Introduction
The solution of many problems related to the interaction of our societies with nature, such as climate change and loss of biodiversity requires deep structural changes in key areas of human activity, including our transport, energy, agriculture, and other systems (STRN 2010). The field of sustainability transitions research has set out to understand how such deep structural change happens and how it can be steered (Rip & Kemp 1998; Grin et al. 2010). Transition modelling is a growing research area within the broader field of sustainability transitions research. In this paper we define ‘transition models’ as the application of existing modelling methodologies to explain the dynamics of transitions. Transition models can be seen as a new application field for established modelling science for understanding the complex behaviours of societal system changes. A survey of peer-reviewed articles reveals a developing interest in this area, with 41 articles[1] published on transitions modelling up to 2016 compared to 15 articles up to 2012.
Holtz et al. (2015) discuss the possible uses and benefits of modelling in transitions research providing examples of modelling to develop scenarios for examining transition narratives and to explore transition dynamics. Papachristos (2014) argues that simulation modelling can study system interactions and support policymaking for transitions.
There are a few publications that have reviewed transitions models. Timmermans & de Haan (2008) found almost no modelling research and proposed some mathematical and computational approaches, including the importance of increasing returns to scale in economic representations. Holtz (2011) argued that transitions models need to address transitions in specific contexts to enable the development of strong microfoundations and empirical validation. Safarzynska et al. (2012) reviewed evolutionary approaches to modelling transitions and Zeppini et al. (2014) consider threshold models of transitions. Halbe et al. (2015) argue that Integrated Assessment Models, environmental modelling and socio-ecological modelling have similar characteristics to models in transitions research and develop a classification of uses of models from these fields in comparison to transitions models. Li et al. (2015) review existing socio-technical energy transitions models and the extent to which they include factors covered by transitions theory.
The objective of this paper is to clearly identify a niche for sustainability transition modelling in relation to the broader range of existing modelling approaches. It identifies some specific approaches which modellers could use to contribute to the field of sustainability transitions research. As such, this paper is complementary to the general discussion of the state of transitions modelling and its potential in Holtz et al. (2015). Of the many model uses in transitions research (Holtz et al. 2015), the models we will consider in particular are models for understanding the dynamics of transitions in socio-technical systems towards sustainability. We do not aim to identify an ‘ideal’ or ‘preferred’ approach, nor do we suggest excluding approaches to modelling transitions. A wide range of different kinds of models and model uses are also viable and useful in transitions research. The comparison of different classes of model provides researchers with a starting point for their choice of an approach to modelling transitions, in order to address the characteristics of the research question they face.
We look at modelling approaches in other fields to identify a portfolio of approaches we think will be useful for transitions modelling. Many approaches can potentially be used to model sustainability transitions, so it is not feasible to undertake a comprehensive review. Instead, we adopt the reverse approach and review key sustainability transition concepts and issues that sustainability transition models are intended to address.
Section 2 discusses the characteristics of sustainability transitions as identified in the sustainability transitions literature (Rip & Kemp 1998; Geels 2002; Geels & Schot 2007; Grin et al. 2010; STRN 2010) and the features in models that could represent these characteristics. Transitions are complex, multifaceted processes, thus we argue that there are several separate qualities that can contribute to making a model a ‘transitions model’. We distil a list of key features from a broadly acknowledged, empirical group of transition characteristics – though not all of them will be addressed by each model.
Section 3 then identifies some of the main strands in modelling socio-technological change and discusses the extent to which they address these characteristics. Examples of the models are provided. Modelling approaches can be grouped both in terms of the theoretical approach to social and technological change used and in terms of the simulation methodology. Environmental or eco-innovation has an extensive literature in economic modelling, especially in energy and climate change policy research using macroeconomic modelling approaches incorporating endogenous technical change and combined energy technology-energy economics models (Köhler et al. 2006; Popp et al. 2010; Li et al. 2015; Babatunde et al. 2017). Integrated Assessment Models (IAMs) of climate change combine energy technology and macroeconomic models with a representation of climate change (Clarke et al. 2014; Bruckner 2016).
Evolutionary economics (Nelson & Winter 1982; Beinhocker 2006) generalises economic analysis using the analogy of biology to consider economic dynamics through variation, selection and differential replication. These ideas have been applied to the simulation of microeconomic dynamics (Safarzynska et al. 2012; Zeppini & van den Bergh 2011). Beinhocker (2006) shows how evolutionary economics is a part of complexity science in general. Complexity science has also been applied in simulation models of innovation processes (Geroski 2000; Frenken 2006).
These literatures are limited in that they do not directly include ecosystem feedbacks in their analyses. This is the objective of the Socio-ecological systems (SES) literature (Halbe et al. 2015; Haberl et al. 2016). Modelling in this field was reviewed by Schlüter et al. (2012). While these frameworks provide a typology of theories that could be applied in modelling sustainability transitions, there are also two general simulation approaches that that can model and implement transitions. System Dynamics (SD) is a modelling approach that emphasises the generation of dynamics through feedbacks between system elements (Sterman 1994; Moallemi et al. 2017; Papachristos 2017; Walrave & Raven 2016). Computational social science agent-based modelling (CSS ABM) is a strand of research that uses simulation methods with large numbers of decision makers to analyse social systems from a bottom-up perspective (Gilbert & Troitzsch 2005; Heckbert et al. 2010; Holtz et al. 2015).
These four modelling frameworks and two simulation approaches are used to provide a typology of the modelling literatures that could address the features of sustainability transitions identified in Section 2. Actual simulation models necessarily combine a theory with a simulation approach such that there are many overlaps between these six categories. We discuss how the different approaches are interrelated.
We then summarise the capabilities of these six modelling literatures with respect to their ability to represent the critical features of sustainability transitions. We argue that the modelling approaches of which we are aware are limited in their ability to cover some of the critical features of transitions and identify a research need for a new class of models which addresses these gaps.
Specifying (Empirical) Characteristics of Transitions
We quote here from STRN (2010):
"The starting point for transitions research is a recognition that many environmental problems, such as climate change, loss of biodiversity, resource depletion (clean water, oil, forests, fish stocks), are formidable societal challenges, whose solution requires deep structural changes in key areas of human activity, including our transport, energy, agriculture, housing, manufacturing, leisure and other systems. Furthermore, we recognise that the crucial challenge for sustainable development is the fact that existing systems tend to be very difficult to ‘dislodge’ out of their current state, because they are stabilized by various processes that lead to path dependent developments and ‘entrapment’ lock in. A variety of highly institutionalised processes tend to perpetuate existing systems:In transitions research we call these mutually reinforcing processes a ‘socio-technical regime’.” (STRN 2010)
- the knowledge, capabilities and employment of various actors relevant to the maintenance of existing systems;
- the technical infrastructures and institutions (that have developed over time to service those systems);
- the economies of scale and markets of incumbent systems;
- the social significance of these systems, and their links to political power;
- the mutually reliant clusters of technologies used by these systems; and,
- the everyday practices and lifestyle values that have come to rely on these systems.
These features mean that transitions involve what the transitions literature calls socio-technological systems. Following the neo-Schumpeterian ideas of radical technological change leading to Kondratiev or Long Waves of growth (Köhler 2012), such systems involve co-evolution between the political, scientific, economic, technology and culture sub-systems (Freeman & Louçã 2001). The consequence of this is that such socio-technical systems need to be understood as non-linear, complex systems.
From this empirically based observation, which emphasises transitions to sustainability and therefore the link between nature and society, we draw on the work of Halbe et al. (2015), Geels & Schot (2007), Rotmans et al. (2001), Smith et al. (2005; 2010, Coenen et al. (2012) and Shove & Walker (2010) in order to provide a more complete list of characteristics. Socio-technical transitions:
- Profoundly alter the way a societal system[2] functions and the actors, practices, institutions and technologies involved in production and consumption. During a transition, new products, services, business models, regulations, norms, organizations and infrastructures may emerge, complementing and/or substituting existing ones. Transitions can be differentiated from other kinds of social or technical change because they address a system change which alters the ways a socio-technical system functions. They involve a change from one socio-technical regime to another in which the new is simultaneously constituted as the old unravels;
- Have dynamics that typically start slowly due to multiple sources of inertia (STRN 2010) in the old regime (although various transition patterns have been distinguished in the literature);
- Are polycentric processes of societal system change: multiple actors, multiple factors, multiple temporal and spatial scales are relevant for shaping transition dynamics. They can hence be initiated and driven from various directions (behaviour/social practice and expectations, cultural changes, technology and economy trends, institutional change, environmental changes, policy) and from various levels (e.g. citizen-initiatives or EU policy);
- May be triggered purposively or emerge from ongoing developments;
- Are open, path dependent processes with uncertain outcomes. The dynamics are not only determined by external developments and conditions (“landscape developments”) but also emerge endogenously from interactions within the system. The nature, timing and intensity of interactions are crucial for the unfolding dynamics.
From transitions characteristics to model features
The essential features of transitions identified above imply some key modelling features for ‘transitions models’ for the purposes of this review:
- Capability of representing non-linear behaviour
Transitions occur over periods of time in which change is happening faster or slower. An archetypical pattern is that of an S-curve, in which the rate of change is initially slow, then accelerates, and slows down again as the new regime configuration stabilizes. A transitions model should be able to reproduce such variations of the rate of change and other dynamics through which the end-state of the transition is not proportional to changes in the initial state. A special class of non-linear behaviour that is particularly pertinent to transitions is path-dependence. - Capability of representing qualitatively different system states
A transition implies that the configuration of elements fulfilling a particular societal function changes i.e. new elements are included, old ones are dropped, elements might adapt, and the interactions between elements are reconfigured. Therefore, a transition is not just change towards more or less of the same. This aspect of a transition should be (explicitly or implicitly) captured by transitions models. - Capability of representing changes in social values and norms
Transitions to sustainability also involve changes in the value system of society and actors. This will lead to changes in the decision making rules (preferences, in economics terminology). Models should be capable of representing changes in the decision making structure. - Capability of representing diversity and heterogeneity
Transitions involve diverse actor groups (producers, consumers, politicians, NGOs etc.) and actors within these groups are heterogeneous (e.g. producers following different strategies, consumers having different preferences). These differences should be represented in the structure of a transitions model. - Capability of representing dynamics at and across different scales
There is also a consensus in the literature that transitions bridge different scales. As is acknowledged by Geels & Schot (2010, in Grin et al. 2010) among others, this can be seen as an application of Giddens’ Theory of Structuration (1984), in which agents act within a set of social structures. Their actions can change these structures, such that there are potentially feedbacks between the micro and macro levels in societal systems. The different scales can be spatial (e.g. local, regional, global), temporal (e.g. years, decades, centuries), functional (e.g. in societal sub-systems – economy, policy, science, education, etc.), epistemological (e.g. microeconomic vs. macroeconomic) or institutional (e.g. in the legal context constitutions, laws and directives) scales. In the multi-level perspective (Grin et al. 2010) niches can be considered as a micro-level phenomenon, while regimes are 'meso' to macro level and the landscape is macro-level. For example, an energy transition is influenced by global climate agreements, by national or federal regulations, as well as local initiatives that become engaged in energy production and become new players in the game. - Capability of incorporating open processes and uncertainties or contingencies
Transitions are influenced by unpredictable events that by their very nature cannot be predicted, such as the development of radical innovations and political decisions. If the system is responsive to these events (or not), the future transition dynamics might change direction (or not).
Current Modelling Literatures which Address the Characteristics of Transitions
In this section we review some of the main relevant strands in modelling of long-term transformational changes with regards to their ability to address the characteristics of sustainability transitions we have identified in the previous section.
Eco-innovation in energy-economy models and IAMs
Transitions research can be seen as a subset of innovation research. Modelling of innovation processes is a very extensive literature, but is mainly based on management and/or economics methods (Fagerberg et al. 2006). In eco-innovation, economics models of energy systems (so-called energy-economy models) are categorised into three types (Köhler et al. 2006, Popp et al. 2010; Li et al. 2015). Bottom-up sectoral models include energy demand and technologies (MARKAL: Loulou et al. 2004; the TIMES/TIAM family of energy system and Integrated Assessment Models: ETSAP 2017a; Li et al. 2015; MESSAGE: Messner and Strubegger 1995). Top-down models have a macroeconomic structure including energy demand (MERGE: Manne et al. 1995). Hybrid models combine a macroeconomic structure with extra detail for the energy sector (REMIND-R: Leimbach et al. 2010, E3MG: Köhler et al. 2006). This literature has included endogenous growth with increasing returns to scale through knowledge and learning curves.
The broadest modelling approach in these literatures is Integrated Assessment Modelling (IAMs), which couples economics and technology representations to environmental emissions. Feedbacks of environmental changes into economies and technologies are limited. Examples are IMAGE (Stehfest et al. 2014), TIAM (ETSAP 2017b).
These models are programmed in general purpose software such as C++ or economic modelling software, especially GAMS (e.g. in the TIMES and TIAM model family).
Links to other model categories
Most of these models are founded on economic theory with cost minimisation of a technology mix to achieve required climate mitigation goals. They are linked to the other theories in the sense that they all include costs or prices in the simulation. However, the representation of decision making and the resulting dynamics is different to the other groups of theories. These models do not use system dynamics (SD) or agent based model (ABM) methods.
Capability of representing non-linear behaviour
Endogenous growth models and learning curve models explicitly include non-linearities in their mathematical formulation. In this sense, they are non-linear models. However, for optimal growth models, the possible solutions identified by the model are constrained through the dynamic optimization procedure. In this sense, they cannot have the same flexibility of outcomes as e.g. the evolutionary economics models discussed below. They are used to answer questions such as: which is the cost minimizing technological pathway to achieve a given environmental objective?
Capability of representing qualitatively different system states
The ability of these methods to represent qualitatively different states is limited, because they rely on historical data, mainly generated by the current socio-technical structures and regime. If alternative technologies are represented, as in many energy economics models for example, a transition to a different set of technologies can be modelled. They will often require the input of exogenous changes e.g. in market structure of an electricity supply network to allow for large scale household power generation through decentralised renewable or demand management.
Capability of representing changes in social values and norms
Eco-innovation models are not very suitable for addressing cultural shifts and changes in the preference structures of decision making agents. They can allow for changes in preferences through exogenous scenario inputs (e.g. van Sluisveld et al. 2016). The limitation here is that changes in culture or institutions, such as the development of an environmental movement supporting stronger environmental policies in response to perceived climate change can only be addressed by exogenous assumptions.
Capability of representing diversity and heterogeneity
These modelling approaches do not usually include heterogeneity explicitly in the decision making agents. Diversity across economic sectors and countries is included in large scale models, but diverse actor groups are usually limited to aggregated producers, consumers and a social decision maker in some of the macroeconomics based models.
Capability of representing dynamics at and across different scales:
These modelling approaches can be used across scales, both by aggregating results e.g. in climate change assessment and by disaggregating results e.g. from global greenhouse gas emissions scenarios to sectoral or regional economic activity. Some of these models, in particular the Integrated Assessment Models, include both macro- and microeconomic and technology features in a single model (Köhler et al. 2006).
Capability of incorporating uncertainties or contingencies
The different modelling approaches outlined here differ considerably in how they address uncertainties. The larger scale energy-economy models and IAMs may include statistical estimations of their parameters. However, the analysis of uncertainties in these models is limited. They address uncertainty through scenario analysis. The eco-innovation literature also uses scenario analysis to allow for contingent futures.
These economic eco-innovation models have adopted non-linear features, but have closely constrained economic structures and are therefore limited in their ability to represent the behavioural changes and resultant qualitative system changes associated with socio-technical system change. They can be used to simulate paths of technology outcomes that represent large scale change, as with the IAMs used for climate mitigation scenario development.
Evolutionary economics
Evolutionary modelling in economic and technology innovation literatures represents processes of change and competition in a population of decision makers or agents using three core concepts from evolutionary biology (Safarzynska et al. 2012): variation, selection and differential replication. Populations of agents in evolutionary models interact and reproduce, and the “genome” of individual agents changes through mutation or recombination. This alters agents' fitness to their environment with the result being success or failure to pass their “genome” to future agent generations.
Evolutionary theories and models have been applied in innovation and complex adaptive systems research (Safarzynska et al. 2012). In socio-technical transition studies, innovation leads to variation in supply and demand practices, technology competition and institutional changes. In evolutionary economics, heterogeneity varies along three dimensions: variety, balance, and disparity (2010). Selection acts to reduce the heterogeneity that variation generates. This approach has been applied in energy policy and sustainability innovations research (Stirling 2010). Additional concepts applied in evolutionary economics research include: bounded rationality, path dependence and lock-in, group selection and co-evolutionary dynamics (van den Bergh & Gowdy 2009; Gazheli et al. 2015).
It follows that evolutionary thinking can contribute to studying a wide variety of changes in consumer preferences, social structure and institutions, in addition to technology innovation. Evolutionary simulation models of demand and supply dynamics have already been developed and the insights produced can be applied or are directly relevant to transitions. Some of the most prominent models in the literature are e.g.: Silverberg et al. 1988; Windrum & Birchenhall 1998, 2005; Janssen & Jager 2002; Oltra & Saint-Jean 2005; Windrum et al. 2009; Malerba et al. 1999, 2001, 2008; Safarzynska & van den Bergh 2010 and Valente 2012. Some of the models are more accurately described as co-evolutionary as they incorporate two or more populations (supply, demand) that are linked together, with each one influencing the evolutionary trajectory of the other. Windrum & Birchenhall (1998) developed such a model, with firms innovating to attract consumers. Different firms sell products with unique characteristics to consumers. The consumers are distributed over a set of classes with differing preferences. Firms innovate to change their product characteristics and consumers can change the product they choose, depending on their preferences that change over time. Windrum et al. (2009) developed this framework to include the pollution characteristics of the competing firms and products. These models use general simulation software e.g. Mathematica.
Links to other model categories
Models using an evolutionary economics approach to innovation are based on economic decision making and as such extend the economic models discussed above. Beinhocker (2006) reviews the literature applying complexity science to economics and shows that models applying evolutionary structures to innovation can be considered as complexity models. Therefore, they are a sub-set of complexity models discussed below. We treat them separately here because the explicit application of the principles of evolution to simulation models of innovation is an established literature.
Capability of representing non-linear behaviour
Evolutionary models have been used to reproduce non-linear patterns on the firm level but also on a more aggregate industry level. An early model was developed to look at organizational adaptation of search strategies, competences and aspirations in a dynamic environment (Levinthal & Marc 1981). Another classic illustration of non-linear behaviour is diffusion processes in an evolutionary environment with technological and behavioural agent heterogeneity and learning processes (Silverberg et al. 1988).
Capability of representing qualitatively different system states
Evolutionary models of demand-supply co-evolution have been used to see whether market competition can reach a locked-in state of a single dominant firm-product combination (Windrum & Birchenhall 1998). More recently models have been developed to explore policies to move the market out of a state of lock-in and towards less carbon intensive trajectories (Safarzynska & van den Bergh 2010).
Capability of representing changes in social values and norms
Evolutionary models are able to represent emergent norms values and preferences of individual agents, or even emergent normative change at the population level through changes in the agent "genome". For example, they can represent how the agent acting as a consumer derives utility not only from the intrinsic value of a good, but also from its social embeddedness.
Capability of representing diversity and heterogeneity
Heterogeneity is an inherent aspect of the evolutionary approach to modelling and heterogeneity is therefore included in all these models. The representation of diverse actor groups is also a feature of the models of e.g. Silverberg et al. (1988) and Windrum & Birchenhall (1998).
Capability of representing dynamics at and across different scales
Evolutionary thinking has been applied at different spatial and organizational/institutional levels. For example, the model of Levinthal & March (1981) is at the organization level while the models of Windrum & Birchenhall (1998), Windrum et al. (2009), Safarzynska & van den Bergh (2010) are at the level of firm populations. This class of models concentrates on macro phenomena as emerging as patterns from micro-level interactions, rather than explicit representation of macro-level structures.
Capability to incorporate uncertainties or contingencies
Models do incorporate uncertainties both in the stochasticity of change and adaptation of a single organization to its environment (Levinthal & March 1981) and at the level of uncertainties impacting a population of organizations.
Evolutionary modelling is a very flexible approach and can (in principle) incorporate all the features of transitions models identified in Section 2. However, applications so far have concentrated on the microeconomic level. There are few examples of evolutionary models of macroeconomic processes, or of the behaviour of socio-technical regimes and their organisational complexity. Therefore, their application to normative change has so far been limited.
Complex systems models
Under this category we include frameworks and themes such as ‘complex, adaptive systems’ (e.g. Holland 1992), ‘self-organisation’ (e.g. Kaufmann 1993), ‘self-organised criticality’ (e.g. Bak 1996) and a variety of others. These approaches are advocated and were pioneered by the Santa Fe Institute. The sciences of complexity are not a theoretically unified field, rather they are a portfolio of approaches to understand a class of phenomena – referred to as ‘complex’. They draw on various – though typically exact, or mathematical – fields of research, such as statistical and non-linear physics, theoretical biology and computer science.
Transitions are usually considered to be an example of complex phenomena and it is therefore no surprise to find key terms such as non-linearity or (co-)evolution to be common in both fields. Transition Management in particular has adopted this perspective and some of the concepts of complexity (see e.g. Rotmans & Loorbach 2009), though not in the formal mathematical or modelling manifestations. Given this kinship one would perhaps expect a blooming field at the interface of transitions and complexity studies exchanging methods, concepts and cases but in practice the overlap seems limited in the modelling arena. Exceptions are the complexity modelling studies on innovation by means of diffusion, network and percolation models. Frenken (2006) provides an overview of some of these under three headings: NK models, complex network models, and percolation models.
NK models were introduced by Kaufmann (1993) as generalised models of genetic evolution. In an NK model, each individual in the set of N elements interacts with K other elements within a fitness landscape. As such, they can potentially be applied to a large class of phenomena including many that are relevant to transitions research. Unlike the evolutionary economics models summarised above, economic variables do not necessarily explicitly determine the interactions. Instead, they emphasise search strategies over an abstract fitness space. One such application can be found in Alkemade et al. (2009). In this model, a single agent searches over a fitness landscape of different design configurations. Alternatively, a decentralised search where only one element changes at a time can be simulated. Local search generates local optima as Nash equilibria (Zeppini et al 2014).
Complex network models make extensive use of graph-theoretical representations. The nodes in these networks could be agents such as individuals or firms, but also technologies (Frenken 2006, p.144). Network measures such as cliquishness and average path length are used to study how easily knowledge is created and exchanged for example (Frenken 2006, p.146).
Percolation models are a class of models adopted from physics models of diffusion of a liquid through a porous medium. The models simulate the adoption of new technologies by neighbouring elements, with adoption dependent on a willingness to pay parameter. Interesting phenomena in these models are phase transitions which represent abrupt shifts from one qualitatively distinct state to another, for example from limited adoption to mass adoption of a technology. Examples of these models can be found in Zeppini et al. (2014) and de Haan (2008).
We can extend the categories suggested by Frenken (2006) to also include diffusion models. These models can be used to see how ‘something’ (e.g. knowledge, use of a technology) spreads through a population of agents or across a spatial region. Geroski (2000) reviews models for S-curves of innovation diffusion, including epidemic models, probit models, density dependence models and finally the evolutionary models of competition between variants of a technology as developed by e.g. Silverberg et al. (1988).
As with the evolutionary economics models, these models use general simulations software e.g. Mathematica, MATLAB, etc.
Links to other model categories
As the Sciences of Complexity are such a conceptually and methodologically eclectic field, there are several overlaps with some of the other literatures discussed in this part of the article. To give a – non-exhaustive – illustration: there is considerable overlap with eco-innovation models in modelling diffusion of innovation. As discussed above, there is overlap in the use of evolutionary principles. Agent-Based Modelling is an accepted approach to model complex systems, and so is System Dynamics. Non-linear population dynamics are interests shared by complexity researchers and socio-ecological modellers alike as discussed in 3.61 - 3.70 below.
Capability of representing non-linear behaviour
All complexity phenomena share this aspect and consequently the approaches used by the sciences of complexity typically feature this aspect very prominently. Path dependency is also a key aspect. Some complexity models feature hysteresis, which can be considered a form of path dependency.
Capability of representing qualitatively different system states
Phase transitions – which per definition lead to qualitatively different states – are a central concern in complexity models. Complex, adaptive systems models may evolve to qualitatively different states.
Capability of representing changes in social values and norms
In principle, the normative aspects of transitions can be addressed methodologically with complexity models just as easily as the agent-based models of computational social science. However, in practice this aspect is usually not explored much.
Capability of representing diversity and heterogeneity
The situation here is similar to that for the normative aspects. Though this is theoretically a core component of what makes systems complex and adaptive, this is not necessarily strongly reflected in the models employed.
Capability of representing dynamics at and across different scales
The ability to represent different scales is in some sense a matter of implementation, a methodological choice rather than a capacity complexity models inherently do or do not have. However, some models of non-linear dynamics, particularly those concerned with pattern formation (see e.g. van Saarloos 2003), feature emergent scales or allow these to be studied. Emergent scales are scales that do not feature explicitly in the equations that govern the model but that appear ‘spontaneously’. A well-known example is ripples on a sandy beach that feature wavelengths seemingly unrelated to those of the water waves or the size of the grains of sand.
Capability to incorporate uncertainties or contingencies
This is a common feature of complexity models, often even endogenously represented. The non-linearity of the models entails an intrinsic degree of unpredictability. Stochasticity of the models can be used to represent contingency.
In summary, this is in principle a broad class of highly non-linear models. Their application to normative change and diverse actor groups is limited in the current literature.
Computational social science: Agent-based models
A strand of research within Computational Social Science (CSS) uses Agent-Based Modelling (ABM) to analyse behaviour of complex social systems from a bottom-up perspective (Gilbert & Troitzsch 2005; Heckbert et al. 2010; Hedstrom 2005). We discuss the practical ability in general of ABMs to meet the transition characteristics, referring to two models where appropriate: the classic Sugar Scape model (Epstein & Axtell 1996) and the MATISSE ABM of transitions (Bergman et al. 2008; Köhler et al. 2009). The model core consists of the agents that represent interacting decision makers. Using ABM allows for the generation of emergent phenomena on the level of a group, organisation or other collection of actors – be they spatial or temporal patterns or characteristic statistical distributions of variables of interest. As such, ABM is one method of implementing complex systems. The representation of multiple agents enables actors to have differing behaviours or strategies. In the context of sustainability transitions analysis, this enables differentiation between regime(s) and niches, as well as distributions of choices of different firms or consumer/household decision makers.
The Sugarscape model (Epstein & Axtel 1996) used agents and their local interactions with each other in finding a resource necessary for survival (sugar) to demonstrate some of the fundamental properties (or stylised facts) of real economies and markets. The Santa-Fe institute used an ABM to analyse stock-market behaviour (Arthur et al. 1996; Ehrentreich 2008). Various ABMs have been developed to simulate eco-innovation (Schwoon 2006; Chappin & Dijkema 2010). Percolation models of technology adoption are discussed in Section 3.28; they are one approach using an Agent-Based structure to simulate innovation processes.
Chappin & Dijkema (2010) and Chappin (2011) use ABMs to study transitions in energy systems. Schwoon (2006) used an ABM to look at fuel cell vehicle adoption. Van der Vooren & Alkemade (2012) develop an ABM to analyse the competition between new technologies and an incumbent technology in low carbon vehicles.
The MATISSE model of transitions to low carbon mobility (Bergman et al. 2008; Köhler et al. 2009) uses an ABM to represent niche and regime actors as well as the mobility lifestyle decision of households. In this model, households decide upon their mobility behaviour through a choice of the regime mobility lifestyle or one of the niches. The choice depends on the similarity of the regime/niche characteristics (e.g. environmental performance, cost, preference for private transport, preference for short distance trips) to the preferences of the household. The regime and niches innovate in accordance with different strategies to maintain support from households (regime) or increase their influence (niches) allowing for changing households' preferences.
ABMs use a range of software applications. The MATISSE model is written using Repast Simphony. AnyLogic and Netlogo are commonly used ABM applications.
Links to other model categories
Computational social science starts from the understanding that social systems are a class of complex systems. Therefore, CSS models are a sub-set of the complex system models discussed above. Because evolutionary economics models also study interactions between actors with varying individual properties or strategies, they can also be considered as agent-based models. CSS ABMs can be seen as emphasising large scale societal phenomena, while evolutionary models have tended to concentrate on micro-level processes of innovation. The overlap is however extensive. The socio-ecological models discussed below are a particular class of CSS models that represent ecological systems in addition to social systems. The overlap is emphasised by the use of agent-based approaches in the socio-ecological models.
System dynamics modelling (see below) can be regarded as a complementary approach to ABMs. Models such as the MATISSE transitions model combine systems dynamics and ABM methods (Köhler et al. 2009).
Capability of representing non-linear behaviour
Agent-based models typically start with an initial state that is out of equilibrium and during the simulation the mechanisms in the model generate emergent system-level behaviour. Exogenous drivers that affect these mechanisms are often important: they typically drive changes in agent decisions that again lead to evolving system-level behaviour over simulated time. The observed behaviour is typically non-linear and the process of pattern formation is modelled endogenously. Different attractors in the simulated system may represent stable regimes. What ABMs typically do not model is how existing patterns disintegrate. Therefore, modelling the decomposition of an initially stable regime would be an interesting extension of current CSS ABM modelling.
Capability of representing qualitatively different system states
Some CSS ABMs are not only able to represent different system states but can also endogenously generate qualitatively different emergent phenomena from the same underlying mechanisms, when some parameter values are changed, or under the different sets of random numbers. This is a core strength of ABMs. For instance, in the MATISSE model, various qualitatively different types of system behaviour have been demonstrated. One possibility is a steady, but relatively smooth transition away from conventional transport technologies to a new dominant technology e.g. hydrogen (Köhler et al. 2009). Another possibility is a complex set of waves of support for different technologies, with no single technology dominating over the 50 years simulation period. The purpose of such modelling is to connect sets of assumptions with their resulting sets of system-level behaviours. Furthermore, in ABMs, actors are often assumed to learn, and/or actors with particular characteristics are selected based on their performance in some evolutionary process and this causes non-linear behaviour and possibly different system states. In the Sugar Scape model, for instance, the agent population evolves because agents are selected with a high ability to spot resources and low specific consumption. Such learning and evolution, however, typically does not imply the introduction of completely new actors or elements in the model, but rather a variation and selection of characteristics of existing ones.
Capability of representing changes in social values and norms
ABMs are able to represent social interactions among agents as well as learning and cognition to high degrees of complexity and sophistication. They are, therefore, able to represent changes in norms, values and preferences of agents, including emergent normative change on the group or societal level (e.g. Conte et al. 2013). However, highly sophisticated representations of cognition and emergent norms come at the price of high model complexity and epistemic uncertainty and therefore most CSS ABMs choose simpler representations.
Capability of representing diversity and heterogeneity
Incorporating actor heterogeneity is seen as a major strength of ABMs and is one of the key reasons to adopt this approach in computational social sciences. ABMs usually include heterogeneity in values of agent’s attributes. Agents can also have diverse behavioural rules, that correspond sometimes to different roles (e.g. producers and consumers or mobility services and households in the MATISSE model).
Capability of representing dynamics at and across different scales
CSS ABMs often represent processes on different scales that interact in the model. For some experiments conducted with the Sugar Scape model, the artificial landscape is divided into two areas which have alternating seasons. This introduces a spatial sub-scale into the model, as well as the temporal scale of seasonal change which complements faster agent behaviour of moving and harvesting, and slower time scales of agents’ reproduction (births and deaths).
Capability to incorporate uncertainties or contingencies
CSS ABMs involve multiple feedbacks between the model elements, facilitating the representation of contingencies and uncertainties, for instance in the order in which agents make their decisions. Uncertainties can be captured as exogenous variables that affect individual decisions.
ABMs are in principle able to represent all transition characteristics identified above, because they can represent any target system and any process operating it at the intended level of detail. Individual models exist that meet single characteristics to a very high degree. Practical limitations of the approach arise from the conceptual and theoretical basis for representing micro-level social science phenomena, from the availability of data for calibration and validation of models, as well as from model complexity that becomes increasingly unmanageable if ever more model aspects are represented in a highly sophisticated way (Sun et al. 2016; Schulze et al. 2017). Therefore, existing CSS ABMs typically meet only a few of the model feature criteria.
System Dynamics models
System Dynamics (SD) is an approach to model, simulate and enhance learning of complex systems (Sterman 1994). System dynamics models provide an endogenous view on how the dynamic behaviour of a system unfolds, generated solely from system element interactions taking place within the system boundary (Richardson 2011). SD models are developed based on causal relations between stock and flow variables and constant parameters (Sterman 2000). The causal relations can form feedback loops that reinforce a certain system behaviour (reinforcing loops), or balance it and direct system towards stability (balancing loops). Several studies have used the SD approach to model transition processes (Li et al. 2015; Moallemi et al. 2016; Papachristos 2011, 2017; Struben & Sterman 2008; Walrave & Raven 2016; Yücel & Chiong Meza 2008). The extent to which SD models satisfy the characteristics identified depends on their underpinning theoretical framework, the way that the model is formulated and their connections to other modelling approaches. These models use several well-established software applications for SD modelling including VENSIM, STELLA, Anylogic and POWERSIM (Systemdynamics 2017). In the following, the strengths and limitations of the SD approach in each characteristic is discussed with a couple of modelling examples.
Links to other model categories
SD as a general modelling approach can be applied to many different types of problems. SD models of eco-innovation such as the ASTRA model (Astra 2017) use the economic theory of the eco-innovation models. The representation of dynamics through feedback between system elements means that SD can be used to simulate complex systems including evolutionary economics structures. The emphasis on the particular properties of feedbacks between nodes of the system is different to the CSS ABMs, where the feedbacks between agents tend to be similar and the emphasis is more on the variation in behaviours between the agents.
Capability of representing non-linear behaviour
SD models describe the dynamic interactions between multiple system components, that form reinforcing or balancing feedback loops. They can produce non-linear behaviours between the initial and end states. This chain of feedback loops can generate threshold effects and time-delayed behaviours. It can also result in accumulative but profound changes in the functioning of systems. Sterman (1982) has represented this characteristic by describing the non-linear dynamics of a drastic shift in the energy system from conventional to unconventional sources. This shift was represented by the non-linear behaviours in the price, production and consumption of energy along with their non-linear impacts on the standard of living, inflation and economic growth. The model structure differentiated between intermediate and long-term non-linear effects of energy depletion on the economy through feedback loops with the effect of several physical and information delays. It was also able to reproduce the different paces of the transition, i.e. whether it happens gradually (a smooth change) or suddenly (a crisis).
Capability of representing qualitatively different system states
Depending on the chosen time horizon in simulation, SD models can represent different states of a system quantitatively with stock variables. Their quantitative values change through the accumulation of flow variables which are the results of interactions between several internal and external variables. They can be interpreted in different qualitative states of system. As an example, Sterman (1982) considered energy transition as a long-term process of transformation in an intergenerational timescale. The model was then able to capture possible pathways and journeys from 1950 to 2050. It also represented changes between qualitatively different states in energy production, energy import, etc. In another example, Walrave & Raven (2016) used a transition-based SD model to reproduce different transition pathways, e.g. de-alignment and re-alignment pathway and technological substitution pathways, with different qualitative end-states. Moallemi et al. (2017) developed a SD transitions model to simulate the qualitative transformation of Indian electricity sector, as a sustainability transition, over 25 years period from 1990 to 2015.
Capability of representing changes in social values and norms
The SD approach can include normative changes as one of the drivers of transition dynamics in models. Yücel & Chiong Meza (2008) used an SD approach to model changes in actors’ preference in actor decisions about different options. They refer to these changes as ‘mechanisms related to actors’ behavioural identity’. Generally, the use of participatory modelling (Vennix 1996) can facilitate the integration of social norms and values on the direction and desire for a direction of change in change processes as a part of the process of model development (see Moallemi & Malekpour (2017) for the integration of a participatory approach in a SD transitions model). However, most SD modelling applications take an aggregated view of the system components and do not deal with actor-level changes in norms and values.
Capability of representing diversity and heterogeneity
SD models can represent diversity in the sense that they include different internal and external components. For example, Sterman (1982) modelled the dynamics of energy-economy system based on the interactions of its multiple aspects including production (e.g. goods, capital and energy production), households (e.g. labour and the consumer of the goods), financial (e.g. interest rates and inflation), government (e.g. monetary policies, taxes and energy policies) and OPEC (e.g. imports, energy price). Various sources of energy and types of goods were also taken into account. Nevertheless, SD models do not fully regard actor heterogeneity in terms of variations in consumer and producer’s behaviour. This can be explained by the aggregated view of SD approach in modelling of system interactions. The capability of system dynamics models to incorporate diversity and heterogeneity effectively and its implications for analysis purposes has been explored in Rahmandad and Sterman (2008).
Capability of representing dynamics at and across different scales
The SD approach can model changes at different rates (i.e. timescales). These models are also capable of representing dynamics across different spatial and organisational scales although in most cases they choose to concentrate on a specific scale (e.g. national, sectoral or organisational scale) for the sake of having a well-defined boundary. An example of a spatially multi-scale model can be found in Sterman (1982), where the decision making process was modelled based on the economic behaviours of actors at firm and individual levels. The model also explained changes in price of energy, amount of import/exports, etc. as the global-scale driving forces for energy transition. A more recent example explores the geopolitical consequences of shale gas, using two interconnected models to account for national and international dynamics (Auping et al. 2016).
Capability of incorporating uncertainties or contingencies
SD models represent changes through stock accumulation processes that create path-dependent system behaviours. It can include contingencies as external variables, imposing a sudden change on the endogenously driven dynamics of the system. The SD approach, in its classical form, deals with uncertainties to a limited extent by conducting sensitivity analysis tests for uncertain parameters (see Steel 2008; Sterman 1982) for some examples). However, it can be linked to other approaches, such as exploratory modelling, to fully address deep uncertainty conditions. In this case, SD model can incorporate every possible variation in input parameters as well as in model structures and can generate an ensemble of plausible future scenarios. Kwakkel & Pruyt (2013), Kwakkel et al. (2013) and Moallemi et al. (2017a) have discussed the use of exploratory modelling with SD for dealing with deep uncertainty.
In summary, SD is a very adaptable modelling approach. It is non-linear and has been used to model systems across scales to a limited extent. The treatment of changes in norms and behavioural diversity is usually limited, as are applications to modelling qualitative changes in systems.
Socio-Ecological Systems (SES) modelling
SES modelling has the objective of modelling interlinked dynamics of social and ecological systems. It has been developed from natural resources modelling literatures in ecology, economics and conservation. Examples of SES models are Christensen et al. (2011), which models fishing costs and benefits or Fletcher & Hilbert (2007) who analyse path dependency and resilience in land use systems. Becu et al. (2003) used an agent based model to consider water management. Schlüter et al. (2012) reviewed this emerging field and conclude that it does not have a unified analytical or methodological framework, but combines approaches and insights from different fields in a similar way to transitions modelling. SES are addressed as complex, co-evolutionary adaptive systems. Schlüter et al. (2012) identify issues of the inherent uncertainty and the emergence of macroscale patterns from microscale drivers of human behaviour as major research themes in the field. These lead to the consideration of resilience of SES and strategies for resilience in natural resource management. Halbe et al. (2015) consider that the similarities between social-ecological modelling and transition modelling are strong with regards to multi-domain and multi-level interactions, path-dependency and involvement of multiple actors. Other characteristics of transitions are also shared, but to a lower extent.
The Vienna School of Social Ecology (Haberl et al. 2016) is an example of this socio-ecological modelling approach. It tries to address the interplay between decisions taken by different types of actors, socioeconomic processes and political and institutional framework conditions on the one hand and essential ecological patterns and processes on the other. The aim is to apply those models to structure interdisciplinary communication processes by making variables and their interaction explicit. Researchers use participative model development, where models are created together with stakeholders, and work on different spatial and temporal scales, ranging from local studies to global changes in the diet system, land use and biomass flows. In one project they used an integrated socio-ecological model SERD (Simulation of Ecological Compatibility of Regional Development). The model includes an agent-based actor module coupled with a spatially explicit land use module and a biophysical stock-flow module capable of simulating socio-ecological material flows (Gaube & Haberl 2013). This group of models applies some of the approaches discussed above to society-nature interaction, an aspect which is missing in most models of innovation. This model used the AnyLogic simulation software combined with a GIS mapping system.
Links to other model categories
The combined environmental and socio-economic analyses of this approach mean that they can be considered as one form of IAM (Integrated Assessment Model), although the model structure is different to the climate policy IAMs such as IMAGE noted (see eco-innovation models). The use of agent based modelling in e.g. the SERD model mean that they are within the class of CSS ABMs and are models of complex systems as discussed above. They are identified as a separate class of model because they place an emphasis on the coupling of ecological and social processes which is not the case in most complexity models of innovation or in the CSS ABMs.
Capability of representing non-linear behaviour
This approach models the dynamic feedbacks between interconnected social and natural resource systems and therefore can represent and facilitate research on the non-linear behaviour of these systems.
Capability of representing qualitatively different system states
The SES models have the objective of modelling coupled ecological and social system dynamics. The SERD model (Gaube & Haberl 2013) illustrates the use of these models to simulate coevolution across social and ecological systems. They can therefore generate scenarios of system state changes, as emergent macroscale patterns.
Capability of representing changes in social values and norms
The use of participatory modelling also facilitates the integration of social norms and values on the direction and desire for a direction of change in change processes. In this way, norms and values can be incorporated in the model as a part of the process of model development.
Capability of representing diversity and heterogeneity
The inclusion of different types of actors in these models enables the representation of diversity and heterogeneity.
Capability of representing dynamics at and across different scales
This modelling literature has an explicit focus on the dynamic interaction between microscale drivers and emergent macroscale patterns and therefore does consider dynamics across different scales.
Capability to incorporate uncertainties or contingencies
The simulation of these models with multiple interactions between agents and ecological systems involves the representation of future contingencies. Analysis of uncertainties is seen by Schlüter et al. (2012) as a continuing challenge for this field.
Because SES models have adopted the modelling approaches of complex systems and CSS ABMs, they have the same potential advantages of being in principle able to represent all the features of transitions identified above. The main challenge for SES models is common with other IAM approaches: the very wide scope of linking ecosystems to socio-technical systems easily leads to very complex models.
Discussion
The ability of the different kinds of models reviewed to address the characteristics of transition models identified in Section 2 is summarised by the diagrams in Figure 1. In order to provide an overview and some comparability between model approaches, we have provided in these diagrams a quasi-quantitative scoring of each model approach with respect to each transition characteristic, based on the discussion in Section 3 and our background knowledge. Figure 1 represents the current practice in the modelling strands discussed.
The comparison of the approaches and their characteristics shows that they have different strengths and weaknesses in terms of the transition characteristics. The energy system models (Li et al. 2015; ETSAP 2017a) and climate policy IAMs (Stehfest et al. 2014; ETSAP 2017b) provide quantitative forecasts of energy markets and emissions with an emphasis on market structures. They are extensively used for policy support. The three classes of general complexity complexity models: ecological economics (Silverberg et al. 1988; Windrum et al. 2009; Safarzynska & van den Bergh 2010), complex systems (Frenken 2006; Alkemade et al. 2009; Zeppini et al. 2014) and CSS ABMs (Ehrentreich 2008; Köhler et al. 2009; Chappin & Dijkema 2010) are often more abstract, but include more detailed and complex models of socio-economic systems, behaviours and dynamics. The SD models (Moallemi et al. 2016; Papachristos 2011; 2017; Walrave & Raven 2016) emphasise system analysis with dynamics generated by feedbacks. The SES models (Becu et al. 2003; Gaube & Haberl 2013) extend the CSS ABMs to include ecosystem feedbacks in an integrated assessment.
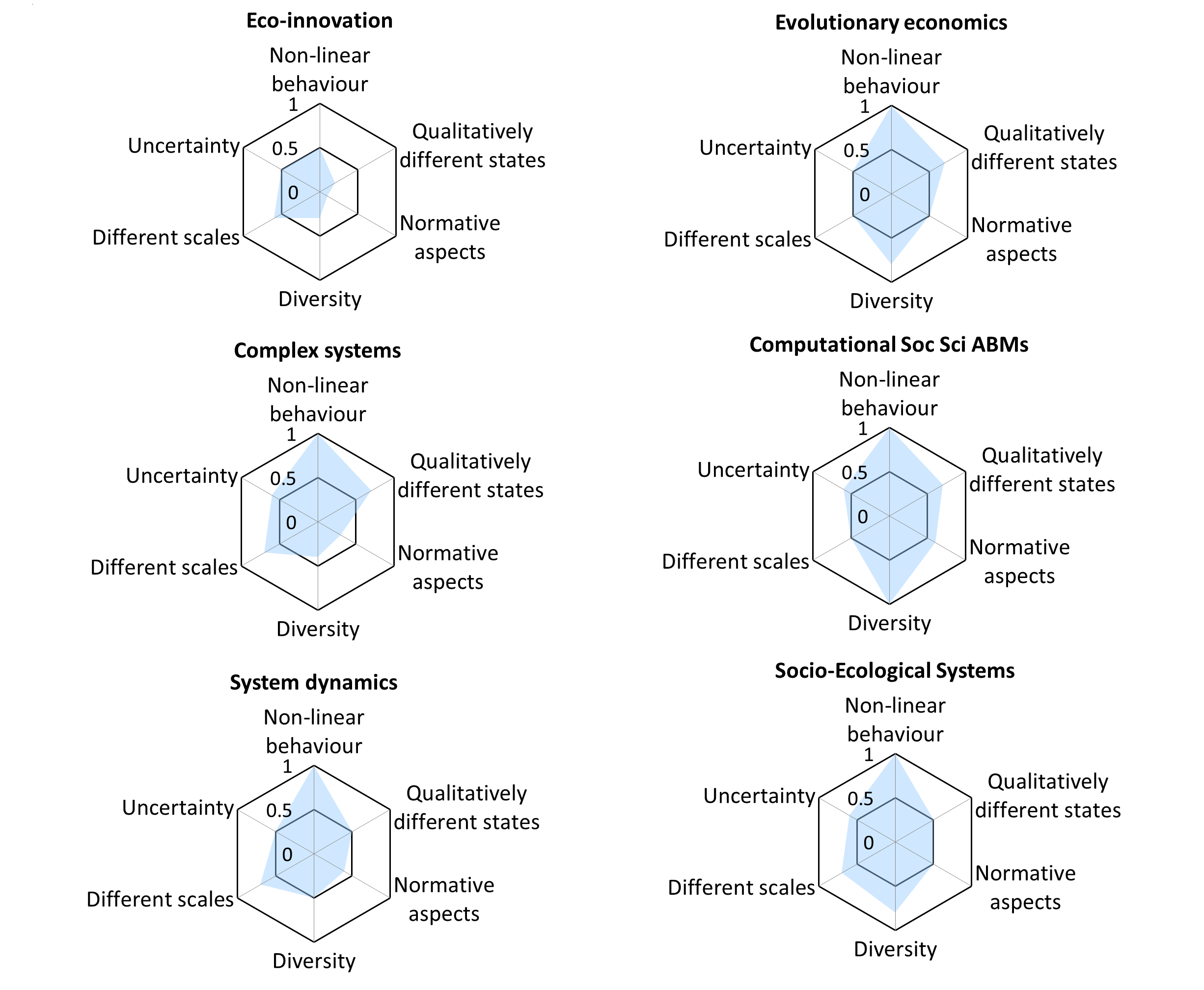
A first conclusion is therefore that it is possible for transitions modelling to make use of a variety of approaches. Different models and approaches can emphasise different aspects of sustainability transitions research. Figure 1 furthermore provides transition researchers with a starting point for their choice of a modelling approach, whose characteristics should correspond to the characteristics of the research question they face. It is also important to note that some models can be regarded as combinations of these approaches. Köhler et al. (2009) combine the SD and CSS ABM approaches and Gaube & Haberl (2013) include a CSS ABM as part of an SES model.
However, a clear picture (based on a qualitative assessment of the modelling approaches) does emerge for the set of types of models considered. The ability to represent non-linear system behaviour and path dependency is high, because of the system nature of these approaches. They are all built around system concepts of a set of modelling entities with feedbacks and behaviour rules that may have positive or negative feedback effects. Taken together, the set of model types can also represent heterogeneity well, especially the CSS ABMs and evolutionary models.
The ability to represent different scales within the same model is more mixed. While all the approaches can be applied in principle to both micro and macro levels of analysis, evolutionary economics models and complex systems models have been used more for micro level analysis. The energy system models (Li et al. 2015) climate policy IAMs (Köhler et al. 2006) and SES models (Schlüter et al. 2012) are applied to more aggregated levels, with simpler representations of micro-level behaviour. Most models in these literatures have used a ‘bottom-up’ system approach, where the model is built up from small scale components which can then exhibit emergent large scale system behaviour, a feature of complex systems. Some models also have higher level structure included as well, such as regime and landscape features in Köhler et al. (2009) or some of the SD models (Papachristos 2011; Moallemi et al. 2016). The energy-economy models (ETSAP 2017a; Leimbach et al. 2010) and IAMs often represent the macroeconomic scale while having a basis in microeconomic theory (Köhler et al. 2006; Stehfest et al. 2014; ETSAP 2017b).
The model approaches are weakest in representing qualitatively different states and the normative aspects of change, such as new fields of social activity, new ways of living or changes in cultural values. It is important to emphasise here that we know of no other modelling approaches that consider such topics that do a better job. The problem is more an inherent problem of modelling evolving complex social systems. In order to implement a computer model, it is necessary to specify beforehand the variables, relationships and data. Evolution requires however the emergence of new patterns of behaviour though both random changes and recombination i.e. new combinations of science, technology/products, markets, institutions and culture. Such processes have only rarely been applied in sustainability transitions modelling. Recombinant technologies are explored in e.g. Frenken et al. (2012), Van den Bergh (2008) and Zeppini & van den Bergh (2011), but these concentrate on technologies rather than social changes or norms.
Another area of qualitative change where models are limited is in addressing changes in the system structure e.g. markets and other institutions, norms and values, emerging new actor groups. Epstein & Axtell (1996) have however demonstrated that the representation of emergent institutions using a CSS ABM approach is possible. Sustainability transitions involve by definition the creation and emergence of new socio-technological systems, which involve these structural changes. Developing models of changes in these areas requires the incorporation of mechanisms for changing decision rules or heuristics, as opposed to changing decisions based on changes in e.g. relative prices in economic models. Evolutionary models with recombination would seem to be promising approaches for exploring structural change. Zeppini et al. (2014) develop this argument, citing examples of evolutionary economics models that model recombinant innovation (Zeppini & van den Bergh 2011; Frenken et al. 2012).
The incorporation of uncertainty and contingencies presents a particularly difficult problem for models in this field. Since all the approaches use non-linear simulation methods, contingencies/uncertainties are incorporated in all the models. However, the flexibility this allows and the need to consider system changes in the future result in a very high level of uncertainty in the projections generated by such models. Uncertainty is addressed through limited scenario analysis, rather than statistical methods of uncertainty analysis. Holtz et al. (2015) suggest that in the context of (highly) complex socio-technical systems addressed in transitions research, models can be used for simulation experiments to assess the consequences of uncertainties. Kwakkel, Auping & Pruyt (2013) have discussed the use of exploratory modelling with SD for dealing with uncertainty. Halbe et al. (2015) discuss approaches used for addressing uncertainty. Sensitivity analyses are widely applied (Bennett et al. 2010; Schlüter et al. 2012). Monte Carlo methods have also been applied to some ABMs of ecosystems (e.g. Schouten et al. 2014).
What is often not explicitly represented are the feedbacks between socio-technical systems and the biosphere. It can be argued that models taking the environmental issue as given (e.g. climate change as a social and policy issue) can regard the feedbacks from the biosphere as constant in the timescale of the model. However, since the argument for sustainability policy is at least partly based on the desire to reduce the impacts of ecosystem change on society, it should be a part of analysis in sustainability transitions. This is an important contribution of the SES (socio-ecological systems) models (Schlüter et al. 2012) and of the IAMs (Stehfest et al. 2014). For other approaches, there is usually at most an (often implicit) unidirectional link – from human activity to emissions through the use of technology. Some system dynamics models, such as Meadows’ Limits to Growth (Meadows et al. 1972) and more recently Feng et al. (2013) have addressed these issues of feedbacks from the biosphere to socio-technical systems.
Conclusions: Identifying a Niche for Transitions Modelling
In this paper, we have reviewed some of the main strands in modelling of socio-technological change. While Holtz et al. (2015) review the possible roles of simulation modelling in transitions research, the present paper is intended to discuss the ability of these approaches to address key characteristics of sustainability transitions as identified by empirical research (Rotmans et al. 2001; Smith et al. 2005, 2010; Coenen et al. 2012; Grin, Rotmans & Schot 2010; STRN 2010). We have identified six features that we argue models require in order to address these characteristics:
- Capability of representing non-linear behaviour
- Capability of representing qualitatively different system states
- Capability of representing changes in social values and norms
- Capability of representing diversity and heterogeneity
- Capability of representing dynamics at and across different scales
- Capability of incorporating open processes and uncertainties or contingencies
It is not the objective of this paper to argue that some modelling approaches are not relevant because they do not have these features. However, we argue that a model that seeks to represent the social processes that according to the current literature make up sustainability transitions does need to include (several of) these features. This review provides transition researchers with a starting point for their choice of a modelling approach, which should address the characteristics of the research question they face.
We identify six approaches that are of particular relevance to modelling transitions:
- Eco-innovation (energy-economy models and Integrated Assessment Models)
- Evolutionary economics
- Complex systems
- Computational social science: Agent-Based models
- System Dynamics (SD)
- Socio-ecological systems (SES)
These modelling approaches can address many of the required characteristics that differentiate sustainability transitions from other socio-economic dynamics or innovations. The most critical features are the representation of qualitatively different states and of the normative aspects of change, i.e. that of representing profound or qualitative changes in societal systems including actors, practices, institutions and technologies. Such changes are linked in transitions theory with changes in norms and culture (Freeman & Louçã 2001; Grin, Rotmans & Schot 2010). These are also identified as areas where modelling is limited, even in the analyses that include transitions thinking. Analogous to physical systems, the evolutionary economics and complex systems models are intended to allow for emergent system properties or phase changes (Safarzynska & van den Bergh 2010; Zeppini et al. 2014; de Haan 2008).
However, there are very few representations of transitions to different states of combined economic, technical, social and institutional systems as identified in the transitions literature (e.g. Grin, Rotmans & Schot 2010). CSS ABMs (Epstein & Axtell 1996) and evolutionary economics models including recombinant innovation (Zeppini & van den Berg 2011; Frenken et al. 2012) indicate approaches for generating new institutions and social/economic structures.
The importance of these aspects and the lack of model approaches to address them suggests innovative models need to be developed that explicitly address the co-evolution of preferences/values, institutions/norms, technological innovations and behaviour. This would enable models to address the emergence and stabilization of niches and the discontinuation of regimes with a broader indicator set than only market shares of technologies (Grin, Rotmans & Schot 2010; STRN 2010). This suggests one possible direction of development for transitions modelling: models of co-evolution of behaviours and technologies towards sustainability, involving change in the structure of the societal and technical systems. For such a development, an early and fundamental challenge will be to define more precisely “change in the structure of the societal and technical systems”, and how it can be represented in a computer model. The literature on sustainability transitions and eco-innovation provides some suggestions for pathways towards sustainability (e.g. Geels & Schot 2007; Papachristos et al. 2013), but these require interpretation for the development of formal relationships that can be implemented in a simulation model.
The representation in simulation models of cognitive and regulative institutions and the dynamics of formation and changes of norms and values (Elsenbroich & Gilbert 2013 provide a recent review of methods) is also an area for further investigation, and an important building block for models of the broader type mentioned beforehand.
A further direction where the current modelling can be developed is the modelling of ecosystems coupled with transitions in socio-technical systems. This would be an extension of current Integrated Assessment Modelling of environmental policy issues such as water catchment (Becu et al. 2003) or forestry and also urban sprawl, where the response times of the eco-system are of the same order as the changes in socio-technical systems. The socio-ecological modelling literature also provides a relevant modelling basis for the ecosystem-social system feedbacks (Fletcher & Hilbert 2007; Gaube & Haberl 2013).
The linkages between the niche and meso/macro scales (regime, landscape) also require further research. As Rotmans et al. (2001) and Geels & Schot (2007) argue, the relative timing of events and feedbacks between the scales and (socio-technical) subsystems (niches, innovation systems, multiple regimes) determine the possible pathways of transition.
Notes
- Based on the search conducted on 10 of October 2016 with the following string in Scopus: TITLE-ABS-KEY (("sustainability transition*") OR ("socio-technical transitions") OR ("Societal transition") OR ("multi-level perspective") OR ("transition management")) AND TITLE-ABS-KEY ((modelling) OR ("transition model") OR (simulation)). The irrelevant articles were removed from the results. The papers published in the proceedings of IST (sustainability transitions research network) conferences in 2015 and 2016 were added manually, because IST is the only conference specifically on transitions research.
- Societal systems as we use the term here include the regime as defined by STRN (2010) as well as niches that relate to the same societal function as the regime.
References
ALKEMADE, F., Frenken, K., Hekkert, M.P., & Schwoon, M. (2009). A complex systems methodology to transition management. Journal of Evolutionary Economics, 19, 527–543. [doi:10.1007/s00191-009-0144-x]
ARTHUR, W.B., Holland, J.H., LeBaron, B., Palmer, R., & Tayler, P., (1996). Asset Pricing under Endogenous Expectations in an Artificial Stock Market. http://www2.econ.iastate.edu/tesfatsi/ahlpt96.pdf.
ASTRA (2017) www.astra-model.eu. Accessed 19/10/2017.
AUPING, W.L, Pruyt, E., de Jong, S., & Kwakkel, J.H. (2016). The geopolitical impact of the shale revolution: Exploring consequences on energy prices and rentier states. Energy Policy, 98, 390-399.
BABATUNDE, K.A., Begum, R.A., & Said, F.F. (2017). Application of computable general equilibrium (CGE) to climate change mitigation policy: A systematic review. Renewable and Sustainable Energy Reviews, 78, pp. 61-71. [doi:10.1016/j.rser.2017.04.064]
BAK, P. (1996). How Nature Works: The Science of Self-Organized Criticality. New York: Copernicus Books.
BECU, N., Perez, P., Walker, A., Barreteau, O., & Page, C.L. (2003). Agent based simulation of a small catchment water management in Northern Thailand description of the CATCHSCAPE model. Ecological Modelling, 170, 319-331- [doi:10.1016/S0304-3800(03)00236-9]
BEINHOCKER, E. (2006). The Origin of Wealth. Boston, MA: Harvard Business Review Press.
BENNETT, N.D., Croke, B.F.W., Jakeman, A.J., Newham, L.T.H., & Norton, J.P. (2010). ‘Performance evaluation of environmental models’. In: D.A. Swayne, W. Yang, A. Rizolli, A. Voinov, T. Filatova (Eds.), International Environmental Modelling and Software Society (iEMSs), 2010 International Congress on Environmental Modelling and Software, Ottawa, Canada.
BERGMAN, N., Haxeltine, A., Whitmarsh, L., Köhler, J., Schilperoord, M., & Rotmans, J. (2008). Modelling socio-technical transition patterns and pathways. Journal of Artificial Societies and Social Simulation, 11(3), 7: https://www.jasss.org/11/3/7.html.
BRUCKNER, T. (2016). Decarbonizing the global energy system: An updated summary of the IPCC report on mitigating climate change. Energy Technology, 4(1), 19–30. [doi:10.1002/ente.201500387]
CLARKE L., K. Jiang, K. Akimoto, M. Babiker, G. Blanford, K. Fisher-Vanden, J.-C. Hourcade, V. Krey, E. Kriegler, A. Löschel,D. McCollum, S. Paltsev, S. Rose, P. R. Shukla, M. Tavoni, B. C. C. van der Zwaan, & van Vuuren, D.P. (2014). ‘Assessing Transformation Pathways’. In Edenhofer, O., R. Pichs-Madruga, Y. Sokona, E. Farahani, S. Kadner, K. Seyboth, A. Adler, I. Baum, S. Brunner, P. Eickemeier, B. Kriemann, J. Savolainen, S. Schlömer, C. von Stechow, T. Zwickel & J.C. Minx (Eds.), Climate Change 2014: Mitigation of Climate Change. Contribution of Working Group III to the Fifth Assessment Report of the Intergovernmental Panel on Climate Change. Cambridge, United Kingdom and New York, NY, USA: Cambridge University Press.
CHAPPIN, E.J.L. (2011). Simulating Energy Transitions. Delft University of Technology.
CHAPPIN E.J.L. & Dijkema, G.P.J. (2010) Agent-based modelling of energy infrastructure transitions. International Journal of Critical Infrastructures, 6(2), 106-30.
CHRISTENSEN, V., Steenbeek, J. and Failler, P. (2011). A combined ecosystem and value chain modelling approach for evaluating societal cost and benefit of fishing, Ecological Modelling, 222, 857-864. [doi:10.1016/j.ecolmodel.2010.09.030]
COENEN, L., Benneworth, P., & Truffer, B. (2012). Towards a spatial perspective on sustainability transitions. Research Policy, 41(6), 968-979.
CONTE, R. Andrighetto, G. & Campennì, M. (Eds.) (2013). Minding Norms: Mechanisms and Dynamics of Social Order in Agent Societies. Oxford Series on Cognitive Models and Architectures. New York: Oxford University Press. [doi:10.1093/acprof:oso/9780199812677.001.0001]
DE HAAN, F.J. (2008). The dynamics of functioning: Investigating societal transitions with partial differential equations. Computational and Mathematical Organization Theory, 14, 302–319.
EHRENTREICH, N. (2008). Agent-based modeling: The Santa Fe Institute artificial stock market model revisited. Berlin, Heildelberg: Springer.
ELSENBROICH, C. & Gilbert, N. (2013). Modelling Norms. Berlin: Springer-Verlag.
EPSTEIN, J.M. & Axtell, R. (1996). Growing Artificial Societies: social science from the bottom up. Massachusetts: MIT Press. [doi:10.7551/mitpress/3374.001.0001]
ETSAP (2017a) Energy Systems Analysis Tools. www.iea-etsap.org. Accessed 19/10/2017
ETSAP (2017b) Global Energy Systems Analysis Applications, www.iea-etsap.org/index.php/applications/global. Accessed 19/10/2017
FAGERBERG, J., Mowery, D. & Nelson, R., (eds.) (2006). The Oxford Handbook of Innovation. Oxford: Oxford University Press.
FENG, Y.Y., Chen, S.Q. & Zhang, L.X. (2013). System dynamics modeling for urban energy consumption and CO2 emissions: A case study of Beijing, China. Ecological Modelling, 252, 44-52. [doi:10.1016/j.ecolmodel.2012.09.008]
FLETCHER, C.S. & Hilbert, D.W. (2007). Resilience in landscape exploitation systems. Ecological Modelling, 201, 440–452.
FREEMAN, C. & Louçã, F. (2001). As Time Goes By: From the Industrial Revolutions to the Information Revolution. New York: Oxford University Press.
FRENKEN, K., (2006). Technological innovation and complexity theory. Economics of Innovation and New Technology, 15(2), 137-155. [doi:10.1080/10438590500141453 ]
FRENKEN, K., Izquierdo, L.R., & Zeppini, P. (2012). Branching innovation, recombinant innovation and endogenous technological transitions. Environmental Innovation and Societal Transitions, 4, 25-35. [doi:10.1016/j.eist.2012.06.001]
GAUBE, V., & Haberl, H. (2013). 'Using integrated models to analyze socio-ecological system dynamics in Long-Term Socio-ecological Research - Austrian Experiences.' In S.J. Singh, H. Haberl, M. Chertow, M. Mirtl, & M. Schmid (Eds.), Long Term Socio-Ecological Research Studies in Society-Nature Interactions Across Spatial and Temporal Scales. Dordretch: Springer, pp. 53-75.
GAZHELI, A., Antal, M. & van den Bergh, J. (2015). The behavioral basis of policies fostering long-run transitions: Stakeholders, limited rationality and social context. Futures, 69, 14-30. [doi:10.1016/j.futures.2015.03.008]
GEELS, F.W. (2002). Technological transitions as evolutionary reconfiguration processes: A multi-level perspective and a case-study. Research Policy, 31(8), 1257-1274.
GEELS, F.W. & Schot, J. (2007). Typology of sociotechnical transition pathways. Research Policy, 36(3), 399-417. [doi:10.1016/j.respol.2007.01.003]
GEELS F.W, & Schot J. (2010) 'The dynamics of transitions: a socio-technical perspective'. In Grin, J.; Rotmans, J.; Schot, J. W. (Eds), Transitions to sustainable development. Part II, Routledge Studies in Sustainability Transitions. New York: Routledge, pp. 105-220.
GEROSKI, P. (2000). Models of technology diffusion. Research Policy, 29(4-5), 603-625. [doi:10.1016/S0048-7333(99)00092-X]
GIDDENS, A. (1984). The Constitution of Society: Outline of the Theory of Structuration. Cambridge: Polity Press.
GILBERT, N., & Troitzsch, K.G. (2005). Simulation for the Social Scientist. New York, NY: Open University Press.
GRIN, J., Rotmans, J. & Schot, J. (2010). Transitions to Sustainable Development. London: Routledge.
HABERL, H., Fischer-Kowalski, M., Krausmann, F. & Winiwarter, V. (Eds.) (2016). Social Ecology Society-Nature Relations across Time and Space. Berlin: Springer.
HALBE J., Reusser D.E., Holtz G., Haasnoot M., Stosius A., Avenhaus W. & Kwakkel J.H. (2015). Lessons for model use in transition research: A survey and comparison with other research areas. Environmental Innovation and Societal Transitions, 15, 194-210.
HECKBERT, S., Baynes, T. & Reeson, A. (2010). Agent-based modeling in ecological economics. Annals of the New York Academy of Sciences, 1185, 39–53. [doi:10.1111/j.1749-6632.2009.05286.x]
HEDSTROM, P. (2005). Dissecting the Social: On the Principles of Analytical Sociology. Cambridge: Cambridge University Press.
HOLLAND, J.H. (1992). Complex Adaptive Systems. Daedalus, 121, 17–30.
HOLTZ, G. (2011). Modelling transitions: an appraisal of experiences and suggestions for research. Environmental Innovation Societal Transitions, 1(2), 167–186.
HOLTZ, G., Alkemade F., de Haan F., Köhler J., Trutnevyte E., Luthe T., Halbe, J., Papachristos, G., Chappin, E., Kwakkel, J. & Ruutu, S. (2015). Prospects of modelling societal transitions: Position paper of an emerging community. Environmental Innovation and Societal Transitions, 17, 41-58. [doi:10.1016/j.eist.2015.05.006]
JANSSEN, M.A., & Jager, W. (2002). Simulating diffusion of green products. Coevolution of firms and consumers. Journal of Evolutionary Economics, 12, 283–306.
KAUFMANN, S.A. (1993). The Origins of Order: Self-Organization and Selection in Evolution. New York, Oxford: Oxford University Press.
KÖHLER, J., Grubb, M., Popp, D. & Edenhofer, O. (2006). The transition to endogenous technical change in climate-economy models: A technical overview to the innovation modeling comparison project. Energy Journal, 27, 17-55.
KÖHLER, J., Whitmarsh, L., Nykvist, B., Schilperoord Bergman, N. & Haxeltine, A. (2009). A transition model for sustainable mobility. Ecological Economics, 68, 2985-2995. [doi:10.1016/j.ecolecon.2009.06.027]
KÖHLER, J. (2012). A comparison of the Neo-Schumpeterian theory of Kondratiev waves and the Multi-Level Perspective on transitions. Environmental Innovation and Societal Transitions, 3, 1-15.
KWAKKEL, J.H., Auping, W.L. & Pruyt, E. (2013). Dynamic scenario discovery under deep uncertainty: The future of copper. Technological Forecasting and Social Change, 80(4), 789-800. [doi:10.1016/j.techfore.2012.09.012]
KWAKKEL, J.H. & Pruyt, E. (2013). Exploratory Modeling and Analysis, an approach for model-based foresight under deep uncertainty. Technological Forecasting and Social Change, 80(3), 419-431.
LEIMBACH M., Bauer, N., Baumstark, L., Luken, M., & Edenhofer, O. (2010). Technological change and international trade—insights from REMIND-R. Energy Journal, 31(1), 109-136. [doi:10.5547/ISSN0195-6574-EJ-Vol31-NoSI-5]
LEVINTHAL, D. & March, J.G. (1981). A model of adaptive organizational search. Journal of Economic Behavior and Organization, 2(4), 307-333.
LI, F.G.N., Trutnevyte, E., & Strachan, N. (2015). A review of socio-technical energy transition (STET) models. Technological Forecasting and Social Change, 100, 290-305. [doi:10.1016/j.techfore.2015.07.017]
LOULOU, R., Goldstein, G. & Noble K. (2004). Documentation for the MARKAL Family of Models, Energy Technology Systems Analysis Programme (ETSAP), Paris, France: International Energy Agency (IEA).
MALERBA, F., Nelson, R., Orsenigo, L. & Winter, S. (1999). History friendly models of industry evolution: the computer industry. Industrial and Corporate Change, 8(1), 3-41. [doi:10.1093/icc/8.1.3]
MALERBA, F., Nelson, R., Orsenigo, L. & Winter, S. (2001). Competition and industrial policies in a ‘history friendly’ model of the evolution of the computer industry. International Journal of Industrial Organisation, 19, 635-664.
MALERBA, F., Nelson, R., Orsenigo, L. & Winter, S. (2008). Public policies and changing boundaries of firms in a history friendly model of the coevolution of the computer and semiconductor industries. Journal of Economic Behaviour and Organisation, 67(2), 355-380. [doi:10.1016/j.jebo.2006.10.010]
MANNE, A, Mendelsohn, R. & Richels, R. (1995). MERGE. Energy Policy, 23, 17-34.
MEADOWS, D. H., Meadows, D. L., Randers, J., & Behrens III, W.W. (1972). The Limits to Growth. The Club of Rome. Washington, DC: Potomac.
MESSNER, S., & Strubegger, M. (1995). User's Guide for MESSAGE III. Laxenburg, Austria: International Institute for Applied Systems Analysis (IIASA).
MOALLEMI, E.A., Aye, L., de Haan, F.J. & Webb, J.M. (2016). Policy analysis of renewable electricity development in India: From a transition modelling perspective. Paper presented to The 34th International Conference of the System Dynamics Society,Delft, Netherlands, July 17 – July 21, 2016.
MOALLEMI, E. A., Aye, L., de Haan, F. J., & Webb, J. M. (2017). A dual narrative-modelling approach for evaluating socio-technical transitions in electricity sectors. Journal of Cleaner Production, 162, 1210-1224.
MOALLEMI, E. A., & Malekpour, S. (2017). A participatory exploratory modelling approach for long-term planning in energy transitions. Energy Research & Social Science, In press.
NELSON, R. & Winter, S.G. (1982). An Evolutionary Theory of Economic Change. Cambridge, Massachusetts: The Belknap Press.
OLTRA, O., & Saint-Jean, M. (2005). The dynamics of environmental innovations: three stylized trajectories of clean technology. Economics of Innovation and New Technology, 14, 189-212. [doi:10.1080/1043859042000226202]
PAPACHRISTOS, G. (2011). A system dynamics model of sociotechnical regime transitions. Environmental Innovation and Societal Transitions, 1(2), 202-233.
PAPACHRISTOS, G. (2014). Towards multi-system sociotechnical transitions: Why simulate. Technology Analysis and Strategic Management, 26(9), 1037-1055. [doi:10.1080/09537325.2014.944148]
PAPACHRISTOS, G. (2017). Diversity in technology competition. The link between platforms and sociotechnical transitions. Renewable and Sustainable Energy Reviews, 73, 291-306.
PAPACHRISTOS, G., Sofianos, A., & Adamides, E. (2013). System interactions in socio-technical transitions: Extending the Multi-Level Perspective. Environmental Innovation and Societal Transitions, 7, 53-69. [doi:10.1016/j.eist.2013.03.002]
POPP, D., Newell, R.G. & Jaffe, A.B. (2010). 'Energy, the environment, and technological Change.' In H.B. Hall & N. Rosenberg (Eds.), Handbook of the Economics of Innovation, Volume 2. Elsevier, pp. 873-937. [doi:10.1016/S0169-7218(10)02005-8 ]
RAHMANDAD, H. & Sterman, J. (2008). Heterogeneity and network structure in the dynamics of diffusion: Comparing agent-based and differential equation models. Management Science, 54(5), 998-1014. [doi:10.1287/mnsc.1070.0787]
RICHARDSON, G.P. (2011). Reflections on the foundations of system dynamics. System Dynamic Review, 27(3), 219-243. [doi:10.1002/sdr.462 ]
RIP, A. & Kemp, R. (1998). ‘Technological Change.’ In S. Rayner, E. L. Malone (Eds,), Human choice and climate change. Vol. II, Resources and Technology, Columbus, Ohio: Battelle Press, 327-399.
ROTMANS, J., Kemp R. & van Asselt, M. (2001). More evolution than revolution: Transition management in public policy. Foresight, 3(1), 15-31. [doi:10.1108/14636680110803003 ]
ROTMANS, J. & Loorbach, D. (2009). Complexity and Transition Management. Journal of Industrial Ecology, 13, 184–196. [doi:10.1111/j.1530-9290.2009.00116.x]
SAFARZYNSKA, K. & van den Bergh, J.C.J.M. (2010). Demand–supply coevolution with multiple increasing returns: Policy analysis for unlocking and system transitions. Technological Forecasting and Social Change, 77, 297-317. [doi:10.1016/j.techfore.2009.07.001 ]
SAFARZYNSKA, K., Frenken, K. & van den Bergh, J.C.J.M. (2012). Evolutionary theorizing and modeling of sustainability transitions. Research Policy, 41(6), 1011–1024. [doi:10.1016/j.respol.2011.10.014]
SCHLÜTER, M., McAllister, R.R.J., Arlinghaus, R., Bunnefeld, N., Eisenack, K., Hölker, F., Milner-Gulland, E.J., Müller, B., Nicholson, E., Quaas, M. & Stöven M. (2012). New horizons for managing the environment: A review of coupled social-ecological systems modelling. Natural Resource Modeling, 25 (1), 219-272. [doi:10.1111/j.1939-7445.2011.00108.x ]
SCHOUTEN, M., Verwaart, T. & Heijman, W. (2014). Comparing two sensitivity analysis approaches for two scenarios with a spatially explicit rural agent-based model. Environmental Modelling Software, 54, 196–210. [doi:10.1016/j.envsoft.2014.01.003]
SCHULZE, J., Müller, B., Groeneveld, J., & Grimm, V., (2017). Agent-based modelling of social-ecological systems: achievements, challenges, and a way forward. Journal of Artificial Societies and Social Simulation, 20(2), 8: https://www.jasss.org/20/2/8.html. [doi:10.18564/jasss.3423 ]
SCHWOON, M. (2006). Simulating the adoption of fuel cell vehicles. Journal of Evolutionary Economics, 16, 435–472. [doi:10.1007/s00191-006-0026-4]
SHOVE, E. & Walker, G. (2010). Governing transitions in the sustainability of everyday life. Research Policy, 39(4), 471-476. [doi:10.1016/j.respol.2010.01.019 ]
SILVERBERG, G., Dosi, G. & Orsenigo, L. (1988). Innovation, diversity and diffusion: A self-organization model. Economic Journal, 98(393), 1032-1054. [doi:10.2307/2233718]
SMITH, A., & Stirling, A. (2010). The politics of social-ecological resilience and sustainable socio-technical transitions. Ecology and Society, 15(1), 11. [doi:10.5751/ES-03218-150111 ]
SMITH, A., Stirling, A. & Berkhout, F. (2005). The governance of sustainable socio-technical transitions. Research Policy, 34(10), 1491-1510. [doi:10.1016/j.respol.2005.07.005]
STEEL, K.D. (2008). Energy system development in Africa: the case of grid and off-grid power in Kenya. PhD thesis, Massachusetts Institute of Technology.
STEHFEST E.‚ D.P. van Vuuren‚ L. Bouwman‚ T.Kram‚ R. Alkemade‚ M. Bakkenes‚ H. Biemans, A. Bouwman‚ M. den Elzen‚ J. Janse‚ P. Lucas‚ J. van Minnen‚ C. Müller‚ & A. Prins (2014). IMAGE 3.0‚ PBL. http://www.pbl.nl/en/publications/integrated-assessment-of-global-environmental-change-with-IMAGE-3.0.
STERMAN, J. (1982). The energy transition and the economy: a system dynamics approach. PhD, Massachusetts Institute of Technology.
STERMAN, J.D. (1994). Learning in and about complex systems. System Dynamics Review, 19(2-3), 291-330. [doi:10.1002/sdr.4260100214]
STERMAN, J. (2000). Business dynamics: systems thinking and modeling for a complex world. Irwin-McGraw-Hill.
STIRLING, A. (2010). Multicriteria diversity analysis: A novel heuristic framework for appraising energy portfolios. Energy Policy, 38(4), 1622-1634. [doi:10.1016/j.enpol.2009.02.023]
STRN (2010). A mission statement and research agenda for the Sustainability Transitions Research Network: http://www.transitionsnetwork.org/files/STRN_research_agenda_20_August_2010(2).pdf. Accessed 06.03.2017.
STRUBEN, J. & Sterman, J.D. (2008). Transition challenges for alternative fuel vehicle and transportation systems. Environment and Planning B, 35, 1070-1097. [doi:10.1068/b33022t]
SUN, Z., Lorscheid, I., Millington, J.D., Lauf, S., Magliocca, N.R., Groeneveld, J., Balbi, S., Nolzen, H., Müller, B., Schulze, J., & Buchmann, C.M. (2016). Simple or complicated agent-based models? A complicated issue. Environmental Modelling & Software. 86, 56-67. [doi:10.1016/j.envsoft.2016.09.006 ]
SYSTEMDYNAMICS (2017). Tools for system dynamics tools. http://www.systemdynamics.org. Accessed 19/10/2017
TIMMERMANS, J. & de Haan, H. (2008). Special issue on computational and mathematical approaches to societal transitions. Computational and Mathematical Organization Theory, 14, 263–265 [doi:10.1007/s10588-008-9037-z ]
VALENTE, M. (2012). Evolutionary demand: A model for boundedly rational consumers. Journal of Evolutionary Economics, 22(5), 1029-1080. [doi:10.1007/s00191-012-0290-4]
VAN DEN BERGH, J.C.J.M. (2008). Optimal diversity: Increasing returns versus recombinant innovation. Journal of Economic Behaviour and Organization, 68, 565-580. [doi:10.1016/j.jebo.2008.09.003 ]
VAN DEN BERGH, J.C.J.M. & Gowdy, J.M. (2009). A group selection perspective on economic behavior, institutions and organizations. Journal of Economic Behavior and Organization, 72, 1-20. [doi:10.1016/j.jebo.2009.04.017]
VAN DER VOOREN, A. & Alkemade, F. (2012). Managing the diffusion of low emission Vehicles. IEEE Transactions on engineering Management, 59(4), 728-740 [doi:10.1109/TEM.2012.2185802 ]
VAN SAARLOOS, W. (2003). Front propagation into unstable states. Physical Reports, 386, 29–222. [doi:10.1016/j.physrep.2003.08.001]
VAN SLUISVELD Mariësse A.E., Martínez Herreras, S., Daioglou, V. & van Vuuren D. P. (2016). Exploring the implications of lifestyle change in 2 °C mitigation scenarios using the IMAGE integrated assessment model. Technological Forecasting and Social Change, 102, 309–319. [doi:10.1016/j.techfore.2015.08.013 ]
VENNIX A.M.J. (1996). Group Model Building: Facilitating team learning using system dynamics. New York: Wiley & Sons.
WALRAVE, B. & Raven, R. (2016). Modelling the dynamics of technological innovation systems. Research Policy, 45(9), 1833-44. [doi:10.1016/j.respol.2016.05.011 ]
WINDRUM, P. & Birchenhall, C. (1998). Is life cycle theory a special case?: Dominant designs and emergence of market niches through coevolutionary learning. Structural Change and Economic Dynamics, 9, 109-134. [doi:10.1016/S0954-349X(97)00039-8]
WINDRUM, P. & Birchenhall, C. (2005). Structural change in the presence of network externalities: a coevolutionary model of technological successions. Journal of Evolutionary Economics, 15, 123-148. [doi:10.1007/s00191-004-0226-8 ]
WINDRUM, P., Carli, T. & Birchenhall, C. (2009b). Environmental impact, quality, and price: Consumer trade-offs and the development of environmentally friendly technologies. Technological Forecasting and Social Change, 76, 552-566. [doi:10.1016/j.techfore.2008.04.012]
YÜCEL, G. & Chiong Meza, C. (2008). Studying transition dynamics via focusing on underlying feedback interactions. Computational and Mathematical Organization Theory, 14(4), 320-49.
ZEPPINI, P. & van den Bergh, J.C.J.M. (2011). Competing recombinant technologies for environmental innovation: Extending Arthur's model of lock-in. Industry and Innovation, 18(3), 317-334. [doi:10.1080/13662716.2011.561031]
ZEPPINI, P., Frenken, K. & Kupers, R. (2014). Thresholds models of technological transitions. Environmental Innovation and Societal Transitions, 11, 54-70.