Introduction
Both tacit and explicit knowledge have been identified as important key resources shaping the behavior and competitiveness of firms (Conner 1991; Easterby-Smith & Lyles 2003). Classic organization theory argues that organizations are composed of individuals. Thus, organizations cannot learn by themselves and learn only through their members (Cyert & March 1963; March 1991; Miller et al. 2006). Meanwhile, individuals adjust their beliefs (knowledge) based on organizational knowledge (March 1991). Thus, organizational knowledge both shapes and is shaped by individuals’ knowledge (Miller et al. 2006). In general, organizations embed past knowledge into their organizational memory, which is stored in and retrieved from the following two types of “retention bins”: employees and documents (Walsh & Ungson 1991). However, forgetfulness occurs when knowledge is lost over time (Darr et al. 1995), which is typically due to employee turnover (Easterby-Smith & Lyles 2011; Levitt & March 1988), loss or destruction of archival records, knowledge obsolescence (Argote 2012), and individual forgetting (Blaschke & Schoeneborn 2006). This study focuses on individual forgetting within the setting of organizational forgetting.
Individuals who create, transfer and retain knowledge in an organization (Xu et al. 2014) are subject to forgetting (Holan & Phillips 2004; Blaschke & Schoeneborn 2006; Kluge & Gronau 2018), which is caused by the actual loss and decay of knowledge from individual memory (Jolly & Wakeland 2009). Therefore, individual knowledge evaporates over time, and forgetting is generally regarded as an unfavorable factor in organizational knowledge management. In particular, some scholars have noted that the nature of individual forgetting and the underlying processes that drive forgetting are key issues that need more attention in the continued development of organizational knowledge management (Holan & Phillips 2004). Therefore, attempts to manage organization knowledge must also include the management of individual forgetting.
Individual forgetting, representing the decay or depreciation of knowledge, likely diminishes organizational effectiveness (Argote 2012, chapter 2). Despite the efforts exerted by many scholars studying individual forgetting, only a few scholars in the management field have focused on the impact of forgetting on organizational performance. Blaschke & Schoeneborn (2006) first extended March’s (1991) model by introducing forgetting to explore the effects of forgetting on the organizational knowledge level. Based on March’s model extension, Miller & Martignoni (2016) further examined how forgetting regulates the tradeoff between exploration and exploitation in organizational learning. Although these two studies explore the mechanisms by which forgetting affects organizational learning performance, only a part of the story of forgetting in knowledge management has been told, and how to manage forgetting by adopting different knowledge management strategies remains unclear. Because of this gap, this study focuses on management strategies for individual forgetting on the basis of previous studies in an attempt to solve the following two interrelated issues: (1) What knowledge management strategies exist and can they effectively manage individual forgetting? (2) Are there any differences in the effects of different knowledge management strategies in managing individual forgetting? To answer these questions, consistent with Miller & Martignoni’s (2016) research, individual forgetting is considered, and classical knowledge management strategies are introduced to construct an agent-based simulation model by extending March’s model to investigate the joint impacts of forgetting and different knowledge management strategies on the organizational knowledge level. A series of simulation experiments are performed, and the results provide insight into the management of individual forgetting in theory and may help business managers design knowledge management strategies in practice to achieve higher efficiency and effectiveness in managing individual forgetting.
The remainder of the paper is organized in four parts. First, we review related work in the literature related to forgetting and knowledge management strategies. Second, we construct an agent-based simulation model that parameterizes several knowledge management strategies and the degree of forgetting. Third, we carry out a series of simulation experiments and analyze the simulation results separately. Finally, we discuss our conclusions.
Related Work
Forgetting
Since memory is the carrier of individual knowledge retention, memory has been studied by scholars for some time, especially in connection with organizational learning and knowledge management (Levitt & March 1988). Scholars have found that memory is a process that is largely concerned with the following two asymmetric functions: remembering and forgetting. These two processes simultaneously create a shared version of the past to be used in the present. However, the primary function of memory is forgetting rather than remembering (Blaschke & Schoeneborn 2006) because agents free their memory capacity for new knowledge only if they forget prior knowledge (Dittrich et al. 2002). Therefore, individual forgetting is a core issue that cannot be ignored in knowledge management and organizational learning (Zhang & Zhou 2009).
The question of whether forgetting is due to interference or natural decay has attracted considerable attention among early researchers. Jenkins & Dallenbach (1924) designed a classic experiment by comparing memory for nonsense syllables when subjects slept through the retention interval compared to when they remained awake, and the results showed that subjects recalled more items when they slept than when they remained awake, supporting interference theory. Although scholars believed that the version of decay theory that attributed a causal role to the passage of time is conceptually flawed, decay theory has not been abandoned. McGeogh (1932) proposed an obvious possibility that cannot be ruled out and formed a more reasonable decay theory, which posits that forgetting is due to the deterioration of organic traces due to natural metabolic processes. This version of decay theory was widely adopted in subsequent cognitive psychology and knowledge management. Researchers in the field of cognitive psychology (Hulse et al. 1975) defined forgetting as the following two effects: the actual loss of knowledge from individual memory and knowledge decay over time (Jolly & Wakeland 2009). Scholars in the field of knowledge management believe that forgetting results from the actual decay of knowledge from individual memory because individual forgetting is generally regarded as a psychological process in which individuals’ recollection of specific knowledge embedded in their memory deteriorates over time (Darr et al. 1995; Wixted 2004). Based on this view, Blaschke & Schoeneborn (2006) first considered forgetting in organizational learning and described the process of individual forgetting in a more specific way; these authors regarded individual forgetting as the probability of converting a belief into a nonbelief under the condition that the belief of individuals does not match the organizational code norms. Inspired by this study, Miller & Martignoni (2016) extended beyond the precedent by defining the forgetting of individuals independent of the organizational code, suggesting that forgetting occurs to convert a nonzero belief to a zero regardless of the matching relationship between an individual’s belief and organizational code norms. Our study draws upon Miller & Martignoni's (2016) definition of forgetting because forgetting is believed to be rooted in human nature. Therefore, in our model, individuals learn and forget independent of the organizational code.
Knowledge management strategy
To better manage knowledge resources in organizations, firms adopt some knowledge management initiatives (Davenport & Prusak 1998; Alavi & Leidener 2001; Chennamaneni 2007). This so-called knowledge management includes the explicit and systematic management of vital knowledge and its associated processes of creation, organization, diffusion, use and exploitation (Skyrme 2000). Thus, knowledge management is based on building an organization’s ability to acquire, organize and diffuse knowledge throughout the organization to improve the organization’s effectiveness, efficiency and competitiveness (Alavi & Leidner 1999).
So far, scholars have proposed different types of strategies for managing knowledge. Scholars represented by March (1991) believed that the core strategy of knowledge management is the maintenance of a balance between exploration and exploitation by firms. Moreover, they have proposed the following two orientations of knowledge management: conservative and aggressive (Zack 1999). Firms oriented toward exploiting internal knowledge exhibit the most conservative knowledge strategy, while unbounded innovators (those who closely integrate knowledge exploration and exploitation without regard to organizational boundaries) represent the most aggressive strategy. However, some scholars believe that a key enabler of knowledge management is knowledge sharing (Nonaka & Takeuchi 1995); thus, a firm must develop an effective knowledge sharing process and encourage its employees to share knowledge (Bock & Kim 2002). Hansen et al. (1999) proposed the following two knowledge management strategies (KMSs), which are commonly used by successful organizations to share knowledge: personalization and codification. In personalization, knowledge is closely tied to the person who developed the knowledge and is shared mainly through social networking between individuals (Jasimuddin & Zhang 2009), whereas in codification, knowledge is extracted from the person and stored in organizational files, and anyone in the organization can access and use the codified knowledge without having to contact the person who originally developed such knowledge.
This paper draws upon the KMS typology proposed by Hansen et al. (1999) as it is well known and widely accepted in the field of knowledge management, and the concepts of personalization and codification are easily understood by academicians and practitioners. In addition, considering that some companies still use both codification and personalization approaches, we introduce a mixed strategy by mixing codification and personalization.
The Model
March (1991) presented the following classic agent-based model demonstrating the reciprocal nature of learning within organizations: organizations learn from individuals, and individuals learn from organizations. This model has received widespread attention, and many modelers have extended this model in different ways. For example, Miller et al. (2006) added direct interpersonal learning, allowing knowledge transfer among persons and between person and documents to be considered in the model. Kane & Alavi (2007) allowed agents to learn directly from their team members or through an information system consisting of knowledge repositories and communication technologies. Fang et al. (2010) examined learning among agents situated in semi-isolated groups within an organization.
Although prior models consider learning at different speeds, such models omit other key aspects of human memory, which is limited to current learning and has a tendency to forget the past. Therefore, some scholars began to incorporate forgetting into March’s model. For example, Blaschke & Schoeneborn (2006) first extended March’s (1991) model by introducing forgetting. Miller and Martignoni (2016) modeled organizational learning based on March’s model with forgetting and derived some novel implications. Wu et al. (2019) examined the effects of BIET (entrepreneurial teams in business incubators) learning, forgetting and exit and entry on the knowledge level. These studies provide a theoretical basis for this study. To retain the major features of these studies, the model with forgetting based on March’s model was further expanded by introducing three KMSs.
Specification
Following March’s (1991) work on organizational learning, our model is as follows.
Environment. In our model, an external reality independent of the organization members’ beliefs exists and is modeled as an m-dimensional vector. Each dimension is randomly assigned a value of 1 or -1 with equal probability.
Organization member. The modeled organization consists of n individuals, each of whom holds beliefs about each of the m dimensions of the environment. Individuals’ beliefs are represented by values of 1, 0, or -1. A value of 1 or -1 indicates commitments to particular beliefs about reality, whereas 0 reflects the absence of a belief about a particular dimension of the environment.
Similar to Miller & Martignoni’s (2016) study, the y dimensions randomly selected for each individual are initially assigned nonzero beliefs with equal probability, and the remaining m - y dimensions are assigned zero beliefs. The default value of y is set to m/2.
Forgetting. Followed Miller & Martignoni’s (2016) study, forgetting is modeled as a nonzero belief of an individual and replaced by a value of 0 at each dimension with probability f.
Knowledge management strategy (KMS). Our model considers the following three types of KMSs: personalization strategy at the horizontal level, codification strategy at the vertical level, and a mixed strategy that combines the codification and personalization strategies.
The codification strategy (CS) refers to encoding and storing knowledge by using an organizational code that represents the dominant beliefs among organization members, which is also modeled as an m-dimensional vector. The dimensions of the organizational code are initially assigned zero beliefs, although they are modified by replacing (with probability POI) the corresponding belief of the code with the dominant belief of the individuals who have a higher knowledge level than the organization code (Miller et al. 2006). Meanwhile, we define two scenarios based on whether the organizational code is provided as feedback to individuals. When feedback regarding the organizational code is provided to individuals, each individual can learn from the dimensions whose value is not 0 in the organizational code by replacing each belief with a corresponding belief of the code with probability POI; we set POI=0.5 by default and call this scenario YFB (Yes Feedback). When individuals do not receive feedback regarding the organizational code, individuals do not learn from the organizational code; we set POI=0 by default and call this scenario NFB (No Feedback).
The personalization strategy (PS) refers to developing networks among members in an organization allowing knowledge to be transferred effectively. Similar to Lazer & Friedman’s (2007) study, the individuals are located in a random network with average degree equals to 4, which has the same average degree as Miller et al.’s (2006) grid network. The generation of random networks is first to generate a ring with \(n\) nodes, each of which has links with its 4 neighbours on either side, and then each link is reconnected to another node that is randomly picked. Each individual in the random network searches for the linked neighbor who has higher performance than the rest neighbors and herself, and learns form this neighbors by copy each belief of the neighbors with probability PII.
The mixed strategy (MS) refers to the simultaneous implementation of the PS and CS. The MS allows agents to learn from other individuals while establishing an organizational code for knowledge encoding that can also be learned from by agents based on whether feedback occurs.
Outcome measures
Individuals can hold both correct and incorrect beliefs about reality (March 1991; Miller et al. 2006). Each dimension of an individual’s beliefs that equals (does not equal) the corresponding dimension of reality suggests that the dimension holds correct (incorrect) knowledge. March (1991) computed individuals’ knowledge as a proportion of their correct beliefs. However, Miller et al. (2006) defined an individual’s knowledge level (IKL) as the number of correct beliefs that match reality minus the number that do not match divided by m. In our model, we use Miller et al.’s approach because the performance of random initial beliefs is near 0 on average.
The organizational knowledge level (OKL) is typically evaluated by two criteria. One criterion is the average knowledge level of the organization members (AKL), which is defined as the average of all individuals’ knowledge. The other criterion is the knowledge level of the organizational code (CKL), which is calculated based on the calculation method of the individual knowledge level. In general, the larger of AKL and CKL is generally chosen to represent the organizational knowledge level (OKL).
Analysis and Results
A series of simulation experiments with different parameters (see Table 1) were carried out using NetLogo. Similar to many previous studies related to March’s model, the default values were m=100 (Miller et al. 2006; Miller & Lin 2010), n=100 (Miller & Lin 2010; Kane & Alavi 2007), and POI=0.5 (Xu et al. 2014). However, even if we change the values of m (m=50 and m=150), n (n=50 and n=150), and POI (POI=0.4 and POI=0.6), the results remain consistent.
Each experiment run was 200 periods as the results showed that all experiments needed less than 200 periods to reach equilibrium. In addition, each experiment was iterated 100 times to avoid stochastic effects. The average of the last-period data over 100 experiments was used as the basis for the analysis.
We began our analysis by separately exploring the impacts of different KMSs and forgetting on the organizational knowledge level. Then, we compare the effects of three KMSs in managing forgetting.
Parameter | Values | Meaning |
m | 100 | Number of reality domains |
n | 100 | Number of individuals |
y | m/2 | Initial number of nonzero beliefs |
POI | 0.5 | Rate of learning by organization code from individuals |
PIO | 0, 0.5 | Rate of learning by individuals from organization code |
PII | 0, 0.1, 0.2, 0.3, 0.4, 0.5, 0.6, 0.7, 0.8, 0.9, 1 | Rate of interpersonal learning |
f | 0, 0.05, 0.1, 0.15, 0.2 | Rate of forgetting |
KMS | CS, PS, MS | Knowledge management strategy |
FB | YFB (PIO=0.5), NFB (PIO=0) | Receiving or not receiving feedback |
Effects of forgetting and the personalization strategy
The PS is a decentralized knowledge management approach, and its application is adjusted by the interpersonal learning rate. Figure 1 illustrates the effects of the interpersonal learning rate (PII) and forgetting (f) on the AKL. Previous scholars have found that PII exhibits an inverted-U relationship with AKL, suggesting that maintaining a moderate interpersonal learning rate is conducive to improvement in the organizational knowledge level. This paper draws the same conclusion and further finds that forgetting adjusts the interpersonal learning rate at the optimal AKL. When f is small, the PII that achieves the optimal AKL is smaller. As f increases, the PII that achieves the optimal AKL increases accordingly because when f is low, organizations should focus on exploration rather than exploitation due to the limited negative effects of forgetting; thus, maintaining a lower rate of interpersonal learning is beneficial for the long-term performance of organizations. When f is high, individual knowledge is lost faster. However, appropriate improvement in the interpersonal learning rate at this time can alleviate the loss of individual knowledge to a certain extent through interactive memory and maintain the long-term exploration of an organization. Therefore, for the PS to be effective, it is necessary to identify the optimal interpersonal learning rate based on the forgetting rate to maximize the organizational knowledge level.
Figure 1 also shows that when PII is less than 0.9, greater f corresponds to lower AKL. When PII is greater than 0.9, greater f corresponds to greater AKL. This result indicates that forgetting is not always harmful and can slow the speed of knowledge convergence by decreasing knowledge in some dimensions in organizations in excessive exploitation resulting from a very high interpersonal learning rate, thus maintaining the diversity of knowledge within the organization, which helps individuals extend their search for novel knowledge and increases the possibility of finding better knowledge.
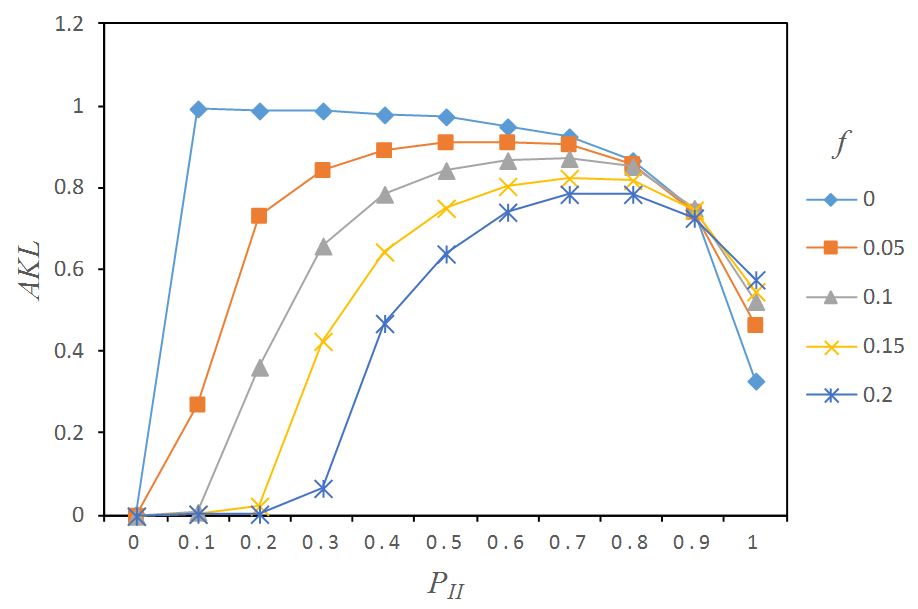
Effects of forgetting and the codification strategy
The CS is a centralized knowledge management approach, and the result of knowledge coding is represented by an organizational code. Therefore, the question that organizations must consider in CS is whether it is necessary to feed back the organizational code to members because previous studies have shown that this affects the balance of exploration and exploitation within organizations.
Figure 2 illustrates the effects of forgetting (f) and feedback (FB) on the AKL and CKL. Unsurprisingly, AKL equals 0 or decreases gradually as f increases, which is consistent with the general understanding that forgetting makes individuals lose knowledge continuously. However, forgetting is not always negatively correlated with CKL, which is influenced by organizational code feedback. As we can see in Figure 2, the yellow line called CKL-YNB shows a trend to first increase and then decrease. In other words, forgetting exhibits an inverted U-shaped relationship with CKL when the organizational code is fed back to organization members because a proper improvement in forgetting when f is low does not have a greater impact on individual knowledge but can introduce more knowledge diversity to the organization, which is good for the long-term adaptability of the organization and results in some improvement in CKL. A high f could seriously damage the knowledge structure of individuals and directly affect the performance of the organizational code. Thus, CKL continues to decrease as f increases.
Figure 2 shows that CKL is always better than AKL under the same situation (with or without feedback), indicating that the CS can help organizations retain knowledge and reduce the adverse effects of forgetting to some extent. However, CKL is not always better than AKL in different situations (comparison of with-feedback and without-feedback conditions) depending on the forgetting level. If f is low, AKL with feedback is superior to CKL without feedback, and the opposite result is obtained when f is high, suggesting that whether the organizational code is provided as feedback does not affect the application effect of the CS. Therefore, the boundary condition that determines whether the organizational code is provided as feedback must be identified.
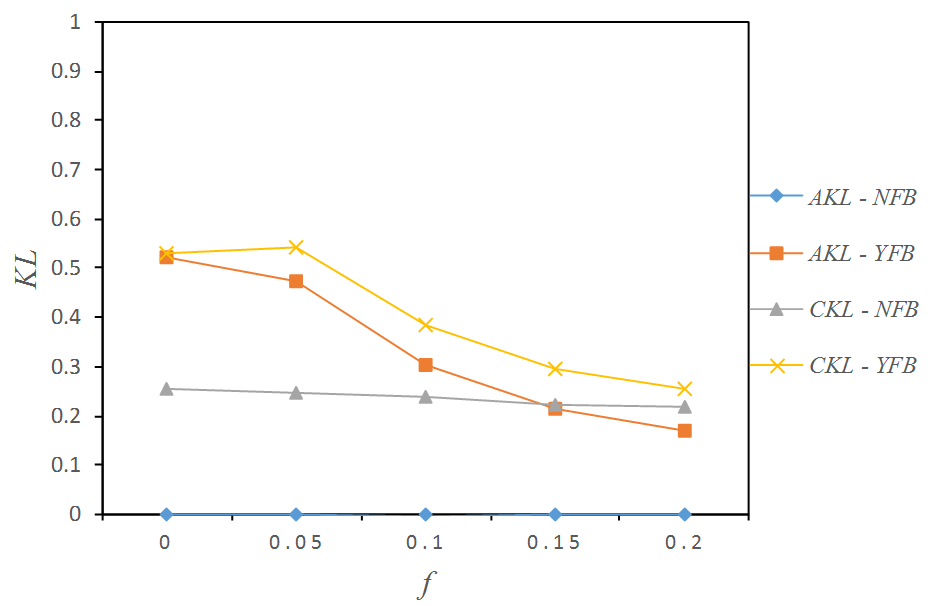
Effects of forgetting and the mixed strategy
The effects of the MS that integrates centralized and decentralized knowledge management approaches are explored in this section. We first examined the joint effects of the interpersonal learning rate (PII) and forgetting (f) on the CKL and AKL, and the results are shown in Figure 3. We found that regardless of whether the organizational code is fed back to members, PII always exhibits an inverted-U relationship with CKL and AKL and is not affected by the forgetting rate. However, forgetting adjusts the interpersonal learning rate at the optimal CKL and AKL. If f is small, the PII that achieves the optimal CKL and AKL is small, although it could increase accordingly as f increases. More importantly, comparing Figure 3a with 3b or Figure 3c with 3d, when the forgetting rate is relatively high, the PII that achieves the optimal AKL or CKL is further reduced as feedback regarding the organizational code is provided to members because the feedback regarding the organizational code further accelerates knowledge convergence, thus reducing knowledge diversity. Therefore, reducing the interpersonal learning rate can slow the speed of knowledge convergence, which promotes the maintenance of the balance of exploration and exploitation and achieves a higher AKL and OKL in the long term.
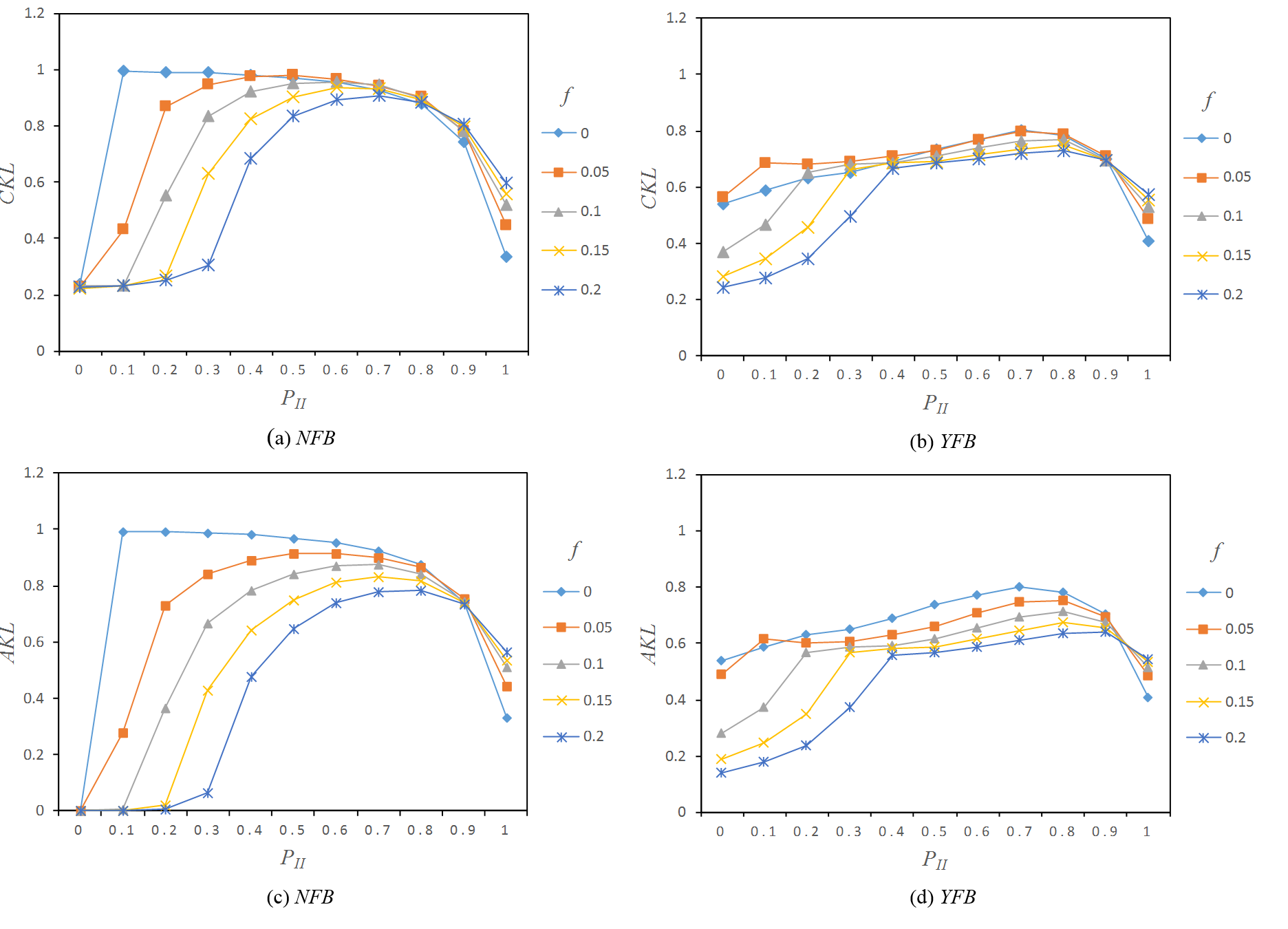
Second, we examined whether CKL is always better than AKL within the MS. Figure 4 illustrates the effects of the interpersonal learning rate (PII ) and organizational code feedback (FB) on AKL and CKL with a different f. As observed in Figure 4, the CKL is always better than the AKL under the same situation. However, similar to the CS, the CKL is not always better than the AKL in different situations. When f is low (f=0.05 and f=0.1) and PII is low, the AKL with feedback is superior to the CKL without feedback; however, when f is low (f=0.05 and f=0.1) and PII is high, the AKL without feedback is better than the CKL with feedback. In the case of high forgetting (f=0.15 and f=0.2), AKL with feedback is only superior to CKL without feedback when PII is within a proper range; moreover, AKL without feedback is better than CKL with feedback when PII is high. These results indicate that when an organization applies the MS, it must focus not only on the boundary conditions of whether the organizational code is provided as feedback but also the impact of the interpersonal learning rate and forgetting rate.
In addition, Figure 4 shows that when PII is less than a critical value r that refers to a value of PII corresponding to the intersection of the line of CKL-YFB (AKL-YFB) and CKL-NFB(AKL-NFB) in Figure 4, the CKL (AKL) with feedback is always better than the CKL (AKL) without feedback, and when PII is greater than critical value r, the CKL (AKL) without feedback is superior to the CKL (AKL) with feedback. Thus, when PII is low, the organizational code should not be fed back to members, but the opposite is true when PII is high. Simultaneously, the value of r is regulated by f, and a greater f corresponds to a greater value of r because higher forgetting introduces more knowledge diversity and, thus, directly destroys the balance the organization has already achieved, whereas a corresponding increase in the rate of interpersonal learning accelerates knowledge convergence and eventually enables the organization to achieve a new balance between exploration and exploitation.
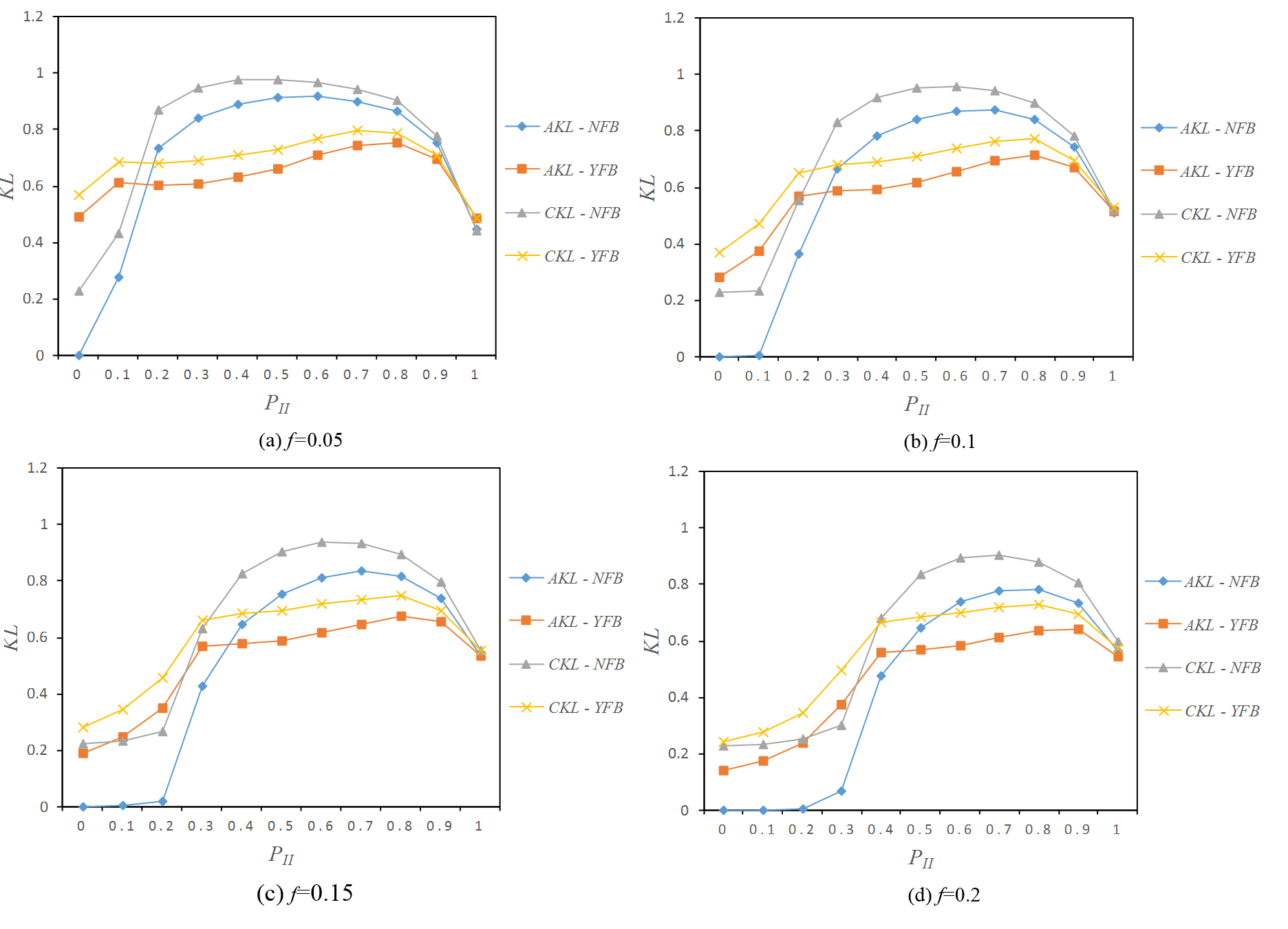
Comparison of the three knowledge management strategies
This section mainly discusses how to choose a suitable KMS based on forgetting. To further compare the three KMSs, AKL is used to represent the organization knowledge level (OKL) in the PS, and CKL is chosen to represent the OKL in the CS and MS because the above studies showed that CKL is always better than AKL in the same situation.
The analysis shown in Figure 5 addresses the joint effects of forgetting (f) and KMSs on the OKL with different pII. Notably, in the extreme case of no individual forgetting, the PS always performs as well as the MS without feedback, except for when PII is very high (PII=1). However, when individual forgetting exists, the three influencing patterns of forgetting (f) and KMSs on the OKL with different PII are shown in Figure 5. (1) When PII is low (PII=0.1 and PII=0.3), as shown in Figure 5(a) and 5(b), the MS without feedback performs best when f is relatively low, and the MS with feedback performs best when f is relatively high. (2) When PII is relatively high (0.5=PII<=0.9), as shown in Figure 5(c), 5(d) and 5(e), the MS without feedback always performs best. (3) When PII is very high (PII=1), as shown in Figure 5(f), under different degrees of forgetting, different KMSs must be selected. If f is low, the CS with feedback performs best; if f is moderate, the MS with feedback performs best; and if f is high, the MS without feedback performs best.
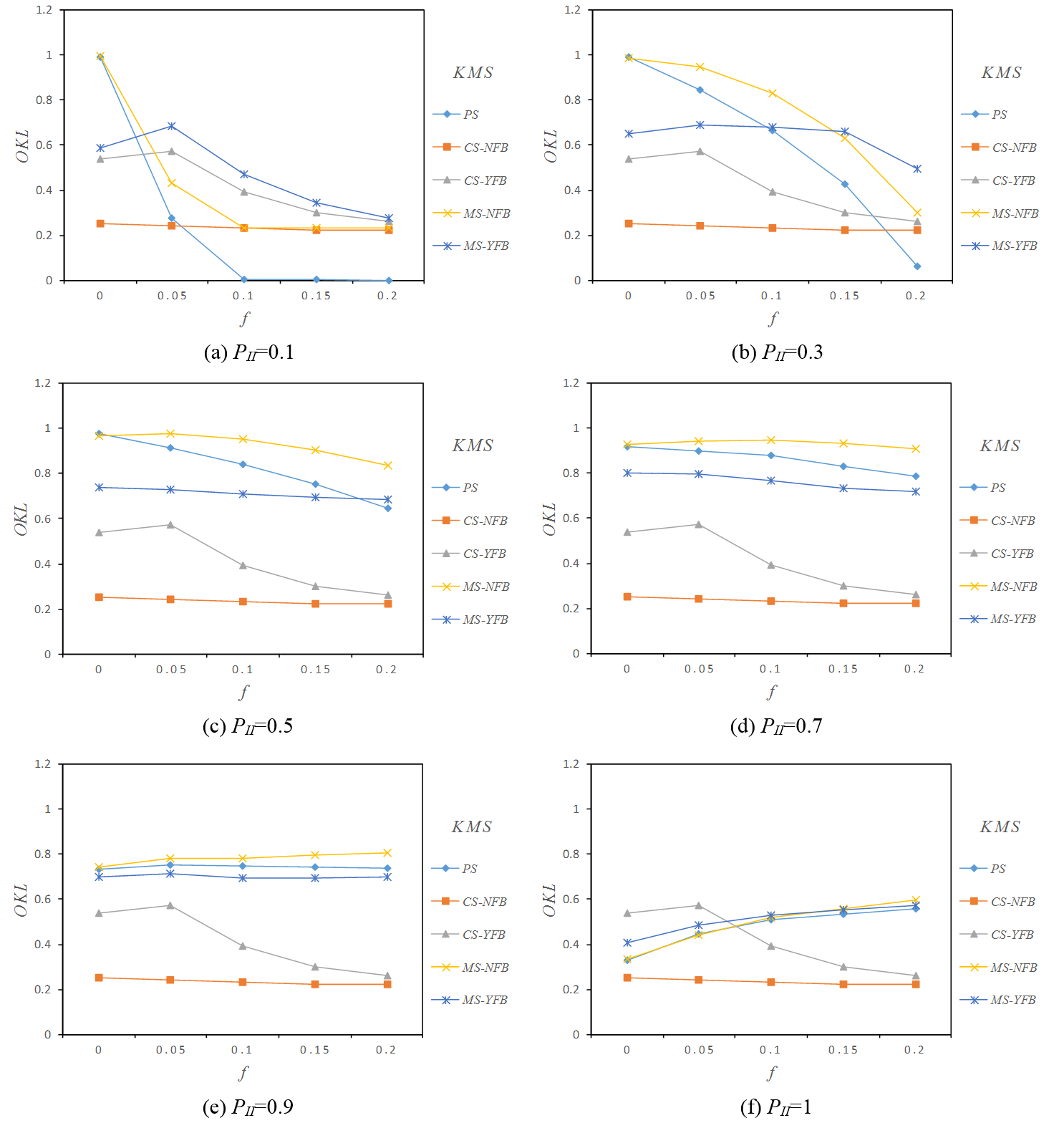
Discussion
Main conclusions
Managing forgetting is a major activity in enterprise knowledge management. The considerable amount of effort and time that such management requires are comparable to those allocated to other traditional components of knowledge management, such as knowledge acquisition and transfer. Therefore, an agent-based simulation based on March’s model was constructed by introducing forgetting and different KMSs (codification, personalization, and mixed) to analyze and compare the effects of different KMSs in managing individual forgetting to answer the following two questions: whether each KMS can effectively manage individual forgetting and whether any differences exist in the effects of the different KMSs in managing individual forgetting.
First, the simulation results showed that the three KMSs are effective for managing individual forgetting. Specifically, regarding the PS, which was affected by the interpersonal learning rate, the interpersonal learning rate exhibits an inverted-U relationship with the AKL of organization members; when the interpersonal learning rate is very high, the individual forgetting rate is greater, and the AKL of organization members is higher, thus implying that the PS can effectively manage individual forgetting. Regarding the CS, its application is affected by whether the organizational code is provided as feedback to organization members, whereas the CS is always beneficial regardless of forgetting with and without feedback. Regarding the MS, which is simultaneously affected by the interpersonal learning rate and whether the organizational code is provided as feedback to organization members, the interpersonal learning rate exhibits an inverted-U relationship with the AKL of organizational members and the knowledge level of the organizational code. Regardless of whether feedback is provided, when the interpersonal learning rate is very high, individual forgetting is greater, the AKL of organization members is higher, and the knowledge level of the organizational code is higher. Thus, the MS is always beneficial regardless of forgetting in cases with and without feedback.
Second, the simulation results showed that certain factors, such as the interpersonal learning rate, forgetting rate and whether the organizational code is provided as feedback, are key factors affecting the choice of KMSs. For an enterprise to better manage individual forgetting, it is necessary to choose different KMSs according to different conditions. In the extreme case of no individual forgetting, both the PS and MS without feedback can be adopted in most scenarios. However, when individual forgetting exists, different KMSs should be chosen under different conditions. Specifically, (1) when the interpersonal learning rate is relatively low, if forgetting is low, the best choice is to adopt the mixed strategy without feedback, and if forgetting is high, the best choice is to adopt the MS with feedback. (2) When the interpersonal learning rate is relatively high, the best choice is to adopt the MS without feedback regardless of forgetting. (3) When the interpersonal learning rate is very high, if forgetting is low, the best choice is to adopt the CS with feedback; if forgetting is moderate, the best choice is to adopt the MS with feedback; and if forgetting is high, the best choice is to adopt the MS without feedback.
Theoretical contribution
The theoretical contribution of this study can be summarized as follows.
Enriching individual forgetting research in organization learning. An agent-based simulation model was first proposed by March in 1991 to demonstrate the organizational learning process, and the simulation results showed that organizations must maintain a balance between the exploration of novel knowledge and the exploitation of known knowledge to gain superior knowledge and maintain strong adaptability in the long run. March’s model has been widely accepted, and some scholars have expanded this model from different perspectives. However, these models extending the work conducted by March generally ignore that the amount of knowledge that each individual can acquire and recall is limited. Inspired by this gap, Blaschke & Schoeneborn (2006) first incorporated individual forgetting into March’s model and simultaneously modeled organizational code with forgetting and individual forgetting, where individual forgetting depends on the code norms. Recently, Miller & Martignoni (2016) further studied individual forgetting based on March’s model by considering the context of interpersonal learning that exists among organization members. These studies focus on the role of individual forgetting in balancing exploration and exploitation in organizational learning and reveal that forgetting relentlessly diminishes individuals’ knowledge and that their learning rate needs to more than offset this ongoing erosion of beliefs; however, these studies provide some methods to only partially manage forgetting. Focusing on this research gap, this paper further discusses the organizational learning model with forgetting and systematically reveals the management strategies of individual forgetting, thus enriching previous studies investigating individual forgetting in organization learning.
Greater insight into individual forgetting. Compared with existing research related to individual forgetting, this study systematically reveals the double-sided effect of individual forgetting. On the one hand, forgetting is considered a detrimental outcome of the failure to retrieve and retain known knowledge, resulting in the loss and decay of individual knowledge. On the other hand, forgetting directly affects the diversity of knowledge, which helps extend exploration within the organization and increases the likelihood that the organization discovers new knowledge. However, the ultimate effect of forgetting depends on the balance between exploration and exploitation within the organization. In general, the positive effect of individual forgetting is greater than the negative effect on an organization with overexploitation because forgetting slows the convergence to consensus and impedes exploitation. In fact, some scholars in the organizational learning field argue that organizations are usually inclined toward overexploitation (March 1991; Groysberg & Lee 2009). Thus, nonintervention to individual forgetting may be beneficial for organizations to reach a new balance between exploration and exploitation in an economical way.
Enriched understanding of knowledge management strategies. By studying 25 different firms, including consulting and healthcare firms, Hansen et al. (1999) first summarized the following two approaches of KMSs: codification and personalization. Many subsequent studies have mentioned and/or discussed these two strategies. The research discussed in this paper enriches our understanding of KMSs in three main ways. First, this study introduced individual forgetting to research focusing on CS and PS for the first time. This variable, which has been ignored by previous research, may be an important factor in the application of these strategies. Second, some studies have discussed the choice of strategy. For example, Hansen et al. (1999) noted that a company’s choice of strategy is far from arbitrary and depends on the way the company serves its clients, the economics of its business, and the people it hires. Liu et al. (2013) provide a systematic framework for organizations to analyze their knowledge reuse processes and balance the CS and PS within their knowledge strategy according to a cost/benefit analysis. However, these studies share the common assumption that the CS must provide feedback regarding the encoding knowledge to the members. This paper compared the organization’s knowledge level in both feedback and non-feedback situations and drew different conclusions, thus providing a new basis for the choice of strategy. Finally, in recent years, many studies have revealed that organizations should adopt an MS (Greiner et al. 2007; Ajith Kumar & Ganesh 2011). The conclusions of this paper are that adopting an MS is not always beneficial and that the CS is better in the case of a higher individual interpersonal learning rate and lower forgetting. Thus, these findings deepen our understanding of the application scenarios for an MS.
Identify strategies for managing individual forgetting. Scholars generally believe that organization knowledge is composed of the individual knowledge of employees and documented knowledge filed in a company’s property (Globerson 1987). Much of the organization knowledge is embrained or embodied in individuals (Blackler 1995), although organizations provide the context in which members’ memories operate (Paoli & Prencipe 2003). Therefore, it is more important to pay attention to individual forgetting. Previous studies have emphasized that forgetting is an important dilemma in organizational knowledge management and should be treated via the creation, transfer and retention of knowledge. However, forgetting has not received enough attention thus far, and strategies used to manage individual forgetting are still very scarce. The research described in this paper provides a relatively systematic strategy for the first time. This paper identifies the key influencing factors, their optimal values for each KMS in managing individual forgetting processes, and the boundary conditions for applying different KMSs. Thus, this work provides theoretical guidance for enterprises to choose the appropriate KMS to compensate for the shortcomings of existing research.
Managerial implications
This study constructed a simple model to derive organizational implications for managing individual forgetting through different KMSs. To design our model, we drew upon prior agent-based models of organization learning proposed by March (1991), Miller & Martignoni’s (2016) characterization of individual forgetting, and classic KMSs stemming from Hansen et al.’s (1999) influential study. From this perspective, we can only highlight these connections to prior research as a basis for claiming that our model is valid. Although we have not established our model’s external validity, nevertheless, we see value in continuing this line of research because of its potential practical implications. Forgetting appears much too pervasive in organizations to neglect. The conclusions of this study can provide management implications for the selection of appropriate forgetting management strategies by enterprises. First, the results showed that a PS should not be used alone and should be applied together with a CS. Second, when forgetting is not common, if the learning ability of the organization members is very strong, the PS and CS cannot be used together, and the CS alone can perform better. Third, the combination of a PS and CS can achieve better results in most cases. When adopting an MS, to achieve a higher organizational knowledge level, managers should properly promote interpersonal learning among organization members in the PS and should not feed the encoding documents back to the organization members in the CS.
Further research directions
To deepen our understanding of managing individual forgetting, our research can be extended from the following aspects. First, the MS and CS without feedback in this paper retain knowledge to the greatest extent, although encoding knowledge is shelved, which is not consistent with reality. Subsequently, non-feedback provides feedback at a very low frequency or feedback only to a few decision-makers to verify whether our conclusions remain unchanged. Second, it could be useful to add scope to our model that incorporate other factors known to affect organizational knowledge, such as the network structure (Lazer & Friedman 2007) and knowledge encoding methods (Miller et al. 2006). In this study, a random network is used by default to describe the relationship among members of an organization. However, the relationship among organization members may be more complicated and diverse in reality. Thus, the method used to manage individual forgetting from the perspective of the network structure is a research topic worth exploring in the future. Similarly, our model adopts encoding methods based on best practices in the MS and CS (as in Miller et al. 2006), and other encoding methods, such as majority wins (Hastie & Kameda 2005), and how they affect our conclusions are worthy of further investigation.
Acknowledgements
We acknowledge support from the National Social Science Foundation of China (Grant No.15XGL001) and gratefully acknowledge valuable comments and helpful suggestions from the JASSS reviewers.Model Documentation
The model was programmed using NetLogo 5.0 and the code is available at https://www.comses.net/codebases/afc56959-44e1-4d55-9e8e-18a03c83bf94/releases/1.0.0/.References
AJITH Kumar, J., & Ganesh, L. S. (2011). Balancing knowledge strategy: codification and personalization during product development. Journal of Knowledge Management, 15(1), 118-135. [doi:10.1108/13673271111108738]
ALAVI, M., & Leidner, D. (1999). Knowledge management systems: issues, challenges, and benefits. Communications of the Association for Information Systems, 1(1), 7. [doi:10.17705/1cais.00107]
ALAVI, M., & Leidner, D. E. (2001). Knowledge management and knowledge management systems: Conceptual foundations and research issues. MIS Quarterly, 107-136. [doi:10.2307/3250961]
ARGOTE, L. (2012). Organizational Learning: Creating, Retaining and Transferring Knowledge. Berlin: Springer Science & Business Media.
BLASCHKE, S., & Schoeneborn, D. (2006). The forgotten function of forgetting: Revisiting exploration and exploitation in organizational learning. Soziale Systeme, 12(1), 100-120. [doi:10.1515/sosys-2006-0107]
BLACKLER, F. (1995). Knowledge, knowledge work and organizations: An overview and interpretation. Organization Studies, 16(6), 1021-1046. [doi:10.1177/017084069501600605]
BOCK, G. W., & Kim, Y. G. (2002). Breaking the myths of rewards: An exploratory study of attitudes about knowledge sharing. Information Resources Management Journal (IRMJ), 15(2), 14-21. [doi:10.4018/irmj.2002040102]
CHENNAMANENI, A. (2007). Determinants of Knowledge Sharing Behaviors: Developing and Testing an Integrated Theoretical Model. The University of Texas at Arlington, Arlington, Texas.
CONNER, K. R. (1991). A historical comparison of resource-based theory and five schools of thought within industrial organization economics: Do we have a new theory of the firm?. Journal of Management, 17(1), 121-154. [doi:10.1177/014920639101700109]
CYERT, R. M., & March, J. G. (1963). A Behavioral Theory of the Firm. New York, NJ: Englewood Cliffs.
DARR, E. D., Argote, L., & Epple, D. (1995). The acquisition, transfer, and depreciation of knowledge in service organizations: Productivity in franchises. Management Science, 41(11), 1750-1762. [doi:10.1287/mnsc.41.11.1750]
DAVENPORT, T. H., & Prusak, L. (1998). Working Knowledge: How Organizations Manage What They Know. Harvard Business Press.
DITTRICH, P., Kron, T., & Banzhaf, W. (2002). On the scalability of social order-modeling the problem of double and multi contingency following Luhmann. Journal of Artificial Societies and Social Simulation, 6(1), 3: https://www.jasss.org/6/1/3.html.
EASTERBY-SMITH, M. P. V., & Lyles, M. (2003). Organizational learning and knowledge management: agendas for future research. In: The Blackwell Handbook of Organizational Learning and Knowledge Management.
EASTERBY-SMITH, M., & Lyles, M. A. (2011). In praise of organizational forgetting. Journal of Management Inquiry, 20(3), 311-316. [doi:10.1177/1056492611408508]
FANG, C., Lee, J., & Schilling, M. A. (2010). Balancing exploration and exploitation through structural design: The isolation of subgroups and organizational learning. Organization Science, 21(3), 625-642. [doi:10.1287/orsc.1090.0468]
GREINER, M. E., Böhmann, T., & Krcmar, H. (2007). A strategy for knowledge management. Journal of Knowledge Management, 11(6), 3-15. [doi:10.1108/13673270710832127]
GLOBERSON, S. (1987). Incorporating forgetting into learning curves. International Journal of Operations & Production Management, 7(4), 80-94. [doi:10.1108/eb054802]
GROYSBERG, B., & Lee, L. E. (2009). Hiring stars and their colleagues: Exploration and exploitation in professional service firms. Organization Science, 20(4), 740-758. [doi:10.1287/orsc.1090.0430]
HANSEN, M. T., Nohria, N., & Tierney, T. (1999). What’s your strategy for managing knowledge. The Knowledge Management Yearbook 2000–2001, 1-10.
HASTIE, R., & Kameda, T. (2005). The robust beauty of majority rules in group decisions. Psychological Review, 112(2), 494-508. [doi:10.1037/0033-295x.112.2.494]
HOLAN, P. M. D., & Phillips, N. (2004). Remembrance of things past? The dynamics of organizational forgetting. Management Science, 50(11), 1603-1613. [doi:10.1287/mnsc.1040.0273]
HULSE, S. H., Deese, J., & Egeth, H. (1975). The Psychology of Learning. New York: McGraw-Hill.
JASIMUDDIN, S. M., & Zhang, Z. (2009). The symbiosis mechanism for effective knowledge transfer. Journal of the Operational Research Society, 60(5), 706-716. [doi:10.1057/palgrave.jors.2602613]
JENKINS JB, Dallenbach KM. (1924). Oblivescence during sleep and waking. American Journal of Psychology, 35, 605-12 [doi:10.2307/1414040]
JOLLY, R., & Wakeland, W. (2009). Using agent based simulation and game theory analysis to study knowledge flow in organizations: The KMscape. International Journal of Knowledge Management (IJKM), 5(1), 17-28. [doi:10.4018/jkm.2009010102]
KANE, G. C., & Alavi, M. (2007). Information technology and organizational learning: An investigation of exploration and exploitation processes. Organization Science, 18(5), 796-812. [doi:10.1287/orsc.1070.0286]
KLUGE, A., & Gronau, N. (2018). Intentional forgetting in organizations: the importance of eliminating retrieval cues for implementing new routines. Frontiers in Psychology, 9. [doi:10.3389/fpsyg.2018.00051]
LAZER, D., & Friedman, A. (2007). The network structure of exploration and exploitation. Administrative Science Quarterly, 52(4), 667-694. [doi:10.2189/asqu.52.4.667]
LEVITT, B., & March, J. G. (1988). Organizational learning. Annual Review of Sociology, 14(1), 319-338.
LIU, H., Chai, K. H., & Nebus, J. F. (2013). Balancing codification and personalization for knowledge reuse: A Markov decision process approach. Journal of Knowledge Management, 17(17), 755-772. [doi:10.1108/jkm-04-2013-0127]
MARCH, J. G. (1991). Exploration and exploitation in organizational learning. Organization Science, 2(1), 71-87.
MCGEOGH, J. A. Forgetting and the law of disuse. Psychological Review, 1932, 39, 352–370.
MILLER, K. D., & Lin, S. J. (2010). Different truths in different worlds. Organization Science, 21(1), 97-114. [doi:10.1287/orsc.1080.0409]
MILLER, K. D., & Martignoni, D. (2016). Organizational learning with forgetting: Reconsidering the exploration–exploitation tradeoff. Strategic Organization, 14(1), 53-72. [doi:10.1177/1476127015608337]
MILLER, K. D., Zhao, M., & Calantone, R. J. (2006). Adding interpersonal learning and tacit knowledge to March's exploration-exploitation model. Academy of Management Journal, 49(4), 709-722. [doi:10.5465/amj.2006.22083027]
NONAKA, I., & Takeuchi, H. (1995). The Knowledge-Creating Company: How Japanese Companies Create the Dynamics of Innovation. Oxford University Press.
PAOLI, M., & Prencipe, A. (2003). Memory of the organisation and memories within the organisation. Journal of Management and Governance, 7(2), 145-162.
SKYRME, D. J. (2000). Developing a knowledge strategy: from management to leadership. Knowledge management: Classic and contemporary works, 61-83.
WALSH, J. P., & Ungson, G. R. (1991). Organizational memory. Academy of Management Review, 16(1), 57-91.
WIXTED, J. T. (2004). The psychology and neuroscience of forgetting. Annual Review of Psychology, 55, 235-269. [doi:10.1146/annurev.psych.55.090902.141555]
WU, W., Ma, S., Wang, K., Tsai, S. B., & Lin, W. P. (2019). Entrepreneurial Team Learning, Forgetting and Knowledge Levels in Business Incubators: An Exploration and Exploitation Perspective. Journal of Artificial Societies & Social Simulation, 22(1), 10: https://www.jasss.org/22/1/10.html. [doi:10.18564/jasss.3932]
XU, B., Liu, R., & Liu, W. (2014). Individual bias and organizational objectivity: An agent-based simulation. Journal of Artificial Societies and Social Simulation, 17(2), 2: https://www.jasss.org/17/2/2.html. [doi:10.18564/jasss.2426]
ZACK, M. H. (1999). Developing a knowledge strategy. California Management Review, 41(3), 125-145. [doi:10.2307/41166000]
ZHANG, Y., & Zhou, Y. (2009). Knowledge forgetting: Properties and applications. Artificial Intelligence, 173(16-17), 1525-1537. [doi:10.1016/j.artint.2009.07.005]