population.
First of all, it is necessary to clarify what the agents actual underlying preferences are, and what is part of the strategy. The agents prefer to behave according to their built-in ordering of options: For example, an agent who favours option 5 on the model’s scale would prefer 5 to everything, 4 and 6 to anything but 5 and be indifferent between 4 and 6, and so on. However, there is also a social component, since it pays off to coordinate on a norm in many cases. For example, a member of an emerging political movement might have a very specific preference on the subject matter, but he or she also has an interest to be part of a democratic majority to realize something as close as possible to his or her interest. As mentioned before, Equation 2 can actually be reinterpreted as a definition of an agent’s actual payoff, since it takes into account the degree of coordination as well as individual preference. The weights can express the different importance of coordination with different people – for example, coordinating with your co-workers or family seems very valuable, while being aligned with all the distant members of your political community seems less important. For the current discussion, the assumption is \(\forall i \forall j w_i(j) = w_j(i)\).
Given that, what goes wrong in the case of an unpopular norm is that the overall sum of payoffs could be increased by shifting the norm. Of course, this definition comes at the cost of accepting interpersonal comparability, at least with respect to one issue. But this is hard to avoid if one wants to characterize unpopular norms as collectively irrational or a problem for social welfare, since such norms are not necessarily Pareto-inefficient. Imagine the following example: Assume there are exactly two options the agent can choose from, and there are n = 3 agents, one of which prefers option 1, the other two option 2. Perfect coordination on either option is Pareto-optimal, but coordination on 1 represents an unpopular norm. The implication would be that – whatever the exact normative concept applied – interpersonal judgments of welfare are involved, which is commonly avoided in rational choice analysis of social systems.
There are three options to handle this complication:
- Revise the judgment on unpopular norms.
- Explicitly consider unpopular norms a moral problem, rather than a failure of rationality.
- Provide an understanding of collective irrationality that includes unpopular norms.
The first two options are trivial, and they are also compatible with each other. The third alternative is more difficult, but it is able to capture the idea that if only the group understood the process well enough, they would have a reason to behave differently without reference to ethical judgment at all. While the example of unpopular norms and a particular model are not sufficient to determine how such a concept of collective irrationality should be defined, there is an important insight for a social theory of rationality: The social processes involved are of crucial importance.
The question of rationality on a social level cannot solely rely on judging the actual outcomes by standards like Pareto-efficiency. An argument on the rationality of a certain behavioural pattern has to take into account how well the process generating the pattern fares statistically in the environment it is applied to. Some decision environments are very difficult to adapt to because of fundamental uncertainty, while others lend themselves to be solved optimally. Moreover, the resources a certain process requires are an important feature that looking at results does not reflect. Finally, it is important to compare the process to its actual alternatives. For example, as voting theorists already knew at least since Condorcet (cf. Pacuit 2012, Sec. 3.1), majority vote can create apparently irrational results. But, if it actually should be considered collectively irrational for a certain group depends on the decision environment, the available resources and the alternatives – and the same is true for the process of decision under social influence modelled in this paper. Therefore, the fact that the model reproduces the undesirable outcome of an unpopular norm in a significant number of scenarios does not in itself constitute an argument for the irrationality of the modelled process.
Finally, a remark on the ontology of groups: The discussion of collective (ir)rationality does not necessarily require ontological assumptions about the existence – or non-existence – of collective entities. It can be understood as a property of the distribution of beliefs and preferences of a group. This does not imply that group rationality can be reduced to individual rationality. In fact, results like Arrow’s Theorem (Arrow 1950) can be understood as implying the impossibility of such a reduction. Rather, individual rationality cannot be defined without reference to the social processes it is embedded in.
Limitations
While the model meets all the benchmarks set in the introductory section and thereby provides a valid theoretical model of the evolution of unpopular norms under pluralistic ignorance, there are some important limitations that ought to be mentioned. They fall in three broad classes:
- Idealizations that lay bare the dynamics of a single social process, isolated from the various influences interfering with it in the real world
- Simplifying assumptions that enable the computational analysis of the model
- Domain restrictions.
Important examples of the first kind of idealizations are the lack of belief revision, the exclusion of external events, and the backward-looking, i.e., non-strategic behaviour of the agents. It is possible to relax all these assumptions – in fact, it would be highly interesting – but adding these factors would create a new model of multiply intertwined processes. Such additions are easily fit into the model, as the exploration of central sources of information has shown, but they go beyond what the current investigation set out to achieve. In particular, if one wants to extend the model to arbitrary time scales, it becomes crucial to include factors like belief revision and external events. Insofar, these idealizations can also be understood as domain restrictions.
Besides explaining the phenomenon by the most simple fundamental process sufficient, there are additional reasons to adopt these constraints: The effects of belief revision, for example, seem to depend heavily on the particulars of the sequencing. How often and when belief revision would take place would be crucial to its effects on the process. Note that this kind of idealization is also responsible for the impossibility of the quantitative fitting of data. To account for real-world data, it is usually necessary to include not only a multiplicity of processes, but also to include a number of theoretically meaningless parameters for calibration. The model is not supposed to be used in such a research agenda; its main purpose is to provide a formalization of a social theory to allow for the exploration of its implications.
The second kind of idealizations, on the other hand, could be relaxed not by fundamentally changing the model, but by gradually varying certain factors. In the decision procedure, the main assumptions concern the choice set and the weighing of other agents. With respect to the choice set, it has been assumed that it does not make a relevant difference to have an 11-point scale to having a 7-point scale. While this seems plausible, it has not yet been explicitly shown. Varying the weights of various agents is a more intricate problem, since there are infinitely many options to model differential weighing. Agents could weigh themselves disproportionately, they could emphasize the importance of well-connected nodes in their neighbourhood, or intentionally de-emphasize them. For the network structure, the very basic version of preferential attachment has been chosen. However, there are many alternatives to growing an artificial social network. As the analysis of hub influence has shown, the model behaviour depends on the stratification of the network into hubs and peripheral nodes, but there are alternative algorithms to generate such networks that have not yet been tested.
The results of the analysis of preferential attachment allow speculations that can be tested by future research. The most common alternatives would be random graphs and small-world networks, as mentioned above. The prediction for a random graph (Erdös & Rényi 1960) is straightforward: The binomial degree distribution implies that hubs of the same degree are less likely than in the scale-free preferential attachment network. Therefore, pluralistic ignorance becomes less likely. The case of a small-world network is more complicated. Informally, if the network evolution starts from what will become the bridging agents, these agents would be more influential than a random agent from within a cluster. The effect should be smaller than in the case of preferential attachment, since most of the additional influence of the bridging agents is indirect, and therefore weak under the assumption of equal weights. Given no such assumption, pluralistic ignorance seems once again improbable. The degree distribution falls between that of a ring lattice and the aforementioned random graph depending on the parametrization, and both assign low probabilities to large degrees – as compared to the scale-free distribution generated by preferential attachment. A more interesting perspective on a small-world based model might be to compare the within-cluster expectation distributions of behavioural expectation with the global preference distribution.
Finally, the restricted domain is not so much a limitation, but rather a necessity of scientific modelling. The model imposes two major constraints on the class of potential target systems: First, the model has to behave approximately as described by preferential attachment. To even judge this, it has to be assumed that the network grows sufficiently large: Small kinship groups, for example, are certainly out of scope. The empirical literature on preferential attachment has found fitting power law distributions in scientific collaboration and citation networks as well as the collaboration network of movie actors (Jeong et al. 2003). Furthermore, the World Wide Web (Donato et al. 2004) and the link structure of Twitter (Java et al. 2007) have been found to follow power laws in their degree distribution. There is some selective bias in the tested examples, since these are all networks on which data is comparatively easy to obtain. Keeping this in mind as a caveat, one can tentatively generalize: When agents are able to choose links without strict spatial or formal limitations and linking oneself to well-connected nodes appears in some sense advantageous, the network structure arising from preferential attachment seems to be an empirically adequate model. Note, that a network evolution following the rules of a formal hierarchy often will generate a highly skewed degree distribution, too, if it is assumed that links represent the perception of behaviour and are usually not bidirectional. Thus, there might be a broader class of applications, but for the discussion in this paper, the applicability of preferential attachment limits the model domain.
Second, as mentioned before, the target system’s time scale should not make it absurd to exclude external events or belief revision. For example, if there is a major election, a media campaign, or intense public discourse, the model may only be applied to the process before that event. In general, the model fits best when a relatively decentralized process of opinion formation about behavioural expectations takes place over time, with more and more agents entering the system. Norm formation within some growing grass-roots political movements or the workplace population in an expanding company can provide examples for processes operating in accordance with these constraints. Note that application is meant in a qualitative sense, not a quantitative analysis or prediction.
Conclusions
Summary
It has been shown that the evolution of an unpopular norm via pluralistic ignorance can be modelled by the combination of agents deciding under social influence given the informational restrictions of a social network. Relative pluralistic ignorance, denoting a deviation of the overall perceived distribution of norm expectations from the population’s actual preferences, emerges in the model under various parameter configurations. Furthermore, the model turns out to be more general, as it also allows for the emergence of efficient norms in a population of conformist, boundedly rational agents. The driving force is the influence of hubs in the network, which can be either misrepresenting the actual distribution, represent it correctly for the subpopulation at a given point in time, or even be representative of the underlying distribution of preferences for the whole population when the current subpopulation is still way off the unfolding preference distribution. These phenomena lend themselves to interpretations as hubs being a reactionary or avantgardist elite – with elite defined in purely relational terms. The statistical analysis of the relation between the hub preferences and the resulting population-wide norm supports this interpretation.
As further exploration has shown, pluralistic ignorance can arise in networks of varying structural parameters. It occurs most pronounced in configurations where information is particularly sparse and non-representative. More representative information, however, can be provided by central agencies in a minimal intrusive way. At the same time, such a powerful central influence can also perturb the evolution of a norm in a detrimental way, according to the standard provided by the population-wide preference distribution. This analysis connects the decentralized model to those case studies that feature central influence at crucial points. Especially in political contexts, central agencies are pervasive. But, also for small, naturally decentralized groups, establishing a central information instance can be utilized as an intervention.
Future Directions
There are some natural extensions to the model that are to be explored in future research. The most pressing questions are posed by the limitations of the current model. Mechanisms of belief revision, the inclusion of external events or a more extensive study of the robustness of the results under varying network topologies are all valuable options to increase our theoretical understanding of unpopular norms and social influence mechanisms more generally. Another interesting route to consider is a hybrid approach that combines the mechanism of social influence with a more strategic behavioural model. This kind of hybridization is also one of the current problems for formal models of opinion formation (Mäs 2015), which are closely related to the dynamics of norm expectation and behaviour. In particular, this is just an instance of the more general problem to combine strategic, forward-looking behaviour with backward-looking adaptive behaviour in agent-based modelling.
Besides improving the descriptive accuracy and the explanatory power of the model, future research should take a closer look at the application of the model in complex normative arguments about various social phenomena. There is a strong tension between the abstract theoretical character of the model with its many idealizations and the complex world of interventions to quantitatively influence important variables of social welfare. However, in that respect, it is very similar to arguments based on informal theoretical accounts. In their discussion of availability cascades, a related phenomenon of apparent collective irrationality, Kuran & Sunstein (1999) make no actual quantitative claim. Based on an empirically supported theory of individual behaviour, they develop a theoretical account and suggest concrete interventions – without providing any quantification of the effects. It is not yet clear how – if at all – either kind of argument is valid. However, formal models provide a more transparent way to express theory and draw conclusions. As long as we are interested in counterfactual scenarios to solve social problems as they are represented by unpopular norms, agent-based computer simulations seem to provide an important tool not only for explanation and description, but also to develop interventions and make normative arguments.
Notes
- There is also an extended discussion about the question if norms can always be identified with game theoretic equilibria of some kind. It will be argued in more detail in Section 2 if that is actually a reasonable assumption.
- It is actually quite difficult to ascertain for a specific case that unilateral deviation would be advantageous, since there might be subtle sanctions that were not registered by any particular study. However, the cases of alcohol and sex above seem to be genuine cases of advantageous unilateral deviation, since the actual expectations differed so much from the perceived ones, removing the major motive for sanction. Of course, there is also the phenomenon of false enforcement (Centola et al. 2005), but the burden of proof rests with the party offering the less plausible prediction, which appears to be sanction in these cases.
- There are, by the way, a number of motives to accord with other people’s expectations: It can be necessary to acquire social status (or not to loose it), caused by fear of sanction or a basic preference for conformity. In fact, the different subjects of a social norm, unpopular or not, can be actually motivated to accord with expectations due to quite different factors.
- The case of political climate evolving under pluralistic ignorance does, by the way, not necessarily constitute a social norm, unpopular or not. While it seems plausible to assume a correlation between the existence of certain social norms and a particular political climate, the study cited does not claim something about norms, but rather offers longitudinal data on pluralistic ignorance.
- Note that Elster himself concedes that some rational reconstruction will always be possible. But in some cases, this reconstruction will not be explanatory valuable.
- In this paper, I will actually follow the suggestions towards the end of Elster (1989) and rely on the motivation by conformism; however, this is probably not universal, and can also be dissected more closely into conformity due to factors like fear of sanction, striving for status or simply habituation.
- It is also not apparent how role-differentiated norms in general are to be stated in this terminological framework, but I will assume for the sake of argument that it is in principle possible.
- Note that Axelrod’s definition of a social norm differs significantly, since it only refers to the frequency of norm-following and punishment. While punishment is not necessary according to the definition employed here, behavioural patterns are not sufficient.
- It might not be impossible to do so, but importantly, it would not be a faithful model of the type of situation.
- The mean of realized preferences actually drops below the theoretically underlying mean in this particular case, as a result of random variation in the underlying pseudo-random number generation process. Note, that the deviation is actually very mall (< 0.1), which is not an unlikely deviation from the actual mean for a medium-sized sample.
- Note that the model has a built-in self-correction mechanism, since for finite \(m\), new agents entering the system will always assign some weight to themselves and therefore new information on the true underlying distribution of preferences keeps entering the process, leading to a correction in the long run, notwithstanding the potential for a strongly mistaken norm at intermediate timesteps. The model itself is always evaluated with respect to short to medium term results, since those are realistically of more significance to actual human societies.
References
ALBERT, R. & Barabási, A.-L. (2002). Statistical mechanics of complex networks. Reviews of Modern Physics, 74(1), 47. [doi:10.1103/RevModPhys.74.47]
ARROW, K. J. (1950). A difficulty in the concept of social welfare. The Journal of Political Economy, 58(4), 328–346.
AXELROD, R. (1984). The Evolution of Cooperation. New York: Basic Books.
AXELROD, R. (1986). An evolutionary approach to norms. American Political Science Review, 80(04), 1095–1111.
BARABÁSI, A.-L. & Albert, R. (1999). Emergence of scaling in random networks. Science, 286(5439), 509–512 [doi:10.1126/science.286.5439.509]
BICCHIERI, C. (2005). The Grammar of Society: The Nature and Dynamics of Social Norms. Cambridge, MA: Cambridge University Press.
BICCHIERI, C. & Fukui, Y. (1999). The great illusion: Ignorance, informational cascades, and the persistence of unpopular norms. Business Ethics Quarterly, 9(01), 127–155. [doi:10.2307/3857639]
BLACK, D. (1948). On the rationale of group decision-making. The Journal of Political Economy, 56(1), 23–34.
CENTOLA, D., Willer, R. & Macy, M. (2005). The emperor’s dilemma: A computational model of self-enforcing norms. American Journal of Sociology, 110(4), 1009–1040. [doi:10.1086/427321]
DARLEY, J. M. & Latane, B. (1968). Bystander intervention in emergencies: Diffusion of responsibility. Journal of personality and social psychology, 8(4p1), 377.
DONATO, D., Laura, L., Leonardi, S. & Millozzi, S. (2004). Large scale properties of the webgraph. The European Physical Journal B-Condensed Matter and Complex Systems, 38(2), 239–243. [doi:10.1140/epjb/e2004-00056-6]
ELSTER, J. (1989). Social norms and economic theory. The Journal of Economic Perspectives, 3(4), 99-117.
ELSTER, J. (1990). Norms of revenge. Ethics, 100(4), 862–885, [doi:10.1086/293238]
EPSTEIN, J. M. (1999). Agent-based computational models and generative social science. Complexity, 4(5), 41–60.
ERDÖS, P. & Rényi, A. (1960). On the evolution of random graphs. Publ. Math. Inst. Hungar. Acad. Sci, 5, 17–61.
GËRXHANI, K. & Bruggeman, J. (2015). Time lag and communication in changing unpopular norms. PloS One, 10(4): e0124715.
GINTIS, H. (2009). The Bounds of Reason: Game Theory and the Unification of the Behavioral Sciences. Princeton, NJ: Princeton University Press.
HEGSELMANN, R. & Krause, U. (2002). Opinion dynamics and bounded confidence models, analysis, and simulation. Journal of Artificial Societies and Social Simulation, 5(3), 2: https://www.jasss.org/5/3/2.html.
HEGSELMANN, R. & Krause, U. (2015). Opinion dynamics under the influence of radical groups, charismatic leaders, and other constant signals: A simple unifying model. Networks and Heterogeneous Media, 10(3), 477–509. [doi:10.3934/nhm.2015.10.477]
HEGSELMANN, R., & Krause, U. (2006). Truth and cognitive division of labour: First steps towards a computer aided social epistemology. Journal of Artificial Societies and Social Simulation, 9(3), 10: https://www.jasss.org/9/3/10.html
JAVA, A., Song, X., Finin, T. & Tseng, B. (2007). Why we twitter: understanding microblogging usage and communities. In Proceedings of the 9th WebKDD and 1st SNA-KDD 2007 workshop on Web mining and social network analysis, (pp. 56–65). ACM. [doi:10.1145/1348549.1348556]
JEONG, H., Néda, Z. & Barabási, A.-L. (2003). Measuring preferential attachment in evolving networks. EPL (Europhysics Letters), 61(4), 567.
JOAS, H. & Knöbl, W. (2013). Sozialtheorie: Zwanzig einführende Vorlesungen. Suhrkamp Verlag.
KAUFFMAN, K. (1981). Prison officers’ attitudes and perceptions of attitudes a case of pluralistic ignorance. Journal of Research in Crime and Delinquency, 18(2), 272–294.
KRONEBERG, C. (2014). ‘Frames, scripts, and variable rationality: An integrative theory of action’. In Analytical Sociology, (ed G. Manzo), John Wiley & Sons, Ltd, Chichester, United Kingdom, pp. 95–123. [doi:10.1002/9781118762707.ch04]
KURAN, T. & Sunstein, C. R. (1999). Availability cascades and risk regulation. Stanford Law Review, 51, 683–768.
LAMBERT, T. A., Kahn, A. S. & Apple, K. J. (2003). Pluralistic ignorance and hooking up. Journal of sex research, 40(2), 129–133. [doi:10.1080/00224490309552174]
LEHRER, K. & Wagner, C. (2012). Rational Consensus in Science and Society: A Philosophical and Mathematical Study, vol. 24. Springer Science & Business Media.
MÄS, M. (2015). ‘Modelle sozialer Beeinflussung’. In N. Braun & N. Saam (Eds.), Handbuch Modellbildung und Simulation in den Sozialwissenschaen, Heidelberg, Berlin: Springer Verlag, pp. 971–997. [doi:10.1007/978-3-658-01164-2_34]
O’GORMAN, H. J. (1975). Pluralistic ignorance and white estimates of white support for racial segregation. Public Opinion Quarterly, 39(3), 313–330.
PACUIT, E. (2012). ‘Voting methods’. In E. N. Zalta (Ed.), The Stanford Encyclopedia of Philosophy. Winter 2012 edn.
PRENTICE, D. A. & Miller, D. T. (1993). Pluralistic ignorance and alcohol use on campus: Some consequences of misperceiving the social norm. Journal of personality and social psychology, 64(2), 243.
RAUB, W., Buskens, V. & Corten, R. (2015). ‘Social dilemmas and cooperation’. In N. Braun & N. Saam (Eds.), Handbuch Modellbildung und Simulation in den Sozialwissenschaen, Heidelberg, Germany: Springer Verlag, 597–626. [doi:10.1007/978-3-658-01164-2_21]
ROTH, A. E., Prasnikar, V., Okuno-Fujiwara, M. & Zamir, S. (1991). Bargaining and market behavior in Jerusalem, Ljubljana, Pittsburgh, and Tokyo: An experimental study. The American Economic Review, 81(5), 1068–1095.
SHAMIR, J. & Shamir, M. (1997). Pluralistic ignorance across issues and over time: Information cues and biases. Public Opinion Quarterly, 61, 2, 227–260. [doi:10.1086/297794]
SKYRMS, B. (2004). The Stag Hunt and the Evolution of Social Structure. Cambridge, MA: Cambridge University Press.
SKYRMS, B. (2014). Evolution of the Social Contract. Cambridge, MA: Cambridge University Press. [doi:10.1017/CBO9781139924825]
WATTS, D. J. & Strogatz, S. H. (1998). Collective dynamics of small-world networks. Nature, 393(6684), 440–44.