Sustaining Collective Action in Urban Community Gardens

, , ,
and
aDelft University of Technology, Netherlands; bCML Industrial Ecology, Leiden University, Netherlands; cMartin-Luther-University Halle-Wittenberg, Germany
Journal of Artificial
Societies and Social Simulation 24 (3) 3
<https://www.jasss.org/24/3/3.html>
DOI: 10.18564/jasss.4506
Received: 02-Mar-2020 Accepted: 20-Apr-2021 Published: 30-Jun-2021
Abstract
This paper presents an agent-based model that explores the conditions for ongoing participation in community gardening projects. We tested the effects of Ostrom's well-known Design Principles for collective action and used an extensive database collected in 123 cases in Germany and two case studies in the Netherlands to validate it. The model used the Institutional Analysis and Development (IAD) framework and integrated decision mechanisms derived from the Theory of Reasoned Action (TRA). This allowed us to analyse volunteer participation in urban community gardens over time, based on the garden's institutions (Design Principles) and the volunteer's intention to join gardening. This intention was influenced by the volunteer's expectations and past experiences in the garden (TRA). We found that not all Design Principles lead to higher levels of participation but rather, participation depends on specific combinations of the Design Principles. We highlight the need to update the assumption about sanctioning in such systems: sanctioning is not always beneficial, and may be counter-productive in certain contexts.Introduction
Urban resilience has been defined as "the ability of an urban system and all its constituent socio-ecological and socio-technical networks across temporal and spatial scales to maintain or rapidly return to desired functions in the face of a disturbance, to adapt to change, and to quickly transform systems that limit current or future adaptive capacity" (Meerow et al. 2016). Because cities are complex systems, achieving urban resilience is not simple. In the urban physical environment, the use of space must deal with a context of diversity, anonymity and change (Huron 2017). Among the challenges to be faced, we highlight the increasing social stratification and unequal allocation of resources (Chelleri et al. 2015; Sassen 2011). Short circuit economies provide forms of sociality and co-production, necessary to survive under global financial fluxes, which can lead to community social resilience (Derkzen et al. 2017; Petrescu et al. 2016).
Community initiatives are a good example of such local economies. By community, we mean an organised group of people willing to contribute on an equal-to-equal basis to voluntary collective action in order to gain tangible or intangible benefits (Foster 2011; Schauppenlehner-Kloyber & Penker 2016). Such initiatives, in cities, are examples of political processes involving society at large, in a more or less self-organised way, which can lead to urban resilience (Anderies 2014; Kim & Lim 2016). Urban citizens are engaging more and more in collectively managed shared spaces and resources: the urban commons (Foster 2011). The commons are the result of the old practice of community management of natural resources. They have greatly diversified to embrace both tangible and intangible resources (Hess 2008). Community gardens are a well-established example of urban commons: they are spaces mainly dedicated to growing crops, and which are managed and operated by members of the local community. As other urban commons, the focus is on the open space being managed collectively by members crafting their own rules, rather than on specific ownership rights (Colding & Barthel 2013). While subsistence-driven collective action holds in certain countries, these spaces are more and more recognised for proposing alternative ecosystems to urban consumerism (Bowers 2009), enhancing well-being (Robson et al. 2015) and connecting to nature (Łapniewska 2017).
Recent research has shown the social benefits of urban community gardens: health, well-being, education, knowledge, food security, environmental justice, social cohesion and social capital (Rogge et al. 2018; Safransky 2017; Łapniewska 2017). This gives way to multiple motivations to participate. Like other urban commons, urban community gardens can be managed top-down by the local government, with the active participation of the citizens, or in a bottom-up way (Schauppenlehner-Kloyber & Penker 2016). In most cases, and traditionally, such gardens rely on self-organisation and collective action, independently from government management and without the strict requirement of private ownership (Foster & Iaione 2015). Such self-organisation relies on rules which are agreed upon by the garden users. These rules influence interactions between the garden users and the success of the initiative: keeping the community active (often volunteers) and maintaining the resource (garden). Urban community gardens can thus be seen as complex systems. In the self-organisation scenario, urban gardening is relatively independent from periodic municipal changes and that makes it a relevant case study for urban resilience (Colding & Barthel 2013).
However, urban community gardens face other difficulties. A summary of studies on community gardens mentions volunteer drop off as a challenge that community gardens face, for example because of land access issues, soil contamination, lack of water, safety issues, funding, cultural differences issues, neighbourhood complaints and waiting lists (Guitart et al. 2012). Some volunteers may take more yield than others, thus creating tensions (Charles 2012). These issues can diminish the willingness of the whole group to contribute, which harms the functioning of collective management (Butler 2013). While some communities have successfully surmounted them, in many cases low participation remains a weakness. In that sense, they present features that are also found in Common-Pool Resources (CPR): it is difficult or undesirable to exclude people from the resource, and the consumption of one user diminishes the possibilities for other users (Ostrom et al. 1994a; Ostrom 2005). One of the issues commonly found in CPRs is free-riding, or "taking without giving". The study of institutions could bring understanding to such issues. By institutions, we mean an ensemble of rules, prescriptive or constraining, set by a group of individuals in order to organise repetitive and structured interactions (Ostrom 1990).
Major contributions within this field were made by the Nobel laureate Elinor Ostrom. Thanks to numerous case studies of collective resources management across the world, such as fisheries, forestries and irrigation systems, Ostrom has proposed 8 Design Principles which act as guidelines for robust collective resources management (Agrawal 2002; Cox et al. 2010; Ostrom 2005; Ostrom 2009a). Assuming a similar collective management of urban community gardens, it is still unclear how Ostrom’s Design Principles could affect community involvement. To the best of our knowledge, apart from a recent Master thesis, which hinted that the Design Principles could be used to study urban community gardens (Butler 2013), there is no published work which discusses such link. Secondly, urban gardening is also cited for its strong social value, for example through a sense of conviviality, recreation, education and well-being. Particularly in higher income regions, community gardens’ social functions are more often prevailing (Rogge et al. 2018). We suppose that such motivations affect the community involvement.
In this paper, we investigate whether applying the Design Principles actually helps to sustain urban community gardens. By sustaining, we mean maintaining volunteer participation over time. We use Agent-Based Modelling (ABM) to study the effect of Ostrom’s Design Principles on the evolution of the garden’s volunteer participation. ABM allows more exploration of the institutional and human arrangements which can influence gardening participation. We calibrate the model using empirical data and literature. In the next section, we introduce the useful theories along with our empirical data. Subsequently, we explain the dynamics of our model, before presenting and discussing its results.
Theoretical Background
An urban community garden represents a socio-ecological system with the following components: the resource system (the garden), the resource units (garden yield and added social value), the users (volunteers coming to garden) and the governance system (institutions for the community management of the garden) (Ostrom 2009b). Participation in urban community gardens is a matter of collective and individual decision-making in a social-ecological system, where potential participants need to:
- be motivated enough to join in the gardening activity ; we assume the participants’ motivations to be multiple and to evolve over time;
- be satisfied with their experience in the garden ; we assume that this experience depends on
- the state of the garden (availability of resources)
- the garden community’s institutions (rules to which participants should abide to)
- the other participants (number of participants and their behaviour)
We leave aside the dynamics of the physical resource, to focus exclusively on the participants’ behaviour and decisions. We study the evolution of a system where, at a given instant, users decide whether or not to participate and assess the outcomes of that participation. We describe in the following subsections which theories we choose in order to deal with the motivation issue, the garden institutions and the effect of other participants.
The institutional analysis and development (IAD) framework
The IAD framework is a descriptive framework originally designed to study systems of self-governance. It helps to understand the way in which institutions operate and change over time within such systems. It is in particular relevant in the field of CPR management (Anderies & Janssen 2016). We apply the IAD at the operational level of governance, which focuses on the practical decisions of the individuals who take certain actions as a consequence of collective choice processes.
The IAD is centred around the action arena, where decision-making takes place: actors (potential participants) choose from several actions (Ostrom et al. 1994b) (Figure 1). The composition of the action arena depends on explicit external variables, which define the physical system, on the characteristics of the community of potential participants and on the rules-in-use (or institutions). An action arena consists of several action situations. In each action situation, diverse actions and participants can be specified for the chosen level of governance (here operational). Action situations capture decision-making processes and assign actions to participants. The IAD has later been simplified by Ostrom (2011) to only keep an action situation box. However, this simplification still enables describing its components in detail. From here on, we use the concept of action situations instead of action arena for the sake of modelling.
The action situations lead to certain patterns of interaction which can be evaluated by the participants, for example in terms of effectiveness, cost or sustainability. This evaluation potentially modifies not only the initial properties, but also the possible action situations and participants in the future.
The IAD framework is very relevant for this study as it provides a strong basis for the conceptualisation of an agent-based model (Ghorbani et al. 2013). In particular, the external variables can be used to describe the state of the garden, the community of potential participants and the institutions in place (or rules in use). Furthermore, it allows us to keep track of the link between various variables, especially the institutions and the motivations to join gardening.
Ostrom’s design principles
These principles help design institutions for robust collective resource management (Cox et al. 2010). We use them to frame our garden institutions: they correspond to the Rules-In-Use that influence the action situations. These principles can be applied in various degrees to design institutions for urban community gardens which we explain in Table 1.
Design Principles (name) | Definition and use in existing literature |
---|---|
Spatial boundaries | They delineate the realm of application of internal rules (Poteete et al. 2010; Wilson et al. 2013), affecting accessibility. They may also create an illusion of closed space which blocks out the community for which the garden is intended (Milburn & Vail 2010). Community gardens may be fenced or unfenced, while still mostly remaining open in access(Müller 2007; Nettle 2014; Spilková 2017) |
Group boundaries | They generally facilitate rule enforcement (Poteete et al. 2010): roles create new obligations and rights, such as the possibility to take yield from the garden (Butler 2013; Milburn & Vail 2010). |
Proportional equivalence benefits-costs | This considers local conditions and inputs to better match contribution and rewarded (Anderies 2014; Schauppenlehner-Kloyber & Penker 2016). Perceived inequity can lead to evaluate the rules as unfair, which may increase the proportion of rule violation, as with lower garden yield available for regular members (Butler 2013) |
Collective-choice arrangements | These allow the group members to create new rules or adapt existing ones, which increases the likelihood that rules fit local circumstances, change over time to reflect local environmental and social dynamics, and are considered fair by participants. (Ostrom 2005; Poteete et al. 2010; Wilson et al. 2013). |
Monitoring | This allows keeping track of actions and possible violations of rules in the group. When violators are likely to be sanctioned, the effect of monitoring is an increased confidence among users that they can cooperate without the fear that others are taking advantage of them (Wilson et al. 2013). In community gardens, this consists of accounting for participation and rule conformity, which can be done by peers, a coordinator or through a log book (Butler 2013). |
Graduated sanctions | The sanction is proportional to the violation (Wilson et al. 2013). Sanctions bring confidence to the other users, that offenders will not continue harming the group’s interests (Ostrom 2005). Butler (2013) noted four options for community gardens: no sanction at all, the offender can be told off, the offender is not allowed on the garden anymore. The last option is a graduation of the three previous options: telling off, suspension and cancellation of entry rights. |
Conflict-resolution mechanisms | Accessible and low-cost means to solve conflicts, which are a key issue in shared urban spaces (Foster & Iaione 2015). Internal conflicts can happen for example through the self-appropriation of collective goods (Petrescu et al. 2016), or because of different agendas (Foster & Iaione 2015; Pearson & Firth 2012). Mechanisms follow different approaches: solving cases individually, referring to a committee or encouraging people to talk informally about conflicts before bringing it to the next meetings to look for mediation (Butler 2013). Such mechanisms are designed to prevent conflicts which could harm trust and overall participation (Ostrom 2005; Poteete et al. 2010) |
Recognition of rights to organise | Smaller units of decision makers have authority over certain matters (Wilson et al. 2013). This recognition comes from the local municipality allowing or not the gardening project (Butler 2013), therefore impacting the lifetime of the collective action. |
Nestled enterprises | For more complex resources, part of larger systems, the activities related to the previous design principles may be organised in multiple layers of nested enterprises (Anderies 2014; Foster & Iaione 2019). |
Theory of reasoned action
The motivational issue is handled in the action situation component of the IAD framework. However, the mechanisms by which actors become participants according to the perceived outcomes, and what actions they perform, still need to be formally described for urban community gardens. In this work, we needed a theory which enables the incorporation of a broad range of individual motivations as well as the social influence of trust. According to Darnton (2008), three theories matches this purpose : the Theory of Reasoned Action (TRA), the Theory of Planned Behaviour (TPB) and the Risk-as-feelings (RAF) model. TRA is the most fit to our empirical cases, as we noted no perceived behavioural control (TPB), and no complexification with emotions (RAF). We therefore use the Theory of Reasoned Action (TRA) (Fishbein & Ajzen 2011) to explain the decision making of individual agents in a group through their attitude (individual motivations) and subjective norm (group drivers).
In our model, we extend the TRA with inputs from Mui (2002). In the TRA, a resulting behaviour depends both on attitudes (which correspond to individual motivations) and subjective norms (Figure 2). Such norms represent a perceived social pressure to perform, or not, a given behaviour (Fishbein & Ajzen 2011). This induces action reciprocity, which is proportional to the normative belief’s strength (Mui 2002). In the belief that others perform a certain behaviour, we can recognise trust as defined by Mui: "a subjective expectation an agent has about another agent’s future behaviour based on the history of their encounters". This trust is fuelled by reputation, which is the "perception that an agent has of another agent’s intentions and norms" (Mui 2002). So, by perceiving a reputation, an individual forms an image of the norms active in a group and trusts others to comply to this norm, and therefore, feels a pressure to comply to this norm as well.
Urban gardeners expect each other to perform well enough to maintain a good state of the garden. The social pressure results from the shared desire to have a functional social-ecological system. At the same time, each gardener has individual motivations. The TRA precisely takes both the individual and collective component into consideration. Therefore, the TRA appears as a powerful explanation for the decision making of individuals in an urban community garden.
In community gardens, we can recognise various behavioural beliefs: gardeners can for instance expect to enjoy the gardening or to receive yield. Normative beliefs translate the expectation resulting from trust and reciprocity: gardeners are more willing to contribute when they know that the other participants will do the same (Chalise 2015). As mentioned above, our case-study has varied activities (such as gardening, education, meditation), for which participants probably have diverse normative and behavioural beliefs. This makes TRA relevant in our study. We describe these beliefs later in this paper and in the Appendix (Table 12).
Methodology
In order to study the behavioural mechanisms at stake in urban community gardens, we build an agent-based model which follows the IAD framework structure. We therefore need to collect data to inform some of the IAD’s components: the biophysical conditions, the community attributes, the rules-in-use and the possible action situations. We propose to describe the rules-in-use based on Ostrom’s Design principles.
In the following sections, a detailed model description can be found in a dedicated appendix structured as an ODD.
Empirical data for the model
In addition to the available literature on community gardens, we use a database of 123 urban community gardens in Germany, collected through a survey on social sustainability (Rogge et al. 2018) and two case studies in the Netherlands to qualitatively and quantitatively build our model, which we will further elaborate in this section. In Table 14 in the Appendix, we present the variables of interest and the origin of their feeding data.
We used the database of 123 community gardens to calibrate our model: categorisation of the institutional variables, motivations of people to join gardening, and most of the ranges for the parameters explored with our model (see model description). We draw some insights from the literature and this empirical data to build a model that studies the longevity of participation in urban community gardens. The model is then validated with the data from two case studies in the Netherlands by customising the parameter setup of the model to represent these cases (e.g. in terms of garden size).
We conducted two sets of interviews in two community gardens in Rotterdam (the Netherlands). Our questions are presented in the Appendix, Table 13. We have selected these two gardens because their internal rules closely match Ostrom’s Design Principles. These two gardens have been active for a sufficiently long period to be relevant to our study. The first garden is called Gandhi Tuin and was active from 2011 to 2018, date at which it stopped. The second one is called Vredestuin and was launched around 2013. Our data was collected in 2018, a few months before the end of Gandhi Tuin activity. This gives us at least 5 years of information regarding the on-site collective action. Both gardens were managed by the Vredestuin association and maintained by volunteers from the neighbourhood, which participated in gardening twice a week. In both gardens, anyone could be a volunteer. This resulted in a diverse group of gardeners, including (temporally) unemployed people, people incapable to work, and participants with varying experience in gardening and permaculture. In addition to gardening and decision-making, participants cooked and ate together when harvest was available. The community also hosted educational activities such as lectures, workshops, discussions, documentary nights, yoga and meditation classes in a classroom on the site. Participants, friends and families donated many materials to the garden and several social organisations supported the garden financially.
The institutions (such as membership, access rules, decision rules, monitoring) are framed using the terminology of the Design Principles, as commonly observed in literature and in our database of urban gardens in Germany (Table 1). After translating these principles into practical institutional variables (see ODD, Table 9), the values applied to these variables are inspired from the Rotterdam cases. We further populate our model, based on the Rotterdam examples, with values for the community attributes (size, motivations of individuals) and for the occurrence of conflicts. We describe in the Appendix our data sources (Table 14).
The beliefs, or "motivations", to join urban community gardening are very diverse, and listed in the Appendix (Table 12): many social benefits draw people in, beyond food production. Another important attribute of a community is the extent to which its members share the same core values and goals; this common understanding (McGinnis 2011) contributes to building up social capital. In the urban gardening context, common understanding is justified by the larger role played by informal rules than by formal rules and sanctions in the appropriation process (Butler 2013).
Comparison between data and model outcomes
From our model, we expect to identify certain collective outcomes (e.g. gardening duration, interpersonal trust, sense of cohesion) from the implemented institutional arrangements (design principles). The results are given in the shape of correlation tables and conditional inference decision trees.
Decision trees are popular statistical models for regression analysis. They consist of a prediction rule based on recursive partitioning. The sequence of the binary partitions (or splits) forms a tree. Conditional inference trees (Ctrees) are more broadly used for the simpler construction, with respect to the popular Classification and Regression tree (CART). Ctrees also seem to handle categorical variables better (Hothorn et al. 2006; Venkatasubramaniam et al. 2017). We therefore use Ctrees for an advanced analysis of the institutional pathways leading to higher or lower participation. They also represent a handy graphical support to our field discussions. We use the \(ctree\) function of the R party package. Because the trees tend to get very large, only splitting rules with a p-value < 0.01 are taken into account. Furthermore, we subdivide continuous institutional variables in three parts categorised as ‘low’, ‘medium’ and ‘high’ for more meaningful and interpretable analyses.
We test the validity of our model in two ways. Firstly, we compare our main model outcomes with the insights on social sustainability extracted from the German dataset. The comparison is made at paragraphs 6.21 to 6.25. Secondly, we receive feedback on our intermediate and final model outcomes, via the decision trees described above, from a Rotterdam garden expert. Frequent contact with the gardeners in Rotterdam helped us evaluating our model and its practical use. Gandhi Tuin and Vredestuin had a slightly different institutional structure, which diversifies the feedback given by our case studies on our model outcomes. The fact that Gandhi Tuin stopped functioning several months after our data collection does not affect the validation process, as we were still able to exchange with their garden leaders. In addition, this gave us the opportunity to confront our model with a real case of failed collective action (paragraphs 6.26 to 6.29).
We consider an urban community garden as a complex system with outcomes that influence the physical, social and cultural context of the community. The complexity arises mainly from their institutional diversity in supporting collective use and social interaction (Rogge & Theesfeld 2018). Here we list five outcomes:
- Yield: it is the locally-grown product of gardening activities.
- Trust: community gardening involves the commitment to certain tasks and an attention to others. Fulfilling these requirements increases the social interactions and the overall level of trust among the gardening community. This results in a positive feedback loop based on reciprocity (Chalise 2015). As noted by McGinnis (2011), trust appears among the attributes of the community through the social and cultural context.
- Social cohesion: McGinnis (2011) defines social capital as (1) resources that an individual can draw upon in terms of relying on others to provide support or assistance in times of need, and (2) a group’s aggregate supply of such potential assistance, as generated by stable networks of important interactions among members of that community. Social cohesion corresponds to this second definition: the extent to which community garden participants form relationships with each other and offer each other mutual help (de Kam & Needham 2004; Veen et al. 2016). We measure social cohesion by the number of mutual dyadic ties within the group (Friedkin 2004).
- Gardening duration: this is an important outcome in our study, because it reflects the duration of the participation in an urban community garden. (Cox et al. 2010) have used a similar measure of success, although we make it a continuous variable rather than a binary one, except to facilitate the comparison of our model outcomes with the Rotterdam cases (paragraphs 6.26 to 6.29).
- Too-much-work: gardeners leave if maintaining the garden requires more effort than they expected. Chalise (2015) determines the amount of work by the amount of activities leading to a desired quality.
An Agent-Based Model of Community Gardening
We present the agent-based model of the participation behaviour within urban gardening communities, inspired from data collected in Germany and the Netherlands.
Core concepts of the model
Our model is an abstract representation of an urban community garden, with the following concepts:
- Agents: gardeners and potential gardeners of the community garden
- Individual strategies: the agents decide to participate based on behavioural beliefs (individual level) and normative beliefs (social pressure) (see Figure 2).
- Institutions: the gardeners are bound to follow institutions, which in our case are based on Ostrom’s Design Principles.
- Outcome: yield, social cohesion, trust and gardening duration
Structure of the model following the IAD framework
We build our model based on the overall structure of the Institutional Analysis and Development (IAD) framework (Ostrom 2005). We aim to gain insight into the influence of the design principles, without considering external regulations.
The external variables in the IAD framework (Biophysical conditions, Attributes of Community and Rules-in-Use) determine the Action situations taken by the members of a system; the resulting Interactions and their Outcomes are evaluated to update the external variables and the actions taken. In our case, the Biophysical Conditions and Attributes of the community components are defined using structured interviews in our case-study and the German database (Rogge et al. 2018). The Rules-in-Use component reflects which of the Ostrom’s Design Principles are manifesting in the system and how. For each agent, taking an action is defined using the Theory of Reasoned Action (Darnton 2008; Fishbein & Ajzen 2011): a resulting behaviour depends both on attitudes (deriving from evaluative beliefs) and subjective norms (deriving from normative beliefs and motivation to comply with these). In our system the agent’s behaviour (Action Situations components) is affected by the rules (i.e., Design Principles), subjective norms and attitudes. We detail below each of the IAD’s components, from our modelling perspective.
Rules-in-use: Institutions
In our model, we derive several institutional variables from Ostrom’s Design Principles. In Table 1, we have listed and detailed the relevant Design Principles which influence participation in urban gardens. The implementations in the model are explained below following the situation in the German dataset.
- Spatial boundaries – we assume two choices for this principle: a closed fence/hedge or no fence/hedge around the garden. Therefore this principle is implemented as a boolean: garden boundaries are either active (true) or inactive (false). Having a garden boundary:
- decreases the risk of yield being stolen;
- makes rule enforcement easier (Poteete et al. 2010);
- worsens the evaluation of the belief of land availability, when deciding to join gardening (Milburn & Vail 2010).
- Monitoring – this implies a higher probability of sanctioning (Wilson et al. 2013). We vary monitoring intensity across a range of values to clarify its impact (see ODD, Table 9).
- Group boundaries – Possibilities range from an open-to-all flat organisation, to a hierarchy of roles such as key-holders, members, employees, trustees, and/or committee members. When this principle is inactive, everyone is allowed to join and take yield. In some cases, a membership fee is asked to become a member. The fee may be an obstacle and therefore reduces the intention to participate. We test the effect of the fee by varying its value in the formula below:
- Collective-choice arrangements – The garden management rights are usually held by a core group, which sets up mostly roughly-defined rules. If active, this principle means that all gardeners have the opportunity to alter the existing rules. We assume in this case that the gardeners are less inclined to violate the rules which they co-designed. The global probability of violating a rule, implemented as a range, is decreased accordingly before the first simulation step (see ODD, Table 9).
- Proportional equivalence benefits/costs – There are neither formal nor structured appropriation rules: the yield share is taken by the people present in the garden with no specified limit. We therefore implement this principle as follows: contributors receive an ideally fair amount of yield. They choose their own amount; they violate a rule and can be told off or sanctioned if this amount is higher than a set value.
- Graduated sanctions – When this principle is active, the agent violating a rule is told off a first set amount of times. In case of recurrent violation, the agent is suspended for a set amount of time, before risking indefinite access denial.
- Conflict-resolution mechanisms – This principle is implemented as a range determining the harm caused by a conflict (see ODD, Table 9).
- Recognition of rights to organise – From some informal discussions in the field in Germany and the Netherlands, it seems that the absence of recognition may trigger a feeling of togetherness within the community. Because this principle relies on external actors and has not reached consensus regarding its effects, we leave it out of our research.
- Nestled enterprises – The urban gardening cases at hand being relatively simple systems, this principle is not relevant and left out of the model.
\[MemberIntention = Intention - fee\] |
with: | |
\(Intention\) | the behavioural intention to go gardening, based on the evaluations and importance of each behavioural belief and normative belief |
\(fee\) | a range between 0 and 0.9 ; 0 meaning ‘no fee for yield’ and 0.9 meaning ‘a fee for yield which is only worth paying for people that regard yield very important’ |
We define a probability of yield being stolen as a garden characteristic. The way in which rule enforcement gets easier with garden boundaries is unclear, just as the probability of a rule violation being sanctioned is also unclear. Therefore, we vary the probability of sanctioning in our model across a range and test this separately from the garden boundaries boolean.
There is no straightforward transcription of each principle into the model. Therefore, we have, in certain cases, grouped the effects of several design principles into one institutional variable of our model. We clarify our conceptualisation and labelling of these principles in the ODD at Table 9.
Material conditions
We consider here the yield and the uncomfortable conditions (defined above). Regarding the yield taking action situation, we assume the following. The yield is divided in equal shares; one share for each gardener. The minimum amount a gardener can take is 0, the maximum amount a gardener might take is defined by \(DPMaxTakingMoreThanShare\), which we set for an experiment. The fair amount is 1. The gardeners randomly choose to wish for an amount of yield higher than 0 and lower than the maximum amount. When gardeners take their randomly chosen share, the amount of yield decreases. When the amount of yield decreases too much and gardeners cannot take their chosen share anymore, they evaluate the yield taking of that session negatively. This results in a decrease of \(e_{yield}\).
Community
A new community first consists of initiators, who visit the garden regardless of their beliefs for a set amount of gardening sessions. In our model, we assume that the number of potential gardeners is a function of the number of gardeners. This is formalised following Chalise (2015): each gardener speaks to a set amount of individuals about the garden, of which a set percentage decides to give gardening a try. After this first try, the individual becomes a potential gardener. The repeated engagement of potential gardeners depends on their beliefs strengths and beliefs evaluation. The belief strengths for the attitudes (cohesion, social time, education, sustainability \(\dots\)) are an average of the values found in the German database (Rogge et al. 2018) and those found in the Dutch cases Gandhi Tuin and Vredestuin. The amount of potential gardeners and gardeners decreases yearly by a fixed percentage ; this accounts for volunteers leaving due to reasons other than events happening in the community garden. The network in which agents interact is a random network.
Conflicts occur with a set return period in our simulations, based on the observations in Gandhi Tuin.
The flowchart in Figure 3 provides an overview of the model.
Action situations
In this study of urban community gardens, we define three action situations:
- Contributing to the garden: At this stage, all agents have the position of potential gardener. If the agent decides to contribute, it is given the position gardener. The decision depends both on the agent’s behavioural intention (Figure 2) and on the Design Principles for garden boundaries and graduated sanctions. For some of the potential outcomes, the gardener has full control over his or her decision (e.g., enjoying nature or enhancing spiritual practice). Some outcomes are uncertain, such as access to fresh food. Some are certain but not under control of the gardeners (e.g., bad gardening conditions).
We describe the decision making of agents with the Theory of Reasoned Action: agents have evaluative beliefs and strengths for that belief (Darnton 2008). A belief is defined as the subjective probability that an object has a certain attribute, which is determined by the information accessible in memory (Fishbein & Ajzen 2011). The strength value for each belief is based on our database. When the agent visits the community garden, it combines the strength it gives to each belief and the evaluation of this belief according to its past experiences, if existing. For the first visit, the beliefs’ evaluations are set to a neutral value. Parameter set-up is detailed in the next section. Beliefs are then re-evaluated at each simulation step. - Choosing an amount of yield to take: In the action situation of yield taking, only gardeners participate. Who can take the position to take yield, depends on the Design Principles for garden boundaries and appropriation between benefits and costs. These principles can be translated by the following questions, respectively: has the volunteer paid the access fee allowing it to participate and take yield? Has the volunteer worked enough to deserve taking yield? If these principles are not active, volunteers are free to choose their desired amount of yield. The potential outcomes of taking yield can be having a fair amount, having more than a fair amount or having less than a fair amount. The notion of fairness here depends on the rules in the garden. To simplify, we consider as fair an amount of yield taken that is below a fixed limit (parameter in the model). Any amount taken above would be flagged as a rule violation.
- Violating rules while present on the garden: Gardeners can either contribute fairly or violate a rule. A rule can be violated by mistake, or deliberately (Ostrom 2005). However, such violations are not considered equally across our garden cases, since rules may differ from one garden to another. We therefore, implement the decision to violate a rule as a probability to violate a rule (see ODD in the Appendix).
The three action situations above are regulated by Ostrom’s Design Principles. This is described in detail in the ODD.
From literature and our Rotterdam cases, we find that gardeners have multiple beliefs for joining gardening (Chalise 2015; Drake & Lawson 2015; Guitart et al. 2012). However, some of the beliefs overlap regarding the related practical needs. For example, the concrete action of taking yield may originate from the belief to save money or the belief to consume fresh self-grown food. This is why we merge these beliefs following their related practical need, as shown in the ODD (Table 9). In the Appendix, we also specify our data sources.
In addition to the attitudes, gardeners’ decisions are also affected by subjective norms. In our case, the only effective norm is the pressure felt by volunteers to maintain the garden: it is labelled as needcontribution. Whether a person reciprocates the norm of contributing to the garden, depends on the individual’s norm of reciprocity (Mui, 2002). We therefore, assume reciprocity determines the strength for this normative belief.
The steps characterising decision-making, through the weighing of the different beliefs, is explained in the Behavioural Intention formula visible in our ODD (see the Appendix).
Evaluation criteria
Interactions between agents occur during gardening. As mentioned previously, agents who join gardening are affected by their participation experience, e.g. successful yield taking, binding with other gardeners or experiencing conflicts. The outcomes resulting from these interactions influence the beliefs of the agents. We have detailed this in the ODD section of the Appendix.
Simulation Setup and Sensitivity Analysis
Experiment setup
Our goal is to build experiments which represent combinations of institutional variables. Each variable is thus explored within a predefined range (ODD, Table 9). We do the same with certain parameters (see Table 2). Because we can’t anticipate their effects on our model results, it is important to represent as efficiently as possible these ranges. We use R’s Latin Hypercube Sampling (LHS) package to generate pseudo-random vectors of 100 values for each range (van Dam et al. 2012).
Variable | Dependence | Value origin |
---|---|---|
CollectiveActionFailedTime | dependent | first moment there is 1 or no volunteer on the garden |
Trust | dependent | sum of gardeners’ trust after every tick / total visits. Trust is defined as good encounters / total encounters. |
Cohesion | dependent | sum of gardeners’ cohesion belief after every tick / total visits. Cohesion is defined by the rate of gardeners in the group with whom a gardener has a tie. |
Yield | dependent | sum of gardeners’ yield belief after every tick / total visits. Yield is evaluated positively if the wished amount of yield is received. |
Too much work | dependent | sum of gardeners’ belief for too much work after every tick / total visits. It is evaluated positively if the amount of volunteers is higher than a given threshold. |
DPfee | independent | LHS: \(\in [0 , 0.9]\) |
DPMaxTakingMoreThanShare | independent | LHS: \(\in [1 , 5]\) |
DPglobalprobabilityruleviolation | independent | LHS: \(\in [0.1,0.9]\) |
DPconflictharm | independent | LHS: \(\in [0,100]\) |
DPprobabilitysanctioning | independent | LHS: \(\in [0,0.9]\) |
DPgraduatedsanctions | independent | LHS: \(true / false\) |
DPplotboundaries | independent | LHS: \(true / false\) |
NoAccessSessions | independent | LHS: \(\in [5,20]\) |
MaxAmountTellingOffAfterSuspension | independent | LHS: \(\in [2,8]\) |
Membershipduration | independent | LHS: \(\in [26,104]\) |
MinAmountOfTellingOff | independent | LHS: \(\in [2,10]\) |
MaxAmountOfTellingOff | independent | LHS: \(\in [10,40]\) |
BalanceAttitudeSocialNorm | independent | LHS: \(\in [0.5,4]\) |
ContributingThreshold | independent | LHS: \(\in [3.5,4]\) |
Our model runs for the same period as the Gandhi Tuin project, thus 6 years. Gandhi Tuin had 2 gardening sessions a week. Excluding a 2-week Christmas break, this results in 100 gardening sessions a year and thus 600 in six years. Therefore, unless they collapse earlier, our experiments run for 600 ticks. Our German dataset indicates that conflicts happen "very rarely". Our case study data is also in line with this, as large conflicts appear on average every 2 years, i.e. 200 ticks. Lacking more information on this, we this consider this value as conflict interval in our model.
The success of the experiments is measured by the output variable \(CollectiveActionFailedTime\): the timestamp at which less than two gardeners are present in the garden, causing the simulation to stop. All simulations which could have run longer than 600 ticks (i.e. the number of gardeners at tick 600 is higher than 2) receive the value 600 for this output variable.
In the Netlogo software, experiments are run using the built-in tool \(behaviorspace\) (Wilensky & Shargel 2002). We run 100 repetitions of each experiment.
Sensitivity analysis
We use the one-factor-at-a-time (OFAT) methodology to gain insight on the sensitivity of the model to uncertain parameters and define the parameter space in which the experiments will be conducted (ten Broeke et al. 2016). OFAT is executed by setting a baseline of parameter values for all parameters: these are presented in the column "base value" of Table 3. We vary each uncertain parameter individually across a range of values, bounded by a maximum value; this range may be a single value (e.g., Initiators) or series/ranges of values (e.g.,ContributingThreshold). For each parameter, a series of values consisting of the base value, the range value(s) and the maximum value were tested for 100 repetitions, both with active institutional variables or without (Table 3).
The institutional settings corresponding to active or inactive design principles are presented in Table 4.
Parameter name | Base value | Range | Maximum | CAfailed\(^+\) sensitive (No DPs) | CAfailed sensitive (DPs) |
---|---|---|---|---|---|
ContributingThreshold | 2 | 2.5, 3.5, 4, 4.5, 5, 5.5 | 6 | -0.45*** | -0.32*** |
NoAccessSessions | 10 | 5 | 20 | 0.00 | 0.00 |
MaxAmountTellingOffAfterSuspension | 5 | 2, 8 | 10 | 0.00 | 0.12 |
Membershipduration | 52 | 26 | 104 | 0.00 | 0.00 |
MinAmountOfTellingOff | 5 | 2 | 10 | 0.00 | 0.00 |
MaxAmountOfTellingOff | 20 | 10 | 40 | 0.00 | 0.00 |
BalanceAttitudeSocialNorm | 1 | 2, 3 | 4 | 0.15*** | 0.28*** |
ChanceYieldAvailability | 1 | 0.8 | 0.8 | -0.03 | 0.03** |
ChanceYieldStolenWhenBoundaries | 0 | 0.20 | 0.5 | -0.01 | -0.02** |
VolunteersToFullySee | 3 | 1 | 6 | 0.09** | 0.06 |
AmountOfTasks | 10 | 5 | 20 | -0.05** | -0.03 |
Initiators | 10 | 5 | 20 | 0.14*** | 0.12* |
InitiatorCommittedTime | 52 | 26 | 104 | 0.20*** | 0.33*** |
Sensitivity analysis outcomes
In order to define ranges for the input parameters, we explore simulation scenarios with and without the institutional variables (Table 4). Parameters are evaluated against the output variable \(CollectiveActionFailedTime\) using Spearman correlations and Kruskal-Wallis p-values. This variable indicates the simulation tick when less than two gardeners remain, which is also a measure of the gardening duration. Table 3 shows the sensitivity of \(CollectiveActionFailedTime\) to the model parameters. The garden characteristics (in italic in Table 3) are set to match the case-study during the simulations and are unchanged during the simulations. The analysis shows that only the values for the \(ContributingThreshold\) and \(BalanceAttitudeSocialNorm\) show significant impact on the gardening duration (Table 3). These two parameters result from our theoretical choices, and therefore we have no data for them. We assign them a range of values from which we look for the highest variation of \(CollectiveActionFailedTime\).
Name | Not active | Active |
---|---|---|
DPplotboundaries | Off | On |
DPfee | 0 | 5 |
DPMaxTakingMoreThanShare | 2 | 1 |
DPglobalprobabilityruleviolation | 0.61 | 0.31 |
DPconflictharm | 70 | 30 |
DPprobabilitysanctioning | 0.31 | 0.61 |
DPgraduatedsanctions | Off | On |
\(ContributingThreshold\) is key to determine whether or not a potential gardener becomes gardener. When testing its effect on \(CollectiveActionFailedTime\), we observe a sharp drop of the gardening duration around the value 4 (Figure 4). Our simulations will, therefore, consider values of \(ContributingThreshold\) close to 4 (Table 3). \(BalanceAttitudeSocialNorm\) indicates the values of the weight \(W_2\) of the social norm, with respect to the weight \(W_1\) of the attitudes, as defined in the ODD (see the Appendix). The related scatterplot (Figure 5) shows that the output variable’s values are not significantly impacted by a specific subinterval of \(BalanceAttitudeSocialNorm\) values. The other non-garden related parameters show similar plots. Therefore, we have to take into account the whole range of values in our experiments for these parameters, as indicated in Table 3. The independent variables in Table 2 show the ranges of parameters as they are in our exploratory experiments.
Results
In this section, we first present our model experiments results, obtained through Netlogo’s Behaviorspace tool. Subsequently, we compare our modelling outcomes with the German urban gardens datasets. Finally, we perform a historic replay by using the values of Gandhi Tuin, and comparing the model results with our field observations.
Collective action outcomes
We measure the gardening duration, the overall trust among gardeners, their beliefs for social cohesion, for yield taking and for too-much-work. The simulation outputs are analysed with correlation tables and decision trees from R package party.
The output variable \(CollectiveActionFailedTime\) is our indicator of success, and represents the experiment lifetime.
Figure 6 shows four peaks in the variable’s distribution. The first peak emerges because the initiators can leave the garden, resulting in a drop in gardeners which can cause the collective action to collapse. The next two peaks at 200 and 400 ticks appear because of our implemented periodical conflict appearance. The final peak at 600 ticks is the amount of cases that did not collapse in the first 6 years.
Figure 7 shows the values distribution of the other four outcome variables, which we discuss below.
Collective action outcomes and its dependencies
Among the dependent variables, we observe that the beliefs for trust and cohesion are both strongly and positively correlated with high values of \(CollectiveActionFailedTime\): trust and cohesion lead to longer collective action. The belief for yield is also positively correlated to \(CollectiveActionFailedTime\), but to a lesser extent. This means that gardeners participate longer when they do so for cohesion and trust rather than for physical yield (Table 5).
Dependent variable | Trust | Belief for cohesion | Belief for yield | Belief for too-much-work |
---|---|---|---|---|
CollectiveActionFailedTime | 0.51*** | 0.53*** | 0.4*** | -0.18*** |
Trust | 0.21*** | 0.2*** | -0.06*** | |
Belief for cohesion | 0.1*** | 0.19*** | ||
Belief for yield | -0.23*** | |||
Collective action and the effect of independent institutional and social variables
Table 6 shows the correlations between the five outcomes variables (experiment lifetime, trust, cohesion, yield, Too-much-work) and the independent institutional variables and the social system parameters in our experiments. In the subsequent subsections, we analyse each of the outcomes variables.
independent variable | \(\rho_{CAfailed^+}\) | \(\rho_{trust}\) | \(\rho_{cohesion}\) | \(\rho_{yield}\) | \(\rho_{Too-much-work}\) |
---|---|---|---|---|---|
DPfee | -0.09 *** | 0.06 *** | -0.09 *** | 0.21 *** | -0.03 *** |
DPMaxTakingMoreThanShare | -0.02 *** | 0.08 *** | -0.02 *** | -0.75 *** | 0.08 *** |
DPglobalprobabilityruleviolation | -0.25 *** | -0.38 *** | -0.04 *** | -0.14 *** | 0.11 *** |
DPconflictharm | 0.00 *** | -0.05 *** | -0.02 *** | -0.04 *** | -0.02 *** |
DPprobabilitysanctioning | 0.58 *** | 0.74 *** | 0.25 *** | 0.25 *** | -0.10 *** |
DPgraduatedsanctions | -0.02 *** | 0.00 ** | -0.08 *** | -0.01 *** | 0.08 *** |
DPplotboundaries | 0.01 *** | -0.01 *** | 0.00 | 0.22 *** | -0.01 *** |
NoAccessSessions | 0.02 *** | -0.02 *** | 0.01 *** | -0.01 *** | -0.03 *** |
MaxAmountTellingOffAfterSuspension | 0.00 *** | 0.00 *** | 0.01 *** | -0.01 *** | 0.01 *** |
Membershipduration | -0.02 *** | 0.01 *** | 0.00 *** | 0.00 *** | 0.06 *** |
MinAmountOfTellingOff | 0.05 *** | 0.02 *** | 0.10 *** | 0.01 *** | -0.03 *** |
MaxAmountOfTellingOff | 0.06 *** | 0.00 *** | 0.12 *** | -0.01 *** | -0.05 *** |
BalanceAttitudeSocialNorm | 0.38 *** | -0.14 *** | 0.09 *** | 0.22 *** | -0.27 *** |
ContributingThreshold | -0.12 *** | 0.05 *** | -0.01 *** | -0.06 *** | 0.05 *** |
Experiment lifetime
Regarding the influences of the institutional variables, the lifetime of the experiments is strongly positively correlated with \(DPprobabilitysanctioning\) (\(\rho = 0.58\)), followed by \(BalanceAttitudeSocialNorm\) (Table 6). It is negatively correlated with \(DPglobalprobabilityruleviolation\). This means that participation survives better in the presence of monitoring (probability that a person would be sanctioned), but also when gardeners consider normative beliefs (or social pressure) as important. On the opposite, rules that minimize probability of rule violation are associated with weaker collective action.
Trust
As mentioned earlier, indirect reciprocity is the belief strength for trust, which therefore depends on the occurrence of collaborative encounters within the total encounters. This is also called group reputation.
Trust values vary between 0 and 1, and are most often in the top range (Figure 7). The correlations indicate a high sensitivity of trust to strong sanctioning (\(\rho = 0.74\)). Accordingly, violating rules negatively affects trust (\(\rho = -0.38\)). However, looking at the decision tree for trust reveals that values of trust just as high, can emerge when \(DPprobabilitysanctioning\) is medium or low. This implies that monitoring rules that increase the probability of a person being sanctioned do not necessarily increase the level of trust among gardeners.
Our implementation of \(DPconflictharm\) decreases trust among gardeners whenever a conflict arises (every 200 ticks), with the formula below.
\[TotalConflicts = TotalConflicts + DPconflictharm\] |
\[Trust = \frac{AmountOfGoodEncounters}{TotalEncounters + TotalConflicts}\] |
In the trust formula, the higher the amount of good encounters (encounters with no rule violation), the lesser the impact of the number of conflicts. We assume that a gardener who has witnessed many good encounters would be only marginally affected by the emergence of a conflict. We illustrate the results of this assumption in Figure 8, and make it more visible by setting conflict points exceptionally every 100 ticks, instead of 200. The effect of the conflict points (black arrows in the graphics) on the average trust is dampened over the simulation time, while the amount of good encounters increases regularly.
Cohesion
Cohesion is the extent to which community garden participants form relationships with each other. We measure it by the amount of dyadic ties within the group.
Cohesion values do not cover the full range, as they depend on the ties each gardener can make, which depends on the number of gardeners present and on a set probability \(Relationrate\) for an individual to form a relation with another gardener (Chalise 2015). Among all institutional variables, \(DPprobabilitysanctioning\) has the highest correlation with cohesion, however it is weak (\(\rho = 0.25\)). Active graduated sanctions tend to lower cohesion. This result is coherent with the empirical data, which indicates that graduated sanctions could lead to the suspension or the exclusion of group members. When such a measure is taken, social bonds break.
Yield
Yield has a strong negative correlation with \(DPMaxTakingMoreThanShare\), which is coherent with the assumption that taking more than one’s share reduces the other’s expectations for yield. \(DPfee\) and \(DPplotboundaries\) seem to additionally secure the belief for yield. \(BalanceAttitudeSocialNorm\) also has a positive effect (\(\rho = 0.22\)).
Too-much-work
The belief for Too-much-work is in most experiments low. Consequently, in our model, people are unlikely to quit gardening because of too much work. \(BalanceAttitudeSocialNorm\) is linked with lower beliefs for Too-much-work (\(\rho = -0.27\)): the higher the weight for social norm, the lower the belief for Too-much-work.
Decision tree analysis of the collective action
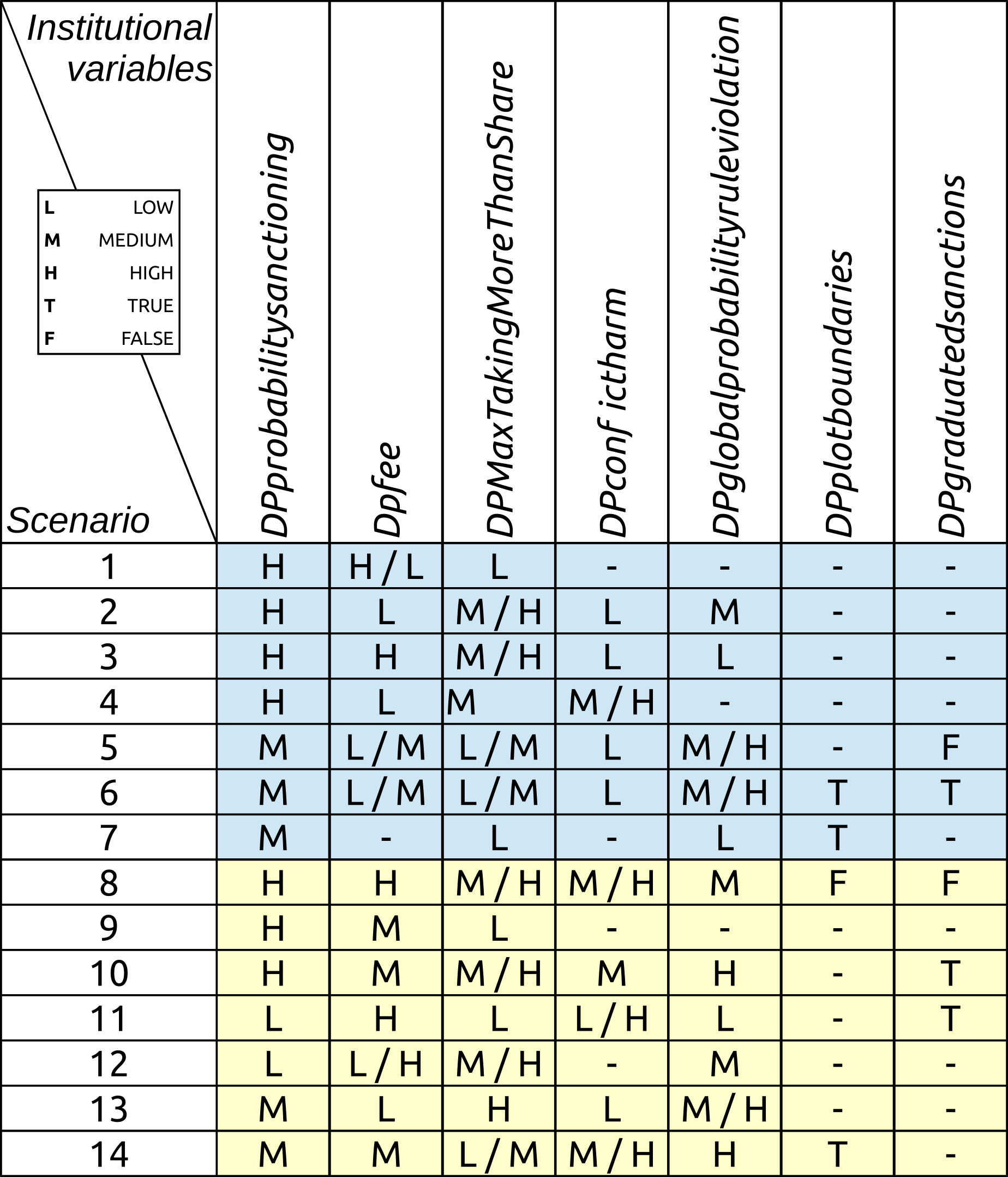
We use decision trees to further analyse our findings of Table 6 with respect to the experiment lifetime variable. This is another way to analyse the experiments as defined earlier in the the simulation setup section. We provide an example in Figure 9. We have categorised the independent variables (institutions and social parameters) into low, medium and high, for a simpler interpretation. In addition, we have also divided \(CollectiveActionFailedTime\) in two categories: the successful cases, sustained for 6 years or more, and the collapsed (failure) cases, which stopped before that time. The Ctree algorithm splits our output dataset in a way to form pathways, which we call "scenarios". These scenarios emerge from the analysis, and are not set by us in the model. Each scenario is linked to a certain ratio of collapsed/successful cases.
Figure 9 is a subset of the decision tree generated for the variable \(CollectiveActionFailedTime\), with 10 nodes visible out of the 283 total nodes. We can recognise three of the successful scenarios from Table 7. The top of the tree is not visible: nodes 1 and 2 which are missing, and should be on top of the tree, correspond to high \(DPprobabilitysanctioning\) and high/low \(DPfee\). Overall, the decision tree shows a clear path dependence for the chance of success. For scenario 3, less conflict-resolution mechanisms at node 16 (increase of \(DPglobalprobabilityruleviolation\) from low to medium) would severely reduce the chance of success.
We have identified in Table 7 14 scenarios, leading either to successful or to failed collective action. According to our decision tree analysis, it is not the institutional variables themselves, but rather their combination that influences the success or failure of the gardening experiment. In other words, certain combinations of Design Principles lead to the success of all experiments, while other combinations lead to early collapse. Most of the combinations generate mixed results in which only a certain percentage of all experiments were successful. Active sanctioning combined with taking low amounts of yield leads to certain success (scenario 1). As a corollary, we observe a free-riding case (scenario 12): a low level of sanctioning, combined with the possibility of taking higher amounts of yield and a medium risk of rule violation, leads to certain collapse, no matter the entrance fee. Sanctioning is however not always key to success. Less active sanctioning leads to similar success only if further principles are applied (scenario 7): collective-choice arrangements (which reduce the probability of rule violation) and garden boundaries. Active sanctioning combined with other principles can also lead to early collapse of the collective action (e.g. scenarios 8, 9 and 10). We will elaborate on that in the Discussion section.
The decision tree has allowed us to pinpoint a path dependency based on combinations of institutional variables. This type of insight did not appear in the correlations (Table 6). Another example is the effect of conflict-resolution mechanisms (\(DPconflictharm\)): their insufficient implementation was the cause of the collective action failure in the Gandhi Tuin case. This result does not appear in the correlation table, but is more visible in the decision trees. In most scenarios (Table 7), well implemented conflict-resolution mechanisms (low \(DPconflictharm\)) are linked with successful experiments.
Comparing model insights to the German cases
The experiment lifetime was not measured in the German dataset, therefore we compare the other four outcomes variables.
Trust
The empirical data from the German cases (Rogge et al. 2018) does not support the strong positive effect of sanctioning on trust. We found \(\rho_{model} = 0.74\)(***) while the German dataset displays \(\rho_{data} = -0.19\)(*). Another contradiction occurs with \(DPglobalprobabilityruleviolation\), which is in the dataset measured by the frequency of complaints regarding either resource use or community interaction. We find \(\rho_{model} = -0.38\)(***) while the dataset shows no correlation. The weak effects of the other institutional variables on trust are similar in the German dataset.
Cohesion
Weak correlations were found between social cohesion and the institutional variables, which is confirmed by the data from the German gardens. However, the German examples did not show such a high correlation \(\rho_{sanctioning} = 0.25\)(***) between the chance of sanctioning (\(DPprobabilitysanctioning\)) and social cohesion. Instead, the initial probability of violating a rule (\(DPglobalprobabilityruleviolation\)) played in those cases a stronger role.
Yield
Our results from Table 6 are similar to insights from the German dataset, except for the strong negative correlation for \(DPMaxTakingMoreThanShare\), since, in the field, gardeners hardly set expectations for yield, as it is not a main belief for them. The weak effects of the other institutional variables on yield are, however, comparable in the dataset.
Too-much-work
From the German gardens, Rogge et al. (2018) found a weak negative effect of community size on social sustainability, an outcome which is simplified in our model as long-lasting participation. This is however, not comparable to our model results (see discussion). Yet, Ostrom (2002) states that community size has no effect on successful management of shared resources. If we assume that gardeners strongly value the collective purpose, then our result is in line with the work of Ostrom (2002).
Comparing model insights to the Dutch cases
We perform a historic replay of the Rotterdam case-studies. This time, we have a unique scenario which is set by the modeller, in such a way to replicate the institutional arrangement of Gandhi Tuin. After running the model with this scenario, we plot five different decision trees, one for each outcome variable. Since the characteristics of Vredestuin are very similar, we use the same scenario for the second case study comparison as well. Decision trees were used to show our model results to the gardeners of both Gandhi Tuin and Vredestuin, in order to receive feedback.
Institutional variable | Gandhi Tuin | Vredestuin |
---|---|---|
\(DPprobablilitysanctioning\) | L | H |
\(DPfee\) | L | L |
\(DPMaxTakingMoreThanShare\) | H | M |
\(DPconflictharm\) | H | L |
\(DPglobalprobabilityruleviolation\) | L | L |
\(DPplotboundaries\) | T | T |
\(DPgraduatedsanctions\) | T | T |
Collapsed cases | 59 % | 2 % |
Trust | 0.78 | 0.92 |
Cohesion | 0.72 | 0.58 |
Yield | 0.52 | 0.93 |
TooMuchWork | 0.08 | 0.00 |
The institutional variables based on the cases of Gandhi tuin and Vredestuin can be found in Table 7. Gandhi Tuin collapsed after 5-6 years. Its garden leaders explained that conflict-resolution mechanisms were insufficiently implemented: a conflict appeared and could not be dealt with efficiently, leading the volunteers to quit. Vredestuin has learned from Gandhi Tuin that people’s behaviour can harm trust when monitoring and sanctioning are poorly applied. \(DPprobabilitysanctioning\) becomes high and \(DPconflictharm\) is low for Vredestuin. In the decision trees obtained for each dependent variable, we looked for the paths which correspond to the two case studies. We have noted the predicted values of our dependent variables in the lower part of Table 7.
The garden expert from Vredestuin felt very confident about the people gardening under this new set of principles. This confirms the rise in trust measured by our model (0.92 against 0.78). In addition, our model predicts a significantly lower proportion of collapsed experiments for the Vredestuin scenario (2 % against 59 % for Gandhi Tuin). This is in line with the fact Gandhi Tuin actually collapsed within 6 years.
Our assumption on the belief for Too-much-work leads to a positive feedback loop. Too-much-work is a barrier to participation (Chalise 2015), which has the effect of reducing the number of gardeners (community size), thereby reinforcing the belief of Too-much-work. Our model indicates very weak effects of most institutional variables on this belief. It is only when gardeners join for a collective purpose (high \(BalanceAttitudeSocialNorm\)), that they are less affected by the amount of work to be done (\(\rho = -0.27\), Table 6). In the Rotterdam cases, only 20 % of the gardeners mentioned being affected by too much work. Our model gives less than 10 % (Table 7), which is a bit underestimated. This supports the idea that community size may not affect the overall gardening duration, since only a minority of gardeners are affected by low attendance and therefore higher workload (see also Figure 7). Overall, our model was able to explain the observations and impressions given to us by the Rotterdam garden leaders.
Discussion
In this paper we explored the role of Ostrom’s design principles for collective action on urban community gardens, a classical example of urban commons. We used the IAD framework and the theory of reasoned action to frame our agent-based model, and then translated Ostrom’s Design Principles into institutional variables. We discuss below the outcomes of our research.
Institutions for successful urban gardening
Ostrom’s Design Principles are perceived as indicators of robust collective action. Our research leads to two main findings: (1) the longevity of urban gardening participation depends on the combination of the Design Principles ; (2) the assumptions behind sanctioning and taking yield should be further investigated.
Although we measured success of urban gardening initiative in a similar manner as Cox et al. (2010), we did not find that all design principles contribute equally to the success of the gardening experiments. Yet, it is worth mentioning here that, as we translated these principles into practical institutional variables, certain principles could not be tested individually (e.g. monitoring). We have identified certain combinations of institutional variables, and by extension, of design principles, which can either lead to the success of the gardening experiments or their failure. This finding is also supported by a study on 60 cases of Common-Pool Resources (CPR) which points out that context-specific combinations of design principles lead to greater success (Baggio et al. 2016). They consider mainly fishery, irrigation and forestry activities. Their measurement of success embraces resource sustainability, an element which we did not consider, and trust, which we measured. For example, they noted the prevalence of both active boundaries and equivalence benefits/costs together in successful CPR cases. Our agent-based model confirms the idea of combinations of Design Principles, without necessarily pointing to the same combinations as those identified in (Baggio et al. 2016). This can be linked to a divergence of assumptions (e.g. indicator of success), modelling translations (e.g. redefined design principles in the ODD at Table 9), and types of resources analysed, which leads us to our second point below.
Urban community gardening is more than a CPR, in the sense that gardeners join not only to create physical value (yield) but also for intangible resources such as cohesion, leisure and education. The absence of physical yield does not necessarily reduce the quality of the resource (urban garden) nor the level of participation. This may be why our results diverge from those of Cox et al. (2010), who dealt specifically with natural resources (e.g. forest, fishery, irrigation, pasture). Our case shows that trust and cohesion are both the main drivers of durable participation (Table 5). This is in line with Baggio et al. (2016), who explain the prevalence of the equivalence benefits/costs Design Principle by the idea of perceived equity, which can be related to trust. At the same time, trust can be seen as one of the main outcomes of urban gardening, rather than yield: it emerges through the collective action processes undergone by the gardeners. This may be why, unlike in the work of Baggio et al. (2016), the equivalence benefits/costs is weakly correlated to trust, our common indicator of success. In other words, because yield extraction plays a lower role in gardening communities, than it does for fishery, irrigation and forestry, a more just equivalence benefits/costs (i.e. proportionality investment/extraction) does not necessarily ensures more success.
Trust appears both as a condition for and a result of successful collective action. Through long-term repeated interactions among the same members of a community, trust as outcome may outweigh trust as a condition and induce adaptability. This may explain why, in Figure 8, gardeners are less affected by conflicts over time, as the number of positive encounters increases.
We also wish to highlight the current emphasis on the role of sanctioning for collective action. The ongoing assumption, in the Ostrom and Common-Pool-Resource literature, is that sanctioning reduces the risk of rule violation through increased compliance. We wish to moderate this claim in the case of urban community gardens, an example of urban commons, with the following two arguments. Rogge et al. (2018) highlighted the higher explanatory effect of trust, rather than sanctioning, in collective (social) actions situations, such as community gardening. In most of the cases from the German community gardens dataset, detailed rules were not of high importance. Conjointly, Rogge et al. (2018) found that monitoring and sanctioning had the lowest impact on social sustainability, among the following factors: community size and heterogeneity, size of the area, perceived trust, size of the management group and rule design. We suspect that monitoring and sanctioning in case of vaguely-defined rules becomes less relevant, which can explain the low impact of this factor on social sustainability. We can rephrase this argument by saying that sanctioning mechanisms are rather an indicator for low social sustainability and compliance problems. Furthermore, regardless of how strictly defined rules are, sanctioning has unclear effects on gardening participation (Table 7). Baggio et al. (2016) have also found an indeterminate link between sanctioning and success, unless when sanctioning goes together with proportional equivalence benefits/costs.
While our results show that rule violation indeed weakens trust, sanctioning does not always imply long-term collective action. Although we took the ongoing assumption of sanctioning, in the sense of Ostrom, into account, we have observed multiple scenarios where active sanctioning leads to early collapse of the collective action (scenarios 8, 9 and 10 in Table 7). Regarding trust, although we measured a high sensitivity of trust to both active sanctioning (\(\rho = 0.74\)) and rule violation (\(\rho = -0.38\)), we have also observed that high values of trust can emerge when sanctioning is weakly implemented. In addition, although cohesion is the most correlated factor with sanctioning (\(\rho = 0.25\)), it is still a relatively weak one. At the same time, active sanctioning impacts cohesion negatively, which we explain by the possibility of loosing social ties when sanctions such as suspension or exclusion are implemented.
To support our claim regarding the ambiguous role of sanctioning in collective action, we also point to recent research on archival records of European commons across the past centuries, which has also highlighted a negative correlation between rules corresponding to sanctioning and the longevity of the commons (Boyd et al. 2018; de Moor et al. 2016). Their research shows that sanctioning may have been used occasionally on long-lasting commons, but most of the time and effort was dedicated to organizing regular meetings. This involvement, implying rules adjustments, information exchange, mutual monitoring and the internalisation of norms, is positively correlated with long-lasting commons, unlike plain sanctioning. Therefore, commons with more effort put on participatory, rather than punishing institutions, tend to last longer. Conscious that our work describes cases of new commons (Hess 2008) with important social motivations, our conclusions are in line with these historical commons. In addition, we found similar conclusions regarding the effective combinations of design principles as Baggio et al. (2016), who studied traditional Common-Pool Resources. We conclude that the existing assertions on the efficacy of sanctioning in cases of voluntary collective action should be moderated and investigated further.
Further developments
In our model we have used the current assumption of sanctioning, which says that sanctions prevent free-riding and therefore extend the lifetime of the urban commons, such as community gardens (Foster 2011; Ostrom 1990). However, our results, the study on social sustainability and recent research indicate that the urban commons do not necessarily revolve around free-riding problems (Borch & Kornberger 2015; Boyd et al. 2018; de Moor et al. 2016). In Europe, as well as in East-African pastoral groups, coercive institutions are less likely to solve collective action problems than "informal, culturally evolved moral norms" are (Boyd et al. 2018 p. 1236). European community gardens proposing various activities, social or not, they are less likely affected by free-riders. The value of the urban commons tends to increase with higher participation, rather than observing resource depletion (Borch & Kornberger 2015). The study of such value emerging from the diversity of members and activities should be looked into, notably in different cultural contexts.
Secondly, we strongly suggest to update the ongoing sanctioning assumption, at least in the circumstances where sanctioning has unclear effects. We imagine two ways:
- Adding the indirect burden created by the existence of plain sanctioning rules: empirical data (Rogge et al. 2018), our model results and recent findings (Boyd et al. 2018; de Moor et al. 2016) are critical towards the effectiveness of sanctioning measures. A hypothesis is that sanctioning may be perceived negatively by potential gardeners, who solely wish to join for leisure, and therefore are discouraged by the presence of punitive mechanisms.
- Including the possibility for people to become gardener solely with benevolent intentions, as commonly observed by Rogge et al. (2018) in the German cases. We have presently assumed that all gardeners make mistakes or violate rules with a given probability which evolves over the simulations in a way to mimic the others’ behaviour. In that case, taking too much yield would not have such a negative effect on yield as the one we measured, although yield was already not a strong belief for gardening in our case study (Table 6).
In addition, we may need to include an indirect form of sanctioning, which happens by gossiping in such communities, as a result of informal monitoring. Both our empirical data and de Moor et al. (2016) point to this. It thus becomes difficult to argue for the absence of sanctioning, as it happens informally even in the absence of plain sanctioning rules.
Regarding urban community resilience, we have voluntarily, in our modelling exercise, restricted the expected outcome to a maintained level of volunteer participation. However, it is perhaps not the most stable state. A resilient urban system should not necessarily return back to one of its previous stable states (Meerow et al. 2016). The abandonment of a community garden is not per se a failure, and may signal the local transition to another state, for example to match new needs or political environments. At this stage of knowledge, both ways to resilience are described in literature: persistence or transition through transformations or changes. Secondly, we have not touched the notion of adaptability of the system, for example with the capacity to change the community rules upon collective decision-making. Such dynamics are possible to study with agent-based modelling (Ghorbani & Bravo 2016).
Regarding our modelling assumption there are several points that may need further development. First, in order to model the design principles, we only considered their effect on the gardening action situations, rather than actually modeling the institutions that are designed based on these principles. This led us to few simplifications of the Design Principles, as explained in Table 9 (ODD). For example, the proportional equivalence benefits/costs was translated into \(DPMaxTakingMoreThanShare\), which indicates how much more than one’s share, a gardener can take, regardless of how much the gardener actually contributed (which is not measured).
Second, in our model, collective action collapses when only one gardener remains. This is a condition, which in real life does not necessarily cause collapse: a gardener could be physically in the garden alone a couple of times, without it causing the end of the collective action. The mechanisms evaluating whether or not collapse occurs thereby were beyond the frame of this research.
Third, in our model, we have not tested the effect of the presence of a core group of members which would take the decisions in the garden. Although recent research suggests that higher social sustainability, which we measure in our work through long-lasting participation, may be attained when decisions are taken by the entire group, rather than by a core group (Rogge et al. 2018), this may still be a point to consider for further extension of the model.
Conclusion
In this research, we have analysed the effects of Ostrom’s Design Principles over time, by studying their dynamic effects on long-term volunteer participation in urban community gardens. Decision trees also proved to be an efficient communication tool in the field.
We have built our model using Ostrom’s Design Principles as the institutional structure which guides and limits the decisions of volunteers to join gardening, a decision itself motivated by individual and collective beliefs, as framed by the Theory of Reasoned Action. We borrowed assumptions from the literature, that the Design Principles lead to a more robust collective resource management and that sanctioning has the notable effect of reducing free-riding, and therefore, enhancing cooperation.
Our model results offer several points of discussion to the Ostrom discourse above. A higher weight placed on social norm facilitates long-lasting participation and increases the trust perceived among gardeners. In addition, the higher the probability for a rule to be violated, the lower the trust. The system performed longer when gardeners joined for social cohesion rather than for taking yield from the garden. This is also confirmed by the fact that higher cohesion values are found when sanctioning is in place. Taking more than its share of yield badly impacts the overall belief for yield taking, while the presence of garden boundaries around the garden increases this belief. Repeated interaction in urban community gardens lowers the negative impact of conflicts. This phenomenon could be linked to trust being generated through such interactions, and suggests a form of adaptability of such communities.
Although we initially found that higher probabilities of sanctioning were correlated with more successful collective action (in terms of lifetime), a closer analysis of the institutional arrangements showed many combinations in which either low sanctioning led to success, or high sanctioning led to failure. Sanctioning has therefore an unclear effect on collective action. More generally, Design Principles do not act in isolation, rather, their specific combination determines the outcomes of the collective action. This was put in evidence thanks to the conditional inference trees. Our study is among the first to confirm this outcome through social simulations, an outcome which was highlighted by recent research Baggio et al. (2016).
We wish to stress the importance to re-evaluate the assumption behind sanctioning, especially for future social simulation work, which is more subject to biased results due to the formulation of assumptions. Although implementing sanctioning in the sense of Ostrom in our model, careful analysis did not always associate successful collective action to higher level of sanctioning. Our empirical database of urban community gardens also indicates a negative correlation between perceived trust among gardeners and active sanctioning. Gardeners do not contribute only for subtractable resources (garden yield), but may contribute for non-subtractable resources as well, which links to the diversity of possible beliefs or motivations (e.g. socialising, education, sustainability purpose). Free-riding, by operating on subtractable resources, is therefore perceived less negatively and the existence of formal sanctioning rules could dissuade gardeners to join, rather than trigger cooperation. Recent research has been contesting the prevalence of sanctions in the management of the commons, suggesting that effort should be put on participatory mechanisms rather than coercive institutions.
Model Documentation
The model description, formatted as an ODD protocol, along with additional information on the data sources, our assumptions, the field study questions and further details on the gardening motivations, can be found in the Appendix.Our model’s Netlogo script along with the LHS parameters used as input in the model can be found in the following online repository:https://www.comses.net/codebases/6ad1edde-d9f3-49f6-ba36-d251ff1886b5/releases/1.1.0/. This folder also contains a pseudocode to help understand the structure of the Netlogo script, and a brief description on how to run the Netlogo script.
Appendix: ODD Model Description
Model overview
Purpose
The purpose of this agent-based model is to study the evolution of volunteer participation over time in urban community gardens, in different institutional contexts (internal rules).
State variables and scales
The model consists of the following concepts:
- Agents - initiators, gardeners and potential gardeners of the community garden. Initiators set up the garden and accept to participate a certain amount of times, regardless of their motivation.
- Individual strategies
- contributing: the agents decide to participate (as gardener) based on behavioural beliefs (individual level, see Table 9) and normative beliefs (social pressure, in our case called social norm);
- taking yield: a gardener chooses an amount of garden yield to take;
- violating a rule: a gardener can violate a garden rule with a certain probability.
- Institutions - The gardeners are bound to follow institutions, which in our case are coded based on Ostrom’s Design Principles (Table 10).
- Outcomes - Agents expect various forms of gratification from participating in urban community gardens. The outcomes are in line with the motivations explained in the Appendix. They are measured, for each experiment, in terms of positive expectation of yield, social cohesion and trust. We also measure the gardening duration, which is defined by the simulation tick at which the collective action stopped.
The model contains several state variables and parameters, either characterizing the agents or the system (Tables 10, 11, 12).
The model runs for a single experimental site over a maximal time of 600 ticks, which corresponds to 6 years.
Beliefs | Practical need | Label |
---|---|---|
Social cohesion/development | Social ties | cohesion |
Enhancing cultural practices | Interaction | social |
Consuming fresh food | Yield | yield |
Saving/making money | Yield | yield |
Enjoying nature | Time on the garden | enjoyinggarden |
Enhancing spiritual practices | Time on the garden | enjoyinggarden |
Environmental sustainability | Contributing to the garden | sustainability |
Education | New knowledge | education |
Land accessibility | Ideal of garden being accessible | landavailability |
Improving health | Time on the garden or yield | yield / enjoyinggarden |
Uncomfortable conditions (negative) | Bad weather or bad conditions | conditions |
Too much work (negative) | Too many tasks | toomuchwork |
Design principle | Assigned Institutional variable | Type variable | Description implementation |
---|---|---|---|
Garden boundaries* | DPplotboundaries | True/false | Boundaries around gardens influence the probability for yield to be stolen, probabilities are indicated by garden |
Garden boundaries | DPprobabilitysanctioning | Floating point \(\in [0.1,0.9]\) | Determines the probability that a rule violation is sanctioned |
Monitoring | |||
Garden boundaries | |||
Garden boundaries | DPfee | Floating point \(\in [0,0.9]\) | Determines the fee to join gardening (0.9 is maximal) |
Collective-choice arrangements | DPglobalprobabilityruleviolation | Floating point \(\in [0.1,0.9]\) | Determines the initial probability of rule violation of a volunteer |
Proportional equivalence benefits/costs | DPMaxTakingMoreThanShare | Floating point \(\in [1,5]\) | Determines the max value of the range from which volunteers randomly choose their desired amount of yield |
Graduated sanctions | DPgraduatedsanctions | Boolean true/false | Determines whether graduated sanctions are active or not |
Conflict-resolution mechanisms | DPconflictharm | Floating point \(\in [0,100]\) | Determines the extent to which a conflict harms trust |
*The potential effect of fences diminishing belief in land availability is not taken into account | |||
State variable | Definition |
---|---|
Lifetime | first moment there is 1 or no volunteer on the garden |
Trust | sum of gardeners’ trust after every tick / total visits. Trust is defined as good encounters / total encounters. |
Cohesion | Sum of gardeners’ cohesion belief after every tick / total visits. Cohesion is defined by the rate of gardeners in the group with whom a gardener has a tie. |
Yield | Sum of gardeners’ yield belief after every tick / total visits. Yield is evaluated positively if the wished amount of yield is received. |
Too much work | Sum of gardeners’ belief for too much work after every tick / total visits. It is evaluated positively if the amount of volunteers is higher than a given threshold. |
Amount of visits | Total number of times an agent became gardener |
Amount of good encounters | Total number of encounters perceived as positive |
Amount of violations | Total number of unsanctioned violations seen |
Amount of own sanctions | Amount of sanctions an agent received. |
Total encounters | Amount of encounters an agent has experienced. |
Parameter | Definition |
---|---|
Cohesion belief strength | Ratio of gardeners with a high belief strength for cohesion. |
Conditions belief strength | Ratio of gardeners with a high belief strength for comfortable conditions. |
Education belief strength | Ratio of gardeners with a high belief strength for education. |
Enjoying garden belief strength | Ratio of gardeners with a high belief strength for enjoying gardening. |
Sustainability belief strength | Ratio of gardeners with a high belief strength for environmental sustainability. |
Land availability belief strength | Ratio of gardeners with a high belief strength for land accessibility. |
Social belief strength | Ratio of gardeners with a high belief strength for social interaction. |
Too-Much-Work belief strength | Ratio of gardeners with a high belief strength for too much work. |
Yield belief strength | Ratio of gardeners with a high belief strength for yield. |
Contributing Threshold | Threshold above which the intention value is high enough for a potential gardener to become gardener |
NoAccessSessions | Amount of sessions an agent cannot join gardening when suspended. |
MaxAmountTellingOffAfterSuspension | Maximum amount a volunteer is told off after being suspended, before having denied access to the garden permanently. |
Membershipduration | Amount of gardening sessions a membership lasts. |
MinAmountOfTellingOff | Minimum amount of times a volunteer is told off before being suspended. |
MaxAmountOfTellingOff | Maximum amount a volunteer can get told off before being suspended |
BalanceAttitudeSocialNorm | The weight of the social norm over individual beliefs (see Behavioural Intention formula below). |
ChanceYieldAvailability | Probability that yield is available on a gardening session. |
ChanceYieldStolenWhenBoundaries | Probability that yield gets stolen when it is available, when there are boundaries around the garden. |
VolunteersToFullySee | Number of other gardeners a gardener can see and evaluate |
AmountOfTasks | Amount of tasks necessary to properly maintain the garden |
Initiators | Amount of initiators the garden started with. |
InitiatorCommittedTime | Amount of time initiators commit. |
Conflict time | Periodic time of conflict. |
Process overview and scheduling
The simulation model consists of two general processes which are depicted in Figure 3.
- Agents participate to the gardening when their intention is higher than the contributing threshold (see subsection Individual Decision Making). The initiators participate for a certain time no matter their motivation.
- Participants, i.e. gardeners, contribute by spending time in the garden. If yield is available, they can take a fair share of it or not, in which case they violate a rule, with a set probability. A violation can be sanctioned, the probability for which depends on the institutions set. The sanction may affect the agent’s rights to come back at the next round. The number of sanctioned violations are counted and influence the participating agents’ belief evaluation values. If yield is not available, the agent’s yield belief evaluation value is updated. Conflicts regarding rules arise periodically: according to how conflict-resolution mechanisms are implemented, this may result in a more or less severe perception of bad encounters. At the end of the round, the actions and outcomes are assessed by the agents: their belief evaluations and probability of violating a rule are hence updated ; the same goes for the attributes of the community and biophysical conditions of the system.
Design concepts
Theoretical and empirical background
We build our model with the overall structure of the Institutional Analysis and Design (IAD) framework (Figure 1, article body): external variables (Biophysical conditions, Attributes of Community and Rules-in-Use) determine the Action situations taken by the agents ; the resulting Interactions and their Outcomes are evaluated to update the external variables and the actions taken. In our case the Biophysical Conditions and Attributes of the community boxes are initially defined thanks to structured interviews in our case-study and to the database of urban community gardens. The Rules-in-Use box derives from Ostrom’s Design Principles, adapted and simplified as visible in Table 10.
For each agent, taking action is evaluated with the formalisation of behaviour dynamics defined in the Theory of Reasoned Action (Figure 2, article body): a resulting behaviour depends both on attitudes and subjective norms (see next subsection).
Individual decision-making and sensing
Based on the TRA, we can formulate the behavioural intention as follows:
\[BI = (A_B)W_1 + (SN)W_2\] |
\(BI\) Behavioural Intention
\(A_B\) Attitude towards performing the behaviour
\(W_1\) Empirically derived weight (see 4.11)
\(SN\) Subjective norm related to performing the behaviour
\(W_2\) Empirically derived weight
The attitude can be calculated by the sum of the belief strength and belief evaluation:
\[A_B = \sum\limits_{i=1}^n b_ie_i\] |
\(b_i\) Belief strength, or the certainty to which the belief is held
\(e_i\) Belief evaluation, the extent to which the attribute is judged to be positive or negative
\(n\) Number of beliefs considered
The social norm is calculated in a similar way:
\[SN = \sum\limits_{i=1}^n b_im_i\] |
\(b_i\) Normative belief strength, or perceived expectation of salient others
\(m_i\) Motivation to comply with the perceived expectation of others.
\(n\) Number of normative beliefs considered
The evaluation of the willingness to participate in community urban gardening is therefore the combination of the functions above:
\[\begin{aligned} Intention & = & ((b_{cohesion} * e_{cohesion}) + (b_{social} * e_{social}) + (b_{yield} * e_{yield}) + (b_{education} * e_{education}) \\ & & + (b_{landavailability} * e_{landavailability}) + (b_{enjoyinggarden} * e_{enjoyinggarden}) \\ & & + (b_{sustainability} * e_{sustainability}) - (b_{conditions} * e_{conditions}) \\ & & - (b_{toomuchwork} * e_{toomuchwork})) * W_1 \\ & & + (b_{needcontribution} * m_{needcontribution}) * W_2\end{aligned}\] |
span class="math inline">\(b_{x}\) Belief strength for x
\(e_{x}\) Belief evaluation for x
\(W_1\) The weight of the attitudes
\(W_2\) The weight of the social norm
The belief evaluation only impacts the overall behavioural intention if the related belief strength is higher than 0. In other words, a gardener for example not volunteering for the purpose of receiving yield, would not see its motivation decrease when no yield is available.
The belief strengths are characteristics of the agents, and range between 0 and 1. They are derived from the survey data in Germany. For example, 80 % join in order to take some garden products, therefore 80 % of the agents generated are given a high value for the corresponding belief strength, between 0.5 and 1. The evaluations also range between 0 and 1. An agent decides to go gardening when the intention is higher than a set decision threshold called \(ContributingThreshold\). An agent either decides to contribute, and thus becomes a gardener, or not to contribute, and becomes or remains a Potential gardener. Gardeners proceed to the next action situations, while Potential gardeners can make the choice to contribute again on the next decision opportunity. This is summarised in Figure 10. The value of this parameter is found by performing a sensitivity analysis with all design principles active and then inactive (see results section).
The weights \(W_1\) and \(W_2\) relate to each other as follows. Each element \(b_im_i\) from the previous formulas has a maximal value of 1. We have 7 positively counted attitudes and 1 social norm, which gives us a maximum total value of our intention formula equal to 8. The weights ratio is therefore:
\[7 * W_1 + 1 * W_2 = 8\] |
\[W_1 = \frac{8 - W_2}{7}\] |
The range of values for \(W_2\) is determined through sensitivity analysis around the ratio \(W_2 / W_1\), based on the study of Eves et al. (2003). This behavioural study is built on the Theory of Planned Behaviour, which is similar to the Theory of Reasoned Action but with the additional component of perceived behavioural control. They determined the weight of attitudes subjective norm by asking 250 people how likely they would perform certain leisure activities in the near future with several scales of 7 possible answers, and how frequently they engaged in such activities in the previous month. The authors thus calculate a numerical value for attitudes and subjective norm. They thus found a values of 5.11 and 5.08, respectively for \(W_2\) and \(W_1\), in the case of participation in team-sports. Gardening is also a collective activity, and we consider a range for \(W_2\) comprised between 0.5 and 4 in order to explore the implications of higher weights of subjective norm. This gives a ratio \(W_2 / W_1\) ranging from 0.47 to 7.
Each agent can assess the behaviour of a certain number of other agents (whether they violated a rule, during contribution on a gardening session). This is parameterized by \(VolunteerToFullySee\).
Learning
At the end of each volunteering session, gardeners evaluate their beliefs according to what happened in the garden. The values of belief evaluation \(e_{x}\) for all beliefs are therefore updated individually for each gardener, with the formula below.
\[e_{belief} = \frac{\sum\limits_{i=1}^n e_{belief, gardeningSession_i}}{AmountOfVisits}\] |
\(e_{belief, gardeningSession_i}\) The evaluation of a belief on gardening session \(i\)
\(AmountOfVisits\) The number of times that an agent chose to become a gardener
- Yield – When the gardener receives a fair share of yield, the belief is evaluated positively. When the gardener does not receive a fair share of yield while it should, the belief is evaluated negatively.
- Social development or cohesion – It is assessed by the density of interpersonal relationships in a group (Friedkin, 2004).
- Some beliefs are always evaluated positively: enjoy gardening, environmental sustainability, cultural practices (requiring at least one other participant on the garden) and land accessibility, when there are no fences.
- Education – Learning happens on the garden (Duchemin et al. 2008; Saldivar-Tanaka & Krasny 2004). Following the concept of learning curves, the more an individual learns, the more time and effort it takes to gain more expertise (Thalheimer 2006) . Therefore, we assume the belief for education starts at 1, and then exponentially decays until 0. The maximum amount of visits after which an agent’s belief for education is 0, is an input parameter. Each agent randomly gets assigned a value between 0 and that input parameter.
- Too-much-work – More tasks require more people. We use a parameter, specific to the garden: the amount of gardeners necessary on a session to sufficiently maintain the garden. Only below this threshold is the session evaluated negatively.
- Uncomfortable conditions – We use a fixed percentage of days with uncomfortable conditions, as provided by the garden leaders of Vredestuin (Netherlands). At the beginning of each session, the agents are given a random number between 0 and 100. If this number is below the percentage above, the conditions are bad and \(e_{conditions}\) has the value 1. Otherwise, it has the value 0.
- Social norm / need of contribution – This evaluation is based on trust and indirect reciprocity. Indirect reciprocity is the belief strength for trust, and gets assigned the highest value, 1. Trust is updated per gardener each round, and becomes the belief evaluation. A gardener can either collaborate or defect during an encounter. Trustworthiness of the group is assessed across encounters with all gardeners. We assume that the reputation of the group directly impacts the individual’s trust.
- Probability of rule violation – Seeing others violating rules increases one’s probability of violating rules (Anderies & Janssen 2016; Ostrom 2005). The initial value of \(ProbabilityRuleviolation\) is determined by the set value of \(DPglobalprobabilityruleviolation\) (Table 10).
\[CurrentCohesion = \frac{PresentTies}{N_{gardeners}}\] |
\(PresentTies\) Amount of relationships an individual has with the other present gardeners
\(N_{gardeners}\) Number of gardeners present
\[Reputation = \frac{p}{n}\] |
\(p\) number of cooperative actions (encounters with someone who is not seen violating a rule or in a bad conflict)
\(n\) number of encounters with others in total
\[ProbabilityRuleviolation = \frac{AmountOfSeenViolationsWithoutSanctions}{TotalEncounters}\] |
\(ProbabilityRuleviolation\) probability for an agent to violate a rule during gardening
\(AmountOfSeenViolationsWithoutSanctions\) amount of violations without sanctions an agent saw
\(TotalEncounters\) total encounters an agent experienced
Our assumptions are summarised in the Appendix (Table 17).
Interaction and collective
Interactions only occur between the gardeners agents, the time of the gardening session. This is open to agents that show a sufficiently high intention to participate (see above), and that are not suspended because of rule violations and the way in which the institutions are implemented (Ostrom Design Principles).
Heterogeneity
Agents are heterogeneous with respect to their belief strengths and homogeneous with respect to all other parameters.
Details
The model has been built in Netlogo. The code to replicate our model is stored on the CoMSES Computational Model Library under the following url: https://www.comses.net/codebases/6ad1edde-d9f3-49f6-ba36-d251ff1886b5/releases/1.1.0/.
Implementation Details
Open NetLogo software. Go to File>Open… [select urbgarden.nlogo]. Select Tools > BehaviorSpace. Choose experiment [ExpFinal] and click Run.
Initialisation
The model starts with giving fixed belief strengths values to agents, according to the general characteristics of an urban community gardening community. All agents start with a belief evaluation of 1 (maximum) for education, land availability, enjoying gardening, sustainability and reciprocity (or trust). They start with a belief evaluation of 0 (minimum) for cohesion. Belief evaluation values evolve over time, with the learning processes described above.
Additional data
Motivations | Justification |
---|---|
Social development | The activities of gardening foster a social environment that enhances the activity itself by providing participants with a social network that becomes important particularly when they are feeling isolated (Duchemin et al. 2008). |
Social cohesion | People feel connected to each other (de Kam & Needham 2004); participants form relationships with one another and offer mutual help, which does not occur in individual gardening systems (Veen et al. 2016) |
Consuming fresh food | It depends on the stage of the garden, biophysical variables but also on the active institutions and the participants’ behaviour (Duchemin et al. 2008); it is a possible source of conflict when it comes to (fair) yield taking (Butler 2013; Charles 2012) or even stolen yield from non-participants (Ruggeri et al. 2016) |
Saving/making money | Eating or selling own garden production is a current practice (Guitart et al. 2012; Patel 1991). |
Improving health | Improving a diet, increased exercise and involvement in nature (Guitart et al. 2012) |
Enjoying nature | This point is debatable when it comes to man-made gardens ; however, this belief is intended as the well-being provided by being outdoor (Rogge et al. 2018) . |
Education | Specific education about gardening (Drake & Lawson 2015) or more general: science, nutrition and environmental education (Guitart et al. 2012) ; indirect social education can also be gained by simply participating (Duchemin et al. 2008) |
Enhancing cultural practices | Cultural practices are broadly defined as the knowledge of ‘what to do, when and where’, and how to interact within a particular culture; in the urban gardening context, this can be translated to integration, particularly for foreign immigrants; in our work, this belief is satisfied by the presence of others in the garden (Rogge et al. 2018). |
Increasing land accessibility | This belief reflects the very common issue of claim to the urban public space, when its accessibility is reduced due to land developments and privatisations (Huron 2015; Williams 2018). Urban community gardens also add the idea of increasing the share of green spaces in the city (Schmelzkopf 2002). This belief can be diminished by the presence of group or garden boundaries (Milburn & Vail 2010). |
Environmental sustainability | Green spaces highly contribute to urban sustainability, such as microclimate regulation, water runoff, pollution mitigation, water filtering or biodiversity ((Colding & Barthel 2013), (Wolch et al. 2014), to which community gardening practices (permaculture, organic farming or conventional) contribute. However, few studies, such as Rogge et al. (2018), have actually evaluated this impact. We translate this belief as the participants’ belief of acting positively towards the environment. |
Enhancing spiritual practice | This relates to the connection to nature achieved through gardening; it acts as meditation, a way to release tensions and developing spirituality (Kingsley et al. 2009), and engaging in more caring connections with other people (Okvat & Zautra 2011). |
Social norm | Trust in contributing to the community, which is higher when other users are reciprocating (Chalise 2015), more likely occurs in smaller groups (Poteete & Ostrom 2004). Reciprocity can be measured in two ways: as a norm in the group and as a variable between two agents. Because we are looking at the relation of an individual with the group, reciprocity as a norm is more applicable. The higher this societal reciprocity, the more one expects all agents to reciprocate (Mui 2002); the group’s overall reputation is an important factor when deciding whether or not to contribute at the individual level. |
Amount of work | It is the amount of activities leading to a desired quality ; gardeners leave if maintaining the garden requires more effort than they expected (Chalise 2015), which can happen when there are not enough gardeners. |
Uncomfortable conditions | We consider here physical conditions, such as bad weather, limiting the willingness to participate (Drake & Lawson 2015; Vercauteren et al. 2013). Other conditions, such as the feeling of not being welcome, are not considered in this work. |
Question | Gandhi Tuin | Vredestuin |
---|---|---|
Are there plot boundaries? | Yes | No |
Are there group boundaries? | No | No |
When a membership structure is active, people can try the garden 3 times before they have to become a member | / | / |
Are there rules towards balanced benefits/costs? | no | no |
Are decisions taken collectively? | yes | no * |
Are there conflict-resolution mechanisms? | yes, but poorly executed | no, but no conflicts arose yet |
How often does a large conflict, which could harm the volunteer’s trust in a collaborative community, occur? | 150 to 200 sessions | / |
Without effective conflict resolution mechanisms in place, what is the probability for such a large conflict to harm the trust of an individual volunteer ? | 1/2 | 1/4 |
Is there monitoring in place? | no | no |
Are there graduated sanctions? | yes | no |
What is the probability that someone is punished (told off) when violating a rule? | between 0.25 and 0.6 | / |
Is your initiative officially recognised and allowed? | yes | yes |
How many other volunteers can a volunteer assess the contribution of during a gardening session? | 3-4 | 3-4 |
What is the chance for an individual to violate a rule during a session (such as not tidying the kitchen, or bringing a dog)? | 1/30 | 1/30 |
What is the minimal amount of volunteers necessary for proper maintenance of garden? | 10 | 10 |
How many core members are there? | 4 | 20-25 |
What is the size of the volunteer pool? | >1000 | >1000 |
What is the fraction of sessions with uncomfortable conditions? | 1/4 | 1/4 |
What is the fraction of sessions with available yield? | 1 | 1 |
What is the fraction of sessions when yield gets stolen while there are no boundaries around the garden? | / | 1/100 |
After how much time does a volunteer stop learning in the garden? | / | 1.5 year |
IAD component | Output variables | Input parameters | Data source |
---|---|---|---|
Biophysical conditions | Product availability | Chance of uncomfortable conditions chance of available product | Gandhi Tuin |
Community attributes | Beliefs types number of initiators pool of potential volunteers beliefs strength (all agents) chance of bonding with others age of initiative (maximum) interaction rate | German database, Gandhi Tuin, Vredestuin, literature, case-study | |
Rules-in-use | Ostrom Design Principles (adapted) | Literature, Gandhi Tuin, Vredestuin | |
Action situations | Tasks | Gandhi Tuin | |
Outcomes | Beliefs evaluation social ties good encounters gardening duration | Conflict rate | Gandhi Tuin |
Quantitative values are only used during validation | |||
Belief label | Proportion of gardeners concerned (%) |
---|---|
Social | 30 |
Cohesion | 90 |
Yield | 60 |
Enjoyinggarden | 80 |
Sustainability | 60 |
education | 50 |
Landavailability | 30 |
Conditions | 60 |
Toomuchwork | 20 |
Assumption | Comment |
---|---|
The impression of being helpful in the garden increases the behavioural intention to contribute as well | |
Initiators usually stay committed to maintaining the garden longer than regular volunteers | |
Gardeners only negatively evaluate yield taking when their fair share of yield is unavailable | |
Enjoy gardening, environmental sustainability, cultural practices (requiring at least 1 other participant on the garden) and land accessibility (when no garden boundaries) are always evaluated positively | |
After 100 to 400 sessions, educational purpose is not a relevant attitude anymore | Varying range |
Agents can be told off 2 to 40 times before being suspended | |
An agent is suspended for 5 to 20 sessions | No cases with suspensions were noted in the examples from Germany |
After having been suspended, an agent can be told off 2 to 10 times before being denied access | |
A conflict can harm trust up to a 100 times worse than seeing someone violate a rule | |
Taking too much yield means taking up to 5 times the fair amount | 5 could be too much |
The probability for rule violation during gardening is between 0.01 and 0.9 | Varying range |
When violating a rule, the probability of being sanctioned is between 0.01 and 0.9 | Varying range |
Membership can last 13 to 52 weeks | |
The perception of Too-much-work is a barrier to participation | Confirmed by (Chalise 2015) |
References
AGRAWAL, A. (2002). Common resources and institutional sustainability. In The drama of the commons. The National Academies Press.
ANDERIES, J., & Janssen, M. (2016). Sustaining the commons. Arizona State University Press.
ANDERIES, J. M. (2014). Embedding built environments in social-ecological systems: Resilience-based design principles. Building Research and Information, 42(2), 130–142. [doi:10.1080/09613218.2013.857455]
BAGGIO, J. A., Barnett, A. J., Perez-Ibara, I., Brady, U., Ratajczyk, E., Rollins, N., Rubiños, C., Shin, H. C., Yu, D. J., Aggarwal, R., Anderies, J. M., & Janssen, M. A. (2016). Explaining success and failure in the commons: The configural nature of Ostrom’s institutional design principles. International Journal of the Commons, 10(2), 417–439. [doi:10.18352/ijc.634]
BORCH, C., & Kornberger, M. (Eds.). (2015). Urban commons: Rethinking the city. Routledge.
BOWERS, C. (2009). Educating for a revitalization of the cultural commons. Canadian Journal of Environmental Education, 14, 196–200.
BOYD, R., Richerson, P. J., Meinzen-Dick, R., de Moor, T., Jackson, M. O., Gjerde, K. M., Harden-Davies, H., Frischmann, B. M., Madison, M. J., Strandburg, K. J., McLean, A. R., & Dye, C. (2018). Tragedy revisited. Science, 362(6420), 1236–1241. [doi:10.1126/science.aaw0911]
BUTLER, A. (2013). Collective action in UK urban community growing projects: A comparative analysis.
CHALISE, N. (2015). Collective action dynamics in urban neighborhoods: A study of urban community gardens. Washington University in St. Louis, PhD Thesis.
CHARLES, D. (2012). At the community garden, it’s community that’s the hard part.
CHELLERI, L., Waters, J. J., Olazabal, M., & Minucci, G. (2015). Resilience trade-offs: Addressing multiple scales and temporal aspects of urban resilience. Environment and Urbanization, 27(1), 181–198. [doi:10.1177/0956247814550780]
COLDING, J., & Barthel, S. (2013). The potential of ’Urban Green Commons’ in the resilience building of cities. Ecological Economics, 86, 156–166. [doi:10.1016/j.ecolecon.2012.10.016]
COX, M., Arnold, G., & Tomás, S. V. (2010). A review of design principles for community-based natural resource management. Ecology and Society, 15(4). [doi:10.5751/es-03704-150438]
DARNTON, A. (2008). Reference report: An overview of behaviour change models and their uses.
DE Kam, G., & Needham, B. (2004). Een hele opgave: Over sociale cohesie als motief bij stedelijke herstructurering. DGW/Nethur Partnership.
DE Moor, T., Laborda-Pemán, M., Lana-Berasain, J. M., van Weeren, R., & Winchester, A. (2016). Ruling the commons. Introducing a new methodology for the analysis of historical commons. International Journal of the Commons, 10(2), 529–588. [doi:10.18352/ijc.760]
DERKZEN, M., Nagendra, H., van Teeffelen, A. J. A., Purushotham, A., & Verburg, P. H. (2017). Shifts in ecosystem service supply in deprived urban areas: Understanding people’s responses and consequences for well-being. Ecology and Society, 22(1), 51. [doi:10.5751/es-09168-220151]
DRAKE, L., & Lawson, L. J. (2015). Results of a US and Canada community garden survey: Shared challenges in garden management amid diverse geographical and organizational contexts. Agriculture and Human Values, 32(2), 241–254. [doi:10.1007/s10460-014-9558-7]
DUCHEMIN, E., Wegmuller, F., & Legault, A. M. (2008). Urban agriculture: Multi-dimensional tools for social development in poor neighbourhoods. Field Actions Science Reports, 1(2008). [doi:10.5194/facts-2-1-2009]
EVES, F., Hoppé, R., & McLaren, L. (2003). Prediction of specific types of physical activity using the theory of planned behavior. Journal of Applied Biobehavioral Research, 8, 77–95. [doi:10.1111/j.1751-9861.2003.tb00086.x]
FISHBEIN, M., & Ajzen, I. (2011). Predicting and changing behavior: The reasoned action approach. Taylor & Francis.
FOSTER, S. R. (2011). Collective action and the urban commons. Notre Dame Law Review, 87(1), 57–133.
FOSTER, S. R., & Iaione, C. (2015). The city as a commons. Yale Law & Policy Review, 34(2), 281.
FOSTER, S. R., & Iaione, C. (2019). Ostrom in the city: Design principles and practices for the urban commons. In B. Hudson, J. Rosenboom, & D. Cole (Eds.), Routledge handbook of the study of the commons (pp. 235–255). Routledge. [doi:10.4324/9781315162782-19]
FRIEDKIN, N. E. (2004). Social cohesion. Annual Review of Sociology, 30(1), 409–425.
GHORBANI, A., Bots, P., Dignum, V., & Dijkema, G. (2013). MAIA: A framework for developing agent-Based social simulations conceptualizing social systems. Journal of Artificial Societies and Social Simulation, 16(2), 9. [doi:10.18564/jasss.2166]
GHORBANI, A., & Bravo, G. (2016). Managing the commons: A simple model of the emergence of institutions through collective action. International Journal of the Commons, 10(1), 200–219.
GUITART, D., Pickering, C., & Byrne, J. (2012). Past results and future directions in urban community gardens research. Urban Forestry & Urban Greening, 11(4), 364–373. [doi:10.1016/j.ufug.2012.06.007]
HESS, C. (2008). Mapping the new commons. In Available at SSRN: https://ssrn.com/abstract=1356835.
HOTHORN, T., Hornik, K., & Zeileis, A. (2006). Unbiased recursive partitioning: A conditional inference framework. Journal of Computational and Graphical Statistics, 15(3), 651–674. [doi:10.1198/106186006x133933]
HURON, A. (2015). Working with strangers in saturated space: Reclaiming and maintaining the urban commons. Antipode, 47(4), 963–979. [doi:10.1111/anti.12141]
HURON, A. (2017). Theorising the urban commons: New thoughts, tensions and paths forward. Urban Studies, 54(4), 1062–1069. [doi:10.1177/0042098016685528]
KIM, D., & Lim, U. (2016). Urban resilience in climate change adaptation: A conceptual framework. Sustainability, 8(5), 405. [doi:10.3390/su8040405]
KINGSLEY, J. Y., Townsend, M., & Henderson-Wilson, C. (2009). Cultivating health and wellbeing: Members’ perceptions of the health benefits of a Port Melbourne community garden. Leisure Studies, 28(2), 207–219. [doi:10.1080/02614360902769894]
MCGINNIS, M. D. (2011). An introduction to IAD and the language of the Ostrom workshop: A simple guide to a complex framework. Policy Studies Journal, 39(1), 169–183. [doi:10.1111/j.1541-0072.2010.00401.x]
MEEROW, S., Newell, J. P., & Stults, M. (2016). Defining urban resilience: A review. Landscape and Urban Planning, 147, 38–49. [doi:10.1016/j.landurbplan.2015.11.011]
MILBURN, L. A. S., & Vail, B. A. (2010). Sowing the seeds of success cultivating a future for community gardens. Landscape Journal, 29(1), 71–89. [doi:10.3368/lj.29.1.71]
MUI, L. (2002). Computational models of trust and reputation: Agents, evolutionary games, and social networks. Massachusetts Institute of Technology, PhD Thesis.
MÜLLER, C. (2007). Intercultural gardens. Urban places for subsistence production and diversity. German Journal of Urban Studies, 46(1), 1–6.
NETTLE, C. (2014). Community gardening as social action. Routledge.
OKVAT, H. A., & Zautra, A. J. (2011). Community gardening: A parsimonious path to individual, community, and environmental resilience. American Journal of Community Psychology, 47(3–4), 374–387. [doi:10.1007/s10464-010-9404-z]
OSTROM, E. (1990). Governing the commons. The evolution of institutions for collective action. Cambridge University Press. [doi:10.1017/cbo9780511807763]
OSTROM, E. (2002). Chapter 24 Common-pool resources and institutions: Toward a revised theory. In B. L. Gardner & G. C. Rousser (Eds.), Handbook of agricultural economics (Vol. 2, pp. 1315–1339). North-Holland. [doi:10.1016/s1574-0072(02)10006-5]
OSTROM, E. (2005). Understanding institutional diversity. Princeton University Press.
OSTROM, E. (2009a). A general framework for analyzing sustainability of social-ecological systems. Science, 325(5939), 419–422. [doi:10.1126/science.1172133]
OSTROM, E. (2009b). Design principles of robust property-Rights institutions: What have we learned? In G. K. Ingram & Y. H. Hong (Eds.), Property rights and land policies (pp. 1–28). Lincoln Institute of Land Policy.
OSTROM, E. (2011). Background on the institutional analysis and development framework. Policy Studies Journal, 39(1), 7–27. [doi:10.1111/j.1541-0072.2010.00394.x]
OSTROM, E., Gardner, R., & Walker, J. (1994). Rules, games, and common-Pool resources. University of Michigan Press. [doi:10.3998/mpub.9739]
OSTROM, E., Gardner, R., Walker, J., & Walker, J. (1994). Rules, games, and common-Pool resources. University of Michigan Press. [doi:10.3998/mpub.9739]
PATEL, I. C. (1991). Gardening’s socioeconomic impacts. Journal of Extension, 29(4), 7–8.
PEARSON, D. H., & Firth, C. (2012). Diversity in community gardens: Evidence from one region in the United Kingdom. Biological Agriculture & Horticulture, 28(3), 147–155. [doi:10.1080/01448765.2012.706400]
PETRESCU, D., Petcou, C., & Baibarac, C. (2016). Co-producing commons-based resilience: Lessons from R-Urban. Building Research & Information, 44(7), 717–736. [doi:10.1080/09613218.2016.1214891]
POTEETE, A. R., Janssen, M. A., & Ostrom, E. (2010). Working together: Collective action, the commons, and multiple methods in practice. Princeton University Press. [doi:10.1515/9781400835157]
POTEETE, A. R., & Ostrom, E. (2004). Heterogeneity, group size and collective action: The role of institutions in forest management. Development and Change, 35(3), 435–461. [doi:10.1111/j.1467-7660.2004.00360.x]
ROBSON, J. P., Sinclair, A. J., & Diduck, A. P. (2015). A study of institutional origins and change in a Canadian urban commons. International Journal of the Commons, 9(2), 698–719. [doi:10.18352/ijc.548]
ROGGE, N., & Theesfeld, I. (2018). Categorizing urban commons: Community gardens in the Rhine-Ruhr agglomeration, Germany. International Journal of the Commons, 12(2), 251–274. [doi:10.18352/ijc.854]
ROGGE, N., Theesfeld, I., & Strassner, C. (2018). Social sustainability through social interaction - A national survey on community gardens in Germany. Sustainability, 10(4), 1085. [doi:10.3390/su10041085]
RUGGERI, G., Mazzocchi, C., & Corsi, S. (2016). Urban gardeners’ motivations in a metropolitan city: The case of Milan. Sustainability, 8(11), 1099. [doi:10.3390/su8111099]
SAFRANSKY, S. (2017). Rethinking land struggle in the postindustrial city. Antipode, 49(4), 1079–1100. [doi:10.1111/anti.12225]
SALDIVAR-TANAKA, L., & Krasny, M. E. (2004). Culturing community development, neighborhood open space, and civic agriculture: The case of Latino community gardens in New York City. Agriculture and Human Values, 21(4), 399–412. [doi:10.1023/b:ahum.0000047207.57128.a5]
SASSEN, S. (2011). La ciudad global es un espacio de contradicciones.
SCHAUPPENLEHNER-KLOYBER, E., & Penker, M. (2016). Between participation and collective action - From occasional liaisons towards long-Term co-Management for urban resilience. Sustainability, 8(7), 664. [doi:10.3390/su8070664]
SCHMELZKOPF, K. (2002). Incommensurability, land use, and the right to space: Community gardens in New York City. Urban Geography, 23(4), 323–343. [doi:10.2747/0272-3638.23.4.323]
SPILKOVÁ, J. (2017). Producing space, cultivating community: The story of Prague’s new community gardens. Agriculture and Human Values, 34(4), 887–897.
TEN Broeke, G., van Voorn, G., & LigTENberg, A. (2016). Which sensitivity analysis method should i use for my agent-based model? Journal of Artificial Societies and Social Simulation, 19(1), 5. [doi:10.18564/jasss.2857]
THALHEIMER, W. (2006). Spacing learning events over time: What the research says.
VAN Dam, K. H., Nikolic, I., & Lukszo, Z. (2012). Agent-Based modelling of socio-Technical systems. Springer Science & Business Media.
VEEN, E. J., Bock, B. B., van den Berg, W., Visser, A. J., & Wiskerke, J. S. (2016). Community gardening and social cohesion: Different designs, different motivations. Local Environment, 21(10), 1271–1287. [doi:10.1080/13549839.2015.1101433]
VENKATASUBRAMANIAM, A., Wolfson, J., Mitchell, N., Barnes, T., Jaka, M., & French, S. (2017). Decision trees in epidemiological research. Emerging Themes in Epidemiology, 14(1), 1–12. [doi:10.1186/s12982-017-0064-4]
VERCAUTEREN, C., Quist, J., van Bueren, E., & Veen, E. (2013). Community gardens as learning spaces for sustainable food practices.
WILENSKY, U., & Shargel, B. (2002). Behaviorspace.
WILLIAMS, M. J. (2018). Urban commons are more-than-property. Geographical Research, 56(1), 16–25. [doi:10.1111/1745-5871.12262]
WILSON, D. S., Ostrom, E., & Cox, M. E. (2013). Generalizing the core design principles for the efficacy of groups. Journal of Economic Behavior & Organization, 90, S21–S32. [doi:10.1016/j.jebo.2012.12.010]
WOLCH, J. R., Byrne, J., & Newell, J. P. (2014). Urban green space, public health, and environmental justice: The challenge of making cities ’just green enough’. Landscape and Urban Planning, 125, 234–244. [doi:10.1016/j.landurbplan.2014.01.017]
ŁAPNIEWSKA, Z. (2017). (Re)claiming space by Urban commons. Review of Radical Political Economics, 49(1), 54–66. [doi:10.1177/0486613415616217]