Introduction
Computational models have been used to assist in developing, implementing and evaluating public policies for at least three decades, but their potential remains to be fully exploited (Johnson 2015; Anzola et al. 2017; Barbrook-Johnson et al. 2017). In this paper, using a selection of examples of computational models used in public policy processes, we (i) consider the roles of models in policy making, (ii) explore policy making as a type of experimentation in relation to model experiments, and (iii) suggest some key lessons for the effective use of models. We also highlight some of the challenges and opportunities facing such models and their use in the future. Our aim is to support the modelling community that reads this journal in its effort to build computational models of public policy that are valuable and useful.
We believe this effort is timely given that computational models, of the type this journal regularly reports on, are now increasingly used by government, business, and civil society as well as in academic communities (Hauke et al. 2017). There are many guides to computational modelling produced for different communities, for example in UK government the ‘Aqua Book’ (reviewed for JASSS in Edmonds 2016), but these are often aimed at practitioner and government audiences, can be highly procedural and technical, generally omit discussion of failure and rarely include deeper reflections on how best to model for public policy. Our aim here is to fill gaps left by these formal guides, to provide reflections aimed at modellers, to use a selection of examples to explore issues in an accessible way, and acknowledge failures and learning from them.
We focus only on computational models that aim to model, or include some modelling of, social processes. Although some of the discussion may apply, we are not directly considering computational models that are purely ecological or technical in their focus, or simpler models such as spreadsheets which may implicitly cover social processes but either do not represent them explicitly, or make extremely simple and strong assumptions. Although ‘computational models of public policy’ is the full and accurate term, and others often use ‘computational policy models’, for the sake of brevity, we will use the term ‘policy models’ throughout the rest of this paper.
Based on our experience, our main recommendations are that policy modelling needs to be conducted with a strong appreciation of the context in which models will be used, and with a concern for their fitness for the purposes for which they are designed and the conclusions drawn from them. Moreover, policy modelling is almost always likely to be of low or no value if done without strong and iterative engagement with the users of the model outputs, i.e. decision makers. Modellers must engage with users in a deep, meaningful, ethically informed and iterative way.
In the remainder of this paper, Section 2 introduces the role of policy models in policy making. Section 3 explores the idea of policy making as a type of experimentation in relation to policy model experiments. We then discuss some examples and experiences of policy modelling (Section 4) and draw out some key lessons to help make policy modelling more effective (Section 5). Finally, Section 6 concludes and discusses some key next steps and other opportunities for computational policy modellers.
The Role of Models in Policy Making
The standard, but now somewhat discredited view of policy making is that it occurs in cycles (for example, see the seminal arguments made in Lindblom 1959 and 1979; and more recently official recognition in HM Treasury 2013). A policy problem comes to light, perhaps through the occurrence of some crisis, a media campaign, or as a response to a political event. This is the agenda setting stage and is followed by policy formulation, gathering support for the policy, implementing the policy, monitoring and evaluating the success of the policy and finally policy maintenance or termination. The cycle then starts again, as new needs or circumstances generate demands for new policies. Although the idea of a policy cycle has the merit of being a clear and straightforward way of conceptualising the development of policy, it has been criticised as being unrealistic and oversimplifying what happens, which is typically highly complex and contingent on multiple sources of pressure and information (Cairney 2013; Moran 2015), and even self-organising (Byrne and Callaghan 2014; Teisman and Klijn 2008).
The idea of a cycle does, however, still help to identify the many components that make up the design and implementation of policy. There are at least two areas where models have a clear and important role to play: in policy design and appraisal, and policy evaluation. Policy appraisal (as defined in HM Treasury 2013), sometimes referred to as ex-ante evaluation, consists of assessing the relative merits of alternative policy prescriptions in meeting the policy objectives. Appraisal findings are a key input into policy design decisions. Policy evaluation either takes a summative approach, examining whether a policy has actually met its objectives (i.e. ex-post), or a more formative approach to see how a policy might be working, for whom and where (HM Treasury 2011). In the formative role, the key goal is learning to inform future iterations of the policy, and others with similar characteristics.
Modelling to support policy design and appraisal
When used ex-ante, a policy model may be used to explore a policy option, helping to identify and specify in detail a consistent policy design (HM Treasury 2013), for example by locating where best a policy might intervene, or by identifying possible synergies or conflicts between the mechanisms of multiple policies. Policy models can also be used to appraise alternative policies, to see which of several possibilities can be expected to yield the best or most robust outcome. In this mode, a policy model is in essence used to ‘experiment’ with alternative policy options and assumptions about the system in which it is intervening, by changing the parameters or the rules in the model and observing what the outcomes are. This is valuable because it saves the time and cost associated with having to run experiments or pilots in the actual policy domain. This concept of the model as an experimental space is discussed in more detail in Section 3 below.
The common assumption is that one builds computational models in order to make predictions. However, prediction, in the sense of predicting the future value of some measure, is in fact often impossible in policy domains. Social and economic phenomena are often complex (in the technical sense, see e.g. Sawyer 2005). This means that how some process evolves depends on random chance, its previous history (‘path dependence’) and the effect of positive and negative feedback loops. Just as with the weather, for which exact forecasting is impossible more than a few days ahead, the future course of many social processes may be literally unknowable in detail, no matter how detailed the model may be. Secondly, a model is necessarily an abstraction from reality, and since it is impossible to isolate sections of society, from outside influences, there may be unexpected exogenous factors that have not been modelled and that affect the outcome.
For these reasons, the ability to make ‘point predictions’, i.e. forecasts of specific values at a specific time in the future, is rarely possible. More possible is a prediction that some event will or will not take place, or qualitative statements about the type or direction of change of values. Understanding what sort of unexpected outcomes can emerge and something of the nature of how these arise also helps design policies that can be responsive to unexpected outcomes when they do arise. It can be particularly helpful in changing environments to use the model to explore what might happen under a range of possible, but different, potential futures - without any commitment about which of these may eventually transpire. Even more valuable is a finding that the model shows that certain outcomes could not be achieved given the assumptions of the model. An example of this is the use of a whole system energy model to develop scenarios that meet the decarbonisation goals set by the EU for 2050 (see, for example, RAEng 2015.)
Rather different from using models to make predictions or generate scenarios is the use of models to formalise and clarify understanding of the processes at work in some domain. If this is done carefully, the model may be valuable as a training or communication tool, demonstrating the mechanisms at work in a policy domain and how they interact.
Modelling to support policy evaluation
To evaluate a policy ex-post, one needs to compare what happened after the policy has been implemented against what would have happened in the absence of the policy (the ‘counterfactual’). To do this, one needs data about the real situation (with the policy evaluation) and data about the situation if the policy had not been implemented (the so-called ‘business as usual’ situation). To obtain the latter, one can use a randomised control trial (RCT) or quasi-experiment (HM Treasury 2011), but this is often difficult, expensive and sometimes impossible to carry out due to the nature of the intervention barring possibility of creating control groups (e.g. a scheme which is accessible to all, or a policy in which local implementation decisions are impossible to control and have a strong effect).
Policy models offer some alternatives. One is to develop a computational model and run simulations with and without implementation of a policy, and then compare formally the two model outcomes with each other and with reality (with the policy implemented), using quantitative analysis. This avoids the problems of having to establish a real-world counterfactual. Once again, the policy model is being used in place of an experiment.
Another alternative is to use more qualitative System Mapping type approaches (e.g. Fuzzy Cognitive Mapping; see Uprichard and Penn 2016), to build qualitative models with different structures and assumptions (to represent the situation with and without the intervention), and again interrogate the different outcomes of the model analyses.
Finally, another use in ex-post evaluation is to use models to refine and test the theory of how policies might have affected an outcome of interest, i.e. to support common theory-based approaches to evaluation such as Theory of Change (see Clark and Taplin 2012), and Logic Mapping (see Hills 2010).
Interrogation of models and model results can be done quantitatively (i.e. through multiple simulations, sensitivity analysis, and ‘what if’ tests), but may also be done in qualitative and participatory fashion with stakeholders, with stakeholders involved in the actual analysis (as opposed to just being shown the results). The choice should be driven by the purpose of the modelling process, and the needs of stakeholders. In both ex-ante and ex-post evaluation, policy models can be powerful tools to use as a route for engaging and informing stakeholders, including the public, about policies and their implications (Voinov and Bousquet 2010). This may be by including stakeholders in the process, decisions, and validation of model design; or it may be later in the process, in using the results of a model to open up discussions with stakeholders, and/or even using the model ‘live’ to explore connections between assumptions, scenarios, and outcomes (Johnson 2015b).
Difficulties in the use of modelling
While, in principle, policy models have all these roles and potential benefits, experience shows that it can be difficult to achieve them (see Taylor 2003; Kolkman et al. 2016, and Section 4 for some examples). The policy process has many characteristics that can make it difficult to incorporate modelling successfully, including[1]:
- The need for acceptability and transparency: policy makers may fall back on more traditional and more widely accepted forms of evidence, especially where the risks associated with the decision are high. Models may appear to act as black boxes that only experts can understand or use, with outputs highly reliant on assumptions that are difficult to validate. Analysts and researchers in government often have little autonomy, and although they may see the value of policy models, it can be difficult for them to communicate this to the decision-makers.
- Change and uncertainty: the environment in which the policy will be implemented, may be highly uncertain, this can undermine model development when beliefs or decisions shift as a result of the modelling process (although this is equally an important outcome and benefit of the modelling process), or other factors.
- Short timescales: the timescales associated with policy decision making are almost always relatively fast, and needs can be difficult to predict, meaning it can be difficult for computational modellers to provide timely support.
- Procurement processes: often departments lack the capability and sufficiently flexible processes to procure complex modelling.
- The political and pragmatic realities of decision making: individuals’ values and political values can hold huge sway, even in the face of empirical evidence (let alone modelling) that may contradict their view, or point towards policy which is politically impossible.
- Stakeholders: There will be many different stakeholders involved in developing, or affected by, policies. It will not be possible to engage all of these in the policy modelling process, and indeed policy makers may be wary of closely involving them in a participatory modelling process.
These characteristics may also apply more widely to evidence and other forms of research and analysis. It is not our suggestion that these characteristics are inherently negative; they may be important and reasonable parts of the policy making process. The important thing to remember, as a modeller, is that a model can only, and should only, provide more information to the process, not a final decision for the policy process to simply implement.
Policy Experiments and Policy Models
Although the roles and uses of policy models are relatively well-described and understood, our perception is that there are still many areas where more use could be made of modelling and that a lack of familiarity with, and confidence in, policy modelling, is restricting its use. Potential users may question whether policy modelling in their domain is sufficiently scientifically established and mature to be safely applied to guiding real-world policies. The difference between applying policies to the real world and making experimental interventions in a policy model might be too big to generate any learning from the latter to inform the former.
Policy pilots
One response is to argue that actual policy implementations are themselves experimental interventions and are therefore of the same character as interventions in a policy model. Boeschen, Gross and Krohn (2017) propose that we live in “experimental societies” and that implementing policies is nothing but conducting “real-world experiments”. Real-world experiments are “a more or less legitimate, methodically guided or carelessly adopted social practice to start something new” (Krohn 2007, 344; own translation). Their outcomes immediately display “success or failure of a design process” (ibid, 347).
A real-world experiment implements one solution for the policy design problem. It does not check for other possible solutions or alternative options, but at best monitors and responds to what is emerging in real time. Implementing policies as a real-world experiment is therefore far from ideal and far removed from the idea of reversibility in the laboratory. In laboratory experiments the experimental system is isolated from its environment in such a way that the effects of single parameters can be observed.
One approach that tries to bridge the gap between the real-world and laboratory experiments is to conduct policy pilots. The use of policy pilots (Greenberg and Shroder 1997; Cabinet Office 2003; Martin and Sanderson 1999) as social experiments is fairly widespread. In a policy pilot, a policy change can be assessed against a counterfactual in a limited context before rolling it out for general implementation. In this way (a small number of) different solutions can be tried out and evaluated, and learning fed back into policy design.
A dominant method for policy pilots is the Randomised Control Trial (RCT) (Greenberg and Shroder 1997; Boruch 1997), well-known from medical research, where a carefully selected treatment group is compared with a control group that is not administered the treatment under scrutiny. RCTs can thus present a halfway house between an idealised laboratory experiment and a real-world experiment. However, the claim that an RCT is capable of reproducing a laboratory situation where rigorous testing against a counterfactual is possible has also been contested (Cabinet Office 2003, 19). It is argued that in principle there is no possibility of social experiments due to the requirement of ceteris paribus (i.e. in the social world, it is impossible to have two experiments with everything equal but the one parameter under scrutiny); that the complex system-environment interactions that are necessary to adequately understand social systems cannot be reproduced in an RCT; and that random allocation is impossible in many domains, so that a ‘neutral’ counterfactual cannot be established. Moreover, it may be a risky political strategy or even unethical to administer a certain benefit in some pilot context but not to the corresponding control group. This is even more the case if the policy would put the selected recipients at a disadvantage (Cabinet Office 2003, 17).
While a pilot can be good for gathering evidence about a single case, it might not serve as a good ‘one-size-fits-all’ role model for other cases in other contexts. Furthermore, it cannot say much about why or how the policy worked or did not work, or decompose the ‘what works’ questions into, ‘what works, where, for whom, at what costs, and under what conditions’? There are also more practical problems to consider, among them time, staff resources and budget. There is general agreement that a good pilot is costly, time-consuming, “administratively cumbersome” and in need of well-trained managing staff (Cabinet Office 2003, 5). There is “a sense of pessimism and disappointment with the way policy pilots and evaluations are currently used and were used in the past (...): poorly designed studies; weak methodologies; impatient political masters; time pressures and unrealistic deadlines” (Seminar on Policy Pilots and Evaluation 2013, 11).
Thus, policy pilots cannot meet the claim to be a happy medium between laboratory experiments, with their isolation strategies capable of parameter variation, and Krohn’s real-world experiments involving complex system-environment interactions in real time. This is where computational policy modelling comes in.
Policy models for policy experimentation
Unlike policy pilots, computational policy models are able to work with ceteris paribus rules, random control, and non-contaminated counterfactuals (see below). Using policy models, we can explore alternative solutions, simply by trying out parameter variations in the model, and experiment with context-specific models and with short, medium and long time horizons. Furthermore, policy models are ethically and politically neutral to build and run, though the use of their outcomes may not be.
Unlike real-world and policy pilots, policy models allow the user to investigate the future. Initially the modellers will seek to reproduce the database describing the initial state of a real-world experiment and then extrapolate simulated structures and dynamics into the future. At first a baseline scenario can be derived: what if there were no changes in the future? This is artificial and, for methodological reasons, boring: nothing much happens but incremental evolution, no event, no surprise, no intervention; changes can then be introduced.
As with real-world experiments, modelling experiments enable recursive learning by stakeholders. Stakeholders can achieve system competence and practical skills through interacting with the model to learn ‘by doing’ how to act in complex situations. With the model, it is not only possible to simulate the real-world experiment envisaged but also to test multiple scenarios for potential real-world experiments via extensive parameter variations. The whole solution space can be checked, where future states are not only accessible but tractable.
This does not imply that it is possible to obtain exact predictions for future states of complex social systems (see the discussion on prediction above). Deciding under uncertainty has to be informed differently:
"Experimenting under conditions of uncertainties of this kind, it appears, will be one of the most distinctive characteristics of decision-making in future societies […], they import and use methods of investigation and research. Among these are conceptual modelling of complex situations, computer simulation of possible futures, and – perhaps most promising – the turning of scenarios into ‘real-world experiments’" (Gross & Krohn 2005, 77).
Regarding the continuum between the extremes of giving no consideration (e.g. with laboratory experiments) and full consideration (e.g. real-world experiments) to complex system-environment interactions, policy modelling experiments indeed sit somewhere in the (happy) middle. We would argue that, where the costs or risks associated with a policy change are high, and the context is complex, it is not only common sense to carry out policy modelling, but it would be unethical not to.
Examples of Policy Models
We have discussed the role of policy models in abstract at some length. It is now important to illustrate the use of policy models using a number of examples of policy modelling drawn from our own experience. These have been selected to offer a wide range of types of model and contexts of application. In the spirit of recording failure as well as success, we mention not only the ultimate outcomes, but also some of the problems and challenges encountered along the way. In the next section, we shall draw out some general lessons from these examples.
Tell-Me
The European-funded TELL ME project focused on health communication associated with influenza epidemics. One output was a prototype agent-based model, intended to be used by health communicators to understand the potential effects of different communication plans under various influenza epidemic scenarios (Figure 1).
The basic structure of the model was determined by its purpose: to compare the potential effects of different communication plans on protective personal behaviour and hence on the spread of an influenza epidemic. This requires two linked models: a behaviour model that simulates the way in which people respond to communication and make decisions about whether to vaccinate or adopt other protective behaviour, and an epidemic model that simulates the spread of influenza. The key model entities are: (i) messages, which together implement the communication plans; (ii) individuals, who receive communication and make decisions about whether to adopt protective behaviour; and (iii) regions, which hold information about the local epidemic state. The major flow of influence is the effect that communication has on attitude and hence behaviour, which affects epidemic transmission and hence incidence. Incidence contributes to perceived risk, which influences behaviour and establishes a feedback relationship (see Badham and Gilbert 2015 for the detailed specification). A fuller description of the model and discussion of its uses can be found in Barbrook-Johnson et al. (2017). A more technical paper on a novel model calibration approach using the Tell Me as an example is presented in Badham et al. (2017).
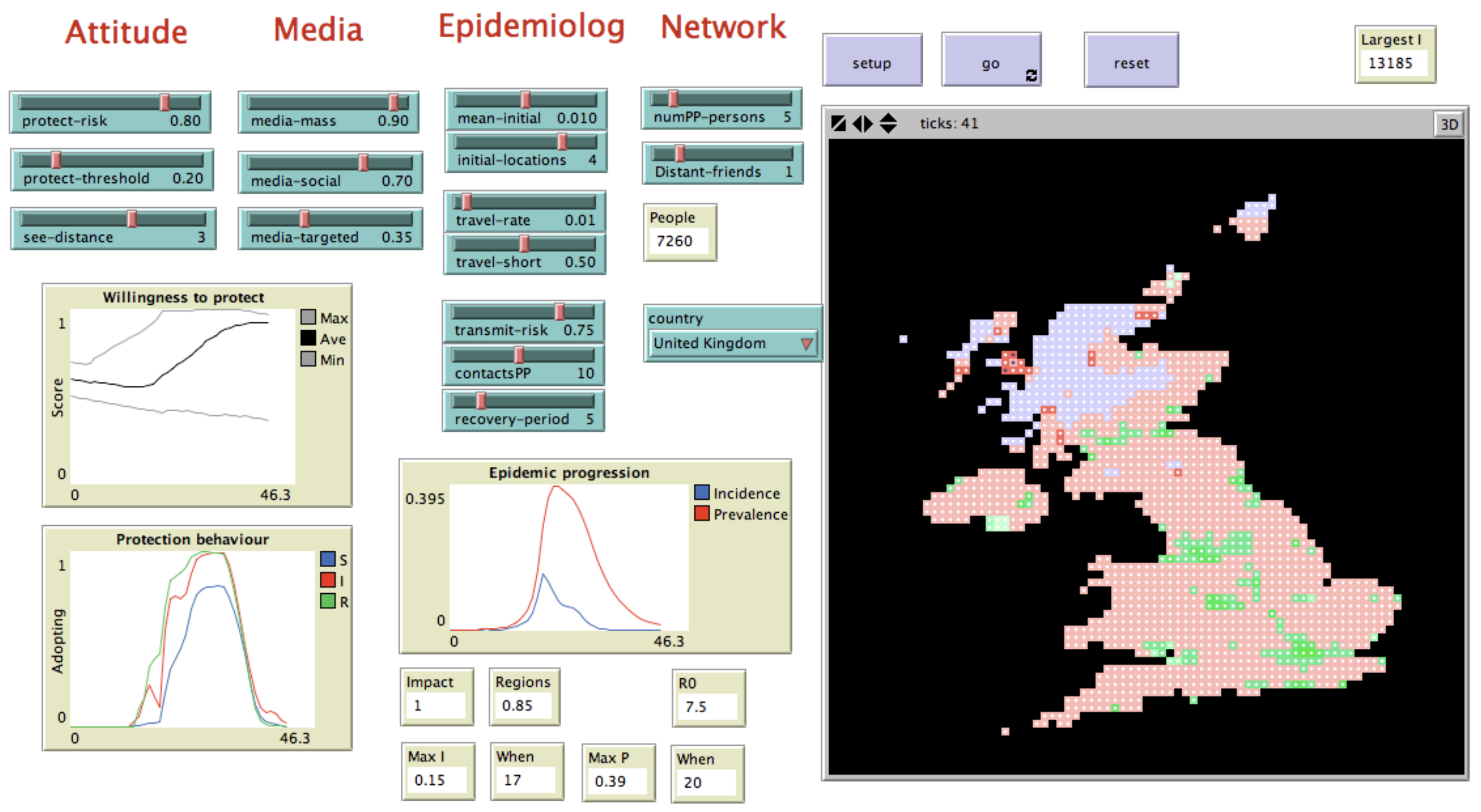
Drawing on findings from stakeholder workshops and the results of the model itself, the modelling team suggest the TELL ME model can be useful: (i) as a teaching tool, (ii) to test theory, and (iii) to inform data collection (Barbrook-Johnson et al. 2017).
HOPES
Practice theories provide an alternative to the theory of planned behaviour and the theory of reasoned action to explore sustainability issues such as energy use, climate change, food production, water scarcity, etc. The central argument is that the routine activities (aka practices) that people carry out in the service of everyday living (e.g. ways of cooking, eating, travelling, etc.), often with some level of automaticity developed over time, should be the focus of inquiry and intervention if the goal is to transform energy- and emissions-intensive ways of living.
The Households and Practices in Energy use Scenarios (HOPES) agent-based model (Narasimhan et al. 2018) was developed to formalise key features of practice theories and to use the model to explore the dynamics of energy use in households. A key theoretical feature that HOPES sought to formalise is the performance of practices, enabled by the coming together of appropriate meanings (mental activities of understanding, knowing how and desiring, Reckwitz 2002), materials (objects, body and mind) and skills (competences). For example, a laundry practice could signify a desire for clean clothes (meaning) realised by using a washing machine (material) and knowing how to operate the washing machine (skill); performance of the practice then results in energy use.
HOPES has two types of agents: households and practices. Elements (meanings, materials and skills) are entities in the model. The model concept is that households choose different elements to perform practices depending on the socio-technical settings unique to each household. The performance of some practices result in energy use while some do not, e.g., using a heater to keep warm results in energy use whereas using a jumper or blanket does not incur energy use. Furthermore, the repeated performance of practices across space and time causes the enabling elements to adapt (e.g. some elements are used more popularly than others), which subsequently affects the future performance of practices and thereby energy use. A rule-based system, developed based on empirical data collected from 60 UK households, was included in HOPES to enable households to choose elements to perform practices. The rule-based approach allowed organising the complex contextual information and socio-technical insights gathered from the empirical study in a structured way to choose the most appropriate actions when faced with incomplete and/or conflicting decisions. HOPES also includes sub-models to calculate the energy use resulting from the performance of practices, e.g. a thermal model of a house is built in to consider the outdoor temperature, the type and size of heater, and the thermostat setpoint to estimate the energy used for thermal comfort practices in each household.
The model is used to test different policy and innovation scenarios to explore the impacts of the performance of practices on energy use. For example, the implementation of a time of use tariff demand response scenario shows that while some demand shifting is possible as a consequence of pricing signals, there is no significant reduction in energy use during peak periods as many households cannot put off using energy (Narasimhan et al. 2018). The overall motivation is that by gaining insight into the trajectories of unsustainable energy consuming practices (and underlying elements) under different scenarios, it might be possible to propose alternative pathways that allow more sustainable practices to take hold.
SWAP
The SWAP model (Johnson 2015a; Johnson 2015b) is an agent-based model of farmers’ making decisions about adopting soil and water conservation (SWC) practices on their land. Developed in NetLogo (Wilensky 1999), the main agents in the model are farmers, who are making decisions about whether to practice SWC or not, and extension agents who are government and non-governmental actors who encourage farmers to adopt. Farmers can also be encouraged or discouraged to change their behaviour depending on what those nearby and in their social networks are doing. The environment is a simple model of the soil quality (Figure 2). The main outcomes of interest are the temporal and spatial patterns of SWC adoption. A full description can be found in Johnson (2015a) and Johnson (2015b).
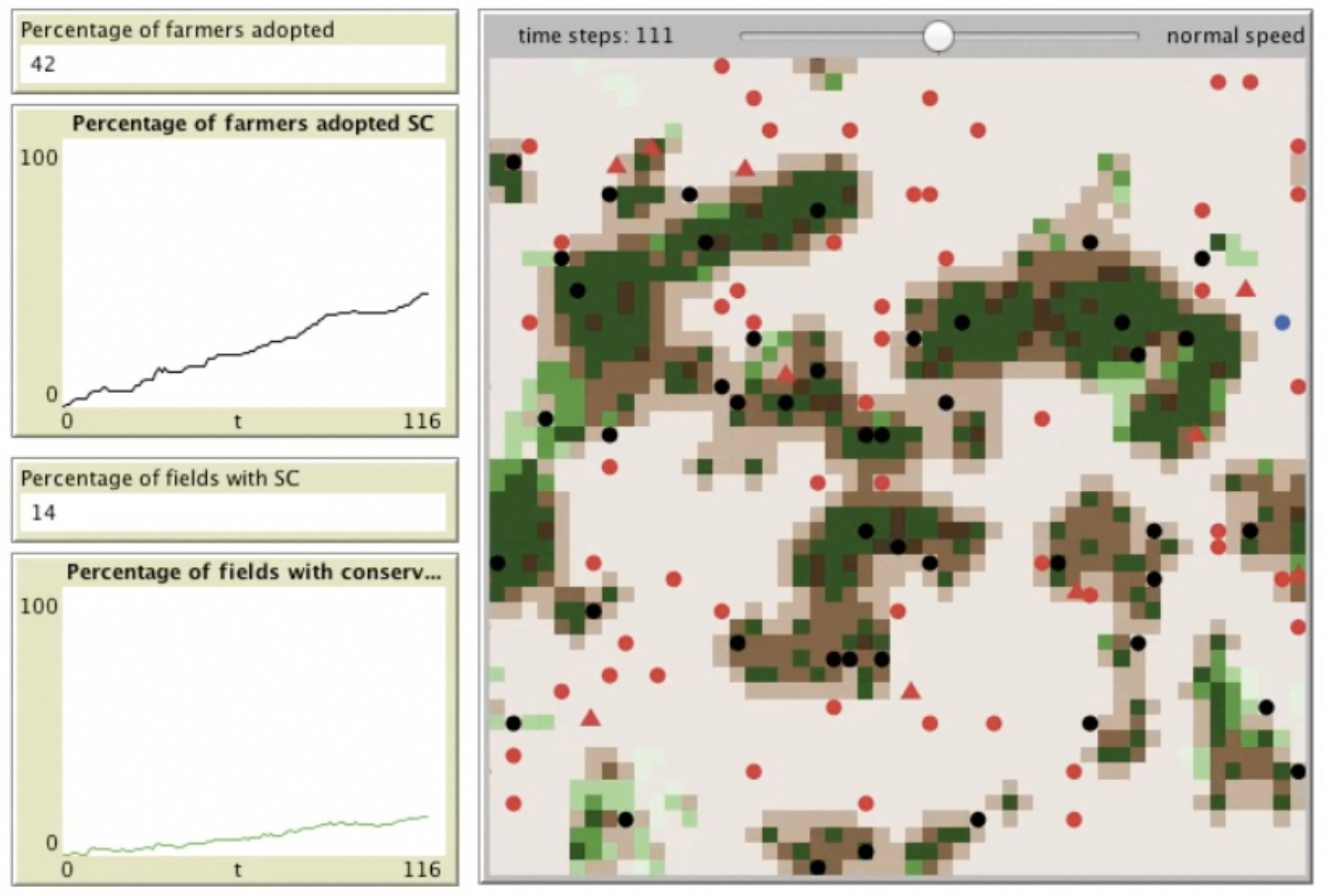
The SWAP model was developed: (i) as an ‘interested amateur’ to be used as a discussion tool to improve the quality of interaction between policy stakeholders; and (ii) as an exploration of the theory on farmer behaviour in the SWC literature.
The model’s use as an ‘interested amateur’ was explored with stakeholders in Ethiopia. Using a model as an interested amateur is a concept inspired by Dennett (2013). Dennett suggests experts often talk past each other, make wrong assumptions about others’ beliefs, and/or do not wish to look stupid by asking basic questions. These failings can often mean experts err on the side of under-explaining issues, and thus fail to come to consensus or agreeable outcomes in discussion. For Dennett, an academic philosopher, the solution is to bring undergraduate students - interested amateurs - into discussions to ask the simple questions, and generally force experts to err on the side of over-explanation.
The SWAP model was used as an interested amateur with a different set of experts, policy makers and officials in Ethiopia. This was done because policies designed to increase adoption of SWC have generally been unsuccessful due to poor calibration to farmers’ needs. This is understood in the literature to be a result of poor interaction between the various stakeholders working on SWC. When used, participants recognised the value of the model and it was successful in aiding discussion. However, participants described an inability to innovate in their work, and viewed stakeholders ‘lower-down’ the policy spectrum as being in more need of discussion tools. A full description of this use of the model can be found in Johnson (2015b).
INFSO-SKIN
The European Commission was expecting to spend around €77 billion on research and development through its Horizon 2020 programme between 2014 and 2020. It is the successor to the previous, rather smaller programme, called Framework 7. When Horizon 2020 was being designed, the Commission wanted to understand how the rules for Framework 7 could be adapted for Horizon 2020 to optimise it for current policy goals, such as increasing the involvement of small and medium enterprises (SMEs).
An agent-based model, INFSO-SKIN, was built to evaluate possible funding policies. The model was set up to reproduce the funding rules, the funded organisations and projects, and the resulting network structures of the Framework 7 programme. This model, extrapolated into the future without any policy changes, was then used as a benchmark for further experiments. Against this baseline scenario, several policy changes that were under consideration for the design of the Horizon 2020 programme were then tested, to understand the effect of a range of policy options: changes to the thematic scope of the programme; the funding instruments; the overall amount of programme funding; and increasing SME participation (Ahrweiler et al. 2015). The results of these simulations ultimately informed the design of Horizon 2020.
Silent Spread and Exodis-FMD
Following the 2001 outbreak of Foot and Mouth Disease (FMD), the UK Department of the Environment, Food and Rural Affairs (Defra) imposed a 20-day standstill period prohibiting any livestock movements off-farm following the arrival of an animal. The 20-day rule caused significant difficulties for farmers. The Lessons Learned Inquiry, which reported in July 2002, recommended that the 20-day standstill remain in place pending a detailed cost-benefit analysis (CBA) of the standstill regime.
Defra commissioned the CBA in September 2002 and a report was required in early 2003 in order to inform changes to the movement regime prior to the spring movements season. This timescale was challenging due to the short timescales and limited data available to inform the cost risk benefit modelling required. A top down model was therefore developed that captured only the essential elements of the decision, combining them in an influence diagram representation of the decision to be made. As wide a range of experts as possible were involved in model development, helping inform the structure of the model, its parameterisation, validation and interpretation of the results. An Agile approach was adopted with detail added to the model in a series of development cycles guided by a steering group.
The resultant 'Silent Spread' model showed that factors such as time to detection of disease, are much more important than length of standstill in determining the size of an outbreak (Risk Solutions 2003). The modelling was critical to the Government’s decision to relax the 20-day movement control to 6 days, subject to commitments from the livestock industry. The iterative, participatory development process generated an unprecedented level of ‘buy-in’ to the results in an area which had previously been marked by deep controversy.
Following this, Defra commissioned further modelling to inform the design of the FMD contingency plan to be followed in the event of an outbreak. For this application, a detailed ‘bottom-up’ model was needed that could reproduce the detailed mechanisms of disease spread and enable the impacts of different control strategies on the spread of a disease to be explored (Risk Solutions 2005).
The model was implemented as an agent-based model using the Exodis™ disease modelling framework (Figure 3). The framework builds a heterogeneous geo-spatial representation of the UK based on farm census data, sets-up the various FMD disease transmission mechanisms and integrates the effects of different control strategies and the resources required to carry out these strategies. The agents in the model are farms. For a given set of outbreak starting conditions and for a given control strategy, the model provides detailed information on how the outbreak might evolve, calculating parameters such as the number of premises infected, the duration of the outbreak, the number of animals culled and/or vaccinated, etc. It produces distributions for each of these parameters to reflect the range of potential outcomes for any outbreak.
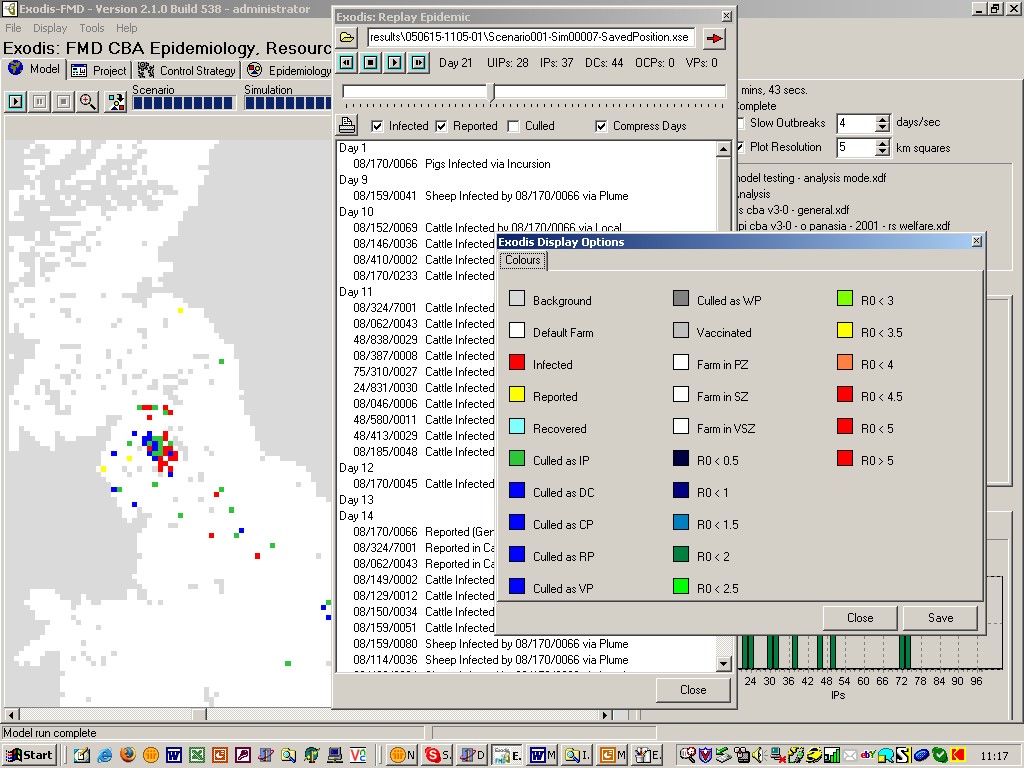
Following the cost benefit analysis work Defra retained a decision support tool that provides a training aid for use during exercises, and to inform decisions in the event of an actual outbreak. The model was used during the emerging outbreak of FMD in 2007 and continues to be used to test proposed changes to the control regime.
The Abstractor Behaviour Model
The abstraction of water from rivers and aquifers in England is controlled by a licensing regime established in the 1960s. The UK Government wish to reform the system to one that encourages abstractors to manage water efficiently and work together to make best use of water. Water abstraction management is a classic ‘wicked’ problem in that it is highly resistant to resolution. Previous attempts to reform the system have failed, partly through not engaging stakeholders in the need for, and nature of, a solution.
Assessing the costs, risks and benefits of the different ways of reforming the system is complex. It needs to take into account:
- The interactions between a complex natural system and the abstractors (including the public water supply, power producers, farmers, and industry),
- That economic, social and climate conditions will change in ways that we cannot predict, and
- The complex way that the new measures will influence individual abstractor behaviours on a day-by-day, year-by-year basis.
Agent-based modelling was ideally suited to explore how the existing and proposed reforms might operate. A multidisciplinary team worked with a wide range of experts and stakeholders to develop an agent-based economic behavioural model integrated with catchment hydrological models on a daily time-step basis (Risk Solutions 2015).
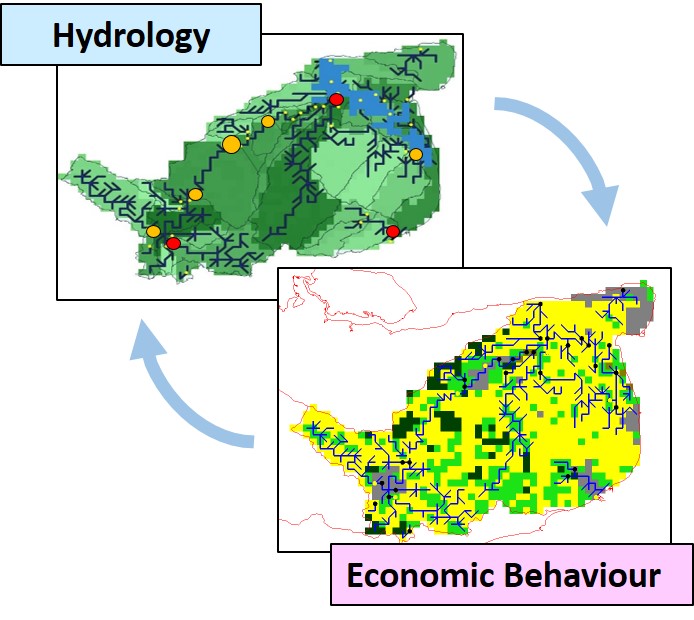
The agent population consists of all of the businesses that have a licence to take water from the rivers and aquifers in a particular river basin (Figure 4). The river basin is modelled in detail using a hydrological model of the rivers, aquifers, and land use with a geo-spatial resolution of 1km by 1km. Each agent makes a series of strategic and operational decisions, and the decision-making evolves with time as water demand and availability changes with economic and climate change. The policy options control water levels in the modelled rivers and aquifers using different mechanisms, and allow different types of water rights trading between agents. The successful achievement of environmental standards is monitored by regulator agents, who take action to further restrict abstraction permissions if necessary. The model was used to explore in detail how the reforms would work in practice. It exposed many unanticipated and often unwelcome effects, and so enabled the design of the reforms to be improved.
Key Lessons for Policy Modellers
From our experience, derived from the example policy models described in the previous section and others we have worked on, we suggest the following are some of the key lessons modellers should carry into their policy modelling efforts:
The process is as important, and often more so, than the outputs
Many government decision processes understandably require quantitative data, for example to complete a Regulatory Impact Appraisal template. A simple set of cost benefit values provides clear, compelling arguments in support of a decision or conclusion. In complex, evolving environments, however, reducing the answer to a limited set of numbers may be neither possible, nor desirable – conveying as they do a level of certainty in understanding which is rarely achievable. Policy modelling in complex environments should be as much, or more, about developing understanding about a problem or decision as it is about the number at the end. Care is needed to ensure that the need, or desire, for numbers, alongside unfamiliarity with, or suspicion of, unfamiliar approaches, does not drive the choice of sub-optimal modelling approaches.
In the water abstraction reform work (Section 4.21), although the modelling did generate numbers to input to the Impact Assessment Template (absorbing a significant proportion of the modelling effort), the greatest benefit of the work was the contribution to designing the policy, which was intimately informed by the more exploratory aspects of the modelling, including both: the discipline provided by the need to articulate the reforms in a way that could be represented in the model; and the understanding of the complex, emergent nature of the system uncovered through running multiple scenarios, sensitivity analyses and what-if scenarios.
In the SWAP model (Section 4.9), the policy value lay entirely in the process of interrogating the model, and using it as a basis for discussions, sharing assumptions and building consensus. An interesting extra dimension was that critiquing design choices generated value for stakeholders. In this role the model is a boundary object (Star and Griesemer 1989), and ‘interested amateur’ (Johnson 2015b) as described above. With stakeholders who do not regularly work together, and/or who do not have the capacity to take ownership and undertake continued use and maintenance of a model, this process-based value is even more likely to be the main benefit of the modelling process.
In the Tell Me model (Section 4.2), we find a similar message. In this example, detailed micro-validation (i.e. sense checking model rules and assumptions) and exploration of their effects on outcomes was one of the main benefits to public health stakeholders involved in the project. The lack of data available to allow rigorous formal validation of the model meant that this was one of the most valuable aspects of the modelling exercise.
The HOPES model (Section 4.5) introduced analysts concerned with developing policies to manage household energy demand to the idea of considering social practices as an alternative to assuming household energy use is determined by individual rational actors making decisions based primarily on cost. The fact that the HOPES model could generate plausible outputs using social practice theory as its foundation was probably more significant to stakeholders than the actual values it yielded.
Models need to be at an appropriate level of abstraction
No model can fully reflect the real world: some details need to be omitted and some boundary needs to be drawn around what is to be modelled. However, it is not the case that the most detailed model is necessarily the best. On the contrary, highly-detailed models may require more data than is or could be available; can be hard to calibrate and validate; and, most importantly, can be hard to understand. Clients, modellers and stakeholders can all struggle with the idea that less can be more and get drawn into trying to model reality instead of the decision essentials. On the other hand, a model that is too simple or too abstract may be impossible to validate, because there is nothing in the model that corresponds to empirical observation, and because the behaviour of the model may bear little relationship to what happens in the world. The optimal level of abstraction will depend on the purpose of the modelling and the nature of the system being modelled (Edmonds 2005). One of the signs of good modelling is pitching the model at the right place in between the two extremes.
The Silent Spread model described in Section 4.15 was a simple model developed at a high level of abstraction. The modelling was required to support a single decision question, could the livestock movement standstill period be reduced or removed? At the time Defra did not routinely collect information on the movement of animals, and so data to inform the modelling was limited. The solution was therefore to develop an abstract model, capturing only those elements essential to the decision. With more time, and a much richer fund of data, it was possible to develop a much more detailed representation of disease spread for the Exodis-FMDTM model. In this case it was also necessary to capture the dynamic interaction of the various control strategies with the spread of a disease, in order to provide a basis for testing these.
HOPES (Section 4.5) started as an abstract model that served as a proof that it is possible to go beyond the conventional but limited approach of analysing energy demand in terms of rational and individual decision making to model energy consuming social practices in the household. Only once this proof of concept version had been demonstrated did the model get extended and refined to incorporate specific social practices (maintaining a comfortable environment, doing the laundry, etc.) that could be calibrated against the data collected from energy sensors installed in the sample households.
One motive for making the HOPES model more concrete was a desire to link it to existing models of the UK energy supply system. These modelled electricity supply from electricity power stations, wind farms, etc. and the interconnecting grid and have been used to develop scenarios for informing decisions about the optimal ways of developing the whole energy system to meet low carbon targets in 2050. However, these supply models incorporated demand functions based on rather simple household utility maximisation assumptions. The HOPES model has been used to improve this aspect of the supply models, but not without difficulty, stemming from the overall complexity of the models, the different disciplinary approaches (the supply models are based on optimising using linear programming techniques; HOPES is an agent-based model), and the different time scales of the simulations (the supply models use time steps of days or years, while HOPES has hourly time steps). This example illustrates well the fact that one needs to think carefully about the appropriate level of abstraction of models, not only in terms of their relevance for stakeholders but also to fit them properly into what can be a whole ecology of related models.
Data and validation challenges must be recognised, but not used as an excuse not to model, or not to use the results
Data challenges
Data is never perfect. Lack of, or poor quality data, frustrates the parameterisation and validation of models. However, lack of data should never be used as an excuse not to model, or not to model an aspect of a problem that is important to the decisions to be made. Collaborative approaches, formal elicitation of expert judgement, explicit modelling of uncertainty and sensitivity analysis can all be used to address a lack of data.
In the Tell Me example (Section 4.2), despite an initial belief by modellers and stakeholders that data was available, it became clear that there was no data that connected policy interventions with behavioural change and outcomes. Behavioural outcome data was at an aggregate level, meaning it is impossible to understand the individual level impacts of the intervention. Data directly connecting intervention and outcome, for each individual, is vital for choosing values for effect size parameters in the model.
In this example, the lack of data should not be seen as a reason not to model. The motivations to model remain. Rather, the lack of data made explicit by the model should be used to inform future data collection. As Barbrook-Johnson et al. (2017) states,
"[data] collection must go alongside continued development of theory and models of decision-making. Improved theories of individual decision-making and interaction will give models a stronger footing on which to base their assumptions. As data and theory improve, so too will the (prototype) models developed using that support. This could then lead to improved data collection and theory building, creating a positive feedback between the three."
Validation challenges
Lack of data can present particular challenges to the formal validation of models, particularly in the complex, changing environments where modelling to explore how the future might unfold can be most useful. In the Tell Me example, the data on behavioural outcomes through time either did not exist, or tended to be at a relatively low resolution. This meant that there was not enough longitudinal outcome data for the model results to be compared with.
Lack of a comprehensive dataset for validation should not be taken to imply the model cannot be validated for its particular purpose. In these circumstances, a layered approach to validation should be used: formal quality assurance processes should be applied from the outset, including the selection of the modelling approach (see for example the Aqua Book, HM Treasury 2015, and Edmonds 2016), alongside formal documented verification and validation processes. Formal validation should involve subject matter experts in collaboration with model output users and modellers and should be an integral part of model development.
Validation must ensure that the model (1) makes technical or scientific sense (2) can reproduce recorded reality (3) is fit for the use it is designed for. Taylor (2013) includes a useful checklist of these and other issues to address and questions to ask when using models in decision making.
The Silent Spread example (Section 4.15) illustrates how a model can be developed and validated in the absence of much ‘hard’ data, through a process of scrutiny of all stages of model development and result generation by subject matter experts, modellers, users and wider stakeholders.
Model development and use needs to be Agile and collaborative
Agile, collaborative processes ensure models remain focused on the policy need and provide for more effective peer review and scrutiny of the modelling process. This requires a high degree of trust between commissioners and modellers from the outset. Policy makers, analysts, model output users, stakeholders, and peer reviewers should be involved, not just at the problem definition, user needs stage, but throughout to ensure that the modelling approach, model structure and level of abstraction, parameterisation, analysis and interpretation of the results remain fit for purpose and focused on need.
At the scoping stage, there needs to be a honest discussion about the best modelling approach and whether existing models will meet needs. Great care needs to be taken when using models for applications they were not originally designed for to ensure that the underlying structure of the model is fit for purpose.
An Agile development approach (Abrahamsson 2017), which iteratively adds functionality and detail to the model through cycles of development, testing and scrutiny, is a good way of managing the tendency for modellers and clients alike to drive towards too great a level of detail and more realistic representations in models than is optimal.
Finally, modellers should be involved in helping interpret the results for decision making. It is impossible to capture in a report all the nuances of the model simplifications, data weaknesses etc. in a way that policy makers can use reliably.
The Silent Spread work (Section 4.15) used a highly participatory approach leading to much improved understanding and cooperation between Defra and industry stakeholders. In contrast, the INFO-SKIN model (Section 4.13) was developed in response to an invitation to tender that had the effect of distancing the stakeholders, that is, the relevant policy makers, from the modellers. The people from the European Commission (EC) who were the clients only met the modellers at the beginning, middle and at the end of the model development and were not therefore much involved in its design. Moreover, the EC personnel changed during the development and by the end there was a rather poor understanding by the clients of the purpose and capabilities of the model. A further issue was that the clients wanted the modellers to draw out specific policy recommendations from the model, while the modellers were happy to test policy options proposed by the clients but did not think it appropriate that they should be devising policies themselves. These are all symptoms of the absence of proper collaboration between the modellers and the commissioners.
The ethics of modelling
Policy modelling requires careful consideration of a wide range of ethical issues, not least because policy models have the potential to change policy and thus directly affect people’s lives. In addition to the basic imperative to ensure that a model is fit for purpose, as discussed above, there is also a need to consider issues arising in connection with the data used to build and calibrate the model and the way in which the results of the model are presented.
When personal data is collected, either explicitly through, for example, a survey, or implicitly, as administrative records or as the side-effect of other activities (such as using social media or mobile phones), not only does one need to abide by data protection laws, but also need to ensure that appropriate informed consent for such use of the data has been obtained (see, for example, the ethical guidelines published by the British Sociological Association (BSA 2017), the Association of Internet Researchers (AoIR 2012) and the Association of Computing Machinery (ACM 1992). There remains a need to bring these guidelines together and to draw out their relevance to modelling.
An important consideration is whether data is representative of the population being modelled. As artificial intelligence researchers have discovered to their cost, basing a model on biased data can lead to biased results and it can be hard to detect this after the event (Knight 2017). This is especially a problem with ‘big data’, where it is easy to assume that because one has a very large volume of data, it must be representative although important but numerically small minorities may be absent.
The results derived from models are always subject to a degree of uncertainty. However, it is easy for modellers and especially the users of models to downplay, intentionally (because they do not believe it will be well received) or unintentionally (expert bias), the degree of uncertainty present, and the implications of that uncertainty for making policy decisions. Users may also put pressure on modellers to downplay uncertainty. Modellers should be clear and confident in their communication of uncertainty but also informative. The user needs to understand what the uncertainty means in terms of the decisions or communications they need to make. This is made more problematic if the model is complex and presented to users as a ‘black box’ that generates results without users being able to investigate for themselves the logic and the assumptions lying behind those results. This is another reason for encouraging collaboration between users and modellers: users can follow the model development process and may then get at least a glimpse of its workings and the assumptions being made; modellers can better understand the context and ensure that the results are presented in a form that is useful.
In the Silent Spread example, decision information was needed quickly, when there was little data available to inform modelling. As wide a range of stakeholders, experts and officials as possible was actively involved in designing, populating and testing the model. Working groups met regularly at every stage of the modelling process. Once results began to emerge the group helped to interrogate and interpret the results, suggesting a range of ways of refining the modelling to test new hypotheses suggested by the outputs. A variety of different ways of presenting the uncertainty in the results was used, in particular the level of residual risk associated with each of the policy options under consideration was clearly illustrated allowing decision makers to take this into account in reaching their decision. The process produced unparalleled acceptance of the final conclusions for policy with the model being described by one expert as the “collective brain of the group”.
Communicating the modelling process, structure and results needs careful planning
Communication is necessary to clearly explain results, and their limitations, ensure that the outputs are used appropriately, and build confidence in the modelling process and outputs. It is the nature of model outputs, consisting of numbers and charts, to appear more certain than they are, and this can mean that the boundary between data and assumption is overlooked. Poor past experience can lead to distrust of modelling. Active collaboration builds confidence in and champions for the work, but it is not possible to involve everyone. Changes of personnel, both in the modelling team and in the policy client can also lead to problems. In complex modelling environments, it is easy to underestimate the communication challenges.
In the Silent Spread work (Section 4.15) the modellers had to work hard to break distrust of modelling brought about by issues surrounding the use of predictive models to support the pre-emptive, contiguous cull during the 2001 outbreak of disease. While at first it was hard to get stakeholders with entrenched and often opposed positions about what the ‘right’ answer was to talk constructively, the model gave them a neutral space to share different perspectives and test the results of these.
In the SWAP model example (Section 4.9), trust was less problematic. Rather it was the communication of the model design, and the conclusions the model (and modeller) could make, which needed to be communicated to stakeholders that were not familiar with formal computational modelling approaches. This led to an accessible form of communicating the model being designed. This still needed to provide the detail of the model assumptions and rules so that it could be used as the basis of discussion. To do this, a combination of pseudo code, simplified (and jargonless) Unified Modelling Language diagrams, and projector presentations of results and the model running were used. The emphasis was placed strongly on the assumptions and step by step rules of the model, rather than the results.
Models need to be maintained
If models can continue to have a role in policy monitoring, development and evaluation after their initial results, they deliver better value, but ensuring that models are properly maintained is difficult within government procurement processes and structures. Plans for maintenance of the model should be discussed at the start of the modelling project.
Open source models are attractive because communities can continue to maintain and scrutinise them, but this is not always an option for policy models which must continue to represent complex policy accurately over time, accounting for changes in policy and the policy environment. Decision makers need to have confidence that this will happen and are unlikely to be prepared to rely on voluntary efforts. Moreover, many policy models need to use confidential data, which cannot be made open source at the necessary level of disaggregation.
Of the models described in Section 4, only Exodis (Section 4.15) is currently being maintained. Securing long-term maintenance arrangements is thus a challenge that is so far rarely met properly.
Conclusions
The technology required for modelling complex domains is in place and increasingly easy to use. However, for policy modelling to achieve its full potential, there needs to be more attention paid to the processes of model development and use. As we have illustrated in this paper, there are many pitfalls along the way in making policy models effective and used. Much of this is ‘craft knowledge’, gained from experience and from making mistakes, which is why we have described key lessons that we have learned from our own varied experience. Nevertheless, where the costs or risks associated with a policy change are high, and the context is complex, it is not only common sense to use policy modelling to inform decision making, but it would be unethical not to.
The most important requirement in our view for successful policy modelling is to encourage communication and collaboration among those involved: the modellers themselves, the clients and stakeholders, the suppliers of data, the users of the model outputs and so on. Academia still has a tendency to work within an ivory tower, making results, and models, available to users only once they have been fully developed and after the work has been published in the research literature. While this approach may work for some formal modelling, it almost certainly will not yield useful policy models that are actually used by decision makers. Instead, as we have emphasised above, policy modelling needs to be collaborative, iterative and Agile. Such an approach has many benefits. Firstly, it provides a sense of ownership of the model and encourages commitment from users about what they may come to see as ‘their’ model, rather than some black box that someone else is imposing on them. Secondly, collaboration helps to prevent modellers making naïve assumptions about the target domain, which is easy to do if one is not a domain expert. Thus, through collaboration, the modellers are educated about the complexities of the world they are trying to represent, but equally, the users are educated about the capabilities and limitations of the model that they are helping to develop. Thirdly, active engagement of stakeholders can help parameterise and sense check models, even where ‘hard’ data is sparse. Lack of data should never be used as an excuse not to model, but the approach needs moderating, an iterative, participative approach to modelling allows data needs to be identified and ways of addressing these developed.
Such a collaborative style of working may be foreign to many government agencies and can involve delicate negotiations about confidentiality, privacy and access to data. However, there does seem to be an inexorable trend towards the greater use of simulation, machine learning and artificial intelligence to aid decision making in government and business, so the culture may have to change to permit and even encourage a more collaborative, Agile modelling approach. When it does, policy modelling will truly have come of age.
Acknowledgements
The support of the following for the preparation of this paper and the examples mentioned is acknowledged:For SWAP: This work was supported by the Economic and Social Research Council (Grant No. ES/J500148/1). Additional support was received from the International Livestock Research Institute and the International Water Management Institute.
For TELL ME: This research has received funding from the European Research Council under the European Union’s Seventh Framework Programme (FP/2007-2013), Grant Agreement number 278723. The full project title is TELL ME: Transparent communication in Epidemics: Learning Lessons from experience, delivering effective Messages, providing Evidence, with details at http://tellmeproject.eu/.
For WholeSEM: The UK Engineering and Physical Sciences Research Council supported this work through the Whole Systems Energy Modelling Consortium (WholeSEM) project (grant EP/K039326/1). http://www.wholesem.ac.uk/.
For Silent Spread and Exodis: This work was funded by Defra. The Risk Solutions’ lead modeller for Silent Spread was Chris Rees and for Exodis, Jon Pocock.
For the Water Abstraction Model: This work was supported by Defra, the Environment Agency, the Welsh Government and Natural Resources Wales. The Risk Solutions’ lead modeller was Jon Pocock. Risk Solutions led a consortium that also included HR Wallingford, Amec, London Economics and Wilson Sherriff.
For CECAN: The Centre for the Evaluation of Complexity Across the Nexus is supported by the Economic and Social Research Council, the Natural Environment Research Council, BEIS, DEFRA, the Environment Agency and the Food Standards Agency, grant ES/N012550/1. http://www.cecan.ac.uk.
Notes
- To help address these issues it is useful for modellers to consider and use the wealth of research on the role of research in the policy process, the science-policy interface and research utilisation, and evidence-based policy. It is not the purpose of this paper to discuss this research, readers are referred to the following sources:
- On the science-policy interface researchers have considered how the two communities of ‘policy makers’ and ‘researchers’ interact. Historically, the divide has been seen as clear (Weiss 1976; Caplan et al. 1975; Caplan 1979), but more recent work explores the continuous interaction and movement between the communities (e.g. Cash et al. 2003; Clark and Holmes 2010).
- On how research is actually used, there have been many conceptualisations and overviews (e.g. Jager 1998; Weible 2008). The most well-known is Weiss (1979) which outlines how research can be used as evidence; a problem-solving tool; one source of information among many; justification for already-made decisions; a tool to delay difficult or sensitive decisions (i.e. ‘we need to do more research on this’, ‘kick it into the long grass’); a source of general enlightenment; and finally, one of many pursuits of society (alongside policy, art, media, law etc.) which all influence each other. A lesson from much of this work is that it is often difficult or impossible to foresee how a model may be used, and this has implications for how the model is designed and maintained, a point we return to in Section 5.
- On evidence-based policy, Cairney (2018) is an excellent starting point.
References
ABRAHAMSSON, P., Salo, O., Ronkainen, J., & Warsta, J. (2017). Agile software development methods: Review and Analysis. arXiv:1709.08439. Accessible at: https://arxiv.org/abs/1709.08439.
ACM (1992). ACM code of ethics and professional conduct. Accessible at: https://www.acm.org/about-acm/acm-code-of-ethics-and-professional-conduct.
AHRWEILER, P., Schilperoord, M, Pyka, A. & Gilbert, N. (2015). Modelling research policy: Ex-ante evaluation of complex policy instruments. Journal of Artificial Societies and Social Simulation 18(4), 5: https://www.jasss.org/18/4/5.html. [doi:10.18564/jasss.2927]
ANZOLA, D., Barbrook-Johnson, P., Salgado, M., & Gilbert, N. (2017). 'Sociology and non-equilibrium social science.' In Johnson, J., Nowak, A., Ormerod, P., Rosewell, B., & Zhang, Y.-C. (Eds.), Non-Equilibrium Social Science and Policy. Springer.
AOIR (2012). Ethical decision-making and Internet research. Recommendations from the AoIR Ethics Working Committee (version 2.0). Accessible at: https://aoir.org/reports/ethics2.pdf.
BADHAM, J., Jansen, C., Shardlow, N., & French, T. (2017). Calibrating with multiple criteria: A demonstration of dominance. Journal of Artificial Societies and Social Simulation, 20(2), 11: https://www.jasss.org/20/2/11.html.
BARBROOK-JOHNSON, P., Badham, J., & Gilbert, N. (2017). Uses of agent-based modeling for health communication: The TELL ME case study. Health Communication. 32(8), 939-944. [doi:10.1080/10410236.2016.1196414]
BOESCHEN, S., Gross, M., & Krohn, W. (eds.) (2017). Experimentelle Gesellschaft. Das Experiment als wissensgesellschaftliches Dispositiv. Baden-Baden: Nomos. (Experimental Society. The Experiment as Dispositive of the Knowledge Society).
BORUCH, R. (1997). Randomised Experiments for Planning and Evaluation.: A Practical Guide. Thousand Oaks, CA: Sage. [doi:10.4135/9781412985574]
BSA (2017). Guidelines on ethical research. Accessible at: https://www.britsoc.co.uk/ethics.
BYRNE, D., & Callaghan, G. (2014). Complexity Theory and the Social Sciences: The State of The Art. Abingdon/ New York, NY: Routledge.
CABINET Office (2003). Trying it out: The role of ‘pilots’ in policy-making: Report of a review of government pilots. London: Cabinet Office, Strategy Unit.
CAIRNEY, P. (2013). Standing on the shoulders of giants: How do we combine the insights of multiple theories in public policy studies?. Policy Studies Journal, 41(1), 1-21. [doi:10.1111/psj.12000]
CAIRNEY, P. (2018). The UK government’s imaginative use of evidence to make policy. British Politics, Online First, 1–22.
CAPLAN, N. (1979). The two-communities theory and knowledge utilization. American Behavioral Scientist. 22(3), 459-470. [doi:10.1177/000276427902200308]
CAPLAN, N., Morrison, A., & Stambaugh, R. (1975). The Use of Social Science Knowledge in Policy Decisions at the National Level: A Report to Respondents. Ann Arbor, MI: Institute for Social Research, University of Michigan.
CASH, D.W., Clark, W.C., Alcock, F., Dickson, N.M., Eckley, N., Guston, D.H., Jäger, J., & Mitchell, R.B. (2003). Knowledge systems for sustainable development. Proceedings of the National Academy of Sciences of the United States of America, 100(14), 8086-91. [doi:10.1073/pnas.1231332100]
CLARK, H., & Taplin, D. (2012). Theory of Change Basics: A Primer on Theory of Change. New York, NY: Actknowledge. Accessible at: http://www.theoryofchange.org/wp-content/uploads/toco_library /pdf/ToCBasics.pdf.
CLARK, R., & Holmes, J. (2010). Improving input from research to environmental policy: challenges of structure and culture. Science and Public Policy. 37(10), 751-764. [doi:10.3152/030234210X534887]
DENNETT, D.C. (2013). Intuition Pumps and Other Tools for Thinking. New York, NY: W. W. Norton & Company.
EDMONDS B., & Moss S. (2005). 'From KISS to KIDS – An ‘Anti-simplistic’ Modelling Approach.' In Davidsson P., Logan B., Takadama K. (eds), Multi-Agent and Multi-Agent-Based Simulation. MABS 2004. Lecture Notes in Computer Science, vol 3415. Berlin, Heidelberg: Springer. [doi:10.1007/978-3-540-32243-6_11]
EDMONDS, B. (2016). Review of: The Aqua Book: Guidance on Producing Quality Analysis for Government. Journal of Artificial Societies and Social Simulation. 19(3), 7: https://www.jasss.org/19/3/reviews/7.html.
GREENBERG, D., & Shroder, M. (1997). The Digest of Social Experiments. Washington D.C.: Urban Institute Press.
GROSS, M., & Krohn, W. (2005). Society as experiment: sociological foundations for a self-experimental society. History of the Human Sciences, 18(2), 63-86.
HAUKE, J., Lorscheid, I., & Meyer, M. (2017). Recent development of social simulation as reflected in JASSS between 2008 and 2014: A citation and co-citation analysis. Journal of Artificial Societies and Social Simulation, 20(1), 5: https://www.jasss.org/20/1/5.html. [doi:10.18564/jasss.3238]
HILLS, D. (2010). Logic Mapping: Hints and Tips. Report commissioned by HM Department of Transport (UK). Accessible at: https://www.gov.uk/government/uploads/system/uploads/attachment_data/file/3817/logicmapping.pdf.
HM TREASURY (2011). The Magenta Book - Guidance for Evaluation. Accessible at: https://www.gov.uk/government/publications/the-magenta-book.
HM TREASURY (2013.) The Green Book: Appraisal and Evaluation in Central Government. Accessible at: https://www.gov.uk/government/publications/the-green-book-appraisal-and-evaluation-in-central-governent.
HM TREASURY (2015). The Aqua Book: Guidance On Producing Quality Analysis for Government. Accessible at: https://www.gov.uk/government/publications/the-aqua-book-guidance-on-producing-quality-analysis-for-government.
JAGER, J. (1998). Current thinking on using scientific findings in environmental policy making. Environmental Modeling and Assessment, 3, 143-153.
JOHNSTON, E. W., & Desouza, K. C. (Eds.). (2015). Governance in the Information Era: Theory and Practice of Policy informatics. London: Routledge. [doi:10.1023/A:1019066907165]
JOHNSON, P. G. (2015a). The SWAP model : policy and theory applications for agent-based modelling of soil and water conservation adoption. Doctoral thesis, University of Surrey. Accessible at: http://epubs.surrey.ac.uk/807166/.
JOHNSON, P. G. (2015b). Agent-based models as “interested amateurs”. Land. 4(2), 281-299. [doi:10.3390/land4020281]
KNIGHT, W. (2017). Forget killer robots—bias is the real AI danger. MIT Technology Review, 3 October 2017. Accessible at: https://www.technologyreview.com/s/608986/forget-killer-robotsbias-is-the-real-ai-danger/.
KOLKMAN, D. A., Campo, P., Balke-Visser, T., & Gilbert, N. (2016). How to build models for government: criteria driving model acceptance in policymaking. Policy Sciences, 49(4), 1–16. [doi:10.1007/s11077-016-9250-4]
KROHN, W. (2007). Realexperimente – Die Modernisierung der "offenen Gesellschaft" durch experimentelle Forschung. Erwägen – Wissen – Ethik EWE 18(3), 343-356.
LINDBLOM, C. E. (1959). The science of “muddling through”. Public Administration Review, 19(2), 79-88. [doi:10.2307/973677]
LINDBLOM, C. E. (1979). Still muddling, not yet through. Public Administration Review, 39(6), 517-526.
MARTIN, S., & Sanderson, I. (1999). Evaluating public policy experiments. Measuring outcomes, monitoring processes or managing pilots?. Evaluation, 5(3), 245-258. [doi:10.1177/13563899922208977]
MORAN, M. (2015). Politics and Governance in the UK. London: Palgrave Macmillan.
NARASIMHAN, K., Gilbert, N., Hope, A., & Roberts, T. (2017). Demystifying Energy Demand using a Practice-centric Agent-based Model. Working paper retrieved from: http://cress.soc.surrey.ac.uk/web/publications/working-papers.
RAENG (2015). A critical time for UK energy policy: What must be done now to deliver the UK’s future energy system. Royal Academy of Engineering. Accessible at: https://www.raeng.org.uk/publications/reports/a-critical-time-for-uk-energy-policy.
RECKWITZ, A. (2002). Toward a theory of social practices: A development in culturalist theorizing. European Journal of Social Theory, 5(2), 243–263.
RISK SOLUTIONS (2003). FMD CBA Phase 2 - Integrated findings, summary report. Accessible at: http://webarchive.nationalarchives.gov.uk/20100713185142/http:/www.defra.gov.uk/foodfarm/farmanimal/movements/costbenefit/documents/integrated_summary2.pdf.
RISK SOLUTIONS (2005). Cost benefit analysis of foot and mouth disease controls. Accessible at: http://www.elika.eus/datos/articulos/Archivo136/DEFRA_Faftosa.pdf.
RISK SOLUTIONS (2015). The impact of water abstraction reform - Final report, 2015. Accessible at: http://sciencesearch.defra.gov.uk/Document.aspx?Document=13715_WT1563_TheImpactofWaterAbstractionReform-FinalReport.pdf.
SEMINAR on Policy Pilots and Evaluation (2013). LSHTM London. Accessible at: http://piru.lshtm.ac.uk/assets/files/Policy%20Pilots%20report%20final%20version.pdf.
SAWYER, R. K. (2005). Social Emergence: Societies as Complex Systems. Cambridge, MA: Cambridge University Press.
STAR, S. and Griesemer, J. (1989). Institutional ecology, 'translations' and boundary objects: Amateurs and professionals in Berkeley's Museum of Vertebrate Zoology, 1907-39. Social Studies of Science, 19(3), 387–420. [doi:10.1177/030631289019003001]
TAYLOR, N. (2003). Review of the use of models in informing disease control policy development and adjustment. A report for DEFRA by Veterinary Epidemiology and Economics Research Unit. Accessible at: http://www.veeru.rdg.ac.uk/documents/UseofModelsinDiseaseControlPolicy.pdf.
TEISMAN, G., & Klijn, E.-H. (2008). Complexity theory and public management. Public Management Review, 10(3), 287–297. [doi:10.1080/14719030802002451]
UPRICHARD, E. and Penn, A. (2016). Dependency models. CECAN evaluation and policy practice note. Accessible at: https://www.cecan.ac.uk/resources.
VOINOV, A., & Bousquet, F. (2010). Modelling with stakeholders. Environmental Modelling & Software, 25(11), 1268–1281. [doi:10.1016/j.envsoft.2010.03.007]
WEIBLE, C.M. (2008). Expert-based information and policy subsystems: A review and synthesis. Policy Studies Journal, 36(4), 615-635.
WEISS, C. (1976). Policy research in university: Practical aid or academic exercise?. Policy Studies Journal, 4(3), 224-228. [doi:10.1111/j.1541-0072.1976.tb01557.x]
WEISS, C. (1979). The many meanings of research utilization. Public Administration Review, 39(5), 426-431.
WILENSKY, U. (1999). NetLogo; Center for Connected Learning and Computer-Based Modeling, Northwestern University: Evanston, IL, USA, 1999. Available online: http://ccl.northwestern.edu/netlogo.