Introduction
Agent based modeling (ABM) became a commonly known, if not broadly used, method in the 1990s and is now widely accepted as a standard modeling approach across many disciplines. Despite significant interest in the ABM approach, broader adoption is hindered by a set of methodological and conceptual challenges. After several decades of development, ABMs still remain difficult to design and use for many scholars, students, and policy makers, and can present challenges to standard inference frameworks that hinder their interpretation and understanding. These difficulties apply in particular to models that represent complicated spatial patterns and processes across a broad range of human, natural, and human-environment systems. Spatial ABMs are those where space is an explicit component in the model, and geographical proximity effects form part of model behavior, and will be the focus of this examination.
This paper originated in a session on the methodological issues of spatial ABMs at a workshop sponsored by United States National Science Foundation (NSF) on the state of the art in agent-based modeling (An et al. 2017). Spatial agent based models (SABM) have long been of interest to many different disciplines and it comes as little surprise that different fields have different modeling standards, foci, and practices. As such, there is much to gain from fostering communication and sharing knowledge among disciplines. Some research areas, such as land-change, ecology, and political opinion dynamics, have seen a relatively greater degree of communication given their shared concepts and problems, but even then, the vast majority of this ABM research occurs within specific disciplines (Lorscheid et al. 2019). We offer a framework for exploring a range of methodological challenges tied to engagements by fields invested in SABM, including anthropology, ecology, economics, geography, and the spatial sciences in general.
Framework for exploring methodological challenges
This paper identifies and structures key methodological challenges in the development and use of spatial ABMs and offers potential solutions from many disciplines. There has been interest in defining these challenges for nearly as long as spatial agent-based modeling has existed. Workshop participants — before, during, and after the event — discussed obstacles and identified key papers, milestones achieved, and issues tackled by a range of scholars and research fields, and have identified the challenges to spatial ABM. Spatiality may be considered in explicit terms but it is also often implicit to the context of a given problem domain like ecology or biogeography, or social systems including land use, voting systems, or disease propagation.
It is illuminating, and somewhat humbling, to consider how persistent most of the methodological challenges remain. Table 1 summarizes these challenges elucidated by papers that workshop participants identified as especially helpful overviews of agent based modeling with a spatial focus (or that speak to spatial problems) published over a period of twenty years (1997 to 2017). Interestingly, most challenges are evergreen in the sense that they are considered as pressing today as they were two decades ago. Some topics are conceptual in that they explore the epistemology of modeling, the role of ABM in generative science, or questions about whether or how these models can advance theory. The majority of the issues raised are methodological in some way and span from programming and operationalizing models through to conducting calibration, verification, and validation; all the way to sharing and documenting models and their results. Many of these challenges are shared by modeling in general but all take on additional dimensions when considering the role of space and place in particular. Of course, any focus on methodological issues necessarily bears on conceptual topics given that theory and method are scientifically entwined. This relationship may hold unusually so for ABM given its primary role in generative science and process exploration in many areas of academic inquiry (Epstein 1999).
Paper | Author(s) | Challenges |
1 | Axelrod (1997) | Programming and operationalizing models Model verification via internal validity Analyzing and sharing complex results Model replication Building a modeling community and interdisciplinarity |
2 | Parker, Manson, Janssen, Hoffmann, & Deadman (2003) | Abstraction versus realism Building an experimental frame in space and time Understanding complexity and complex results Representing decisions and behavior Empirical parameterization and model validation Communication of results Model replication and documentation |
3 | Crooks, Castle, & Batty (2008) | Model purpose (e.g., description vs. prediction) and whether ABM is best choice Extent model is based in, or can advance, theory Replication with complicated and subtly varying models Verification, calibration and validation Choosing appropriate agent representation with respect to aggregation and dynamics Making models more easily operationalized Model communication and sharing |
4 | Crooks & Heppenstall (2012)
| Verification, validation, and calibration Communication and visualization Degree of model abstraction vs. nature of the system being modeled Need to conduct multiple runs and explore parameter space Path dependence and complexity |
5 | Filatova, Verburg, Parker, & Stannard (2013) | Model design and parameterization Verification, validation and sensitivity analysis Integrating models across domains Spatial representation |
6 | O’Sullivan et al. (2016) | Balancing empirically rich models with simpler theory Model evaluation for parameters and structure Difficulty of hybrid modeling Communicating model processes and outcome to stakeholders |
7 | Schulze, Mulller, Groeneveld, & Grimm (2017) | Model purpose and definition Ad hoc design versus being based on generic principles or theory Representation of agent decision making and behavior Model documentation and reproducibility Testing and calibration Theory development and testable new predictions |
8 | Wallentin (2017) | Theory construction/contribution and generalization Need to balance emergence with structure in complex systems Yet another agent based model syndrome Model validation Model communication |
Instead of creating a new list that is largely replicative of those existing — as profoundly valuable as these lists are and continue to be — we instead developed a framework of cross-cutting themes for considering key methodological challenges for spatial ABM identified by the overviews noted above and by many other studies of more specific issues. In addition to adding a new perspective on methodological challenges, this framework gets at the premise that methodological issues are often implicated in various ways by a variety of themes. These cross-cutting themes include: model definitions; issues of space and scale; capturing time and process; model development; and modeling frameworks and tools. Table 2 describes these areas and offers key examples and citations that identify specific challenges from the papers noted above (in square brackets) and one or two other key papers. These and many other works are referenced throughout the paper.
Challenge | Key examples and issues |
Definitions | Defining agents in a way that captures complexity [3] (Couclelis 2001) Abstraction versus realism, including avoiding too simple or too complex agents [2, 6] (O’Sullivan et al. 2012) Choosing appropriate agent representation with respect to aggregation and dynamics [3], making models fully agent based (Tesfatsion et al. 2017), and the limits of simplicity (Clarke 2004) Representing decisions and behavior [2, 3, 7], including basic features (Gilbert 2008), spatiality (Filatova 2015), and networks (Morgan & Daigneault 2015) YAAWN (“Yet Another ABM”) syndrome [8] (O’Sullivan et al. 2016) |
Space | Basic notions of space in models (Manson & O’Sullivan 2006), conceptual space versus applied model concepts (Dainton 2001), and degrees of spatiality (Stanilov 2012) Spatial representation and modeling (Birch, Oom, & Beecham 2007), including modeling discrete or continuous space (O’Sullivan & Perry 2013), data models including raster, vector, and object (Hammam, Moore, & Whigham 2007), and networks (Andris 2016) Context specific models (Crooks et al. 2008) Scale, pattern, and process (Turner et al. 1989) Identifying the role of space in reality vs. model (Wallentin 2017), especially as related to data vs. theory (Railsback & Grimm 2011) Representation (Ajelli et al. 2010) and scale impacts on modeling (Shook & Wang 2015) |
Time | Building an experimental frame in space and time [2] and choosing spatial representation [5] including between equal-time and event-based (O’Sullivan & Perry 2013) and synchronous or asynchronous updating (Ruxton 1996) Path dependence, emergence, and complexity [1, 4, 8] including interaction effects and heterogeneity (Couclelis 2001) Generative science (Millington, O’Sullivan, & Perry 2012) and its relationship to standardized models with standard concepts of prediction and change over time (Stillman et al. 2015) Spatiotemporal modeling needs (Jjumba & Dragicevic 2016; O’Sullivan 2005) including impacts of representation on model function (Peuquet & Duan 1995) Data types related to aggregation and spatiality (An et al. 2015) and challenges of big data and dynamics (Clarke 2003; Parry & Bithell 2012) Motion over space (Torrens 2010) and what it means for pattern vs. process (O’Sullivan et al. 2006) |
Model development | Identifying how ABM contribute to theory [3], especially in terms avoiding ad hoc design at the cost of generic principles or theory or creating testable predictions [7, 8]; (Axelrod, Riolo, & Cohen 2002; Gilbert & Troitzsch 2005) Model purpose in terms of pragmatic and paradigmatic models [3] (DeAngelis & Grimm 2014), especially as tied to model design and parameterization [5], and parsimony, simplicity, and complexity (Batty & Torrens 2005) Validation, verification, and calibration [1 - 8], including the needs for multiple runs [1, 4], challenges of evaluation for complex models (Grimm et al. 2005; Manson 2007), and need to include mixed approaches (Koch et al. 2019) and a range of data (Robinson et al. 2007) Replication experimentation and generative science [1, 2, 3, 7] (Batty 2008) and linking models for conceptual advances (Van Nes & Scheffer 2005) as well as linking multiple data models to ABM (Grignard et al. 2013) Analyzing, sharing, and communicating complex results [1 - 8] (Grimm et al. 2010; Polhill, Parker, Brown, & Grimm 2008) |
Modeling frameworks | Programming and operationalizing models [1], including making models more easily operationalized [3], and dealing with programming from scratch (Crooks et al. 2008) Complexity of choosing among existing systems (Tobias & Hofmann 2004) and modeling packages (Kravari & Bassiliades 2015) Dealing with large numbers of agents (Crooks et al. 2008) and the potential (Clarke 2003) and challenges of high-performance computing (Shook, Wang, & Tang 2013) Challenges of hybrid modeling [6]; (Parker et al. 2003) including building out functionality found in other models (Thiele & Grimm 2010) Difficulties of linking spatial tools to ABM (Crooks & Castle 2012) or building spatial functionality into ABM (Grignard et al. 2013; Klugl, Herrler, & Andriotti 2006) Building a modeling community and interdisciplinarity [1] and integrating models across domains [5] including social and natural sciences (O’Sullivan & Manson 2015) |
We build on this framework by examining these methodological issues in spatial ABMs in the remainder of the paper. We first define what we mean by spatial ABM to narrow our object of inquiry and describe how spatiality is the source of both benefits and challenges, including drawing on lessons from economics on defining spatial agents. We then examine the various challenges of including space within ABMs, including the various ways of understanding and representing space, and look to geography and its concepts of scale for insight in dealing with spatiality. We then turn to the temporal aspects that complicate space in ABM, particularly the challenges of integrating space and time, and examine the lessons that experience with ABMs in ecology holds for standardizing dynamics and behaviors. We then look at model development in general and examine ways in which collaboration with anthropologists has advanced this development. We also consider modeling frameworks and tools, with a specific focus on how spatial science is expanding the SABM toolkit.
Defining Spatial ABMs
What is a spatial ABM? We start by exploring the terms agent, environment, and spatial to specifically address the methodological issues connected to spatiality in ABMs in contrast to ABMs or spatial models in general. ABMs have developed in a manner that has led to different definitions of the term agent. Most descriptions rely on listing a set of agent characteristics. Drogoul & Ferber (1999) describe an agent in a model as a virtual entity with nine different features, ranging from the capability to communicate, interact, and reproduce, to possession of skills and resources. Wooldridge & Jennings (1995) list autonomy, social ability, reactivity, and proactivity as critical features of an agent. Gilbert (2008) defines agents in simulation models as:
"…either separate computer programs or, more commonly, distinct parts of a program that are used to represent social actors – individual people, organizations such as firms, or bodies such as nation-states. They are programmed to react to the computational environment in which they are located, where this environment is a model of the real environment in which the social actors operate." (p. 5)
Parker et al. (2003) described agents as autonomous entities that share an environment through communication and interaction with other agents and decision-making as the processes that tie agents to other agents and their environment. Importantly, the characteristics common to these definitions are the autonomy of agents and the ability to interact with other agents and the environment, so we take these characteristics as the bare minimum.
Many definitions show the importance of the environment as a component of ABMs. Gilbert (2008) terms the environment a virtual world in which the agents act. This description poses few restrictions on what qualifies as an environment in ABM. The environment in these models can be a network of agents described by different features with agents being the nodes in a network and their relationship being represented in the form of links between nodes (Riolo, Cohen, & Axelrod 2001). Environments can also be social spaces or networks through which innovation and knowledge spread, such as when social networks help spread agricultural innovations that manifest as land-cover change (Gilbert, Pyka, & Ahrweiler 2001).
ABMs often represent the environment as spatial, including models without a geographic representation of space but possessing agents with coordinate locations. In spatial ABMs, the level of detail of the representation of the geographic environment as well as the level of detail of the agent-environment interaction can vary considerably (Stanilov 2012). Agents often have x and y coordinates, although other representations exist, such as topologically-defined agents that exist in graphs that map onto an environment with spatial characteristics, such as networks of farmers in a simplified agricultural landscape. In many spatial models, the environment is digitally encoded as a grid on which the agent behavior plays out, where the grid cells and their neighbors can have values that influence behavior.
Traditionally, the focus of SABMs has been on the implementation of agents and social processes, and less on detailed descriptions of the geographic environment (Brown et al. 2005). The degree to which space is made explicit in SABM has significant impacts on model performance (Ajelli et al. 2010) and the extent to which the model is accurate and realistic and therefore useful (Barnaud, Le Page, Dumrongrojwatthana, & Trébuil 2013).
- SABM may use implicit or non-geographic notions of space. An ABM of vehicles and transportation flows, for example, or farmers within a trust network, may focus primarily on the network dynamics that are only partially or topologically tied to space. Transportation flows have spatial elements, such as the physical distance between origin and destination pairs that only partially bear on travel time, cost, or mode. Similarly, trust relationships among farmers have a spatial element, as seen in the way neighboring actors are more likely to trade information or ideas, but these are joined by other kinds of network relationships, such as kinship or group affiliation, that may outweigh these spatial aspects (Manson et al. 2016; Morgan & Daigneault 2015).
- Space may be explicitly represented but abstract in how it maps onto reality, such as Tobler’s early work on cellular geographies (1975) and models of segregation (Hegselmann 2017; Sakoda 1971; Schelling 1971). The foundational Sugarscape Model used a two-dimensional grid and mobile agents with x/y coordinates (Epstein & Axtell 1996). More spatially realistic examples include analyses of the coastal land market (Filatova 2015), studies of farmer decisions on land change (Valbuena et al. 2010), or a SABM that models urbanization patterns (Koch et al. 2019).
- Explicit and realistic spatial ABMs may boast one-to-one relationships between agents and a range of environmental features (Stanilov 2012). The ENVISION framework (Bolte et al. 2007) offers models including process representations for urban expansion, vegetation growth, changes in climate, as well as the emergence of wildfires (Spies et al. 2017). More realistic and explicit representations of space abound (Filatova 2015; Wallentin 2017).
There has been a trend toward increasing spatial and temporal explicitness and realism in ABMs as many modelers seek to move from system explanation to future state forecasting. While this trend toward realism may improve the empirical validity and usefulness of ABM in some contexts, there is a risk that the model may not generalize well to other geographies or require a considerable amount of reparameterization. Scale dependent factors play a significant role in mediating spatiotemporal processes (Shook & Wang 2015), an issue we discuss in more detail below.
Lessons from economics: Defining spatial agents
Agent-based Computational Economics (ACE) is a variant of agent-based modeling that focuses specifically on the computational modeling of economic processes (including whole economies) as open-ended dynamic systems of interacting agents (Tesfatsion 2018). Tesfatsion (2017) presented and motivated seven ACE modeling principles that distinguish ACE from other types of modeling. An ACE agent is defined as any software entity capable of acting over time on the basis of its own state (data, attributes, and methods). Thus, agents are locally constructed and can represent a broad range of entities, from individuals to groupings in the social, biological, and physical domains. The state of the system as a whole at any given time is then determined by the ensemble of agent states, interaction among agents, and interaction between agents and their environment. Finally, the role of the ACE modeler is limited to the setting of the initial agent states and to the observation, analysis, and reporting of model outcomes.
Taken together, the seven ACE modeling principles express the fundamental goal of many agent-based modelers: namely, to be able to study real-world systems as historical processes unfolding through time. In addition, however, they also require ACE models to be fully agent based; i.e., all entities capable of acting (passively or consciously) within an ACE computationally-constructed world are to be modeled as some form of agent.
With regard to spatial modeling, ACE differs from other modeling approaches in that all entities defining a spatial environment are modeled as agents along with the decision-making entities that populate this environment. This approach has two key advantages. First, it enhances conceptual transparency by forcing modelers to be careful about the empirical credibility of their modeled cause-effect linkages, since all factors affecting world events (including spatial factors) must be clearly identified as an agent or agent component. Second, it facilitates plug-and-play model scalability.
For example, the ACE watershed basin model developed by Tesfatsion et al. (2017) includes a broad hierarchy of environmental, institutional, and decision-making agents. These include a Basin agent that encompasses an upstream Farmland agent and a downstream City agent as members (Figure 1). In turn, the Farmland agent has decision-making Farmer agent members and the City agent has a Levee agent and a decision-making City Manager agent as members. A Market agent has distinct instantiations into input and output markets for corn production. A Climate agent generates weather patterns (hourly rainfall amounts) for the basin during each simulated year. Finally, a Hydrology agent generates river water-flow outcomes for the basin that depend on Basin, Market, and Climate outcomes. ACE allows a rich and detailed expression of multiple human and environmental factors while piecing them together in a way that captures a range of dynamics that occur over different spatial and temporal scales.
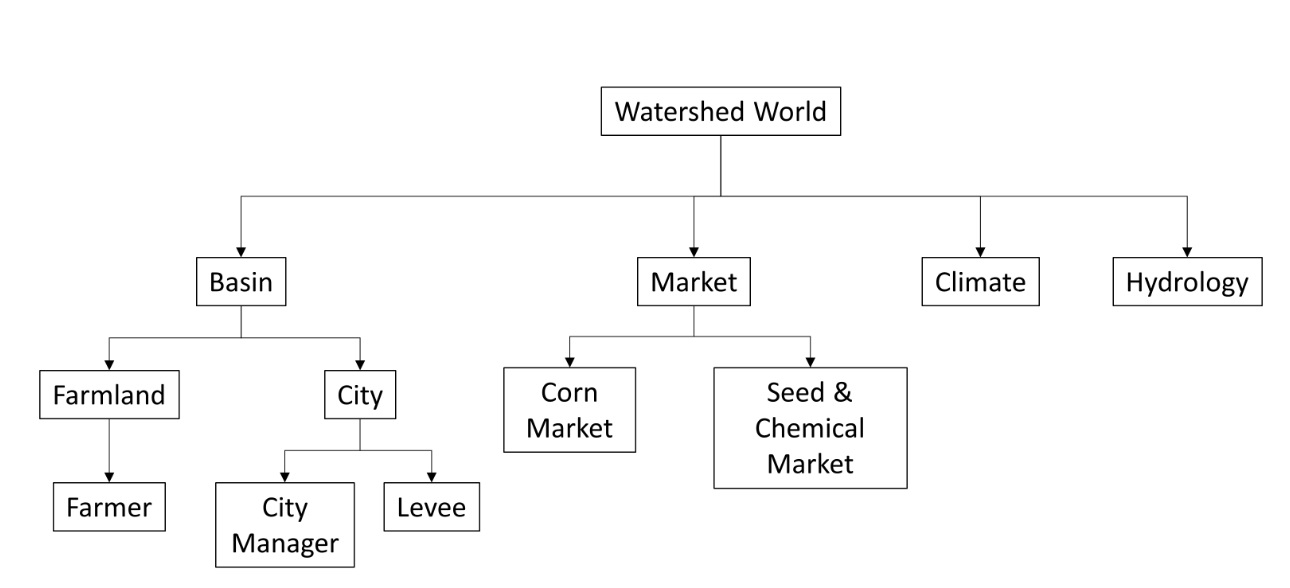
Space
Spatial ABMs are a representationally flexible simulation framework able to incorporate a diversity of theoretical assumptions about space, time, and scale. While this flexibility is beneficial, it may also impact conceptual completeness and computational efficiency (O’Sullivan et al. 2012). Appropriate representations of space and time depend on the specific modeling question, degree of realism sought, and, whether the model formulation is derived from theory or more from empirical data (Couclelis 2001; Railsback & Grimm 2011). Spatial representation in models hinges on how agents relate to space and each other as well as on the choice of data model used to implement space in both agents and environment.
Two common complementary approaches exist for representing how agents perceive and interact with space (Manson & O’Sullivan 2006). The first, absolute space, focuses on how local characteristics influence the decision-making of agents (e.g., Ricardian view) and derives heavily from Newtonian space, where space possesses specific properties. In contrast, relative space is the idea that the relationships among objects define spatial relationships (e.g., bid-rent Alonso or Von Thünen circles) and derives from the broader notion of relationality proposed by Leibniz (Massey 2005). Under this framework, space is represented as relationships among objects; there is no pre-existing container that hosts objects (Dainton 2001).
SABMs typically start with absolute space. Objects may move or change attributes, and events may occur or come into being, but all the while the underlying spatial context or container remains unchanged. Most ABMs represent space as absolute by using coordinate systems that create a reference into which agents can be mapped. This approach has a long tradition within the social and natural sciences and has been used to understand diverse phenomena ranging from urban expansion and agricultural land-use change to tropical ecology and animal migration (Wallentin 2017).
Relative and hybrid views of space in SABM continue to advance with contributions from many disciplines. Early examples included models of human capital accumulation that examine the interplay between local spillover and global market participation (Bala & Sorger 2001). Alexander et al. (2013) modeled perennial energy crop diffusion and market creation in the United Kingdom using both notions of space. In this model, farmer agents calculate their distance to market in order to determine costs, and also utilize local land characteristics to determine production decisions. Network space captures elements of absolute and relative space by treating agents as nodes that are assigned a coordinate pair and a set of relationships to other agents and the environment. Network characteristics have been shown to dramatically impact diverse phenomena across a range of social systems and need to be further incorporated into spatial ABM (Agrawal et al. 2013).
Beyond addressing issues of absolute versus relative space, one of the primary challenges for modelers is whether to treat space as discrete or continuous (O’Sullivan & Perry 2013). Discrete space often relies on a regular tessellation of squares or more complex shapes such as hexagons. Alternatively, continuous space can be represented using vector data objects including points, lines, and polygons. Even more complicated, discrete entities like agents or land parcels may be best represented with vector data (e.g., points for agents, polygons for areas) while smoothly varying surfaces, such as precipitation gradients or elevation, are often best represented with tessellations. Another variant is where agents may take on any vector form, although this possibility remains underexplored (Hammam et al. 2007).
The selection of discrete or continuous space representations is made more real by the choice of data model. The raster data model is common in ABM, where space is gridded into equal-sized cells, and each cell is homogeneous holds one or several key variables. Over time, each cell may change status or attributes according to rules, and accordingly, grids represent the changing status of the cells and their neighbors over time. This data model has been criticized for lack of efficiency — particularly in how the same grids are stored over time and how square grids can introduce geometric artifacts — yet it remains popular given its conceptual simplicity, ease of comprehension and interpretation, and ready availability of mathematical and computational tools that work with matrices and Geographic Information Systems (Birch et al. 2007).
The vector (and by extension, object) data model is gaining ground in ABM. A point is a single location in space defined by a coordinate pair. At many scales of analysis, points serve as appropriate (if necessarily unrealistic) representations of agents. We say unrealistic given how nuanced an complex real agents can be; as Hagerstrand (1982) put it for representing a person with a space-time path, we are tasked with trying to capture “a living body, endowed with memories, feelings, knowledge, imagination and goals – in other words capabilities too rich for any conceivable kind of symbolic representation (p. 324). Yet, scholars forge ahead, even when they know their models incomplete. When agents move, interact with one another or act upon their spatial environment, vectors can accordingly change their attributes or geometry. SABMs are increasingly embracing vector models for both agents and environment because raster models are simple, but they impose computational overhead in mapping individual grid cells onto complex areas that constitute regions, such as modeling land parcels. The vector data model also dovetails with the object-oriented programming paradigm commonly used in languages that implement ABM, in that points, lines, or polygons can be represented as discrete software objects that represent real-world entities.
The network data model is also gaining prominence in ABM. Networks consist of links or edges and nodes that form a graph. Networks can be of interest for the mechanisms driving link formation or for the overall topological and metric characteristics of the graph. Network properties and agent behaviors can be mediated by absolute or relative spatial parameters, or according to social variables such as homophily mechanisms (where similar entities are more likely to be connected) or institutions that define relationships (for an overview see An 2012). Distance in network space is a function of the number of network edges separating individuals, and small network distances can cross vast spatial distances. Network ties can also vary qualitatively and quantitatively in ways that realistically map onto myriad real-world relationships (Manson et al. 2016), including among social media. Yet another variant is where actions of agents cause the structure of the space represented as a network to change (O’Sullivan 2009).
Broader issues exist beyond the challenges posed by choosing among, or reconciling, competing notions of space (absolute, relative) or spatial representations (raster, vector/object, network). Regardless of conceptualization or data representation, there is an ongoing need to more clearly define the role that distance plays on agent decision making, especially since it can be measured in so many ways, including Euclidian distance, cost, perceptual distance, travel time, and network distance (Illenberger, Nagel, & Flötteröd 2013). Further work needs to be done to make tools easier to use for representing both network and geographic space, particularly in the realm of spatial statistics (Andris 2016). Large bodies of work exist on spatiality and spatial networks but have been generated largely by computer scientists and physicists (Barthélemy 2011). Social science would benefit from these conceptualization and methods, while researchers outside of social science could learn more of the domain knowledge found in social science (O’Sullivan & Manson 2015). Finally, it is incumbent upon the modeler to engage with larger debates around space that lie beyond the scope of spatial ABM and this paper, such as being aware of how differing notions of space can influence how people speak about their lived experiences or understanding of space, and extensions including potential loss of indigenous space, for example or fixing on simple notions of how spaces are used as opposed to contested views (Fox, Suryanata, Hershock, & Pramono 2006).
Lessons from geography: Scale and space
Scale concepts improve our understanding of space and place. Many fields recognize scale, but for geography it is a primary organizing concept. While a range of epistemological orientations exist around scale (Manson 2008), it is often used to define the basic terms of the modeled system in regard to spatial resolution, or grain, and the extent of data. More broadly, it encompasses temporal, spatial, and organizational scales of a host of human, natural, and human-environmental systems. Thinking on scale continues to change and evolve given how complicated it is as a concept (Herod 2010; Phillips 2012).
Beyond resolution and extent, there are two main approaches to thinking about scale in spatial ABM (Evans & Kelley 2004). The first form of scale typically used in ABM is hierarchical, where different scales are understood as nested hierarchies. In this conception of scale, analysis can be done at the scale of the body, the urban neighborhood, the regional, the national, or the global, and over days, months, years, decades or centuries. Importantly, phenomena at local space-time extents can produce phenomena at greater extents. In ABM work that draws on the complexity sciences or uses concepts from complex systems, higher scale phenomena are often seen emerging non-linearly from more detailed-scale agents and interactions among agents or between agents and the environment (Manson, Sun, & Bonsal 2012).
The second approach to scale is to see it as horizontal, flat, or networked; where scale is understood as a function of network connectivity properties. Models of spatial networks and socio-spatial networks have seen major advances due to an influx of highly detailed data. Location-based services, environmental sensor networks, geo-enabled social media, among other geospatial technologies, have produced a deluge of data for scientists (Shelton, Poorthuis, & Zook 2015). Network scales are of relevance across a variety of spatial ABM applications ranging from the diffusion of agricultural innovations (Evans, Phanvilay, Fox, & Vogler 2011) and from international teleconnections driving land-use change (Munroe, McSweeney, Olson, & Mansfield 2014) to disease transmission in epidemiology (Riley 2007).
The ability to represent interactions across scales is often theoretically required and practically necessary in spatial ABM. An et al. (2005) presented an ABM where institutions are represented explicitly and are able to dynamically interact with individual agents. This and other studies exemplify the importance of scale variance, whereby a common phenomenon, namely the interactions of human agents, is represented as two separate human phenomena, namely individual decision-making and larger scale social and institutional actions. While this type of scale variance is a strength of spatial ABM, Tesfatsion (2002) argues that in representing humans, individual human agents should retain their agency, and not be forced to act in a way that is determined without reflecting their own individual agent states, rules, and goals.
In many disciplines, there is a belief that a phenomenon can be studied at an optimal spatial scale, whereby the resolution of inquiry is fit to real-world phenomena. Within discrete representations of space, the critical choice is to relate the grain of the cell to the phenomenon being studied in order to capture the relevant processes. Shook & Wang (2015) demonstrated how the choice of spatial and temporal resolution in an epidemiological SABM dramatically affects the modeled dynamics of disease spread in space and time. Evans & Kelly (2004) provided a similar application in land-change science, showing that spatial resolution affects agent land-use decision making. These and other SABMs illustrate that serious consideration of scale effects is essential to many modeling domains.
Time
Much of the interest in SABMs stems from their ability to model system dynamics. Despite this interest, there are many methodological challenges and opportunities in representing time in these models. Key decisions revolve around how to conceive of time and how to represent it in the model, while others involve how the model is updated in response to the actions of agents and changes in their environment. The nature of the data being used also matters, in that there tend to be several kinds of temporal data and each bears on a range of modeling choices. The advent of big data has only made these choices more demanding.
Most ABMs rely on one of two approaches to modeling time: equal-time and event-based (O’Sullivan & Perry 2013). The equal-time approach maps each model iteration to a common interval of time change (e.g., day, month, or year) and time steps are of equal duration, with agent actions happening (or at least being recorded) synchronously. In contrast, event-based simulations calculate the time elapsed from a previous event to a subsequent event in a queue of events or actions. Equal-time approaches are often preferred for their conceptual simplicity, because all modeling processes operate or are measured at the same pace. However, they may require more computation to simulate the same duration compared to event-based approaches and modelers must carefully select the time step that they need to capture changes, processes, actions, and decisions across multiple scales (Shook & Wang 2015). Event-based time offers the advantage of simulating longer durations with fewer computational costs because agents are only considered at points of change. Similarly, the model does not need to consider long periods of time without changes. This can present a major benefit if there are highly variable levels of activity through time in the simulation and it is also efficient in terms of memory and storage (Peuquet & Duan 1995). However, event-based models can have difficulty capturing continuous processes where the meaning of an event or action is hard to define.
Model update is either synchronous or asynchronous in determining how agents, other objects, and their respective actions and attributes change over time. Synchronous updates are those that happen simultaneously, whereas asynchronous updates happen one at a time. O’Sullivan & Perry (2013) organized updating strategies into three categories. The first is complete synchrony, which is both realistic and computationally challenging as it requires the modeler to make many decisions about how to represent a process. For example, if two agents in a synchronous simulation reach a resource at the same time, the modeler needs to model how agents break such ties. Computationally, a copy of the original model or grid is made to reflect the model behavior, and at the time increment this copy replaces the original. The second strategy, random asynchronous updates, requires agents to update their states and act on the world at every time step in a random order. Without randomization, geometric biases can appear, such as when a grid is always scanned for agents top to bottom and left to right. This randomness is often used when more principled or empirically based approaches to ordering agent actions do not exist. The third strategy, ordered asynchronous updates, is preferable when the exact order of events can be determined from theory or empirical observation. For example, rule changes or new environmental factors can be introduced at specific times.
Most spatial ABMs update time asynchronously using a single thread, though new parallel applications for synchronous updating are in development (Shook et al. 2013). While a seemingly small decision, updating routines can have substantial impacts on model outcomes (Ruxton 1996). Updating routines can also have a large impact on computational performance. When models that require synchronization contain many agents, then synchronous updates can force the model to break many ties for "popular" shared resources (e.g., agents competing for space while moving in a congested area). Tie breaking procedures require additional computation and result in longer simulation times. On the other hand, in uncongested areas synchronous updates can improve performance, because updates can happen simultaneously (i.e., in parallel) rather than forcing each agent to be updated in sequence.
The nature of the model’s data often determines whether an ABM emphasizes event-based or equal-time approaches. Most data tend to fall into two categories, tracking data and spatial panel data (An et al. 2015), which differ in aggregation and spatiality. At the level of an individual, tracking data measure attribute changes of objects, such as agent events or transactions in specific locations at specific times. Spatial panel data include observations at the level of collections (areas) or cross-sectional data, and measure attributes or events of agents or other objects for spatial units or groups of agents. Individual object data may lend itself more to event-based time than cross-sectional units in the sense that their actions are more readily and explicitly calibrated from individual-level data.
Big data — often explicitly temporal — offer both potential and pitfalls to the treatment of time in ABMs, and especially SABMs. The overarching challenge for most modelers is whether they can extract useful knowledge (agent rules, system dynamics) from their data. From this one larger challenge flow three specific ones. First, most modeling tools and frameworks are rarely designed to handle massive amounts of data. This technical disconnect makes it difficult to explore the intersection between ABMs and big data. Overcoming this challenge will require the development of new ABM frameworks that scale to the size and scope of massive datasets, or that employ high performance computing (Clarke 2003; Parry & Bithell 2012). Second, there remains a significant disconnection among different forms of big data that need be connected, explored, and managed in developing new flavors of ABMs. The hope is that these data, once connected, will better support libraries of behaviors and spatial dynamics, as well as offer more robust model calibration and validation (e.g., providing better confidence levels from actual data). All these features could both heighten the appeal to policymakers and begin to align ABMs with other forms of modeling, such as regional economic models, climate models, or those of disasters (Torrens 2014). Third, research is needed to explore where big data can be an asset rather than a hindrance in model design, development, deployment, and usage for decision making. It is unclear whether ABM simulation data should be validated using big data, whether big data should be used to inform the creation of parameters and rules that govern models, or whether there exist myriad other possible intersections between these two emerging approaches. More broadly, even if knowledge extraction can be automated through machine learning techniques, will there always need to be a human element in interpretation? The answer is very likely ‘yes’ given the importance of human interpretation and domain knowledge for preserving the ability to explain and understand system dynamics. Big data for SABMs remains a vibrant and open research area for further exploration, but as explored next, big data are not always a panacea.
Lessons from ecology: Standardized dynamics and behaviors?
There have been a growing number of calls for domain-specific standard sub-models in fields including land-use and land-cover modeling, urban systems, and ecology (Stillman et al. 2015). Other fields, such as land-cover dynamics and forestry, for example, have developed, with some success, generic models that are designed to capture general processes like stream flow, reforestation or evapotranspiration that can be tailored to specific settings. Of course, the fundamental roadblock to developing generic models is possessing a sufficiently accurate and robust understanding of the system in question. Grimm (1999) describes the tension between pragmatic and paradigmatic reasons for ABM, where the former implies a focus on finding the most adequate method to model a system while the latter, paradigmatic motivation, is concerned with rearticulating conceptual paradigms.
While modelers often cry out for more data, availability of large data sets is rarely the main problem in building and using ecological ABMs. More important and difficult is the paradigmatic drive to develop an understanding of agent behavior. Ecological ABMs almost always need information on organism behavior and physiology that determine how individual fitness depends on behavior. This kind of information is often not available, yet too often, producing it is not seen as innovative or fundable science. Consequently, a key challenge for biological and ecological ABMs is to find the funding and motivation to produce the basic knowledge of organisms necessary to build ABMs. For example, much of the existing theory for agent behavior, or behavioral ecology, is not useful for ABMs because this theory does not address feedbacks. Existing theory works for one agent in a world that is not affected by the behavior of other agents, not in a world of interacting agents, and ABM projects get stuck on the problem of how to model decisions where tradeoffs and feedbacks are important. Big data can be used to elucidate some of these feedbacks, but these data can rarely replace theory that drives behavior.
Stillman et al. (2015) argue that ecology is on the cusp of developing a robust and readily modeled understanding of adaptive behavior, energetics, and interactions. Having these concepts in hand could make it possible to develop repertoires of spatial agent behaviors (e.g., movement and selecting spaces more generally, mental maps) as well as spatial dynamics (e.g., agent-agent, agent-environment). Eventually this would allow us to develop more expressive languages for designing and implementing spatially explicit agent models. ABMs of animal movements are a case in point, where empirical findings and subsequent conceptual frameworks (e.g., size of animal matters) lead to regularities that can drive modeling (Tang & Bennett 2010).
Of course, while ecologists look for standardized rules and dynamics, it is not clear whether these would apply to human systems, although we believe these rules would be a step in the right direction. Modelers are developing better and more nuanced treatments of how spatial perception is differentiated among agents. These approaches offer consideration of subjective agent experience of space and open up avenues to incorporate more and ‘thick’ representations of agent systems, or those that provide observations with nuanced and subjective experiences of the participants. Millington, O’Sullivan, & Perry (2012) used sequentially recorded individual states to create narratives that unpack the individual-level dynamics driving bird colony breeding synchrony. Also promising are recent advances combining cognition, machine learning, and spatial ontologies—ontologies for representing spatial concepts—as a way of identifying agent behaviors (Couclelis 2010). This work illustrates the role that subjective individual perspectives and contingent events play in driving temporal dynamics as well as a promising avenue to exploit big data in advancing ABMs, and SABMs in particular.
Spatial Model Development
The SABM development process should ideally encapsulate essential research processes, including hypothesis formulation, model design, parameter choice, behavioral and interaction rules, and validation. What follows are some observations specific to the methodological challenges for spatial ABM development. For broader takes on model development, see Gilbert & Troitzsch (2005), Railsback and Grimm (2011), O’Sullivan and Perry (2013), and Wilensky & Rand (2015). In particular, modelers face basic questions about whether an ABM makes sense for a given problem as well navigating the balance between predictive or explanatory modeling, model design and construction, and simplicity and complexity.
The first and too-often ignored step in model development is simply to determine whether ABM is the most suitable approach. The increasing availability of ABM software platforms such as NetLogo, Repast, MASON and an abundance of data has led to an upsurge of SABM applications that could be better executed through other approaches. Determining whether ABM is the most appropriate technique for simulating a system requires a critical assessment of whether the advantages offered by ABM outweigh the difficulties associated with model development. O’Sullivan et al. (2012) described the ideal characteristics of a system suited to ABM, including heterogeneity of the decision-makers and context of agents; importance of interaction effects among agents and their environment; and being medium-sized in terms of numbers of components, threading the needle between being too large and complicated for mathematical tractability and too small for statistical averaging (a perspective closely tied to early thinking about complexity science, see Weaver 1968).
An important challenge in developing ABM is deciding whether the model is predictive or explanatory. Predictive models aim to simulate a system with a degree of realism so that the results—often those at an aggregate level—can be used empirically. A predictive ABM of a socio-spatial phenomenon (such as crime, traffic, or protests) may include a realistic representation of the underlying environment that allows the models to make predictions about the future state of the real-world directly. Explanatory modeling, in contrast, is typically concerned with refining the theoretical explanations of a phenomenon (Macy & Willer 2002). While ABM can certainly aim to develop predictive capacity, they can also have the goal to increase our understanding of fundamental processes that may appear in a variety of applications (Axelrod 1997).
Another important challenge lies in determining the balance between model design and model construction (Wilensky & Rand 2015). Modelers are generally encouraged to comprehensively plan the characteristics and behaviors of agents, the environment, and all possible interactions. However, there is often pressure or a desire to implement the model, which means that design and implementation in most models coevolve (Crooks et al. 2008). The ABM community has developed innovative means of developing and describing models, including those with spatial elements such as Pattern-Oriented Modeling (Grimm et al. 2005) and standardized ways to design and document models, such as the Overview, Design concepts, and Details (ODD) protocol (Grimm et al. 2010) and extensions such as ODD+D for describing human decision-making (Müller et al. 2013). There is also much promise in the idea of using established and existing modules and submodules to develop building block models (O’Sullivan & Perry 2013). There is also interest in the modelers’ notebook paradigm, which proposes more traceability in model development and could conceivably be implemented via version control systems or other metadata and process-tracking workflows (Grimm et al. 2014).
Another challenge in SABM is striking a balance between simplicity and complexity. Most modelers agree that the level of model complexity needs to be justified by the complexity of the target system, but opinions as to how to reach the ‘appropriate’ level of complexity are polarized. The ‘Keep It Simple, Stupid’ (KISS) argument posits that models should be as simple as possible initially, with additional complexity added only if the model is unable to appropriately represent the system in its simplest form. Conversely, the ‘Keep It Descriptive, Stupid’ (KIDS) approach (Moss & Edmonds 2005) starts with a model that reflects the evidence and knowledge about the target system, however complex it makes the model, and then iteratively removes features that appear to be unnecessary.
Lessons from anthropology: Qualitative modeling and collaboration
Cultural anthropologists bring skills and concepts to the development and implementation of SABMs, especially models that emphasize the relationship between human decision-making and the environment. These scholars employ qualitative research methods including ethnographic fieldwork, interviews, and focus groups to better understand how humans make decisions. These methods offer flexibility and open-endedness that allows for deeper understanding of topics that were unanticipated by the researchers. As the ABM approach is not inherently quantitative (Yang & Gilbert 2008), collaboration with cultural anthropologists lends itself to inform the development and parameterization of SABMs.
The advantages of qualitative methods are well understood in the field of integrated environmental modeling, which has many parallels to the research often accomplished with SABM. Model development and parameterization often relies heavily on stakeholder participation, especially in the form of facilitated workshops (Voinov & Bousquet 2010). These workshops can be helpful at the cost of being resource intensive (in the sense of requiring trained facilitators) and often overwhelming for participants given the amount of time and effort required. They also run the risk of introducing incomplete information and bias into modeling given how human behavior in groups under observation can be performative.
In contrast, qualitative research methods such as ethnographic fieldwork and interviews are a useful way to collect information on the nature and spatial variation of interaction of humans with their living and non-living environment. In-depth and semi-structured interviews can provide additional understanding of the decision-making process and underlying motivations of the interviewee about issues such as interactions with other people or the environment. For example, anthropological field work was essential to developing SABM on social network and institutional relationships in the fire-prone landscapes of Central Oregon (Spies et al. 2017). This hands-on research is also vital to overcome potential biases in knowledge generation in spatial models as such; Barnaud et al. (2013) note how spatial representation can impose arbitrary or unforeseen restrictions on how people view their interactions and attachments to space. Skilled qualitative research can winkle these issues out and deal with them.
However, these collaborations are not without challenges. First, as with many interdisciplinary approaches, it takes much time and intentionality to develop the trust necessary for collaboration (Adams 2014). The second challenge stems from turning qualitative information into quantitative data; even with training and collaboration, qualitative research is painstaking when done well, and converting this knowledge into a model or validation data is even more difficult and exacting. Third is the tension between specificity and generalization in how the interest of cultural anthropologists in exploring individuals and their specific decision-making approaches may be at odds with the goal of simplification and aggregation in model development. However, approaches exist that allow researchers to overcome these challenges, such as concept mapping by using qualitative data for the conceptual modeling stage, especially when model developers serve as facilitators (Gray et al. 2015; Kragt, Robson, & Macleod 2013). More broadly, there is the promise of using ABM to develop narratives as an extension to the expectation of a bottom-up nature for these models (Perry & O’Sullivan 2018).
Modeling Frameworks
Implementing an ABM is more involved than many other modeling approaches. This is partly due to the complexity of the underlying systems that ABMs attempt to simulate and in part because the modeling frameworks themselves are complicated. However, in the last decade a number of toolkits have emerged that substantially reduce the time and cost of implementation (Table 3; see also Abar, Theodoropoulos, Lemarinier, & O’Hare 2017; Clarke 2018; Crooks & Castle 2012; Crooks, Heppenstall, & Malleson 2018; Kravari & Bassiliades 2015). Most agent-based modeling toolkits share similar features but in general there is a trade-off between ease of use (particularly for new programmers) and power; for example, Repast Simphony includes a High Performance Computing extension (North et al. 2013) but this advanced feature can only be leveraged by using the C++ language. A key decision in implementing an ABM is examining how complex and computationally expensive the final model will be, and then deciding whether the additional difficulty in learning more advanced tools will outweigh attendant performance and flexibility advantages.
Swarm | MASON | Repast | NetLogo | GAMA | ENVISION | |
Developers | Santa Fe Institute / SWARM Development Group | Evolutionary Computation Laboratory and Center for Social Complexity, George Mason University | University of Chicago, Department of Social Science Research Computing and Argonne National Laboratory | Centre for Connected Learning and Computer-Based Modeling, Northwestern University | UMMISCO, France | Oregon State University, Biological and Ecological Engineering |
Date of inception | 1996 | 2003 | 2000 | 1999 | 2007 | 2001 |
Implementation language(s) | Objective-C/Java | Java | Java, Microsoft.Net Python, Groovy, ReLogo | Scripting | Proprietary scripting: GAMA Modeling Language | C++ |
Required programming experience | Strong | Strong | Medium to Strong | Basic | Basic to Medium | Strong |
Integrated GIS functionality | Yes (e.g., Kenge GIS library for Raster data) | Yes | Yes | Yes | Yes | Yes |
Integrated charting / graphing / statistics | Yes (e.g., R and S-plus statistical packages) | Yes (e.g., wrappers for JFreeChart) | Yes | Yes | Yes | Yes |
Availability of demonstration models | Yes | Yes | Yes | Yes | Yes | Yes |
As the agent-based research community grows, so too does the range of modeling frameworks and platforms (Kravari & Bassiliades 2015). Does this expansion of frameworks constrain the development of the field, or highlight a strong and vibrant research community? Answering this question involves examining the investment of resources in using and developing SABM frameworks when compared with the development of a range of more ‘bespoke’ models developed using object-oriented programming (OOP) languages and toolboxes such as Repast (North et al. 2013). NetLogo (Wilensky 1999) is widely used within the community in part because it is self-contained and assumes no prior programming knowledge and provides constructs that allow beginners to achieve a lot with very little code (O’Sullivan & Perry 2013; Railsback & Grimm 2011). NetLogo does not include an integrated development environment (IDE), however, which can make it hard to develop large or complicated models. This may lead the modeler to explore other frameworks that better meet their needs.
More broadly, the modeler must consider the advantages and disadvantages of building a model using a conventional programming language versus using existing ABM platforms. The latter offers the advantage of avoiding the reimplementation of common elements such as graphics libraries, common algorithms, data input/output procedures, and analytical tools. Nonetheless, standard OOP languages such as Java, C++, Python, or Visual Basic form the majority of ABM frameworks, and importantly, are also used to extend these frameworks. While eschewing ABM frameworks offers the benefits of increased knowledge and confidence stemming from coding your own model, these frameworks will only grow in popularity given their lower demand for programming skills, greater reproducibility, and ability to leverage open-source improvements more generally. For example, RePast uses GeoTools, a Java GIS Toolkit that is compliant with the Open Geospatial Consortium (OGC) specifications (North et al. 2013), while MASON and NetLogo use libraries from the Java Topology Suite (Sullivan, Coletti, & Luke 2010).
The range and diversity of ABM frameworks in itself is not a problem and we will likely see new and revised approaches to developing ABMs. This process aligns with software development in a range of other areas, such as the development of new programming languages. We are also seeing a greater embrace of hybrid models, where ABMs are combined with regression, systems dynamics, automated calibration and validation metrics and other commonly used approaches (Parker et al. 2003; Wallentin 2017). For these reasons, we see the range and variation in ABM frameworks as a sign of a healthy research community, rather than an ongoing hindrance to the field, although the choice of modeling environment will always need to be well-informed and deliberate.
Lessons from spatial science: Extending spatiality in modeling frameworks
Integration of spatial science and agent-based modeling frameworks has and continues to be a significant challenge for spatial ABMs (Schüle, Herrler, & Klügl 2004; Wallentin 2017). A broad range of standard Geographic Information System (GIS) features are unevenly implemented in ABM. Paltry support for geographic representation and underlying analytics limits SABMs to simplified concepts of space (e.g., gridded cells) and basic spatial methods (e.g., distance-based thresholds) rather than richer spatial concepts (e.g., neighborhoods, safe spaces, spatial networks) and complex analytics (e.g., viewsheds and spatial clustering). Circumventing these limitations generally relies on coupling models and platforms to enable a range of GIS analyses and functions, such as using spatial functions within a statistical package like R (Thiele & Grimm 2010) or engaging in sometimes cumbersome preprocessing and postprocessing of model data in stand-alone GIS platforms. Coupling GIS and ABMs to support complex spatial structures has been used to alleviate some of these challenges (Brown et al. 2005).
There are additional challenges beyond simply representing standard spaces. A GIS tends to have poor support for time and dynamics, which are central to SABMs, and this highlights the need for greater control over the representation and analysis of space and time directly within the ABM frameworks. Network modeling has long been part of SABM but still offers only moderate integration with existing social network analysis tools such as Pajek. Similarly, most SABMs are of two-dimensional spaces but interest is growing in three-dimensional (3D) systems (e.g., ocean environments, indoor spaces, ecology). For some modelers, the problem is less about the modeling as such and more about the challenges of model interpretation with 2-D visualizations. For others, there is a need to model continuously moving agents in 3-D space, including indoor spaces such as homes, cars, and institutions like schools or hospitals. As Wallentin (2017) notes, simulation modeling frameworks have come a long way toward implementing their own spatiality.
A fully integrated modeling system/GIS would overcome many of these fundamental limitations. It would support complex geographic information representations, analytical methods, and the modeling procedures offered by spatial science. One ABM framework that integrates GIS strongly is GAMA or the GIS Agent-based Modeling Architecture (Grignard et al. 2013). GAMA is a development environment for building spatial agent-based simulations and has been used to develop ABMs that range from exploring coastal flood prevention (Becu et al. 2008) and agricultural landscapes (Thierry et al. 2017), to urban accessibility (Fosset et al. 2016). The GIS capabilities of GAMA extend to a range of useful geospatial operations, such as buffering, distances, metrics (such as Moran’s or Gini indexes), clustering, inverse distance weighting, network centrality, and coordinate transformations.
Finally, there is much potential for high performance computing (HPC) and parallel computing in SABM (Tang & Wang 2009). HPC promises to increase the spatial extent and resolution of ABM by overcoming the memory and computing limitations of the desktop environments that host most SABM (Wang 2010). Broadly, there are two ways to parallelize spatial ABM in HPC environments. The most straightforward is the ‘embarrassingly parallel’ approach of running spatial ABM independently across processors, such as when running the model multiple times for sensitivity analyses (Tang, Bennett, & Wang 2011). The second is to decompose the SABM into sub-models that are executed in parallel, which allows for simultaneous, and realistic, updating of agents, but this approach is still a research challenge because it requires conceptualizing space-time interactions closely and carefully (e.g., when agents compete for a resource, multiple agents cannot consume the same resource). SABM present significant load-balancing problems, because parts of the model run at different speeds, yet the next time step requires all elements to be updated before the model can proceed to the succeeding time step. HPC will most likely become common for SABM only when SABM frameworks are integrated into emerging spatial data science tools such as Jupyter notebooks (Shook et al. 2016; Yin et al. 2017). Achieving this form of spatial ABM-HPC platform requires ongoing research into spatiotemporal computation in general and ABM in particular (Shook et al. 2013), and perhaps even reworking of geographical information systems (Gahegan 2018).
Conclusion
Agent-based models are a class of computational models for simulating the actions, behavior, and interactions of autonomous individual or collective entities, with the goal of exploring the impact of one agent or a behavior type on the system as a whole (Clarke 2018). Spatial ABMs add geographic space and spatial distribution to the agents themselves, and to the environments in which they function. SABMs have met with considerable success in the last decade or so, and are used in a variety of disciplines and contexts with positive results.
The authors believe that the challenges and limitations of the method have received increased scrutiny in the growing literature on ABM, with some notable advancements in modeling, and in accommodating spatiality. Some of the generic issues facing SABM development relate to the inevitable choice between building standard models within common modeling systems (notably NetLogo and Repast) and building custom models within object-oriented programming languages. In either case, the modules and libraries that continue to improve have increased utility, and the amount of programming/scripting knowledge required of a potential modeler has fallen as common user interfaces and modular structures have emerged and become more sophisticated. From a technical point of view, there are now few obstacles to SABM as an alternative modeling paradigm when the circumstances dictate.
Why haven’t some of these challenges been addressed? One thought that emerged from the workshop is that researchers have been focusing on data and trying on ABMs for their novelty (especially easy-to-build toy models) and less focused on addressing methodological issues such as robust calibration and validation of these models. Complex models create complex output and the ABM community still needs appropriate tools to analyze these outputs (Ligmann-Zielinska & Sun 2010). There is also the sense that – as with many kinds of research – ABM development is siloed, and disciplines really should talk more. While we all see promise in OpenABM and the broader move towards open and shared development, there is no central community or repository akin to what climate scientists or microbiologists have for depositing code and exchanging best practices. Finally, there is an ongoing need to show that ABMs are robust enough for use by policymakers. There is great potential for these models, but they are affected by the need for validation or dynamic data assimilation that can fuse streams of new data to create real time simulations (Ward, Evans, & Malleson 2016).
Clarke (2018) noted that ABM is suited for simulations where there is no prior precedent, no past data, or when system knowledge is absent. This makes ABM subject to the accuracy of the formalization of the agents and their programmed behaviors. Bithell et al. (2008) pointed out that a principal challenge of ABM is to find sets of rules that best represent the beliefs and desires of humans represented as agents, so that they reflect the cultural context, yet still allow system exploration. This is a challenge for ABMs of physical systems, but even more so for ecological, biological, and human systems, where many unmodeled factors contribute to behavior, and behavior itself is almost always non-deterministic. Some innovations in ABMs have included adding multi-agent interactions, adding learning (modified behavior based on past experience of model behavior) during model sequences, and allowing agents to negotiate, for example using voting rules.
Parker et al. (2003) made a distinction among ABMs between 'generative' vs. 'fitting' (or fitted) models. The former involves setting the environment, then custom designing agents and their behaviors to be credible within the environment. Fitted models use outside data to design the models, again with the environment fixed. As shown, the fitting can be statistical, through calibration, or by making careful, even qualitative, judgements about the agents and their rules by observing the real actors that the agents are intended to simulate. Both of these ABM model types are attractive, yet fail to examine the interactions among the agents and their environment. A change among animal species in a forest ultimately changes the forest, just as the conversion of forest and agricultural land to urban areas impacts natural and human systems.
Yet it is at this human-environment interface where ABMs have seen some success. We note that models serve more functions than simply accurate forecasting—they are also important tools for learning and understanding the nature of systems and for exploring unobserved behaviors. Can a SABM demonstrate emergent features and behaviors that could not be discovered in other ways? Can SABMs be used to test and explore the limits of systems or their tipping points? It is the goal of exploration that holds most promise for ABMs next decade, as we enter a world profoundly changed by human action and a climate-balance seemingly irreversibly damaged. A major strength of ABM has been to serve as a conduit for interdisciplinary sciences engaging on issues facing humanity. It is our hope that spatial agent-based models can play a significant role for those seeking to learn more about, envision, and create better understanding of our changing world.
Acknowledgements
This work is supported in part by the United States National Science Foundation (NSF) Method, Measure & Statistics (MMS) and Geography and Spatial Sciences (GSS) programs (BCS #1638446); the National Institutes of Health (NIH)-supported Minnesota Population Center (R24 HD041023); NIH National Spatiotemporal Population Research Infrastructure (2R01HD057929-11); ESRC-Alan Turing Fellowship (ES/R007918/1); and the New Zealand Ministry of Business, Innovation and Employment (C09X1307). The authors gratefully acknowledge comments and input from the participants of Methodological Issues of Spatial ABMs Workshop at the ABM 17 Symposium (http://complexities.org/ABM17/) and the assistance of the editor and anonymous reviewers. Responsibility for the opinions expressed herein is solely that of the authors.References
ABAR, S., Theodoropoulos, G. K., Lemarinier, P., & O’Hare, G. M. P. (2017). Agent based modelling and simulation tools: A review of the state-of-art software. Computer Science Review, 24, 13–33. [doi:10.1016/j.cosrev.2017.03.001]
ADAMS, L. G. (2014). Putting together a scientific team: collaborative science. Trends in Microbiology, 22(9), 483–485. [doi:10.1016/j.tim.2014.05.001]
AGRAWAL, A., Brown, D. G., Rao, G., Riolo, R., Robinson, D. T., & Bommarito II, M. (2013). Interactions between organizations and networks in common-pool resource governance. Environmental Science & Policy, 25, 138–146. [doi:10.1016/j.envsci.2012.08.004]
AJELLI, M., Gonçalves, B., Balcan, D., Colizza, V., Hu, H., Ramasco, J. J., … Vespignani, A. (2010). Comparing large-scale computational approaches to epidemic modeling: agent-based versus structured metapopulation models. BMC Infectious Diseases, 10(1), 190. [doi:10.1186/1471-2334-10-190]
ALEXANDER, P., Moran, D., Rounsevell, M. D. A., & Smith, P. (2013). Modelling the perennial energy crop market: the role of spatial diffusion. Journal of the Royal Society Interface, 10(88), 20130656. [doi:10.1098/rsif.2013.0656]
AN, L. (2012). Modeling human decisions in coupled human and natural systems: Review of agent-based models. Ecological Modelling, 229, 25–36. [doi:10.1016/j.ecolmodel.2011.07.010]
AN, L., Linderman, M., Qi, J., Shortridge, A., & Liu, J. (2005). Exploring complexity in a human-environment system: an agent-based spatial model for multidisciplinary and multiscale integration. Annals of the Association of American Geographers, 95(1), 54-79. [doi:10.1111/j.1467-8306.2005.00450.x]
AN, L., Tsou, M.-H., Crook, S. E. S., Chun, Y., Spitzberg, B., Gawron, J. M., & Gupta, D. K. (2015). Space–time analysis: Concepts, quantitative methods, and future directions. Annals of the Association of American Geographers, 105(5), 891–914. [doi:10.1080/00045608.2015.1064510]
AN, L., Turner II, B. L., Jankowski, P., Wang, S., & Manson, S. M. (2017). Agent-Based Modeling 2017: Agent-Based Models in the Social, Human-Environment, and Life Sciences. San Diego, California: National Science Foundation.
ANDRIS, C. (2016). Integrating social network data into GISystems. International Journal of Geographical Information Science, 30(10), 2009–2031. [doi:10.1080/13658816.2016.1153103]
AXELROD, R. M. (1997). Advancing the Art of Simulation in the Social Sciences. Complexity, 3(2), 16–22.
AXELROD, R. M., Riolo, R. L., & Cohen, M. D. (2002). Beyond geography: Cooperation with persistent links in the absence of clustered neighborhoods. Personality and Social Psychology Review, 6(4), 341-346. [doi:10.1207/s15327957pspr0604_08]
BALA, V., & Sorger, G. (2001). A spatial-temporal model of human capital accumulation. Journal of Economic Theory, 96(1–2), 153–179. [doi:10.1006/jeth.1999.2629]
BARNAUD, C., Le Page, C., Dumrongrojwatthana, P., & Trébuil, G. (2013). Spatial representations are not neutral: Lessons from a participatory agent-based modelling process in a land-use conflict. Environmental Modelling & Software, 45, 150–159. [doi:10.1016/j.envsoft.2011.11.016]
BARTHÉLEMY, M. (2011). Spatial networks. Physics Reports, 499(1–3), 1–101.
BATTY, M. (2008). Generative social science: a challenge. Environment and Planning B: Planning and Design, 32(2), 191–194.
BATTY, M., & Torrens, P. (2005). Modelling and prediction in a complex world. Futures, 37(7), 745–766. [doi:10.1016/j.futures.2004.11.003]
BECU, N., Neef, A., Schreinemachers, P., & Sangkapitux, C. (2008). Participatory computer simulation to support collective decision-making: Potential and limits of stakeholder involvement. Land Use Policy, 25(4), 498–509. [doi:10.1016/j.landusepol.2007.11.002]
BIRCH, C. P. D., Oom, S. P., & Beecham, J. A. (2007). Rectangular and hexagonal grids used for observation, experiment and simulation in ecology. Ecological Modelling, 206(3–4), 347–359. [doi:10.1016/j.ecolmodel.2007.03.041]
BITHELL, M., Brasington, J., & Richards, K. (2008). Discrete-element, individual-based and agent-based models: Tools for interdisciplinary enquiry in geography? Geoforum, 39(2), 625–642. [doi:10.1016/j.geoforum.2006.10.014]
BOLTE, J., Hulse, D., Gregory, S., & Smith, C. (2007). Modeling biocomplexity – actors, landscapes and alternative futures. Environmental Modelling & Software, 22(5), 570–579. [doi:10.1016/j.envsoft.2005.12.033]
BROWN, D. G., Page, S. E., Riolo, R., Zellner, M., & Rand, W. (2005). Path dependence and the validation of agent-based spatial models of land use. International Journal of Geographical Information Science, 19(2), 153–174. [doi:10.1080/13658810410001713399]
BROWN, D. G., Riolo, R., Robinson, D. T., North, M., & Rand, W. (2005). Spatial process and data models: Toward integration of agent-based models and GIS. Journal of Geographical Systems, 7(1), 25-47. [doi:10.1007/s10109-005-0148-5]
CLARKE, K. C. (2003). Geocomputation’s future at the extremes: high performance computing and nanoclients. Parallel Computing, 29(10), 1281–1295. [doi:10.1016/j.parco.2003.03.001]
CLARKE, K. C. (2004). The limits of simplicity: toward geocomputational honesty in urban modeling. In P. Atkinson, G. Foody, S. Darby, & F. Wu (Eds.), GeoDynamics (pp. 213–232). Boca Raton, FL: CRC Press. [doi:10.1201/9781420038101.ch16]
CLARKE, K. C. (2018). Cellular automata and agent-based models. In Handbook of regional science (pp. 1217–1233). New York: Springer. [doi:10.1007/978-3-642-23430-9_63]
COUCLELIS, H. (2001). Why I no longer work with Agents. In D. C. Parker, T. Berger, & S. M. Manson (Eds.), Special Workshop on Agent-Based Models of Land-Use, Land-Cover Change (pp. 3–5). LUCC Report Series.
COUCLELIS, H. (2010). Ontologies of geographic information. International Journal of Geographical Information Science, 24(12), 1785–1809. [doi:10.1080/13658816.2010.484392]
CROOKS, A. T., Castle, C., & Batty, M. (2008). Key challenges in agent-based modelling for geo-spatial simulation. Computers, Environment and Urban Systems, 32(6), 417–430. [doi:10.1016/j.compenvurbsys.2008.09.004]
CROOKS, A. T., & Castle, C. J. E. (2012). ‘The integration of agent-based modelling and geographical information for geospatial simulation.’ In A. J. Heppenstall, A. T. Crooks, L. M. See, & M. Batty (Eds.), Agent-Based Models of Geographical Systems. Dordrecht, Netherlands: Springer, pp. 219-251. [doi:10.1007/978-90-481-8927-4_12]
CROOKS, A. T., & Heppenstall, A. J. (2012). ‘Introduction to agent-based modelling.’ In A. J. Heppenstall, A. T. Crooks, L. M. See, & M. Batty (Eds.), Agent-Based Models of Geographical Systems. Dordrecht, Netherlands: Springer, pp. 85–105. [doi:10.1007/978-90-481-8927-4_5]
CROOKS, A. T., Heppenstall, A. J., & Malleson, N. (2018). ‘Agent-based modelling.’ In B. Huang (Ed.), Comprehensive Geographic Information Systems. Berlin: Springer, pp. 218–243. [doi:10.1016/b978-0-12-409548-9.09704-9]
DAINTON, B. (2001). Time and Space. Kingston, Canada: McGill-Queen’s University Press.
DEANGELIS, D. L., & Grimm, V. (2014). Individual-based models in ecology after four decades. F1000prime Reports, 6. [doi:10.12703/p6-39]
DROGOUL, A., & Ferber, J. (1999). ‘Multi-agent simulation as a tool for studying emergent processes in societies.’ In N Gilbert & J. Doran (Eds.), Simulating Societies: The Computer Simulation of Social Phenomena. London: UCL Press, pp. 127–142. [doi:10.4324/9781351165129-6]
EPSTEIN, J. M. (1999). Agent-based computational models and generative social science. Complexity, 4(5), 41–60. [doi:10.1002/(sici)1099-0526(199905/06)4:5<41::aid-cplx9>3.0.co;2-f]
EPSTEIN, J. M., & Axtell, R. (1996). Growing Artificial Societies: Social Science From the Bottom Up. Washington, D.C.: Brookings Institution Press. [doi:10.7551/mitpress/3374.001.0001]
EVANS, T. P., & Kelley, H. (2004). Multi-scale analysis of a household level agent-based model of landcover change. Journal of Environmental Management, 72(1–2), 57–72. [doi:10.1016/j.jenvman.2004.02.008]
EVANS, T. P., Phanvilay, K., Fox, J., & Vogler, J. (2011). An agent-based model of agricultural innovation, land-cover change and household inequality: the transition from swidden cultivation to rubber plantations in Laos PDR. Journal of Land Use Science, 6(2–3), 151–173. [doi:10.1080/1747423x.2011.558602]
FILATOVA, T. (2015). Empirical agent-based land market: Integrating adaptive economic behavior in urban land-use models. Computers, Environment and Urban Systems, 54, 397–413. [doi:10.1016/j.compenvurbsys.2014.06.007]
FILATOVA, T., Verburg, P. H., Parker, D. C., & Stannard, C. A. (2013). Spatial agent-based models for socio-ecological systems: Challenges and prospects. Environmental Modelling & Software, 45, 1–7. [doi:10.1016/j.envsoft.2013.03.017]
FOSSET, P., Banos, A., Beck, E., Chardonnel, S., Lang, C., Marilleau, N., … Andre-Poyaud, I. (2016). Exploring intra-urban accessibility and impacts of pollution policies with an agent-based simulation platform: GaMiroD. Systems, 4(1), 5. [doi:10.3390/systems4010005]
FOX, J., Suryanata, K., Hershock, P., & Pramono, A. H. (2006). Mapping power: ironic effects of spatial information technology. Participatory Learning and Action, 54(1), 98–105.
GAHEGAN, M. (2018). Our GIS is too small. The Canadian Geographer/Le Géographe Canadien, 62(1), 15–26. [doi:10.1111/cag.12434]
GILBERT, N. (2008). Agent-Based Models. Thousand Oaks, California: Sage.
GILBERT, N., Pyka, A., & Ahrweiler, P. (2001). Innovation networks-a simulation approach. Journal of Artificial Societies and Social Simulation, 4(3), 8: https://www.jasss.org/4/3/8.html.
GILBERT, N., & Troitzsch, K. G. (2005). Simulation for the Social Scientist (2nd ed.). Open Universisty Press.
GRAY, S. A., Gray, S., De Kok, J. L., Helfgott, A. E. R., O’Dwyer, B., Jordan, R., & Nyaki, A. (2015). Using fuzzy cognitive mapping as a participatory approach to analyze change, preferred states, and perceived resilience of social-ecological systems. Ecology and Society, 20(2). [doi:10.5751/es-07396-200211]
GRIGNARD, A., Taillandier, P., Gaudou, B., Vo, D. A., Huynh, N. Q., & Drogoul, A. (2013). GAMA 1.6: Advancing the art of complex agent-based modeling and simulation. In International Conference on Principles and Practice of Multi-Agent Systems (pp. 117–131). Springer. [doi:10.1007/978-3-642-44927-7_9]
GRIMM, V. (1999). Ten years of individual-based modelling in ecology: what have we learned and what could we learn in the future? Ecological Modelling, 115(2–3), 129–148. [doi:10.1016/s0304-3800(98)00188-4]
GRIMM, V., Augusiak, J., Focks, A., Frank, B. M., Gabsi, F., Johnston, A. S. A., … Radchuk, V. (2014). Towards better modelling and decision support: documenting model development, testing, and analysis using TRACE. Ecological Modelling, 280, 129–139. [doi:10.1016/j.ecolmodel.2014.01.018]
GRIMM, V., Berger, U., DeAngelis, D. L., Polhill, J. G., Giske, J., & Railsback, S. F. (2010). The ODD protocol: a review and first update. Ecological Modelling, 221(23), 2760–2768. [doi:10.1016/j.ecolmodel.2010.08.019]
GRIMM, V., Revilla, E., Berger, U., Jeltsch, F., Mooij, W. M., Railsback, S. F., … DeAngelis, D. L. (2005). Pattern-Oriented Modeling of Agent-Based Complex Systems: Lessons from Ecology. Science, 310(5750), 987–991. [doi:10.1126/science.1116681]
HAGERSTRAND, T. (1982). Diorama, path and project. Tijdschrift Voor Economische En Sociale Geografie, 73(6), 323–339. [doi:10.1111/j.1467-9663.1982.tb01647.x]
HAMMAM, Y., Moore, A., & Whigham, P. (2007). The dynamic geometry of geographical vector agents. Computers, Environment and Urban Systems, 31(5), 502–519. [doi:10.1016/j.compenvurbsys.2007.08.003]
HEGSELMANN, R. (2017). Thomas C. Schelling and James M. Sakoda: The intellectual, technical, and social history of a model. Journal of Artificial Societies and Social Simulation, 20(3), 15: https://www.jasss.org/20/3/15.html. [doi:10.18564/jasss.3511]
HEROD, A. (2010). Scale. New York: Routledge.
ILLENBERGER, J., Nagel, K., & Flötteröd, G. (2013). The role of spatial interaction in social networks. Networks and Spatial Economics, 13(3), 255–282. [doi:10.1007/s11067-012-9180-4]
JJUMBA, A., & Dragicevic, S. (2016). A development of spatiotemporal queries to analyze the simulation outcomes from a voxel automata model. Earth Science Informatics, 9(3), 343–353. [doi:10.1007/s12145-016-0260-8]
KLUGL, F., Herrler, R., & Andriotti, G. K. (2006). Coupling GIS and multi-agent simulation - towards infrastructure for realistic simulation. Computer Systems Science and Engineering, 21(3), 197.
KOCH, J., Dorning, M. A., Van Berkel, D. B., Beck, S. M., Sanchez, G. M., Shashidharan, A., … Meentemeyer, R. K. (2019). Modeling landowner interactions and development patterns at the urban fringe. Landscape and Urban Planning, 182, 101–113. [doi:10.1016/j.landurbplan.2018.09.023]
KRAGT, M. E., Robson, B. J., & Macleod, C. J. A. (2013). Modellers’ roles in structuring integrative research projects. Environmental Modelling & Software, 39, 322–330. [doi:10.1016/j.envsoft.2012.06.015]
KRAVARI, K., & Bassiliades, N. (2015). A survey of agent platforms. Journal of Artificial Societies and Social Simulation, 18(1), 11: https://www.jasss.org/18/1/11.html. [doi:10.18564/jasss.2661]
LIGMANN-ZIELINSKA, A., & Sun, L. (2010). Applying time-dependent variance-based global sensitivity analysis to represent the dynamics of an agent-based model of land use change. International Journal of Geographical Information Science, 24(12), 1829–1850. [doi:10.1080/13658816.2010.490533]
LORSCHEID, I., Berger, U., Grimm, V., & Meyer, M. (2019). From cases to general principles: A call for theory development through agent-based modeling. Ecological Modelling, 393, 153-156. [doi:10.1016/j.ecolmodel.2018.10.006]
MACY, M. W., & Willer, R. (2002). From factors to actors: computational sociology and agent-based modeling. Annual Review of Sociology, 28, 143–166. [doi:10.1146/annurev.soc.28.110601.141117]
MANSON, S. M. (2007). Challenges in evaluating models of geographic complexity. Environment and Planning B, 34(2), 245–260.
MANSON, S. M. (2008). Does scale exist? An epistemological scale continuum for complex human-environment systems. Geoforum, 39(2), 776–788. [doi:10.1016/j.geoforum.2006.09.010]
MANSON, S. M., Jordan, N. R., Nelson, K. C., & Brummel, R. F. (2016). Modeling the effect of social networks on adoption of multifunctional agriculture. Environmental Modelling and Software, 75, 388–401. [doi:10.1016/j.envsoft.2014.09.015]
MANSON, S. M., & O’Sullivan, D. (2006). Complexity theory in the study of space and place. Environment and Planning A, 38(4), 677–692. [doi:10.1068/a37100]
MANSON, S. M., Sun, S., & Bonsal, D. (2012). ‘Agent-based modeling and complexity.’ In A. J. Heppenstall, A. T. Crooks, L. M. See, & M. Batty (Eds.), Agent-Based Models of Geographical Systems. Dordrecht, Netherlands: Springer, pp. 125–139. [doi:10.1007/978-90-481-8927-4_7]
MASSEY, D. (2005). For Space. Thousand Oaks, California: Sage.
MILLINGTON, J. D. A., O’Sullivan, D., & Perry, G. L. W. (2012). Model histories: Narrative explanation in generative simulation modelling. Geoforum, 43(6), 1025–1034. [doi:10.1016/j.geoforum.2012.06.017]
MORGAN, F. J., & Daigneault, A. J. (2015). Estimating impacts of climate change policy on land use: An agent-based modelling approach. PloS One, 10(5), e0127317. [doi:10.1371/journal.pone.0127317]
MOSS, S., & Edmonds, B. (2005). Towards Good Social Science. Journal of Artificial Societies and Social Simulation, 8(4), 13: https://www.jasss.org/20/3/15.html.
MÜLLER, B., Bohn, F., Dreßler, G., Groeneveld, J., Klassert, C., Martin, R., … Schwarz, N. (2013). Describing human decisions in agent-based models–ODD+ D, an extension of the ODD protocol. Environmental Modelling & Software, 48, 37–48. [doi:10.1016/j.envsoft.2013.06.003]
MUNROE, D. K., McSweeney, K., Olson, J. L., & Mansfield, B. (2014). Using economic geography to reinvigorate land-change science. Geoforum, 52, 12–21. [doi:10.1016/j.geoforum.2013.12.005]
NORTH, M. J., Collier, N. T., Ozik, J., Tatara, E. R., Macal, C. M., Bragen, M., & Sydelko, P. (2013). Complex adaptive systems modeling with Repast Simphony. Complex Adaptive Systems Modeling, 1(1), 3. [doi:10.1186/2194-3206-1-3]
O’SULLIVAN, D. (2005). Geographical information science: time changes everything. Progress in Human Geography, 29(6), 749–756.
O’SULLIVAN, D. (2009). Changing neighborhoods—neighborhoods changing: a framework for spatially explicit agent-based models of social systems. Sociological Methods & Research, 37(4), 498–530.
O’SULLIVAN, D., Evans, T. P., Manson, S. M., Metcalf, S., Ligmann-Zielinska, A., & Bone, C. (2016). Strategic directions for agent-based modeling: avoiding the YAAWN syndrome. Journal of Land Use Science, 11(2), 177–187. [doi:10.1080/1747423x.2015.1030463]
O’SULLIVAN, D., & Manson, S. M. (2015). Do Physicists Have ‘Geography Envy’? And What Can Geographers Learn From It? Annals of the Association of American Geographers, 105(4), 704–722. [doi:10.1080/00045608.2015.1039105]
O’SULLIVAN, D., Manson, S. M., Messina, J. P., & Crawford, T. W. (2006). Space, place, and complexity science. Environment and Planning A, 38(4), 611–617.
O’SULLIVAN, D., Millington, J., Perry, G., & Wainwright, J. (2012). Agent-based models–because they’re worth it? In A. J. Heppenstall, A. T. Crooks, L. M. See, & M. Batty (Eds.), Agent-based Models of Geographical Systems (pp. 109–123). Dordrecht, Netherlands: Springer. [doi:10.1007/978-90-481-8927-4_6]
O’SULLIVAN, D., & Perry, G. L. W. (2013). Spatial Simulation: Exploring Pattern and Process. London, United Kingdom: John Wiley & Sons.
PARKER, D. C., Manson, S. M., Janssen, M., Hoffmann, M. J., & Deadman, P. J. (2003). Multi-agent systems for the simulation of land use and land cover change: A review. Annals of the Association of American Geographers, 93(2), 316–340. [doi:10.1111/1467-8306.9302004]
PARRY, H. R., & Bithell, M. (2012). ‘Large scale agent-based modelling: A review and guidelines for model scaling.’ In A. J. Heppenstall, A. T. Crooks, L. M. See, & M. Batty (Eds.), Agent-Based Models of Geographical Systems. Dordrecht, Netherlands: Springer, pp. 271–308. [doi:10.1007/978-90-481-8927-4_14]
PERRY, G. L. W., & O’Sullivan, D. (2018). Identifying Narrative Descriptions in Agent-Based Models Representing Past Human-Environment Interactions. Journal of Archaeological Method and Theory, 25(3), 795–817. [doi:10.1007/s10816-017-9355-x]
PEUQUET, D. J., & Duan, N. (1995). An event-based spatiotemporal data model (ESTDM) for temporal analysis of geographical data. International Journal of Geographical Information Systems, 9(1), 7–24. [doi:10.1080/02693799508902022]
PHILLIPS, J. D. (2012). Synchronization and scale in geomorphic systems. Geomorphology, 137(1), 150–158. [doi:10.1016/j.geomorph.2010.09.028]
POLHILL, J. G., Parker, D. C., Brown, D., & Grimm, V. (2008). Using the ODD Protocol for Describing Three Agent-Based Social Simulation Models of Land-Use Change. Journal of Artificial Societies and Social Simulation, 11(2), 3: https://www.jasss.org/11/2/3.html. [doi:10.18564/jasss.1563]
RAILSBACK, S. F., & Grimm, V. (2011). Agent-Based and Individual-Based Modeling: A Practical Introduction. Princeton, New Jersey: Princeton University Press.
RILEY, S. (2007). Large-scale spatial-transmission models of infectious disease. Science, 316(5829), 1298–1301. [doi:10.1126/science.1134695]
RIOLO, R., Cohen, M., & Axelrod, R. M. (2001). Cooperation without reciprocity. Nature, 414(6862), 441. [doi:10.1038/35106555]
ROBINSON, D. T., Brown, D. G., Parker, D. C., Schreinemachers, P., Janssen, M. A., Huigen, M., … Barnaud, C. (2007). Comparison of empirical methods for building agent-based models in land use science. Journal of Land Use Science, 2(1), 31–55. [doi:10.1080/17474230701201349]
RUXTON, G. D. (1996). Effects of the spatial and temporal ordering of events on the behaviour of a simple cellular automaton. Ecological Modelling, 84(1–3), 311–314. [doi:10.1016/0304-3800(94)00145-6]
SAKODA, J. M. (1971). The checkerboard model of social interaction. Journal of Mathematical Sociology, 1, 119–132.
SCHELLING, T. C. (1971). On the ecology of micromotives. The Public Interest, 25(Fall), 61–98.
SCHÜLE, M., Herrler, R., & Klügl, F. (2004). ‘Coupling gis and multi-agent simulation–towards infrastructure for realistic simulation.’ In Multiagent System Technologies. Cham, Switzerland: Springer, pp. 228–242. [doi:10.1007/978-3-540-30082-3_17]
SCHULZE, J., Muller, B., Groeneveld, J., & Grimm, V. (2017). Agent-Based Modelling of Social-Ecological Systems: Achievements, Challenges, and a Way Forward. Journal of Artificial Societies and Social Simulation, 20(2), 8: https://www.jasss.org/20/2/8.html. [doi:10.18564/jasss.3423]
SHELTON, T., Poorthuis, A., & Zook, M. (2015). Social media and the city: Rethinking urban socio-spatial inequality using user-generated geographic information. Landscape and Urban Planning, 142, 198–211. [doi:10.1016/j.landurbplan.2015.02.020]
SHOOK, E., Hodgson, M. E., Wang, S., Behzad, B., Soltani, K., Hiscox, A., & Ajayakumar, J. (2016). Parallel cartographic modeling: a methodology for parallelizing spatial data processing. International Journal of Geographical Information Science, 30(12), 2355–2376. [doi:10.1080/13658816.2016.1172714]
SHOOK, E., & Wang, S. (2015). Investigating the Influence of Spatial and Temporal Granularities on Agent‐Based Modeling. Geographical Analysis, 47(4), 321–348. [doi:10.1111/gean.12080]
SHOOK, E., Wang, S., & Tang, W. (2013). A communication-aware framework for parallel spatially explicit agent-based models. International Journal of Geographical Information Science, 27(11), 2160–2181. [doi:10.1080/13658816.2013.771740]
SPIES, T. A., White, E., Ager, A., Kline, J. D., Bolte, J. P., Platt, E. K., … Bailey, J. D. (2017). Using an agent-based model to examine forest management outcomes in a fire-prone landscape in Oregon, USA. Ecology and Society, 22(1). [doi:10.5751/es-08841-220125]
STANILOV, K. (2012). ‘Space in agent-based models.’ In A. J. Heppenstall, A. T. Crooks, L. M. See, & M. Batty (Eds.), Agent-Based Models of Geographical Systems. Dordrecht, Netherlands: Springer, pp. 253–269. [doi:10.1007/978-90-481-8927-4_13]
STILLMAN, R. A., Railsback, S. F., Giske, J., Berger, U., & Grimm, V. (2015). Making predictions in a changing world: the benefits of individual-based ecology. BioScience, 65(2), 140–150. [doi:10.1093/biosci/biu192]
SULLIVAN, K., Coletti, M., & Luke, S. (2010). GeoMason: Geospatial support for MASON. Fairfax, VA: George Mason University Technical Report.
TANG, W., & Bennett, D. A. (2010). Agent‐based modeling of animal movement: a review. Geography Compass, 4(7), 682–700. [doi:10.1111/j.1749-8198.2010.00337.x]
TANG, W., Bennett, D. A., & Wang, S. (2011). A parallel agent-based model of land use opinions. Journal of Land Use Science, 6(2–3), 121–135. [doi:10.1080/1747423x.2011.558597]
TANG, W., & Wang, S. (2009). HPABM: A hierarchical parallel simulation framework for spatially‐explicit agent‐based models. Transactions in GIS, 13(3), 315–333. [doi:10.1111/j.1467-9671.2009.01161.x]
TESFATSION, L. (2002). Economic agents and markets as emergent phenomena. Proceedings of the National Academy of Sciences, 99(90003), 7191–7192. [doi:10.1073/pnas.072079199]
TESFATSION, L. (2017). Modeling Economic Systems as Locally-Constructive Sequential Games. Journal of Economic Methodology, 24(4), 384-409. [doi:10.1080/1350178x.2017.1382068]
TESFATSION, L. (2018). Agent-Based Computational Economics: Growing Economies from the Bottom Up. Retrieved from http://www2.econ.iastate.edu/tesfatsi/ace.htm. [doi:10.2139/ssrn.305080]
TESFATSION, L., Rehmann, C. R., Cardoso, D. S., Jie, Y., & Gutowski, W. J. (2017). An agent-based platform for the study of watersheds as coupled natural and human systems. Environmental Modelling & Software, 89, 40–60. [doi:10.1016/j.envsoft.2016.11.021]
THIELE, J. C., & Grimm, V. (2010). NetLogo meets R: Linking agent-based models with a toolbox for their analysis. Environmental Modelling & Software, 25(8), 972–974. [doi:10.1016/j.envsoft.2010.02.008]
THIERRY, H., Vialatte, A., Choisis, J.-P., Gaudou, B., Parry, H., & Monteil, C. (2017). Simulating spatially-explicit crop dynamics of agricultural landscapes: The ATLAS simulator. Ecological Informatics, 40, 62–80. [doi:10.1016/j.ecoinf.2017.05.006]
TOBIAS, R., & Hofmann, C. (2004). Evaluation of free Java-libraries for social-scientific agent based simulation. Journal of Artificial Societies and Social Simulation, 7(1),6: https://www.jasss.org/7/1/6.html.
TOBLER, W. R. (1975). Cellular Geography. Philosophy in Geography, 379–386.
TORRENS, P. M. (2010). Agent-based Models and the Spatial Sciences. Geography Compass, 4(5), 428–448. [doi:10.1111/j.1749-8198.2009.00311.x]
TORRENS, P. M. (2014). High-resolution space–time processes for agents at the built–human interface of urban earthquakes. International Journal of Geographical Information Science, 28(5), 964–986. [doi:10.1080/13658816.2013.835816]
TURNER, M. G., O’Neill, R. V, Gardner, R. H., & Milne, B. T. (1989). Effects of changing spatial scale on the analysis of landscape pattern. Landscape Ecology, 3(3), 153–162. [doi:10.1007/bf00131534]
VALBUENA, D., Verburg, P. H., Veldkamp, A., Bregt, A. K., & Ligtenberg, A. (2010). Effects of farmers’ decisions on the landscape structure of a Dutch rural region: An agent-based approach. Landscape and Urban Planning, 97(2), 98–110. [doi:10.1016/j.landurbplan.2010.05.001]
VAN Nes, E. H., & Scheffer, M. (2005). A strategy to improve the contribution of complex simulation models to ecological theory. Ecological Modelling, 185(2), 153–164. [doi:10.1016/j.ecolmodel.2004.12.001]
VOINOV, A., & Bousquet, F. (2010). Modelling with stakeholders. Environmental Modelling & Software, 25(11), 1268–1281. [doi:10.1016/j.envsoft.2010.03.007]
WALLENTIN, G. (2017). Spatial simulation: A spatial perspective on individual-based ecology—A review. Ecological Modelling, 350, 30–41. [doi:10.1016/j.ecolmodel.2017.01.017]
WANG, S. (2010). A CyberGIS framework for the synthesis of cyberinfrastructure, GIS, and spatial analysis. Annals of the Association of American Geographers, 100(3), 535–557. [doi:10.1080/00045601003791243]
WARD, J. A., Evans, A. J., & Malleson, N. S. (2016). Dynamic calibration of agent-based models using data assimilation. Royal Society Open Science, 3(4), 150703. [doi:10.1098/rsos.150703]
WEAVER, W. (1968). Science and Complexity. American Scientist, 36, 536–544.
WILENSKY, U. (1999). NetLogo. Evanston, IL: Center for Connected Learning and Computer-Based Modeling, Northwestern University. Retrieved from http://ccl.northwestern.edu/netlogo/.
WILENSKY, U., & Rand, W. (2015). An introduction to agent-based modeling: modeling natural, social, and engineered complex systems with NetLogo. Boston, Massachusetts: MIT Press.
WOOLDRIDGE, M., & Jennings, N. R. (1995). Intelligent Agents: Theory and practice. Knowledge Engineering Review, 10, 115–152. [doi:10.1017/s0269888900008122]
YANG, L., & Gilbert, N. (2008). Getting away from numbers: Using qualitative observation for agent-based modeling. Advances in Complex Systems, 11(2), 175–185. [doi:10.1142/s0219525908001556]
YIN, D., Liu, Y., Padmanabhan, A., Terstriep, J., Rush, J., & Wang, S. (2017). ‘A CyberGIS-Jupyter Framework for Geospatial Analytics at Scale.’ In Proceedings of the Practice and Experience in Advanced Research Computing 2017 on Sustainability, Success and Impact. New York: ACM Press, p. 18. [doi:10.1145/3093338.3093378]