Introduction
Contract farming, which is a form of vertical coordination among agricultural supply chain actors, is common practice in agriculture all around the world (Eaton & Shepherd 2001). Its use has recently been expanding, particularly in Asia and Africa (Shepherd 2013; Swinnen & Maertens 2007). Contract farming presents a particular contractual relationship in which farmers produce and deliver designated agricultural commodities and contractors acquire the commodities at a predetermined price (Eaton & Shepherd 2001; Swinnen & Maertens 2007; Shepherd 2013). On one hand, it is of interest to contractors who seek guaranteed outputs of high quality and with consistency. On the other hand, the use of agricultural contracts has become attractive to many small-holder farmers, since the arrangement can assure stable income and access to reliable markets in the modern food supply chain. However, the implementation of contracting programs has several potential risks that would lead to the failure of honoring the agreement from both sides, may it be a contractor or a farmer (Prowse 2012; Shepherd 2013; Will 2015), as this was the case in the Mekong Delta (MKD) region of Vietnam.
The contract rice farming scheme was first applied in Vietnam in 2002 under Decision 80, also known as the “linking together the four house” model, to increase the use of agricultural contracts and promote technological innovation in the rural economy within the country (UNIDROIT 2014). Despite the effort, contract rice farming in the MKD region was unsuccessful with many reported cases of unilateral breaches by either the contractors or farmers (Roberts & Khiem 2005; Khiem & Emor 2005). In 2013, the Vietnamese Government proposed a “large-scale paddy field” model under Decision 62 to pursue a sustainable implementation of the agricultural contracting program. This program under Decision 62 was considered a promising approach in the context of the MKD’s rice supply chain, given the fact that several existing constraints of Decision 80 were addressed with a few successful case studies (Can 2014; Ngan et al. 2015). However, the program expansion has been comparatively slow, with only 11% of total available paddy land in the region currently covered by it (Vietnam Farmer Union 2017; Vietnam Television 2017). Understanding barriers to the growth of this contracting program is thus crucial for the Vietnamese Government and local authorities.
Generally speaking, there are three main obstacles to the expansion of the contract farming scheme in the MKD’s rice supply chain. Firstly, many contractors failed to build trust and invest in long-term relationships with the participants. This lack of trust leads to a low rate of contract farming success (Oxfam Vietnam 2012; Dung 2014). Secondly, several studies revealed that low prices offered from paddy contracts lead to a low rate of participation as well as high rates of breaches from small-holder farmers (Roberts & Khiem 2005; Khiem & Emor 2005). Thirdly, according to World Bank (2013), opportunities for the contractual relationship are limited to enterprises who are not equipped with the required rice processing capacity.
In this paper, we aim to study these three specific issues from the contractor perspective and understand how they impede the success of contract rice farming. We utilize agent-based modeling (ABM), which is well-suited for capturing the dynamics of interactions among individuals and complex combinations of different factors (Bonabeau 2002; An 2012). The strength of ABM is that it is able to predict emergent system-wide behaviors and compare various viable scenarios of different policies, interventions and initiatives, which cannot be observed through empirical studies or statistical analysis alone.
ABM has been widely used to address problems in supply chain management since the 1990s (Labarthe et al. 2007; Oliveira et al. 2016). It has also gained popularity in the research field of agricultural supply chain management (Higgins et al. 2010; Hilletohh & Lättilä 2012; Krejci & Beamon 2012) and agricultural policy analysis (Zimmermann et al. 2009; Kremmydas et al. 2018; Ambekar et al. 2015). However, limited use of ABM approaches can be found in the existing literature on contract farming. To the best of our knowledge, there are only three previous studies, by Handayati et al. (2017), Verwaart et al. (2016), and Khanh et al. (2017), applying ABM to contractual programs of different crops in Indonesia, Kenya, and Vietnam, respectively.
The agent-based model used in this study is an extension of the model proposed by Khanh et al. (2017). Here, we focus on the perspective of contractors in the context of the MKD’s rice supply chain. The context is designed to have the competition of contractors in establishing their contract farming scheme. In a region where agroecological factors favor specific rice varieties, farmers have a choice of engaging with multiple potential buyers. The model is also extended with the introduction of a spot market, in which both farmers and contractors can break the contract to trade with local collectors. These opportunistic behaviors are induced by the volatile nature of recurrent fluctuations in rice prices in the region.
Different combinations of counterfactual scenarios of the contractor’s commitment level, higher offered rate of the contracting price over the spot market price, and availability of rice processing facilities, which correspond to the above-mentioned three obstacles, are then examined. Insights gained from the agent-based model can be beneficial for policy-makers and researchers in Vietnam to understand the difficulties in expanding the contracting program in the MKD’s rice supply chain. Besides that, the model may also be of interest to academic scholars who are keen to apply ABM in agricultural supply chain and contract farming related research.
Background and Motivation
Rice supply chain and spot market
The MKD region plays a central role in Vietnam’s food security both domestically and internationally. Over the past decade, the MKD has accounted for approximately half of Vietnam’s total rice production. The bulk of the MKD’s a rice production has accounted for 95% of Vietnam’s rice exports, a major factor in Vietnam becoming one of the major rice exporting countries in the world (VEPR 2015).
However, the MKD rice system has remained underdeveloped from both the physical and institutional perspectives (World Bank 2011). Most of the rice in the region is undifferentiated and of low quality. Figure 1 shows a traditional rice supply chain in the MKD with six actors between the farm gate and final consumers. It features a modest level of horizontal and vertical coordination (World Bank 2011). Informal commercial ties are common between local collectors and other supply chain players both in upstream and downstream ends. According to Loc & Son (2013), more than 93% of total paddy produced by farmers were sold to collectors from the spot market, and only 4.2% was delivered directly to exporters (shown in Figure 1).
The spot market has long played a key role connecting farmers with downstream players. The MKD has an interlacing drainage and irrigation canal systems serving as the main means for transportation. Rice production in the MKD is fragmented, comprising approximately 1.46 million farmers who grow paddy rice mostly in marginal and small fields (Chen et al. 2015). It would be costly and less convenient for the processors or exporters to buy directly from individual farmers. Local collectors are more efficient in gathering and transporting paddy from the fields to the market.
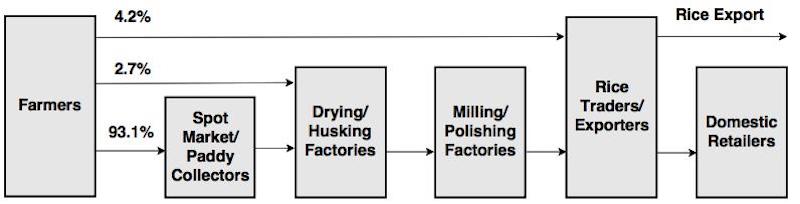
Contract rice farming
According to Minot and Sawyer (2016), contract farming is a form of vertical coordination among agricultural supply chain actors. Two poles of the institutional coordination continuum are open spot markets and vertical integration (Minot et al. 1986; Peterson 2001). The former is the simplest institutional mode of vertical coordination, in which there are no advanced agreements of purchase of agricultural produce between farmers and buyers. The latter is where all supply chain stages ranging from production, processing to distribution are carried out by one firm.
Contract farming presents a particular contractual relationship in which the farmers produce and deliver designated agricultural commodities, while the contractor acquires the commodities at a predetermined price ( Eaton & Shepherd 2001; Swinnen & Maertens 2007; Shepherd 2013). The benefits for farmers and firms are clear and convincing. However, there are also potential disincentives that lead to the failure of honoring the contract agreement from both sides (Prowse 2012; Shepherd 2013; Will 2015), as this was the case in the implementation of contract rice farming in the MKD region.
”Linking together the four house” model
Contract farming was first implemented in Vietnam under Decision No.80/2002/QD-CP (Decision 80). Also known as the “linking together the four house” policy, Decision 80 was one of the few explicit governmental attempts to improve the vertical linkage between small-holder farmers and exporters. The contract farming scheme was expected to be an effective way to draw millions of small farmers in the MKD region into a commercialized supply chain (Asian Development Bank 2007).
However, the contract rice farming application under Decision 80 was largely unsuccessful in the MKD (Roberts & Khiem 2005; Khiem & Emor 2005; Dung 2014; UNIDROIT 2014). The proportion of contract sale was comparatively low. In An Giang, the first province to actively promote the contract farming model, 90% of signed paddy contracts were not fulfilled (Roberts & Khiem 2005; Khiem & Emor 2005). Only for some agricultural products with high processing requirements such as sugarcane, milk, and cigarette, the share of sale from agricultural contracts reached 30% (UNIDROIT 2014).
Breaches of contracts were common from both contractors and farmers (Roberts & Khiem 2005; UNIDROIT 2014). Farmers reneged on contracts when they were offered with a higher price and/or more efficient buying arrangement from paddy collectors. The farmers refused to deliver paddy and declined to repay the advances on inputs provided at the beginning of a cropping season. Large buyers were also reported to have failed to honor the contract. They delayed the purchase to manipulate prices and forced farmers to sell at a lower price or tightened the quality standard to reject excessed paddy (Roberts & Khiem 2005).
“Large-scale paddy field” model
To address the existing constraints in Decision 80 and pursue a sustainable practice, the Vietnamese Government has proposed the “large-scale paddy field” model under Decision 62/2013/QD-TTG. Decision 62 clearly specifies the obligations of related parties in the vertical cooperation, in which farmers, organizations of farmers and contractors/enterprises are the main subjects. It also provides a supportive enabling environment, which includes capital sources for conducting assistant policies (Pham & La 2014; Viet 2015).
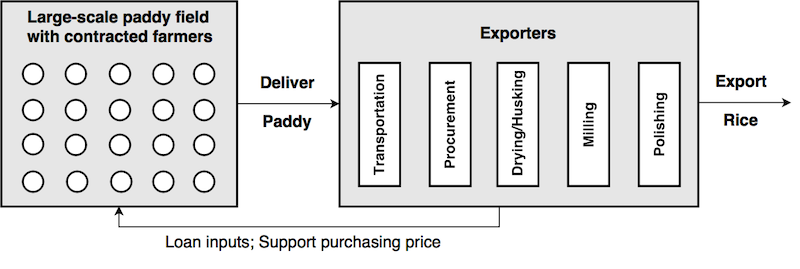
Figure 2 shows the design of a contract rice farming model, which is based on the case of An Giang Plant Protection Joint Stock Company (Ngan et al. 2015). A “large-scale paddy field” is established when the contractor coordinates a group of small farmers and forms an aggregated large area in order to pursue efficiency and maximize profit. Farmers might receive a production loan and technical support to apply environmental-friendly growing methods and produce high-quality rice. The contractors subsequently attain a stable supply source of high-value paddy rice and then trade in the international market. The profits would be shared between contracted farmers through an increased farm-gate price and the contractors to compensate the services provided to farmers and operational activities (Can 2014).
Although advantages of participating in contractual agreement are obvious for the related parties, progress in the expansion of the contract farming scheme in the MKD region under Decision 62 has been comparatively slow (Vietnam Farmer Union 2017; Vietnam Television 2017). It is reported that only 11% of total available paddy land has been used for contract farming. Cases of unilateral breaches from both sides have also been reported (Vietnam Television 2017).
Problems of contract rice farming
There are three main problems leading to the failure of Decision 80 or slow progress in the expansion of Decision 62 of contract rice farming in the MKD. Firstly, many contractors in the region failed to build trust and invest in long-term relationships with farmers, resulting in a low rate of contract farming success (Dung 2014; UNIDROIT 2014). According to Fafchamps (2003), Shepherd (2013) and Will (2015), trust is the most basic and important factor in preventing opportunistic behavior and leading to the success of the contracting agreement. A trust-based relationship between contractors and farmers is also critical in expanding the number of producers involved in the contract farming scheme. Contractors in the MKD are claimed to fail to remain trustworthy by honoring contract obligations when there is an unexpected fluctuation of the market price at the end of the cropping season (Roberts & Khiem 2005; UNIDROIT 2014; Vietnam Television 2017).
Secondly, from world experience, the agricultural contracting scheme is not a cost-effective method for every product (Bijman 2008; Will 2015). Contract farming is commonly found in high-value commodities such as tobacco, sugarcane, coffee, and tea. Growing these commodities, large-scale buyers have more incentive to obtain specific product features, organize good agriculture methods and sell the harvesting at a higher profit margin (Minot et al. 1986). As most of the rice grown in the MKD was of low value, the issues of rice quality and processing are not critical. The contract rice farming scheme may be disadvantageous compared to the spot market, due to high levels of transaction cost in coordinating small-holder farmers. Studies by Khiem & Emor (2005) revealed that offered prices from paddy contracts were usually unable to compete with benefits provided by collectors in the open market, leading to high rates of contract failure.
Thirdly, according to World Bank (2013), the opportunities for the contractual relationship in the MKD are limited by husking, milling and polishing capacity of export firms who are interested and able to provide the package of support. A realistic cost-benefit analysis for the contract farming is particularly crucial to create a viable business for large enterprises as well as a sustainable livelihood for small-holder farmers (Shepherd 2013; Will 2015).
Related work using ABM
ABM is a discrete-event simulation framework that focuses on a population of autonomous and interacting agents. Each individual agent is defined by a distinct set of attributes and behaviors. The agents act on their own rules that allow them to observe, and make changes in response to the interaction with other agents and the environment they are located (North & Macal 2007; Gilbert 2008). The strength of ABM is that it allows a bottom-up approach to model how individuals make decisions in a complex system and predict emergent system-wide behaviors resulting from the interaction and adaptation among those agents (Bonabeau 2002; An 2012; Grimm & Railsback 2011).
ABM has recently gained popularity in the research field of agricultural supply chain management (Higgins et al. 2010; Hilletohh & Lättilä 2012; Krejci & Beamon 2012) and agricultural policy analysis (Zimmermann et al. 2009; Kremmydas et al. 2018; Ambekar et al. 2015). It is a well-suited technique to capture and handle stochastic and dynamic features of the behavior and interaction among supply chain actors as well as the economic aspects of different supply chain processes. It also has the capability to incorporate external uncertainties from an outside environment, including the impact of natural events and the effects of political and social situations, on the decisions of related agricultural supply chain members (Krejci & Beamon 2012; Kremmydas et al. 2018).
However, limited use of ABM approaches can be found in the existing literature of contract farming. Much of the research on contracting agreements in agriculture is either empirical or case-based studies, with only a few focusing on analytical models (Huh & Lall 2013; Federgruen et al. 2015). Handayati et al. (2017) applied ABM in contract farming of tomatoes in the Pangalengan region, Indonesia. They examined how different scenarios of contract farming together with farmer commitment affect the supply chain performance, measured by farmer profits and service levels. Another agent-based model of inclusive business, also known as agricultural contracting, was proposed by Verwaart et al. (2016). The purpose of this model is to evaluate the inclusive business project in the context of agricultural contracts between a local sorghum processor and small-holder farmers in the Meru County, Kenya. Opportunistic behavior of farmers was explicitly modeled with the expected utility of selling produce and the mutual trust among related parties. Parameters of trust, trust development, honesty and risk attitude of the farmers were estimated through behavioral economics games. Khanh et al. (2017) presented a contract farming model of the MKD’s rice supply chain with the use of ABM. The decision-making process of both farmer and contractor agents was based on two factors: cost-benefit analysis and the role of trust. Preliminary results of how farmer and contractor agents’ commitments impact on contracting program performance were discussed. The agent-based model introduced in this study, viewed as an extension of the model proposed by Khanh et al. (2017), focuses on the three obstacles of the expansion of contract rice farming in the MKD region from the perspective of large-scale contractors.
ABM of Contract Rice Farming
Model design
Contract farming process
The model includes two types of agents: farmers and contractors, who are involved directly with the contract farming scheme. Figure 3 presents two stages of the contractual program, pre-harvesting and post-harvesting, in one cropping season. The process is based on the analysis of contract farming practice in the MKD region as well as a conceptual contract farming framework for developing countries proposed by Barrett et al. (2012).
In the pre-harvesting stage, contractors and farmers communicate to establish a contractual relationship. Each contractor determines how to coordinate adjacent farmers into the large-scale paddy field program to fulfil their exporting volume. Pre-harvesting contracts are only initiated when a farmer accepts one of the offers. The contracted farmer obtains technical support to apply sustainable cultivation methods and is required to deliver and sell paddy back to the contractor. The contracted farmer also receives verified paddy seeds for the production as a financial loan from the buyer. A non-contracted farmer, who either declines or receives no offer, grows paddy with traditional practice and trades in the spot market.
In the post-harvesting stage, farmers and contractors observe changes in spot market prices and decide whether to honor or break the contract. If a farmer decides to breach the contract, they can sell to a local collector at a higher spot market price. If the contractor decides to infringe the contract, they can force contracted farmers to sell at a lower contract price than the pre-determined price. If there is no breach by either party, the pre-harvesting contract remains. The farmer sells the harvested paddy and returns the loan to the contractor. The contractor then acquires revenue by exporting polished rice to international buyers. It is assumed that small-holder farmers sell all harvested paddy to buyers, i.e., either contractors or local collectors.
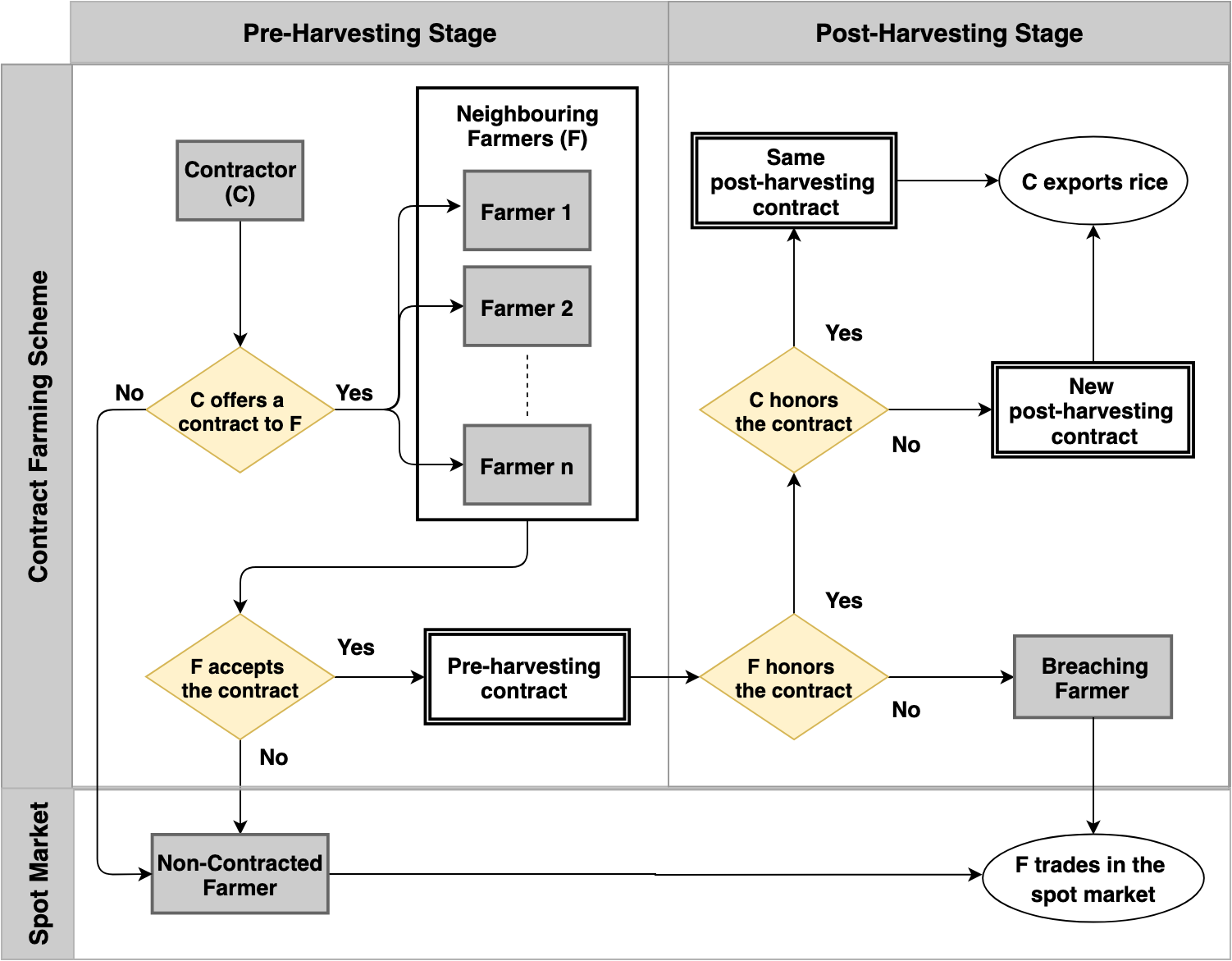
Decision making rules
Agents in the model are assumed to be boundedly rational, making decisions based on preference rankings, which are expressed in scores over potential trading partners. The score calculation that agent \(i\) assigns to agent \(j\) at time \(t\) is the Cobb-Douglas functional form of utility and trust adapted to the proposed function by Klos & Nooteboom (2001) and Nooteboom (2015):
$$score_{ij}(t) = \left(utility_{ij}(t)\right)^{\beta_{i}} \cdot \left(trust_{ij}(t)\right)^{1-\beta_{i}},$$ | (1) |
The utility value is expressed in an exponential utility function that is scaled in the range from zero to one (Garvey 2008; Krejci & Beamon 2015):
$$utility_{ij}(t) = \frac{1 - e^ \frac{-\left(m_{ij}(t)\: -\: m_{i(min)}(t)\right)}{R_{i}}}{1 - e^ \frac{-\left(m_{i(max)}(t)\: -\: m_{i(min)}(t)\right)}{R_{i}}},$$ | (2) |
Equation 3 defines an adaptive trust process between two agents in their ongoing relationship, as presented by Klos & Nooteboom (2001):
$$ trust_{ij}(t) = b_{ij}(t) + (1 - b_{ij}(t)) \cdot \left(1 - \frac{1}{f_{\mathit{CF}} \cdot n_{ij}(t) + 1 - f_{\mathit{CF}} }\right),$$ | (3) |
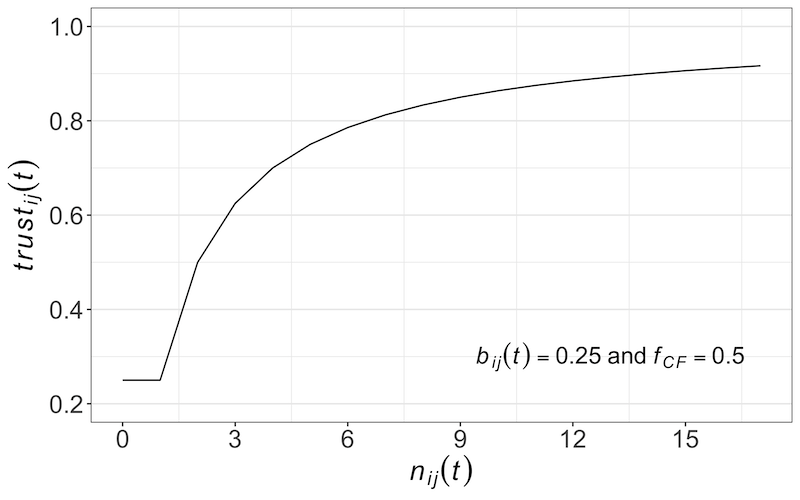
Contractor contract offer
Each contractor, at the pre-harvesting stage of each crop \(t_{pre}\), firstly identifies all prospective farmers, which are located within their farming coverage, \(u_{C}\). The buyer, in each round, sends agricultural contracts to farmers, who are located in the range of \({\bar{u}}_{C}\) further from their location, to achieve their targeted purchasing rice volume. The contract farming proposal continues until either the contractor's objective is fulfilled or the farming coverage is reached to the limit, \(u_{C}\). The contractor is also modeled to repeat the offering one more time with different prospective farmers in the same round to reflect their effort to establish the aggregated paddy grown area.
The contractor, in each offer round, calculates the benefit they earn through the contract with the farmers. Contractor \(i\) agrees to purchase harvesting from farmer \(j\) at proposed price \(p_{ij}(t_{pre})\), processes the dry paddy into export-ready rice, and eventually gains revenue by selling to international buyers. The exporting price, \(\pi_{\mathit{CF}}\), is assumed to be fixed during the simulation run.
$$m_{ij}(t_{pre}) = \left(\pi_{\mathit{CF}} - p_{ij}(t_{pre}) - c_{opr}\right) \cdot q_{j} - g_{j,k} \cdot c_{p,k} \cdot l_{\mathit{CF}} - g_{j,k} \cdot c_{o,{\mathit{CF}}}$$ | (4) |
The offered price, \(p_{ij}(t_{pre})\), at the pre-harvesting stage, is based on the contractor's prediction of spot market price \(p_{\mathit{SM}}(t_{pre})\), with a certain higher rate \(\lambda_{i}\). Both the contractor and farmer agents are modeled to predict the spot market price at time \(t_{pre}\) to be the average of the actual spot market price of the last two seasons.
$$p_{ij}(t_{pre}) = p_{\mathit{SM}}(t_{pre}) \cdot (1 + \lambda_{i})$$ | (5) |
In the large-scale paddy field program, all participating farmers are assumed to acquire the crop yield and incur production costs in the large-scale farmer category regardless of their actual farm size. The volume of rice, \(q_{j}\), delivered by farmer \(j\) after the conversion, is estimated as:
$$q_{j}= v_{large} \cdot g_{j} \cdot r_{conv}$$ | (6) |
The contractor, at this stage, assumes that contracted farmers will commit to the agreement without any breaches. Following Equations (1), (2), and (3), contractor \(i\) calculates \(score_{ij}(t_{pre})\) it allocates to farmer \(j\). The contractor then sends contracts to the farmers, i.e., those who were allocated with the highest score (sorted in descending order) and historically did not breach past contracts, to gather its targeted volume demand.
Farmer contract acceptance
In the pre-harvesting stage, each farmer always has an option to cultivate the paddy by themselves. They receive a credit \(l_{\mathit{SM}}\) paid in advance from a local collector, on the condition that they sell the harvested paddy to the trader in the post-harvesting stage. Farmer \(i\) calculates the potential benefit, \(m_{i,\mathit{SM}}(t)\), with the predicted spot market price, \(p_{\mathit{SM}}(t_{pre})\), crop yield, \(v_{k}\), production cost, \(c_{p,k}\), in accordance to their farm size type \(k\):
$$m_{i,\mathit{SM}}(t_{pre}) = p_{\mathit{SM}}(t_{pre}) \cdot v_{k} \cdot (1 + l_{\mathit{SM}}) \cdot g_{i} - c_{p,k} \cdot g_{i}$$ | (7) |
The farmer might also have offered contracts. The benefit, \(m_{ij}(t_{pre})\), of farmer \(i\) is their expected profit from the contract farming scheme plus the production loan received from a large buyer \(j\). The profit is presumed to be reduced by the autonomy premium, \(\alpha_{i}\), which represents how strongly the farmer values ability to work independently (Key & MacDonald 2006; Krejci & Beamon 2015). To keep the model simple, a value of \(a_{i}\) is initially assigned to each farmer and remains the same during simulation runs.
$$ m_{ij}(t_{pre}) = \frac{(p_{ji}(t_{pre}) \cdot v_{large} - c_{p,large}) \cdot g_{i}} {1 + \alpha_{i}} + c_{p,large} \cdot g_{i} \cdot (1 + l_{\mathit{CF}})$$ | (8) |
The farmer then evaluates all available options and proceeds with the one yielding the highest score. In case the farmer considers engaging in a contractual relationship, they will later decide to honor or break the contract since the predicted spot market price, \(p_{\mathit{SM}}(t_{pre})\), at the initial crop might be different from the later trading price, \(p_{\mathit{SM}}(t_{post})\).
Farmer decision to honor the contract
In the post-harvesting stage, if a farmer commits to the initial agreement, they deliver and expect to sell the harvested produce at the pre-determined price, \(p_{ji}(t_{pre})\). They also return the production loan borrowed from contractor \(j\).
$$m_{ij,honor}(t_{post}) = \frac{(p_{ji}(t_{pre}) \cdot v_{large} - c_{p,large}) \cdot g_{i}}{1 + \alpha_{i}}$$ | (9) |
If a farmer decides to breach the contract, they sell to a local collector at a higher spot market price, \(p_{\mathit{SM}}(t_{post})\), and decline to repay the loan. \(p_{\mathit{SM}}(t_{post})\) is randomly selected among the trading prices in the harvesting period.
A dishonoring farmer also considers future trade suspension with the breached contractor (Fafchamps 2003). The benefit that farmer \(i\) considers in this option will be decreased by the value of the loss relationship with contracted partner \(j\).
$$m_{ij,breach}(t_{post}) = \frac{(p_{\mathit{SM}}(t_{post}) \cdot v_{large} - c_{p,large}) \cdot g_{i}}{1 + \alpha_{i}} - \theta_{F} \cdot m_{ij,loss}$$ | (10) |
The trust value in the contract honoring option is updated with Equation (3), while the trust value in the breaching option is \(trust_{\mathit{SM}}\). If farmer \(i\) decides to renege on the contract, the breached contractor, \(j\), will set their trust base in farmer \(i\) to \(b_{ji(min)}=0\) and the number of trades to initial value \(n_{ji}=0\).
Contractor decision to honor the contract
After farmer \(j\) complies with the agreement, contractor \(i\) decides either to honor or breach the contract. The trust value towards the farmer in both choices is updated with \(trust_{ij}=1\), since the farmer has already delivered the harvested paddy and returned the production loan to the contractor.
If contractor \(i\) decides to infringe the contract, they force the contracted farmer, \(j\), to sell at a lower contract price reflecting the reduction in the current spot market price. The new contracting price, \(p_{ij}(t_{post})\), is based on the average of spot market prices during a three-month post-harvesting time. The contractor's benefit at the post-harvesting stage, \(m_{ij}(t_{post})\), is computed according to Equation (11). In case the contractor reneges on the previous contract, the breached farmer will set their trust base value in the contractor \(b_{ji}=0\), and the number of trades together \(n_{ji}=0\).
$$m_{ij}(t_{post}) = (\pi_{\mathit{CF}} - p_{ij}(t_{post}) - c_{opr}) \cdot q_{j} - g_{j,k} \cdot c_{\mathit{CF}}$$ | (11) |
If the contractor commits to the agreement, the benefit in this honoring option is calculated with Equation (11) using the pre-determined price, \(p_{ij}(t_{pre})\), instead of the lower breaching price, \(p_{ij}(t_{post})\). The number of trades from both sides, \(n_{ij}\) and \(n_{ji}\), then increases by 1 to indicate the growing trust.
Farmer trust update and contractor demand update
At the end of a cropping season, the farmer updates their trust in the contractor partners through communication within the neighborhood defined by the range, \(u_{F}\). The trust-base level of farmer \(i\) will be reduced to a certain extent if there is dishonoring behavior from a large buyer \(j\) with their neighboring farmers. At the same time, farmer \(i\) might have higher trust in contractor \(j\) if their neighbors successfully contracted with the buyer. The change of trust-based level depends on the number of adjacent farmers who have negative or positive experience with contractor \(j\) over all neighbors of farmer \(i\).
$$b_{ij}(t+1) = b_{ij}(t) \cdot (1 - \frac{n_{breach}}{n_{total}}) \cdot (1 + \frac{n_{honor}}{n_{total}})$$ | (12) |
Each contractor, at the end of the season, is modeled to adjust their targeted rice demand for the following crop based on the performance of their contract farming scheme in prior crops. The large buyer calculates their targeted rice demand as the average of total rice volume achieved through their agricultural contracts in the previous two seasons. If the contractor completely fulfills their objective in the last two crops, the demand is expected to be higher at a rate, \(r_d\), than the usual calculation to reflect the potential expansion of the large-scale paddy field program.
Agent details
Farmer agent
Rice production in the MKD is fragmented, comprising approximately 1.46 million farmers who grow paddy in small fields, with the average size of 1.29 hectares (ha) (World Bank 2011). Small-holder farmer agents in the model can be classified into three categories on the basis of their farm size: marginal (0.5-1 ha), small (1-2 ha), and medium (2-3 ha) farmers (Chen et al. 2015). The farmer agents can be further divided into two groups, non-contracted and contracted farmers, which are derived from their participation in the contract farming scheme.
Non-contracted farmers usually apply traditional cultivation methods with an excessive amount of seed, fertilizer, pesticides, and herbicides. In contrast, contracted farmers are required by the contractors to pursue better crop husbandry with fewer inputs in order to save costs and produce higher yield. Crop yields and production costs were collected from a World Bank (2013) research report in the An Giang province, one of the provinces in the MKD region. The data is for ordinary rice, which is of low value, and jasmine rice, which is considered to be among one of the highest quality varieties grown in the region.
There are two major cropping seasons, Winter-Spring (WS) and Summer-Autumn (SA) crops, in the MKD region (FAO 2017). Jasmine paddy is not grown in the SA season, since frequent floods occur during the harvesting period, while ordinary paddy is cultivated in both crops (World Bank 2013). The crop yield and production cost data for each type of rice, grown in each cropping season, and in accordance with different farmer groups and categories, is shown in Table 1.
According to Nhan et al. (2015) and Viet (2015), one of the critical reasons the small-holder farmers in the MKD region decline to participate in the contract farming scheme is that they lost their autonomy of independent production. The autonomy premium, \(\alpha_i\), is assigned to each farmer agent \(i\) following a uniform distribution between \([0.1, 1.0]\)[1].
It is presumed that farmer agents tend to have an unreliable business attitude, since there have been breaches by small-holder producers reported in contract rice farming in the MKD region (Roberts & Khiem 2005; Khiem & Emor 2005; Dung 2014; Vietnam Television 2017). The low level of commitment of the farmer agent, \(n_F\), is randomized following a triangular distribution [0, 2.0, 4.0]. It implies that the longest/maximum time the farmer agent commits in the contractual relationship is 4 cropping seasons.
Non-Contracted | Contracted | ||||||
Marginal | Small/Medium | ||||||
Parameter | Description | WS crop | SA crop | WS crop | SA crop | WS crop | SA crop |
\(g\) | Farm size (ha) | [0.5 − 1] | [1 − 2]/[2 − 3] | Large-scale | |||
Crop yield - ordinary rice (ton/ha) | 6.08 | 4.68 | 6.75 | 5.2 | 7.40 | 5.75 | |
\(v\) | Crop yield - jasmine rice (ton/ha) | 6.38 | 7.09 | 7.77 | |||
Production cost - ordinary rice (million VND/ha) | 21.777 | 16.522 | 20.536 | 15.461 | 18.532 | 13.653 | |
\(c_p\) | Production cost - jasmine rice (million VND/ha) | 23.257 | 22.336 | 19.083 | |||
\(\theta_{F}\) | Commitment level | Triangular Distribution [0.0, 2.0, 4.0] | |||||
\(u_{F}\) | Farmer neighborhood range | 50 | |||||
\(l_{\mathit{SM}}\) | Rate of loan (over the total value of produce) from the spot market | 0.15 | |||||
\(\alpha\) | Autonomy premium | Uniform Distribution [0.1 − 1.0] | |||||
\(\beta_{F}\) | Weight attached to utility over trust | Uniform Distribution [0.1 − 0.9] | |||||
\(b_{ij}(t_0)\) | Initial trust base of farmer \(i\) to contractor \(j\) | Uniform Distribution [0.1 − 0.5] | |||||
\(n_{ij}(t_0)\) | Initial number of trades between farmer \(i\) and contractor \(j\) | 0 |
Because of the past failure of contract farming under Decision 80 (as mentioned in the Contract Rice Farming Section), the farmer agents are assumed to have low trust in the success of the large-scale paddy field program. The trust base of farmer \(i\) toward contractor \(j\), \(b_{ij}(t_0)\), is randomly determined at the start of each simulation run and varies between \([0.1, 0.5]\). Since there is no empirical study of how small-holder farmers in the MKD region value utility over trust, the weight parameter, \(\beta_{F}\), is assigned to each farmer agent, utilizing a uniform distribution in the range of \([0.1, 0.9]\)[2].
Contractor agent
At the pre-harvesting stage, the contractor gives verified paddy seeds as a financial loan to farmers, accounting for 7.5% of the total production cost (Loc & Son 2013; Viet 2015). Contracted farmers are assumed to return the loan after selling the crops to the buyer. The contractor also incurs the operational overhead, which is the cost of hiring workers to support contracted farmers with technical practice (World Bank 2013). At the post-harvesting stage, the contractor processes the harvested dry paddy into polished rice and exports to international buyers. Rice conversion rate \(r_{conv}\) is specified at 0.5 for ordinary rice in both crops and 0.48 for jasmine rice in the WS season. The rate is applied for 5% broken, color graded exporting rice (World Bank 2013).
The costs of husking, milling and polishing activities for both rice varieties were extracted from a research report, conducted by the Institute of Policy and Strategy for Agriculture and Rural Development (IPSARD 2014) during a field trip in An Giang. Exporters associated with the contract farming scheme could also negotiate a better price with buyers because of greater control over the quality of rice. The exporting prices, \(\pi\), for two types of rice either from the spot market or contract farming program were taken from World Bank (2013).
Since large buyers coordinate the contract farming scheme to pursue higher and stable profit, the parameter \(\beta_{C}=0.9\) is fixed for contractor agents. The initial trust base level of contractor \(i\) in farmer \(j\) is set neutrally at \(b_{ij}(t_0)=0.5\). If an agent, either a contractor or a farmer, considers to trade with local collectors, its trust in the spot market is fixed, \(trust_{\mathit{SM}}=0.9\), to reflect a well-established conventional relationship in the MKD region. The trust factor remains at \(f_{\mathit{CF}}=0.5\) during the simulation run for both farmer and contractor agents.
Parameter | Description | Ordinary Rice | Jasmine Rice | |
\(k(t_0)\) | Initial purchasing demand (tons) | 4000 | ||
\(r_d\) | Increasing rate of rice demand | 0.1 | ||
\({\bar{u}}_{C}\) | Range contractors considered in each offer round | 100 | ||
\(u_{C}\) | Contractor farming coverage | 500 | ||
\(l_{\mathit{CF}}\) | Rate of loan (over production cost) to farmers | 0.075 | ||
\(c_{o,\mathit{CF}}\) | Overheads in organizing contract farming (million VND/ton) | 0.408 | ||
\(c_{h,\mathit{SM}}\) | Husking cost in the spot market (million VND/ton) | 1.225 | 1.737 | |
\(c_{m,\mathit{SM}}\) | Milling cost in the spot market (million VND/ton) | 1.066 | 1.899 | |
\(c_{h,\mathit{CF}}\) | Husking cost with contractors’ facilities (million VND/ton) | 0.734 | 0.938 | |
\(c_{m,\mathit{CF}}\) | Milling cost with contractors’ facilities (million VND/ton) | 0.725 | 1.158 | |
\(c_{e}\) | Exporting cost (million VND/ton) | 0.52 | ||
\(\pi_{\mathit{SM}}\) | Exporting price for spot market rice (million VND/ton) | 8.761 | 12.933 | |
\(\pi_{\mathit{CF}}\) | Exporting price for contract farming rice (million VND/ton) | 9.596 | 13.350 | |
\(\beta_{C}\) | Weight contractor attached to utility over trust | 0.9 | ||
\(b_{ij}(t_0)\) | Initial trust base of contractor \(i\) to farmer \(j\) | 0.5 | ||
\(n_{ij}(t_0)\) | Initial number of trades between contractor \(i\) and farmer \(j\) | 0 |
Experiments and Results
Experiment setup
Our agent-based model was implemented in Java using the MASON framework (Luke et al. 2005). Its code can be found on the CoMSES Network website at https://goo.gl/QhLbEY. We ran the simulation 18 steps for ordinary rice and 9 steps for jasmine rice, with each step representing one cropping season. All simulation steps were repeated for 500 independent Monte Carlo trials.
At the start of every Monte Carlo simulation, 3000 farmer agents were created. The proportions of marginal, small, and medium farmers were 54.4%, 32.1%, and 13.5%, respectively. These values were calculated based on research reports from the MKD region (IPSARD 2014; Chen et al. 2015). The simulation model incorporated two contractor agents implementing contract farming activities. All agents were located in a continuous space of size 1000 by 1000 representing a geographic region. Farmer agents were randomly located, while the two contractor agents were set with specific locations: (250,500),(750,500). The locations of agents were set to resemble an agricultural landscape in developing countries, where small-holder producers typically scatter throughout the region while large buyers are located in convenient spots (Chen et al. 2015).
All parameters of individual farmer and contractor agents were initialized according to the values in Table 1 and Table 2. Among those parameters, the trust base \(b_{ij}\), the number of successful trades \(n_{ij}\) between agent \(i\) and agent \(j\), and the purchasing demand \(k\) of contractor agents were updated dynamically during the simulation run. The other listed parameters were set with fixed values.
Available data of monthly spot market prices of dry ordinary paddy in the An Giang province from 2009 to 2017 was gathered from the Information Centre for Agriculture and Rural Development (AgroInfo 2017). Figure 5 shows the volatility of trading prices in the MKD’s ordinary rice supply chain (Chen et al. 2015) with recurrent fluctuations over a nine-year period. For jasmine dry paddy, because of the lack of data, the spot market prices were calculated based on the assumption that they are 1.4 million VND/ton higher than the dry ordinary paddy prices (World Bank 2013). For simplicity’s sake, the dry paddy price traded over the spot market in each crop was calculated as the average of prices in three-month harvest. The harvesting periods were from February to April for the WS crop, and from August to October for the SA crop (FAO 2017). Besides that, the forecast spot market prices, \(p_{\mathit{SM}}(t_{pre})\), at the pre-harvesting stage of the first two crops \(t_1\) and \(t_2\) in Equation 5, were set equal to the median value of actual spot market prices during the nine-year period.
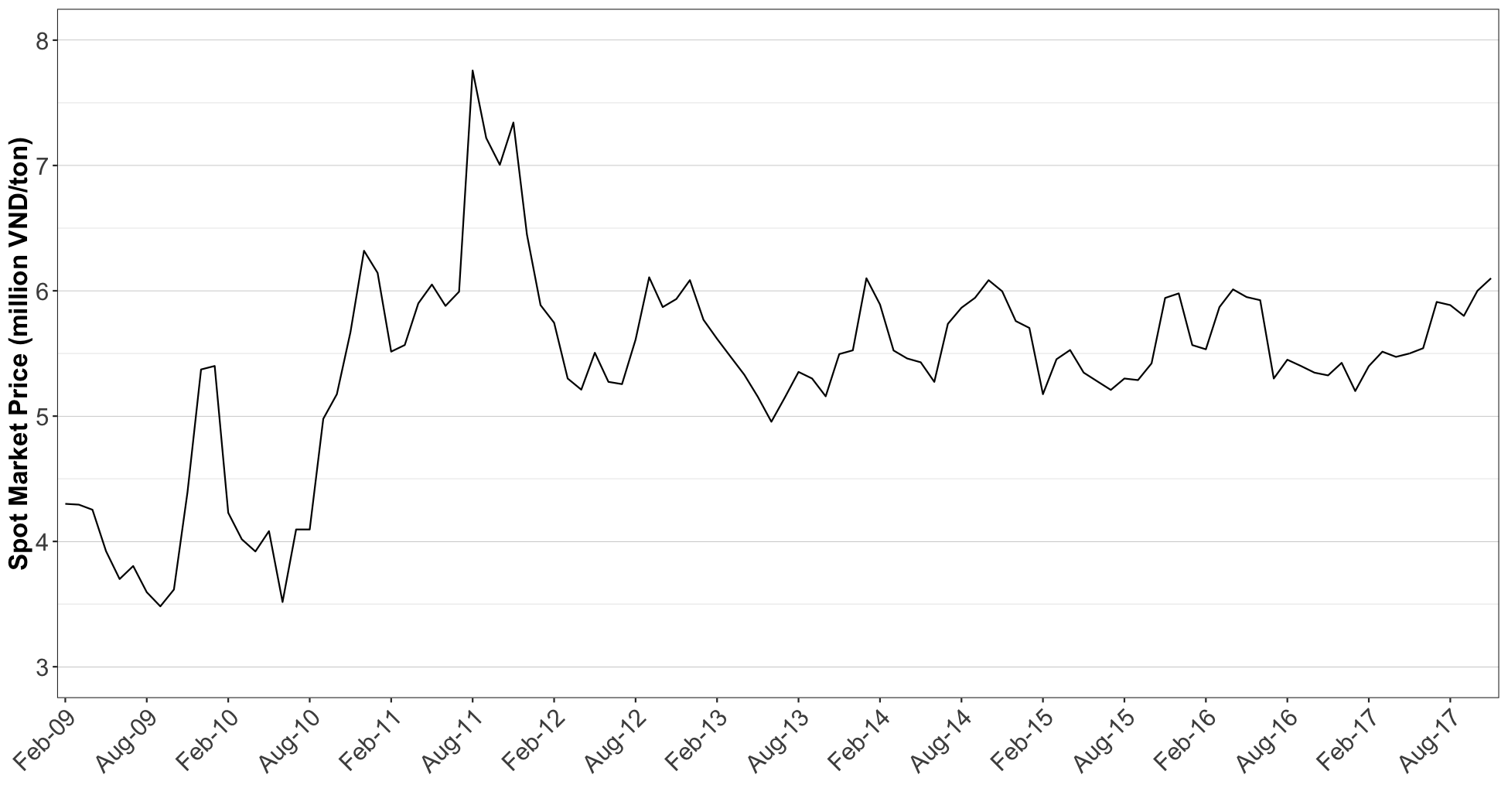
We used the agent-based model to examine three main obstacles of the expansion of contract rice farming in the MKD region from the perspective of large-scale buyers (i.e., contractors). Three corresponding parameters of the contractor agent used for the simulation experiments are as follows:
- Level of commitment \(\theta_C\) in terms of the percentage of contracted farmers that the large buyers breach the contractual relationship with;
- Higher rates of the contracting price over the spot market price, \(\lambda\), that the contractor offers to the farmers;
- Availability of rice processing facilities, \(\delta\).
Parameter | Contractor A | Contractor B |
Commitment level | 0% | [0%, 10%, 20%, 30%] |
Higher offered rates for ordinary rice | 0.2 | [0.0, 0.05, 0.1, 0.15, 0.2, 0.25, 0.3] |
jasmine rice | 0.1 | [0.0, 0.05, 0.1, 0.15, 0.2] |
Processing facilities | True | [True, False] |
Different scenario-based values of these three parameters were examined, as shown in Table 3. The values remained the same during the simulation steps of each Monte Carlo run. In every simulation experiment, one of the two contractor agents – Contractor A as can be seen in Table 3 – was set with fixed values for the three scenario-based parameters. The contractor was modeled to be constantly committed to all farmer agents, \(\theta_{C}=0\%\) = 0%. The proposed contract price by this contractor was set at the most typical (higher) rates in the MKD region with \(\lambda=0.2\) for ordinary rice or \(\lambda=0.1\) for jasmine rice (World Bank 2013). This large buyer agent was modeled to have all adequate capacity, \(\delta=True\), to process export-ready rice products.
Another contractor agent in the model – Contractor B in Table 3 – was setup with varied values in different scenarios. The commitment in the contractual relationship was varied from being committed to being un-trustworthy with 10%, 20%, and 30% of contracted participants. The rate \(\lambda\) was in the range \([0.0,\: 0.05,\: 0.1,\) \(0.15,\: 0.2,\: 0.25,\: 0.3]\) for ordinary rice or \([0.0,\: 0.05,\: 0.1,\: 0.15,\: 0.2]\) for jasmine variety. The buyer was modeled to either be equipped with necessary husking, milling and polishing facilities, \(\delta=True\), or have no rice processing capacity, \(\delta=False\), which requires the utilization of services from other supply chain actors with higher costs.
Different outputs reflecting the contract farming performance of contractor B would then be evaluated in different combinations of scenarios. The simulation model calculates four output variables in each cropping season: the rate of contracted farmers’ breaches, farmers’ trust in the contractor, exporting rice volume, and total profit achieved by the contractor. The contract farming simulation ran separately with two rice varieties. Reported results were calculated by averaging the 500 Monte Carlo trials.
Analysis of results
Evolution of contract farming performance
Figure 6 shows the time-series evolution of contract farming performance with the different combinations of scenarios for both ordinary and jasmine rice. The results of each rice variety include two separate experiments. One experiment examined three scenarios in which fully committed contractors propose different paddy purchasing prices. Another experiment involved the study of contractors, who all offer the typical rate \(\lambda=0.2\) with ordinary rice and \(\lambda=0.1\) with jasmine rice, having varied commitment levels. All contractors in the above settings were set to be equipped with adequate and sufficient rice processing facilities, \(\delta=True\). Performance of a buyer who completely trades over the open market was also included in the results.
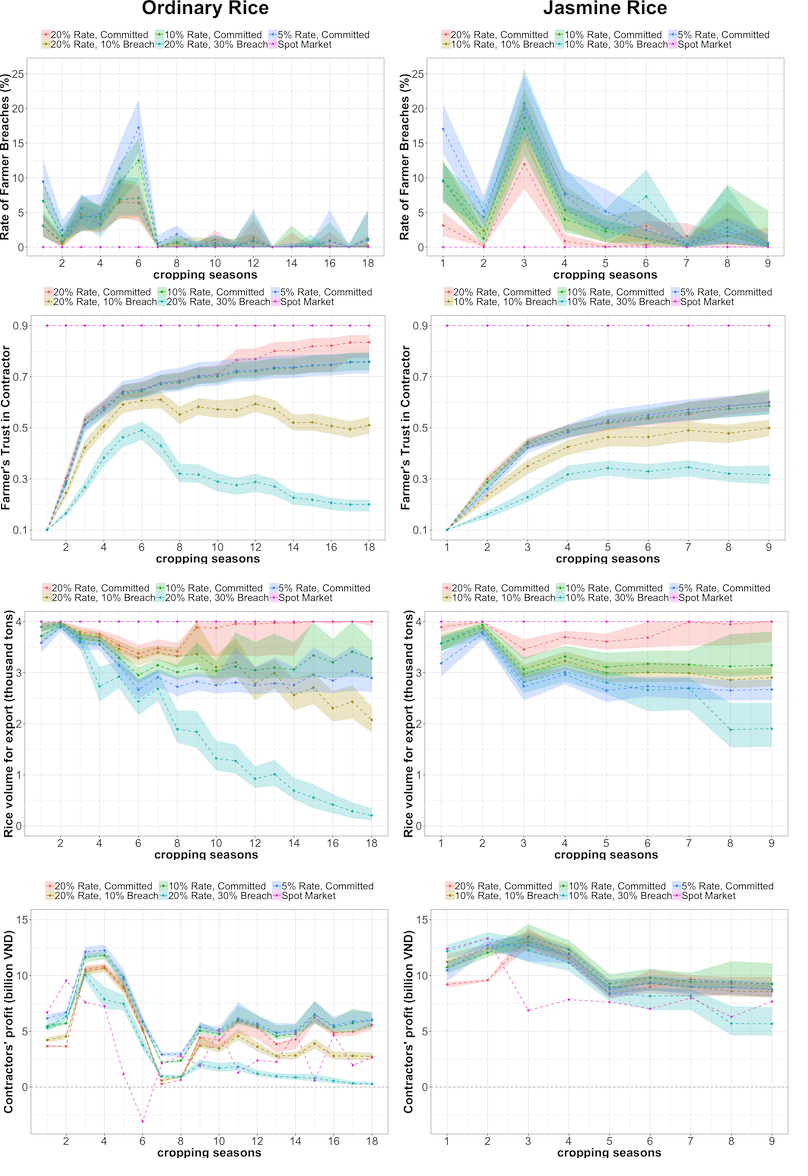
The top two plots in Figure 6 represent the fact that high purchasing prices offered by a trustworthy contractor could impede breaching behaviors from the participants (Eaton & Shepherd 2001; Prowse 2012; Will 2015). Both ordinary and jasmine rice results show a significant decrease in farmer breaches with committed contractors who set the highest rate especially when there are ‘jumps’ in the paddy prices over the open market (see Figure 5 for details). In the 6th crop for ordinary rice, less than 7% of contracted farmers under scenario \(\lambda=20\%\) decide to sell to traders, while the rate of farmer breaches doubles at 14% with scenario \(\lambda=10\%\). A similar pattern can be seen in the 3rd crop in jasmine rice’s case.
Contractors who persist in being committed could sustainably establish long-term trust-based relationship with the existing participants. Farmers’ trust in these contractors grows rapidly in the first few crops and maintains an increasing trend for all simulation runs. There are no significant differences of the farmers’ trust in the trustworthy contractors who proposed different contract rates. However, contractors with a higher offered purchasing price achieve a higher volume of rice. In both ordinary and jasmine rice results, the contractors who advocate their contracted farmers at \(\lambda=20\%\), subsequently attain the targeted initial demand after experiencing shortages of rice supply due to farmer breaches at the early cropping seasons. The contractor with a lower paddy purchasing price could not achieve \(k=4000\) tons of rice, since certain surrounding farmers consider their option not as attractive as services from another large-scale buyer or the local collector.
Among scenarios of the same proposed contract farming rates, contractors with untrustworthy behavior lose trust in the breached farmers and other neighboring farmers. There are downward trends of farmers’ trust in those contractors who have the least commitment level, breaching 30% of the participants, in the results of both rice types. Fewer farmers accept their proposals in the following crops, leading to a considerable decrease of the total rice volume achieved through their large-scale program.
The results reported here are in agreement with the existing evolutionary game theory literature on contractual agreements from both experimental (Chen & Komorita 1994; Dannenberg et al. 2014) and theoretical (Han et al. 2015; Sasaki et al. 2015; Han et al. 2017) perspectives, in that arranging prior commitments leads to a high level of long-term beneficial cooperation. Contractors who are persistent to loan agricultural inputs, provide technical support and acquire rice from the contract farming scheme at a predetermined price from contracted farmers could significantly enhance trust from the participants as well as reduce the number of free-riders. However, the level of prior commitment could also lead to different cooperation rates and interactions (Chen & Komorita 1994). In our results, trustworthy contractors with a more attractive purchasing price achieve a higher volume of rice from the large-scale program and reduce the rate of farmer breaches.
Viability of the contract farming scheme
Figure 7 shows the analysis of viability of the contract rice farming scheme from the perspective of large-scale contractors. We firstly computed a ratio of average profit in all cropping seasons between the contractor and spot market-based buyer to examine the profitability of the scheme. If the profit rate is larger than 1, the contractor is performing better than the spot-market buyer. Otherwise, the contracting program is not a cost-effective method in comparison with complete open-market trading. We also calculated the percentage of average rice volume achieved through the contracting program of the contractor’s initial demand \(k(t_0)=4000\) tons. In each heat map, the y-axis describes different scenarios of contractor commitment \(\theta_C = 0\%\) (breach00), \(\theta_C = 10\%\) (breach10), and \(\theta_C = 30\%\) (breach30), while the x-axis represents varied values of higher contract farming rates over the spot market price.
As shown in the top two heat maps of ordinary and jasmine rice, committed contractors could earn up to 89% and 25% higher profits, respectively, than a buyer trading the same rice variety over the spot market. The contractor with jasmine rice performs at a lower profit rate, since exporting prices of jasmine rice from the contract farming scheme in the MKD region are only 2.45% higher than from the open market. Ordinary rice could be sold to an international buyer at 9.5% higher if it is grown and harvested from the large-scale field program (see Table 2 for details). However, jasmine rice could yield higher profit per ton of rice since it is of high-value in the MKD region. The spot market-based buyer could obtain on average 3.43 billion VND per cropping season with ordinary rice and more than double at 8.54 billion VND with jasmine rice (as shown in the bottom plots of Figure 6).
The simulation results also show that contractors who are untrustworthy to a relatively small portion, such as 10%, of the participants, can still outperform the buyer from the open market in terms of profitability. If contractors breach a large proportion of contracted farmers, they will not be as profitable as the spot market-based trading, especially in the case of contract farming with ordinary rice. Besides that, the large-scale paddy field program is considered not a cost-effective method for contractors when an unreasonably high contract farming rate is offered. In the scenario of \(\lambda=30\%\) with ordinary rice, for example, the contractor incurs a high transaction cost to buy paddy from the participants and that negatively impacts on their earnings.
For results related to the ordinary rice, the contractors earn the most profit when low contract farming rates are offered to participating farmers. However, unattractive paddy purchasing prices result in the limitation in expanding the contract farming scheme as well as fewer small-holder farmers gaining benefits from the program. The lower left heat map in Figure 7 indicates that if the contractors offer attractive contract farming prices at rates (\(\lambda\) ranging from 20% to 30%) higher than the spot market price, more farmers are willing to participate in the large-scale paddy field program. These contractors, especially those who are committed, eventually are able to fulfill on average 96% of the total rice demand, even though they earn less profit compared to scenarios in which lower contract rates are proposed. Dishonest contractors achieve a significantly lower rice volume, particularly when unattractive purchasing prices (\(\lambda\) ranging from 0% to 10%) are offered or a large percentage of contracted farmers (\(\theta_C =30\%\)) are breached.
Similar observations can be seen on results related to the jasmine rice. Honest contractors with \(\lambda\) = 20% could stably establish their relationship with farmers to attain 96% of the maximum required volume. The results also show that contractors who are untrustworthy to 30% of the contracted farmers are still able to attain a certain volume of rice through their scheme and outperform the spot market-based buyer in terms of profit. Since jasmine rice is of high productivity, the contractor could initiate agricultural contracts with new members among the 3000 farmers initially setup in the model to fulfill their demand. As displayed in the top right heat map of Figure 7, contractors with jasmine rice might earn the most profit when they offer the typical rates currently set in the MKD region, i.e., \(\lambda\) = 10%.
Figure 8 shows the profitability of contractors who are not equipped with rice processing facilities in their contract farming scheme, in comparison with the open market-based buyer. The results indicate that the large-scale field program with either ordinary or jasmine rice is not a cost-effective agricultural production method. The profit acquired by exporting high-quality rice could not compensate the supporting services to contracted farmers and operational activities. In most of the scenarios, the incompetent performance of contract rice farming leads to non-viable business for large-scale contractors and discourages them to continue with the program.
Discussion and Future Work
In this paper, we presented an agent-based model to investigate three obstacles of the expansion of contract rice farming in the MKD region from the perspective of large-scale contractors. We focused on financial incentive and trust factors, which affect the decision of relevant parties in engaging and honoring the contracting agreement. The simulation model was designed in the context of the MKD’s rice supply chain with two contractors engaging in the contract rice farming scheme alongside with an open market in which both parties can renege on the agreement. We then evaluated the performances of contractors with different combinations of scenarios related to the three obstacles.
Firstly, regarding the failure in building a long-term trust-based relationship, our results showed a significant reduction in the rice volume and profit achieved from the large-scale field program, if a contractor decides to dishonor a large proportion of the participants. The results also uncovered an interesting observation when the contractors choose to opportunistically break their contract with a relatively small proportion (e.g., 10%) of small-holder farmers. In this case, they could still earn more profit per crop than a spot market-based buyer. This observation might contribute to the explanation of the fact that contractors have been reported to renege contract obligations especially when they could obtain the same type of rice at a lower price over the spot market (Roberts & Khiem 2005; Dung 2014; UNIDROIT 2014; Vietnam Television 2017). While the Vietnamese Government has introduced legal frameworks for contract farming, such as Decision 80 and Decision 62, they need to be enforced effectively. The local authorities should be more proactive in establishing innovative arbitration and dispute resolution mechanisms beyond the judicial system to prevent even the slightest level of opportunistic behavior from related parties.
Secondly, the simulation results showed that fully-equipped contractors could successfully implement the large-scale paddy field program if they stay committed. Being trustworthy especially in the first few cropping seasons could help the contractors quickly improve their trust-based relationship with members. Committed contractors proposing a higher price are able to expand the contract farming scheme with a larger volume of rice but earn less profit per ton of rice. In contrast, contractors with a lower offer might obtain better earnings per ton of rice as well as higher profit in total per cropping season but they could not easily establish contractual agreements with more farmers. The better financial incentives possibly discourage those contractors with a lower paddy purchasing price to enlarge their large-scale model. Results here indicate that the contractors should consider a flexible pricing system in order to adapt to fluctuations in spot market prices, production seasons and crop values. This flexible price system, which can be attractive for both the contractor and the farmer, might lead to a more sustainable contracting program in the MKD’s rice supply chain.
Thirdly, we found that the contract farming scheme for both ordinary and jasmine rice is not a cost-effective method for contractors who are not equipped with husking, processing and polishing machines. The higher cost they incur to transform paddy into export-ready rice by utilizing external services prevents them from engaging in the contracting program. Our results are in agreement with the judgment of World Bank (2013). Since most of the contractors in the MKD region have limited rice processing facilities (Tran et al. 2013), the large-scale paddy field model might not be adopted by the majority of enterprises and could not be expanded sustainably. It is therefore necessary that contract farming is better integrated into government planning and budget allocations, to facilitate access to credit for large-scale contractors. They can enhance their rice processing capability and engage more effectively in the contracting program.
Additional experiments with four contractors and 6000 farmers showed that when more neighboring contractors exhibit untrustworthy behavior, a large buyer would be more tempted to lower the purchasing prices or opportunistically exploit contracted farmers in order to obtain much better profit per crop. In both cases, the contractors could still maintain their contract rice farming program and acquire up to an exporting volume of around 89% during the whole simulation steps. For future work, we hope to extend the current study by varying the number of contractors with different commitment levels and offered purchasing prices to examine the evolutionary dynamics of contract farming performance.
We also plan to integrate evolutionary game theory into our agent-based model in the near future. Instead of assuming agents having sufficient information about the environments to make an optimal decision in advance, we will model each agent with the ability to adapt their strategy through learning by imitation (Nowak & May 1992; Chiong & Kirley 2012). There are relevant studies (Han et al. 2015; Martinez-Vaquero et al. 2015; Han et al. 2017) looking into contractual commitments and proposing mechanisms to resolve cooperation issues with the use of evolutionary game theory. We hope to apply the same framework and examine whether the mechanisms could help to diminish opportunistic behaviors in the context of MKD contract rice farming.
Crop yield and production cost data of the contract rice farming scheme used in this study was based on statistics acquired from the An Giang Plant Protection Joint Stock Company. This company, which is a pioneer and one of the few successful rice contractors, is considered the best scenario under the contract rice farming scheme in the MKD region (World Bank 2013). Future research could focus on the variation in relevant parameter values of different companies or locations across the MKD region to further assess the performance of the large-scale paddy field model. Differences in model prediction could be validated by surveying local farmers and contractors in the corresponding subregion or by interviewing experts.
Last but not least, this agent-based model will be developed as a decision support system to evaluate enabling policies from the Vietnamese Government and local authorities related to the rice supply chain and contract farming in the MKD region. Similar decision supporting tools can be found in the work of Croitoru et al. (2014) and Medda et al. (2017). Different activities including the Government’s land consolidation program and support from farmer organizations toward the emergence of a large-scale paddy field will be assessed to maintain a sustainable contract farming model and improve the livelihood of millions of small-holder farmers in the MKD region.
Acknowledgements
We would like to thank Michael Maes and the anonymous reviewers for their insightful and constructive feedback. The first author, H. K. Nguyen, would like to acknowledge support from an Australian Government Research Training Program scholarship to study a PhD degree in Computer Science at The University of Newcastle, Australia. R. Chiong is the corresponding author of this paper. The third author, M. Chica, is supported through the Ramón y Cajal program (RYC-2016-19800)Notes
- We also tested for [0.01, 1.0] and [0.1, 0.5], and the results did not change significantly.
- We also tested for [0.01, 0.99], and the results did not change significantly.
References
AGROINFO (2017). Agricultural trading price database in Vietnam. http://agro.gov.vn/vn/nguonwmy.aspx. Accessed: 2017-12-01.
AMBEKAR, S., Kapoor, R. & Mehta, P. (2015). Structural mapping of public distribution system using multi-agent systems. Business Process Management Journal, 21(5), 1066–1090.
AN, L. (2012). Modeling human decisions in coupled human and natural systems: Review of agent-based models. Ecological Modelling, 229, 25–36. [doi:10.1016/j.ecolmodel.2011.07.010]
ASIAN Development Bank (2007). 30 cases of contract farming: An analytical overview. Asian Development Bank.
BARRETT, C. B., Bachke, M. E., Bellemare, M. F., Michelson, H. C., Narayanan, S. & Walker, T. F. (2012). Smallholder participation in contract farming: Comparative evidence from five countries. World Development, 40(4), 715– 730. [doi:10.1016/j.worlddev.2011.09.006]
BIJMAN, J. (2008). Contract farming in developing countries: An overview. Tech. rep., Wageningen University, Department of Business Administration.
BONABEAU, E. (2002). Agent-based modeling: Methods and techniques for simulating human systems. Proceedings of the National Academy of Sciences, 99(Supplement 3), 7280–7287. [doi:10.1073/pnas.082080899]
CAN, N. D. (2014). Understanding “Linking together the four house” in rice production and commerce - A case study of An Giang province, Vietnam and lessons learnt from Chiba prefecture, Japan. Tech. rep., Institute of Developing Economies, Japan External Trade Organization.
CHEN, K. Z., Reardon, T. A., Wang, J., Wong, L. & Dao, A. T. (2015). Rice value chains in China, India, Lao PDR, and Vietnam: 2012 Survey results, interpretations, and implications for policy and investment. Tech. rep., Asian Development Bank.
CHEN, X.-P. & Komorita, S. S. (1994). The effects of communication and commitment in a public goods social dilemma. Organizational Behavior and Human Decision Processes, 60(3), 367–386.
CHIONG, R. & Kirley, M. (2012). Effects of iterated interactions in multiplayer spatial evolutionary games. IEEE Transactions on Evolutionary Computation, 16(4), 537–555. [doi:10.1109/TEVC.2011.2167682]
CROITORU, M., Thomopoulos, R. & Tamani, N. (2014). A practical application of argumentation in French agrifood chains. In International Conference on Information Processing and Management of Uncertainty in Knowledge-Based Systems, (pp. 56–66). Springer.
DANNENBERG, A., Lange, A. & Sturm, B. (2014). Participation and commitment in voluntary coalitions to provide public goods. Economica, 81(322), 257–275. [doi:10.1111/ecca.12073]
DUNG, N. M. (2014). Contract farming and enforcement in Vietnam: A case study of the pineapple production contract between farm households and the Dong Giao Agro-Processing company in Ninh Binh province. Tech. rep., East Asian Development Network.
EATON, C. & Shepherd, A. (2001). Contract farming: Partnerships for growth. (No. 145). Food & Agriculture Org.
FAFCHAMPS, M. (2003). Market Institutions in Sub-Saharan Africa: Theory and Evidence. Cambridge, Mass.: The MIT Press.
FAO (2017). Vietnam rice production and export: http://www.fao.org/giews/countrybrief/country.jsp?code=VNM. Accessed: 2017-11-01.
FEDERGRUEN, A., Lall, U. & Simsek, A. S. (2015). Supply chain analysis of contract farming. Tech. rep., Working paper
GARVEY, P. R. (2008). Analytical Methods for Risk Management: A Systems Engineering Perspective. Chapman and Hall/CRC. [doi:10.1201/9781420011395]
GILBERT, N. (2008). Agent-Based Models. London: SAGE Publications, Inc.
GRIMM, V. & Railsback, S. F. (2011). Designing, formulating, and communicating agent-based models. In Heppenstall A.J. et al. (Eds.),Agent-Based Models of Geographical Systems, Berlin Heidelberg: Springer, pp. 361–377. [doi:10.1007/978-90-481-8927-4_17]
GULATI, R. (1995). Does familiarity breed trust? The implications of repeated ties for contractual choice in alliances. Academy of Management Journal, 38(1), 85–112.
HAN, T. A., Pereira, L. M. & Lenaerts, T. (2015). Avoiding or restricting defectors in public goods games? Journal of The Royal Society Interface, 12(103). [doi:10.1098/rsif.2014.1203]
HAN, T. A., Pereira, L. M. & Lenaerts, T. (2017). Evolution of commitment and level of participation in public goods games. Autonomous Agents and Multi-Agent Systems, 31(3), 561–583.
HANDAYATI, Y., Simatupang, T. M., Perdana, T. & Siallagan, M. (2017). A simulation of contract farming using agent based modeling. Journal of Operations and Supply Chain Management, 9(2), 28. [doi:10.12660/joscmv9n2p28-48]
HIGGINS, A. J., Miller, C. J., Archer, A. A., Ton, T., Fletcher, C. S. & McAllister, R. R. J. (2010). Challenges of operations research practice in agricultural value chains. Journal of the Operational Research Society, 61(6), 964–973.
HILLETOHH, P. & Lättilä, L. (2012). Agent based decision support in the supply chain context. Industrial Management & Data Systems, 112(8), 1217–1235. [doi:10.1108/02635571211264636]
HUH, W. T. & Lall, U. (2013). Optimal crop choice, irrigation allocation, and the impact of contract farming. Production and Operations Management, 22(5), 1126-1143.
IPSARD (2014). Rice value chain in the Mekong River Delta. Tech. rep., Institute of Policy and Strategy for Agriculture and Rural Development.
KEY, N. & MacDonald, J. (2006). Agricultural contracting: Trading autonomy for risk reduction. Amber Waves, 4(1), 26.
KHANH, H. N., Chiong, R., Chica, M. & Middleton, R. H. (2017). Agent-based simulation of contract rice farming in the Mekong Delta, Vietnam. In 2017 21st Asia Pacific Symposium on Intelligent and Evolutionary Systems (IES). IEEE. [doi:10.1109/IESYS.2017.8233574]
KHIEM, N. T. & Emor, S. (2005). Linking farmers to markets through contract farming. Markets and Development Bulletin. Central Institute for Economic Management (CIEM).
KLOS, T. B. & Nooteboom, B. (2001). Agent-based computational transaction cost economics. Journalof Economic Dynamics and Control, 25(3-4), 503–526. [doi:10.1016/S0165-1889(00)00034-8]
KREJCI, C. C. & Beamon, B. (2012). Modeling food supply chains using multi-agent simulation. In Proceedings of the 2012 Winter Simulation Conference (WSC). IEEE
KREJCI, C. & Beamon, B. (2015). Impacts of farmer coordination decisions on food supply chain structure. Journal of Artificial Societies and Social Simulation, 18(2), 19: https://www.jasss.org/18/2/19.html. [doi:10.18564/jasss.2727]
KREMMYDAS, D., Athanasiadis, I. N. & Rozakis, S. (2018). A review of agent based modeling for agricultural policy evaluation. Agricultural Systems, 164, 95–106.
LABARTHE, O., Espinasse, B., Ferrarini, A. & Montreuil, B. (2007). Toward a methodological framework for agent-based modelling and simulation of supply chains in a mass customization context. Simulation Modelling Practice and Theory, 15(2), 113–136. [doi:10.1016/j.simpat.2006.09.014]
LOC, V. T. T. & Son, N. P. (2013). Rice value chain in the Mekong Delta, Vietnam: Solutions for rice value added improvement and sustainable development. Tech. rep., Centre for ASEAN Studies.
LUKE, S., Cioui-Revilla, C., Panait, L., Sullivan, K. & Balan, G. (2005). MASON: A multiagent simulation environment. Simulation, 81(7), 517–527. [doi:10.1177/0037549705058073]
MARTINEZ-VAQUERO, L. A., Han, T. A., Pereira, L. M. & Lenaerts, T. (2015). Apology and forgiveness evolve to resolve failures in cooperative agreements. Scientific Reports, 5, 10639
MEDDA, F. R., Caravelli, F., Caschili, S. & Wilson, A. (2017). The implementation of the Uganda agent based model. In Collaborative Approach to Trade, (pp. 149–168). Springer. [doi:10.1007/978-3-319-47039-9_10]
MINOT, N. et al. (1986). Contract farming and its effect on small farmers in less developed countries. Tech. rep., Michigan State University, Department of Agricultural, Food, and Resource Economics.
MINOT, N. & Sawyer, B. (2016). 'Contract farming in developing countries: Theory, practice, and policy implications.' In Devaux, A., Torero, M., Donovan, J., Horton, D. (Eds.), Innovation for Inclusive Value-Chain Development: Successes and Challenges, Washington, D.C.: International Food Policy Research Institute (IFPRI), pp. 127-158.
NGAN, P. H., Dung, T. T. & Babu, S. C. (2015). Private extension provision in Vietnam: A case study of An Giang Plant Protection Joint Stock Company. In Knowledge Driven Development, (pp. 233–252). Elsevier.
NHAN, T. Q., Takeuchi, I. & Hoang, D. V. (2015). Rice contract farming - The potential key to improve rice growers’ income: A farmer level study in An Giang province. Environment Development and Sustainability, 11, 1.
NOOTEBOOM, B. (1999). Interfirm Alliances: International Analysis and Design. London: Routledge.
NOOTEBOOM, B. (2015). Agent-based simulation of trust. Handbook of research methods on trust, (pp. 65–74) North, M. J. & Macal, C. M. (2007). Managing business complexity: discovering strategic solutions with agent-based modeling and simulation. Oxford University Press. [doi:10.4337/9781782547419.00014]
NORTH, M. J. & Macal, C. M. (2007). Managing Business Complexity: Discovering Strategic Solutions with Agent-Based Modeling and Simulation. New York, NY: Oxford University Press.
NOWAK, M. A. & May, R. M. (1992). Evolutionary games and spatial chaos. Nature, 359(6398), 826–829. [doi:10.1038/359826a0]
OLIVEIRA, J. B., Lima, R. S. & Montevechi, J. A. B. (2016). Perspectives and relationships in supply chain simulation: A systematic literature review. Simulation Modelling Practice and Theory, 62, 166–191.
OXFAM Vietnam (2012). Agribusiness expansion in Vietnam. Tech. rep., Oxfam: http://www.oxfamblogs.org/vietnam/2012/08/20/agribusiness-expansion-oxfam-workshop-report/.
PETERSON, H. (2001). Strategic choice along the vertical coordination continuum. The International Food and Agribusiness Management Review, 4(2), 149–166.
PHAM, N. Q. & La, A. H. (2014). Household welfare and pricing of rice: Does the large-scale field model matter for Vietnam? In N. Pavcnik (Ed.), Trade Polices, Household Welfare and Poverty Alleviation: Case Studies from Virtual Institute Academic Network, (pp. 301–335). United Nations, Geneva: UNCTAD.
PROWSE, M. (2012). Contract Farming in Developing Countries: A Review, vol. 12. Citeseer
ROBERTS, M. & Khiem, N. T. (2005). Contract use and paddy quality in the rice supply chain An Giang province, Vietnam. In A. D. Bank (Ed.), Linking farmers to markets through contract farming, (pp. 21–27). Hanoi: Asian Development Bank.
SASAKI, T., Okada, I., Uchida, S. & Chen, X. (2015). Commitment to cooperation and peer punishment: Its evolution. Games, 6(4), 574–587
SHEPHERD, A. (2013). An introduction to contract farming. Technical Centre for Agriculturaland Rural Cooperation (ACP-EU), 29, 10–13.
SWINNEN, J. F. M. & Maertens, M. (2007). Globalization, privatization, and vertical coordination in food value chains in developing and transition countries. Agricultural Economics, 37, 89–102.
TRAN, C., Do, H. & Le, M. (2013). Who has benefited from high rice prices in Vietnam. Tech. rep., Oxfam, IPSARD, Hanoi.
UNIDROIT (2014). Legal policies and regulations governing contract farming in Vietnam. Tech. rep., International Institute for the Unification of Private Law.
VEPR (2015). Rice Marketin Vietnam- Reformand Integration: Market Structure Perspective. Hong Duc Publishing House.
VERWAART, T., Dijkxhoorn, Y., Plaisier, C. & van Wagenberg, C. (2016). Simulating the role of trust in supply development. In 12th Artificial Economics Conference, Rome, 20-21 September 2016.
VIET, H. C. (2015). Horizontal & vertical coordination in rice production in the Mekong Delta, Vietnam. Tech. rep., VNU University of Economics and Business and VEPR.
VIETNAM Farmer Union (2017). Difficulties in expanding large-scale contract rice faming. http://hoinongdan.org.vn/sitepages/news/37/50824/kho-nhan-rong-canh-dong-mau-lon. Accessed: 2017-11-01
VIETNAM Television (2017). Causes for the reduction of rice land with contract farming scheme. http://vtv.vn/trong-nuoc/nguyen-nhan-khien-canh-dong-mau-lon-giam-dien-tich-20170613131304204.htm. Accessed: 2017-11-01.
WILL, M. (2015). Contract Farming Handbook: A Practical Guide for Linking Small-scale Producers and Buyers Through Business Model Innovation, Volume II Selected Tools and Case Examples. GIZ
WORLD Bank (2011). Beyond the ’Rice Bowl’: Building on past gains to enhance the quality, sustainability, and equity of growth in the Mekong Delta. Tech. rep., World Bank, Washington DC: http://www.isgmard.org.vn/vn/VHForums/VHViewTopic.asp?id=4.
WORLD Bank (2013). Vietnam - support for agricultural restructuring project: The financial and economic competitiveness of rice and selected feed crops in northern and southern Vietnam. Tech. rep., World Bank, Washington DC.: http://documents.worldbank.org/curated/en/278271468127185450/Vietnam-Support-for-Agricultural-Restructuring-Project-the-financial-and-economic-competitiveness-of-rice-and-selected-feed-crops-in-northern-and-southern-Vietnam.
ZIMMERMANN, A., Heckelei, T. & Domínguez, I. P. (2009). Modelling farm structural change for integrated ex-ante assessment: Review of methods and determinants. Environmental Science & Policy, 12(5), 601–618. [doi:10.1016/j.envsci.2009.01.014]