Integrating Equity Considerations into Agent-Based Modeling: A Conceptual Framework and Practical Guidance

, , , , ,
and
aDepartment of Industrial and Operations Engineering, University of Michigan, Ann Arbor, MI, USA, United States; bSchool of Environmental and Forest Sciences, University of Washington, Seattle, WA, USA, United States; cDepartment of Civil and Natural Resources Engineering, University of Canterbury, New Zealand; dDepartment of Geography, University of Alabama, Tuscaloosa, AL, USA, United States; eDepartment of Ecological Modelling, Helmholtz-Centre for Environmental Research - UFZ, Leipzig, Germany; fDepartment of Natural Resources and the Environment, Cornell University, Ithaca NY USA, United States
Journal of Artificial
Societies and Social Simulation 25 (3) 1
<https://www.jasss.org/25/3/1.html>
DOI: 10.18564/jasss.4816
Received: 26-Oct-2021 Accepted: 23-Mar-2022 Published: 31-Mar-2022
Abstract
Advancing equity is a complex challenge for society, science, and policy. Agent-based models are increasingly used as scientific tools to advance understanding of systems, inform decision-making, and share knowledge. Yet, equity has not received due attention within the agent-based modeling (ABM) literature. In this paper, we develop a conceptual framework and provide guidance for integrating equity considerations into ABM research and modeling practice. The framework conceptualizes ABM as interfacing with equity outcomes at two levels (the science-society interface and within the model itself) and the modeler as a filter and lens that projects knowledge between the target system and the model. Within the framework, we outline three complementary, equity-advancing action pathways: (1) engage stakeholders, (2) acknowledge positionality and bias, and (3) assess equity with agent-based models. For Pathway 1, we summarize existing guidance within the participatory modeling literature. For Pathway 2, we introduce the positionality and bias document as a tool to promote modeler and stakeholder reflexivity throughout the modeling process. For Pathway 3, we synthesize a typology of approaches for modeling equity and offer a set of preliminary suggestions for best practice. By engaging with these action pathways, modelers both reduce the risks of inadvertently perpetuating inequity and harness the opportunities for ABM to play a larger role in creating a more equitable future.Introduction
Notions of equity, justice, and fairness are increasingly considered as key elements of sustainability and therefore increasingly feature in public, scientific, and policy discourses (Clark & Harley 2020; UN General Assembly 2015). These normative considerations have been driven, in part, by rising levels of inequality (Zucman 2019), the increasing and unequal effects of environmental risks (Tol et al. 2004), and recent events that have encouraged reflection on institutionalized discrimination and unseen biases (e.g., the Black Lives Matter movement). As a tool that interacts with these discourses, it is important for developers and practitioners of agent-based modeling (ABM) to reflect on how the tool can advance or hinder equity and, in particular, on the role of modelers as key agents in this process. With this paper, we aim to outline a vocabulary and a set of promising pathways with which modelers could begin to advance equity, through both their scientific conduct and the models they build.
As the central conceptual lens for this paper, we interpret equity to be about the relative situations of different groups in society, with the normative goal of ‘fairness’ across three ‘dimensions of justice.’ These dimensions encompass how groups are differentially recognized by society (recognitional justice), their ability to affect decision-making (procedural justice), and their access to resources and exposure to burdens (distributional justice) (Fraser 1995; Rawls 2001; Tyler 2000). To assess equity across these dimensions requires additional meta-level considerations around what is ‘fair,’ who and what are the subjects and objects of equity, and how personal values might influence these meta-level considerations (Fraser 1995; Leach et al. 2018; McDermott et al. 2013; Sikor et al. 2014). As an unavoidably normative exercise, examinations of equity necessarily require value judgments.
There are many opportunities for computational models to play a role in improving equity. Models are useful tools for evaluating the effects of potential interventions, such as policy changes or fairer decision-making procedures (Corbett-Davies et al. 2017; Davies 2008), on distributional outcomes. They can be used to formalize multiple worldviews and framings of societal problems, in order to stimulate discussion and facilitate agreement between diverse stakeholders (Lempert & Turner 2021). And they are low-cost and thus potentially more inclusive complements to traditional field research (Murphy et al. 2020). However, there is also a risk that models perpetuate inequity by inadequately considering historical and structural mechanisms of discrimination (Zelner et al. 2022). For instance, models can inadvertently recreate biases in input data (Obermeyer et al. 2019), as well as misrepresent or tokenize stakeholder knowledge (Inman et al. 2021). In order to leverage these opportunities and reduce these risks, concepts of equity need to be integrated into modeling paradigms and practices.
Among modeling approaches, ABM offers a particularly large but underexplored promise to engage with equity. Agent-based models simulate an interacting, heterogeneous population of autonomous actors, and can be applied to investigate the emergence of system-level and distributional outcomes through both top-down and bottom-up processes. Agent-based models are therefore particularly suited to represent problems of (in)equity, relative to other modeling approaches that assume human actors to be homogeneous. Indeed, such features inspired some of the seminal advances within the ABM field, including Schelling’s segregation model (Schelling 1971) and Epstein and Axtell’s Sugarscape (Epstein & Axtell 1996), which sought to explain the emergence of macro-level inequalities from micro-level processes. ABM has since been applied in upwards of 7500 publications in a broad range of academic disciplines (Janssen et al. 2020) and with a huge diversity of modeling purposes and levels of empirical embeddedness (Sun et al. 2016). As ABM has grown in prominence, so too has a substantial base of guidance and best practices for ABM development and communication (Augusiak et al. 2014; Grimm et al. 2020; Groeneveld et al. 2017).
However, there remains a lack of integration of equity considerations into ABM theory and practice. For instance, a Scopus search for the terms ("agent-based" OR "agent based") AND (model*) on 31 March 2021 yielded over 30,000 results. Adding the search term (*equit* OR disparit* OR *justice* OR *fairness*) reduced the results to 344, i.e., just over 1% of publications. Given contemporary discourses about how to advance equity and the untapped potential for ABM to play a role in doing so, such an integration is timely.
In this paper, we advance the basis for the integration of equity with ABM by addressing the following two aims:
- Develop a conceptual framework that positions equity within ABM practice and application;
- Provide actionable pathways for advancing equity in ABM research.
To achieve the first aim, we draw from equity theory and its interactions with the modeling process. For the second aim, we assess the state of practice with respect to the framework by collecting and synthesizing a non-exhaustive sample of research at the equity-ABM interface. We then present guidance for future work based on this synthesis.
We intend our framework and guidance to be of interest to (agent-based) modelers and non-modelers alike. For modelers, we illustrate a set of concrete pathways for integrating equity considerations throughout model development and application. These pathways were developed with ABM in mind, but are applicable to process-based computational modeling in general. For non-modelers interested in equity (e.g., academics, practitioners, or decision-makers), we demonstrate how ABM could improve understanding of the role of equity within systems as well as evaluate interventions to address equity-centered problems.
Given the subjectivity inherent in notions of equity, it is important to acknowledge that we (the authors) all work broadly within the field of sustainability science, which typically assumes a particular normative stance on equity. It is our view that modelers, by virtue of their ability to select and represent features in a virtual world that can be used to influence discussions about actions in the real world, assume a powerful role. With that power comes a responsibility for modelers to reflect on their perspectives, advantages, and blind spots, particularly as they relate to the perspectives and needs of the less powerful. Because we often work in contexts where we are outsiders as researchers from the Global North, we believe that such considerations are particularly important where we risk perpetuating colonial legacies and disempowering the communities in which we work. See Appendix 1 for more detailed personal reflections.
Background
Equity
For this paper, we consider the following elements as necessary to understand and examine equity (Fraser 2009; Leach et al. 2018; McDermott et al. 2013; Sikor et al. 2014):
- Dimensions of justice: What does equity consist of?
- Subjects: Equity of what and for whom?
- Fairness principles (criteria): Why equity and what are its goals?
- Reflexivity: How are the above parameters determined?
Dimensions of justice. There are three dimensions of justice discussed in the literature: recognitional, procedural, and distributional. They represent the ultimate outcomes that equity aspires to achieve, i.e., in a perfect world, justice would be achieved across these three dimensions. Recognitional justice is about acknowledging the unique circumstances, histories, and capabilities of individuals and communities (Fraser 1995; Nussbaum & Sen 1993; Sikor et al. 2014). Recognitional aspects emphasize equity as a context-dependent phenomenon that can only be understood within the culture, beliefs, practices, and institutions of a specific society (McDermott et al. 2013). Procedural justice describes people’s inclusion in and ability to influence decision-making (Arnstein 1969; Tyler 2000), and is closely related to notions of power and agency. Distributional justice, the most commonly and easily assessed, describes how resources and burdens are distributed within society (Rawls 2001). Although conceptually distinct, these three dimensions are interconnected as, for example, procedural injustice can lead to distributional injustice (Leach et al. 2018).
Subjects. Across these dimensions of justice, there exist the overarching questions of “equity of what” and “equity between whom,” i.e., the objects and subjects of equity. Such considerations are inevitably context-specific, but “equity of what” typically relates to primary goods in society (e.g., health, civil rights, income, social respect) (Rawls 2001) and “equity between whom” can incorporate diverse components of socio-cultural identity (e.g., gender, race, age).
Fairness principles (criteria). Underlying any assessment of equity are some criteria of ‘fairness.’ These criteria add an ethical and normative component to equity. An intuitive notion of fairness is egalitarianism (Konow 2003), which aims for an equal distribution of outcomes between people or groups. Prevailing notions of equity, however, adopt a needs-based principle that favors the least advantaged in society (Rawls 2001). This explains the emphasis of much equity discourse (including that in this paper) on elevating marginalized and vulnerable groups. However, an equity assessment can adopt fairness principles other than a needs-based one.
Reflexivity. Decisions and judgements about these previous elements are unavoidably subjective. This final pillar posits that a necessary consideration when examining equity is to reflect on how the other elements are determined. Although such decisions would ideally be made through a democratic process involving all affected parties (Fraser 2009; McDermott et al. 2013), it is in any case important for researchers and decision-makers to reflect on how their positionality (i.e., their socio-cultural identities in relation to the research context Milner 2018) may have influenced these decisions and their outcomes. This is known as reflexivity.
The equity-ABM interface
Although there has thus far been no explicit analysis of the interface of equity and ABM, much existing literature already discusses risks and opportunities for ethical and rigorous ABM practice. In this section, we summarize some of this work and relate the issues raised therein to the equity language introduced in the previous section (see Table 1). We structure our summary into two general levels: the modeling process and the model itself. Given the overlap of ABM with other modeling approaches, we also draw from lessons learned elsewhere, and particularly from machine learning as equity has received significant attention there.
ABM component | Sub-component | Risks to equity |
Modeling process | The social context | Modeler misunderstands the target system Modeler assumes a powerful role Stakeholder engagement is only superficial |
Abstraction | Bias in abstracting to mathematical representation Modeler must reconcile multiple worldviews | |
Interpretation | Modeler interprets the model as reality Problematic interpretation is attached to model outputs Model outputs are interpreted as a decision | |
Model | Purpose | Purpose is unclear, resulting in problematic model interpretation |
Inputs and parameterization | Data contain embedded historical inequities | |
Data inadequately represent marginalized social groups Modeler’s choice of metrics and data sources can (dis)advantage certain social groups | ||
Processes | Model processes misrepresent or are prejudiced against particular groups | |
Outputs | Averaging masks distributional outcomes | |
Elements of equity in model development
The social context. Agent-based models do not exist in a vacuum, and “modeling is a social practice embedded in power relations” (Leblond & Trottier 2016 p. 21). The modeler assumes a powerful role in this process, for instance by selecting data, designing model structures, and interpreting results. In this role, there is a risk that the modeler ignores alternative perspectives and their implications, and thereby reinforces procedural injustices. Stakeholders, i.e., people affecting or affected by actions of the system (Grimble & Chan 1995) (e.g., modeled subjects and model users), can have viewpoints, values, and epistemologies that are discordant with the modeler’s (and with each other’s) (Voinov & Bousquet 2010). Not engaging or only superficially engaging (i.e., tokenizing) these perspectives can reinforce both recognitional and procedural injustices.
Abstraction. Models never represent all details of the target system and must contain some degree of abstraction, both to keep model development tractable (what is modeled) and to make complex constructs amenable to computer code (how it is modeled). The modeler must decide which features of the target system to include and leave out, reconcile multiple worldviews, and map theoretical constructs to observable characteristics (Diallo et al. 2019; Jacobs & Wallach 2021). The flexibility of agent-based models means that they can contain a huge number of structural decisions (Ayllón et al. 2021; Sun et al. 2016), each of which involves some value judgment and therefore potential for bias (Aodha & Edmonds 2017). Such biases may pose risks to recognitional and procedural justice, as abstraction may diminish certain worldviews or result in model structures that inadvertently discriminate or overlook vulnerabilities. For instance, the assumption that more food production equates with better food security can disadvantage vulnerable populations who do not have the means to access that food.
Interpretation. The complement to abstraction is interpretation: the modeler must attach meaning to model outputs. A key risk here is one of ‘reification,’ where the modeler forgets that the model is an abstraction of the target system and the model becomes a set of “theoretical spectacles” that they use to interpret the world (Aodha & Edmonds 2017). A related risk is that model outputs are interpreted as decisions in themselves (e.g., if one intervention is superior to another in the model), rather than as inputs to decision-making processes (Aodha & Edmonds 2017; Shults & Wildman 2019). Depending on the model’s purpose and use, this can generate many kinds of inequity, such as recommending decisions that lead to distributional injustice or promoting an understanding of processes that inadequately recognizes certain communities. For example, abstract agent-based explorations of ethnocentrism (the tendency to favor one’s own group at the expense of others) can be incautiously interpreted as arguments in support of anti-immigrant actions (Hammond & Axelrod 2006; Hartshorn et al. 2013).
Elements of equity within agent-based models
Purpose. All ABM begins with a purpose or a question it seeks to shed light on. Agent-based models are built for a diverse range of purposes, ranging across levels of prediction and explanation (i.e., both pattern and process understanding). Correspondingly, agent-based model structure can take a range of levels of complicatedness and empirical embeddedness (Le Page & Perrotton 2018; Sun et al. 2016). This diversity distinguishes ABM from other computational modeling fields, such as machine learning, which more consistently aims at accurate prediction than process understanding. Different modeling purposes and levels of complicatedness likely raise distinct equity considerations. For instance, highly theoretical modeling purposes may be perceived as less embedded in distinct social contexts, so building recognitional justice may require less stakeholder engagement. Nevertheless, an overarching risk is that a model is applied to a different purpose than originally intended (Edmonds 2017), which can lead to misinterpretation of model outputs. For policy-oriented models, this risks informing bad decisions and thus potentially creating distributional injustice, whereas for theoretically-oriented models it can affect understanding of processes in ways that may compromise recognitional or procedural justice.
Inputs and parameterization. Input data can be biased because data reflect historical inequities (Jacobs & Wallach 2021); it is possible that insufficient data exist for a socio-cultural group (an issue of recognitional injustice), either due to the size of the group or inadequate data collection procedures (Rajkomar et al. 2018). Data collection procedures may also overlook certain processes, for instance if only male members of a community are interviewed. Bias can also arise in model outputs if input data are applied outside of their original domain (Murray et al. 2017). Beyond data themselves, the variables or metrics selected as model inputs can inadvertently discriminate against particular groups. For example, a machine learning algorithm trained to optimize health care costs as a proxy for health led to systemic bias against Black patients (Obermeyer et al. 2019), which was found to be due to the choice of this proxy measure.
Processes. As discussed above under the theme of Abstraction, the rich process-based representation inherent to agent-based models can give rise to equity issues. A similar issue has received substantial scrutiny within the machine learning community through work on algorithmic fairness (Mehrabi et al. 2019; Noble 2018; O’Neil 2016), which acknowledges that the optimal solution with respect to one metric may not be the most fair (Corbett-Davies et al. 2017). Distinct risks also exist for ABM, which frequently aims at more nuanced representations of human behavior. If the behaviors or preferences assumed for a specific social group are inaccurate (i.e., the model inadequately represents behavioral heterogeneity), model outputs and any subsequent recommendations can be biased against that group (Beck et al. 2015).
Outputs. Averaging or summarizing across a population can mask distributional injustices.
Conceptual Framework
To reduce these risks and facilitate wider uptake of equity considerations within ABM research, there is a need to identify actionable strategies for equitable ABM. Here, we incorporate the elements of equity and their interactions with ABM to establish a framework for the equity-ABM interface (Figure 1). The framework intends to provide a structure and language for future inquiry into equity in ABM, but it is not a model or theory that seeks to predict or explain equity outcomes in any particular context (Schlager 2019). The framework is grounded by the following premises:
- Agent-based models are nested within a broader scientific and societal context (grey squares, Figure 1).
- The modeler, in engagement with stakeholders, acts as a filter and a lens for translating knowledge between the target system and the model (white boxes and arrows, Figure 1).
- Equity outcomes can be assessed at the science-society interface as well as within the model (questions in grey squares, Figure 1).
- Advancing equity through ABM will involve (1) engaging stakeholders, (2) acknowledging positionality and bias, and (3) assessing equity in agent-based models (colored annotations, Figure 1).
The first premise introduces two nested meta levels: the agent-based model and the science-society interface. This underscores that agent-based models are not isolated, technical objects. For instance, all agent-based models begin with a purpose (Edmonds 2017; Grimm & Railsback 2013), which generally relates to a topic of interest to science and/or society. Prevailing societal narratives can influence scientific priorities and funding, thereby motivating select model purposes. Thus, even before a model has been designed or built, it cannot be separated from the broader scientific and socio-political environment in which it operates.
The second premise introduces the actors and components within ABM, positioning the modeler as a central, powerful actor and thus as a primary locus for action on equity. The modeler is an integrator who, in an iterative fashion, formulates the model’s purpose, translates it into a model-based format, filters and interprets the model outputs, and communicates these to society (Diallo et al. 2019; Schmolke et al. 2010). Because the modeler is not an impartial or omniscient observer, bias can enter through these processes. Using an analogy of the modeler as a filter and a lens, we conceptualize this bias in two ways: through what the modeler sees and allows to inform the model, i.e., the ‘filter’ that admits or blocks knowledge; and how the modeler understands the world, i.e., the ‘lens’ that can morph knowledge (Figure 1). Engaging stakeholders can reshape the modeler’s effective filter and lens, but never eliminate them. This is for two reasons: (1) the modeler is generally responsible for translating stakeholder knowledge to model code and vice versa, even in highly participatory research (Voinov & Bousquet 2010); and (2) stakeholders also have their own motivations and are an integral part of the target system, meaning they also have biased perceptions (i.e., their own filters and lenses).
The third premise describes how the actors and components in ABM operate within a landscape of equity outcomes. The equity outcomes, which are measured across the three dimensions of justice, exist at the two principal meta levels. At the science-society interface, the subjects of equity comprise the people in the target system, other stakeholders, and the modeler. This is where “real” mechanisms and outcomes of (in)equity exist, for instance in the target system, between modelers and stakeholders, and between stakeholders. Within the model itself, the subjects of equity are the simulated agents. Equity is measured across the dimensions of agent heterogeneity. And (in)equity is produced by the initial conditions and simulated processes.
The final premise introduces three “action pathways”—engage stakeholders, acknowledge positionality, and assess equity—that represent promising arenas for advancing equity in ABM. The action pathways each relate to specific components and actors within ABM (i.e., the boxes and arrows in Figure 1) and, as we describe below, can affect equity outcomes at the two meta levels. The pathways are not necessarily all-encompassing and will likely be refined through further research and learning on this subject, but provide an important grounding for such future research. As we detail below, there is considerable existing experience in engaging with these pathways, thus setting a precedent for their integration into good modeling practice.
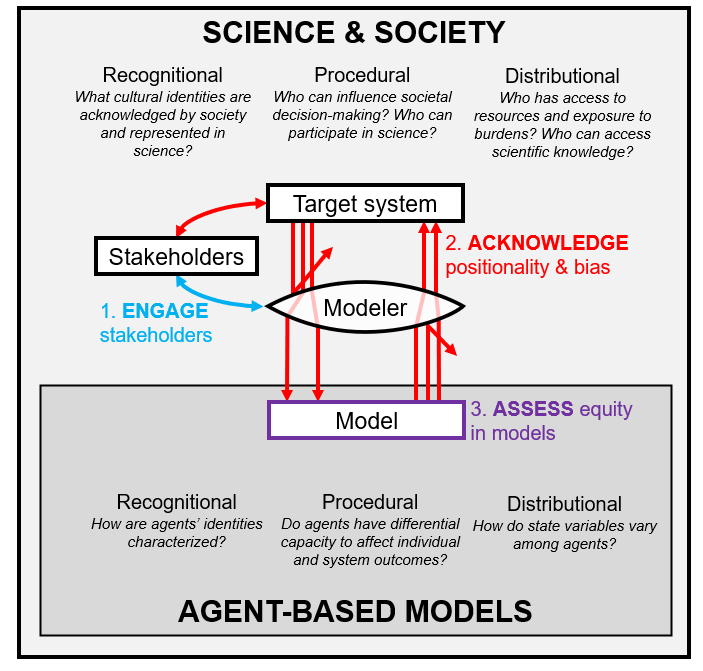
Three Pathways for Integrating Equity Considerations into ABM
In this section, we assess the current state of practice at each pathway, provide suggestions and guidance, and identify a set of preliminary best practice principles for integrating equity considerations into ABM. Our descriptions of each action pathway take distinct forms, due to their differing levels of uptake within the ABM literature.
Although we present the pathways here as conceptually distinct, we emphasize that they are in practice neither mutually exclusive nor independent. For example, engaging stakeholders in participatory modeling requires critical reflection on stakeholder positionality and bias. Thus, it is likely appropriate to bundle approaches from the different action pathways. In addition, the action pathways are not prescriptive, and all may not always be appropriate. For example, since we consider equity only in relation to human experiences, agent-based models of non-human entities (e.g., animals or plants) cannot in themselves be used to assess equity.
Pathway 1: Engage stakeholders
There exists a rich body of research on stakeholder engagement, participatory modeling, and co-production of knowledge (Barreteau et al. 2003; Lemos et al. 2018; Reed 2008; Voinov et al. 2016). Researchers have accordingly developed a range of guidelines for effective stakeholder engagement. Critical insights include the importance of involving stakeholders early within the project (Reed 2008; C. Steger, Klein, et al. 2021) and taking time to develop trust (modeler-stakeholder, stakeholder-stakeholder, and stakeholder-model) (Voinov & Bousquet 2010). Attention should be paid to stakeholders’ motivations for involvement (Voinov et al. 2016), the diversity of stakeholder interests and identities (C. Steger, Hirsch, et al. 2021), who is selected as a leader (Hämäläinen et al. 2020), and potential power dynamics between stakeholders (Barnaud & van Paassen 2013). Stakeholder engagement takes significant time and effort (both for the stakeholders and the modeler) (Voinov et al. 2018), and modeling objectives should be accordingly adjusted to include participatory modeling as a process for shared learning (Reed 2008).
Stakeholder engagement is likely most relevant for applied models designed to represent specific case study contexts. In theoretical or highly abstracted models, it might be difficult to identify who the stakeholders are, or any potential stakeholders may be considered too far downstream from the modeling process to be meaningfully engaged. However, simple models can be highly useful tools for illustrating ideas and theories (Edmonds 2017) and could be developed collaboratively with stakeholders to facilitate shared learning. The process is therefore not one-size-fits-all and clearly must be tailored to individual contexts. Yet, neither is there only one appropriate approach for a given context, and there may be a range of possible levels of stakeholder engagement (Pretty 1995).
Engaging stakeholders in ABM can improve equity through at least two mechanisms. The first is by mitigating problems of recognitional justice in the model representation. Stakeholders each bring their own backgrounds and perspectives on the research problem (i.e., positionalities) that can contribute to reducing the effects of the modeler’s individual bias. Including an intentional mix of diverse positionalities thus reshapes the filter and lens through which knowledge is projected into the model (Figure 1) and has the potential to profoundly affect the ABM process and outcomes (C. Steger, Klein, et al. 2021). For example, stakeholders may identify actors omitted from the model or offer alternative descriptions for model processes. Stakeholder engagement at early stages can affect the problem definition and framing (C. Steger, Klein, et al. 2021; Voinov & Bousquet 2010) and thereby the scope of the model (for instance, if some elements should not be modeled due to problems with positivist interpretations of Indigenous knowledge Smith 2013).
Second, engaging stakeholders can improve equity in the distribution of access to science and knowledge, thus advancing procedural and distributional justice at the science-society interface. Particularly when stakeholders are collaboratively involved in a process of co-production, models can act as boundary objects to facilitate knowledge generation and shared understanding (Lemos et al. 2018; Voinov et al. 2016). This two-way learning process increases the legitimacy of models and drives better decisions (Reed 2008). For example, modeling projects with frequent exchanges between modelers and decision makers have shown greater potential to influence policy making (Weiskopf et al. 2022; Will et al. 2020). Integrating collaboration into scientific funding can generate more effective and usable research outcomes (Arnott et al. 2020). Modelers can also improve access to scientific knowledge by publicly posting their code (e.g., at CoMSES.net), using open-source software (e.g., Netlogo, Python, R), and making research articles open access. Teachers can integrate ABM modules into their courses to diversify their teaching methods and thereby promote inclusivity (Murphy et al. 2020). The contributions to equity through this mechanism are thus threefold: more equitable knowledge production, equity in access to knowledge, and increased propensity for ABM to inform equitable real-world decisions.
We caution here that engagement with stakeholders is a complex process, and when done uncritically or without careful attention to power dynamics, can have adverse or unintended impacts on equity considerations. For example, Steger et al. (2022) describe how their collaborative ABM process only involved participants from groups with historic rights to a communal grassland. These individuals resisted including any variables in the model that might negatively impact their image as good managers of the conservation area (e.g., the presence of “illegal” grass harvesters or grazing). The authors observed a trade-off between building trust and communication with a particular group, and thus improving that group’s receptivity to collaborating with scientists, and potentially obscuring points of inequality between that group and their broader social context. Thus, we encourage modelers to pursue a second pathway towards equity through open acknowledgement of positionality and bias, so that the influences of both the modeling team and stakeholders are made visible.
Pathway 2: Acknowledge positionality and bias
“If a designer does not make his biases explicit, he risks imposing them unconsciously” (Barnaud & van Paassen 2013 p. 4). Modeling invariably leverages tacit knowledge, which reflects a modeler’s worldview (and stakeholders’ worldviews) and therefore contains bias. Acknowledging positionality and bias is thus important for rigorous and equitable model development. This can be achieved through reflexivity, i.e., disciplined self-reflection throughout the research process to identify the effects of subjectivity (Finlay 1998). Reflexivity already features prominently in qualitative research through ‘positionality statements’ (Holmes 2020; Milner 2018) as well as through acknowledging ‘privilege’ in antiracist and feminist methodologies (McIntosh 2020). Yet, acknowledgement of positionality and bias is surprisingly absent in ABM research (and modeling studies in general). To make progress on this, we categorize sources of bias throughout the modeling process and provide a set of reflective questions for assisting modeler and stakeholder reflexivity (Table 1). Reflexivity is a necessary precursor to improved equity outcomes in both the model and in science and society, as we must make internal biases and gaps in our understanding visible before we can work to reduce or overcome them.
We conceptualize these reflections as pertaining to five distinct themes: positionality, problem framing, model inputs, quantification, and model interpretation. The first theme, ‘positionality,’ is about the modeler’s and stakeholders’ identities, how these may influence their experience and perception of the world, and how they relate to the research context (Figure 2; Milner 2018). Here, ‘identity’ constitutes a combination of social and cultural factors, such as race, gender, political beliefs, age, and experience (Figure 2 A). Different facets of a person’s identity may position them as an insider or outsider with respect to the research context (Holmes 2020), together forming a psychological ‘distance’ from the research phenomenon. Psychological distance has potentially divergent implications for bias; as an insider to the research context, one has a lived familiarity and deeper a priori understanding of the context, potentially allowing for a less-biased perspective. However, insiders may be unable to sufficiently detach themselves from the culture to study it without bias (Kusow 2003). Positionality therefore does not seek to elevate a particular identity above another, but to describe how identity shapes and colors perception. Modeler and stakeholder positionality thus provides an overarching understanding from which to interpret bias within the following themes.
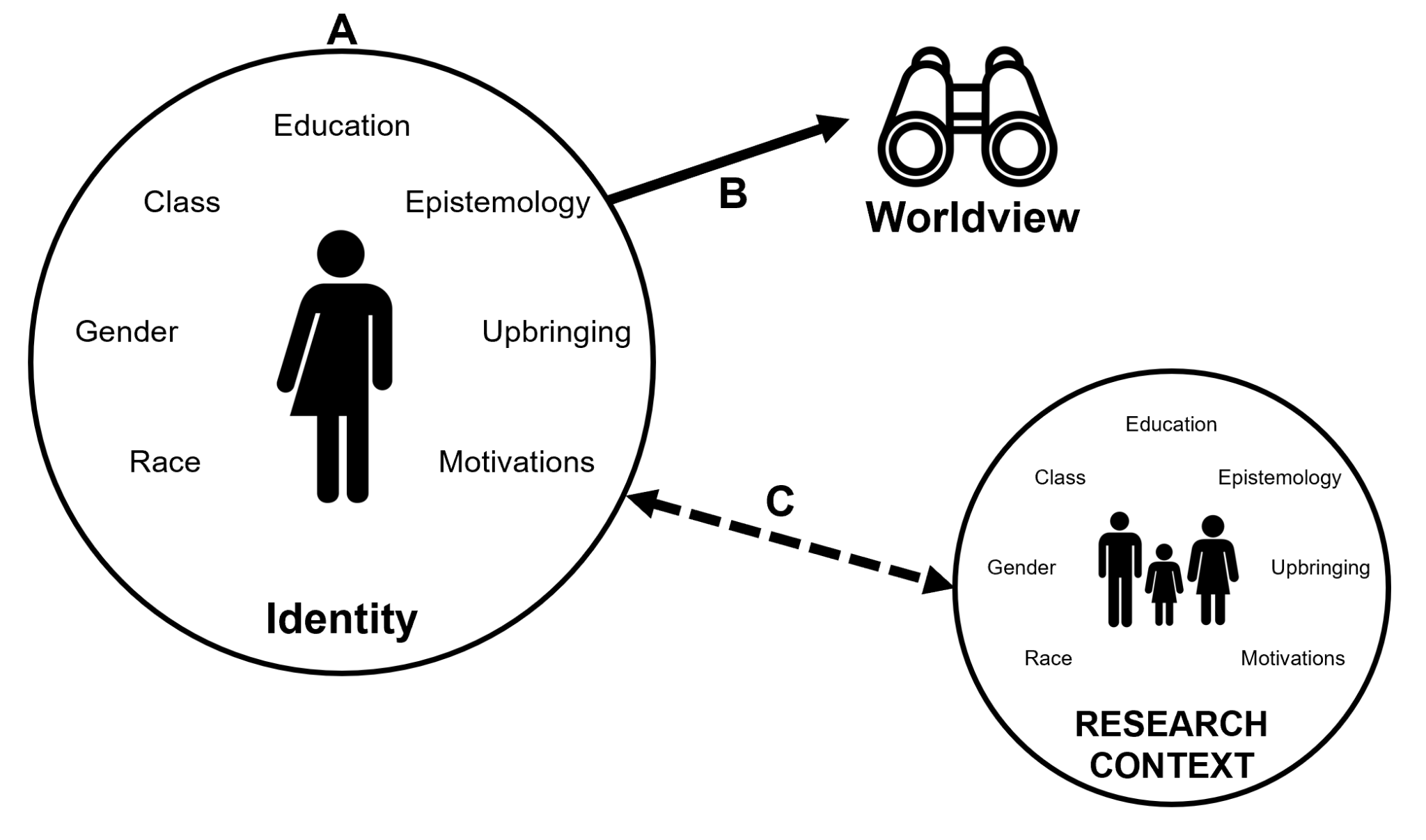
The remaining four themes each correspond to different components of the modeling process, thereby underscoring the importance of reflexivity at all stages of agent-based model development and application. First, ‘framing’ describes the narratives that motivate the research and what problems and solutions these narratives include, exclude, and prioritize (Leach et al. 2010). This is important because “the very act of specifying a problem is a reflection of a perception of reality” (Diallo et al. 2019 p. 16) and there are multiple, valid perceptions of reality. For example, declining rhinoceros populations in Eastern Africa can be framed as a problem of illegal poaching, prompting stricter policing within conservation zones (Büscher & Ramutsindela 2015). However, an alternative framing could describe conservation zones as displacing peasant livelihoods and thereby forcing them to engage in nefarious activities to support their families, prompting enhanced support for local populations to curb rhinoceros population declines. Considering the impact of framing is highly important, as each framing would likely motivate a distinct model design and potentially lead to diverging conclusions. With respect to equity, framing can implicitly place blame or responsibility on different actors (e.g., poachers versus conservation managers), some of whom may be historically marginalized or have less political power to defend their position.
The second of these themes, ‘inputs,’ is about being reflexive about the information that is fed into the model. This requires scrutinizing what information is deemed relevant to include in the simulation (Polhill et al. 2021), particularly if it is time- or context-sensitive (Diallo et al. 2019), as well as whether alternative information sources were intentionally excluded (and, if so, why they were excluded). For the information that is used as input to the model (e.g., for calibration or validation), such data may contain embedded historical inequities (Mehrabi et al. 2019); biases can enter data through many pathways (23 in Mehrabi et al. 2019) and can introduce distinct risks to downstream modeling processes. Modelers thus need to be reflexive about how their use of data may lead to bias.
Third, ‘quantification’ encompasses bias associated with translating knowledge into a model format. The model may contain variables that are latent constructs, the operationalization of which may mismatch theoretical understandings of phenomena (Jacobs & Wallach 2021). Additionally, there may be multiple interpretations or understandings of a process. Subjectivity in process quantification is a well-recognized issue in ABM, where different modelers reach different conclusions given the same set of data, or independent implementations from a single model description lead to different results (Zhang & Robinson 2021). This goes beyond the technical challenge of transparency and good documentation, and arises due to different worldviews and knowledge structures that tacitly filter such information. Subjective abstraction is therefore inevitable regardless of how reflexive a researcher is, so this process should seek to understand and acknowledge the model as a non-neutral abstraction (Barnaud & van Paassen 2013; Voinov et al. 2014), rather than to neutralize it.
Fourth and finally, ‘model interpretation’ closes the loop by examining how modeler and stakeholder positionality might affect the interpretation and communication of model outputs. As subject matter and contextual experts, both modelers and stakeholders likely enter the modeling project with preconceived understandings of the target system, or even preconceived opinions about what they want the model to show. Such preconceptions can color model interpretations. Care must also be taken to ensure that interpretation does not deviate from the model’s original purpose (Edmonds 2017) and is cognizant of any limitations revealed by the above reflection categories. This final theme also encourages reflection on communication strategies, which should strive to be accessible and interpretable to stakeholders beyond the modeling community.
Table 1 enumerates a set of questions that can assist modelers in the reflexive process of understanding, critiquing, mitigating, and acknowledging sources of potential bias across the five themes. The reflexive questions require introspection to consider how the given aspect of the modeling process is subjective and might lead to inequity. Although this reflection does not necessitate changes to the modeling focus or model design, acknowledging positionality and bias should be seen as an iterative and ongoing component of rigorous and ethical modeling practice that, over time, seeks to mitigate potential inequities and therefore influences the direction of model development. Thus, it is important that such efforts do not become perfunctory, post-hoc considerations or a tool to redistribute blame; recognition should be understood as a means for opening doors to deeper engagement with inequity, rather than an end in itself.
To operationalize this reflexivity, we suggest that modelers develop a “positionality and bias document” that contains written reflection on the prompts in Table 1. The document may be narrative or simply a list of reflections on the relevant prompts from the perspective of the principal modeler and/or the modeling team. In modeling research involving stakeholders, it may be useful to also develop (collaboratively with stakeholders) a statement of stakeholder positionality and bias for the stages of the modeling process at which they are involved. We suggest that the document be consulted and updated as necessary throughout the modeling process.
The document is intended primarily as a tool for internal use within the modeling team, for instance to guide self-reflection or group discussions regarding assumptions, processes, and biases. As such, the document need not be shared or publicized. However, for additional transparency, the modeler can choose to include the document, either in its entirety or a subset, with any written communication of the model and its outputs (e.g., as an appendix to an academic research article Asiedu et al. 2021). In this case, the document complements existing ABM documentation and communication standards, such as the TRACE framework and the ODD protocol (Grimm et al. 2014, 2020). Appendix 1 provides several example positionality and bias documents that use the reflection prompts from Table 1. The Online Supplement provides a Microsoft Word document as a template for researchers.
Theme | Reflection prompts |
1. Positionality | What are the backgrounds and identities of the modeler(s)
and stakeholders? (e.g., race, ethnicity, class, gender, education,
upbringing, political beliefs) How might these identities have influenced how the modeler(s) and stakeholders approach research? (e.g., worldview, epistemology, objectives) How do these identities relate to the participants and/or context of the research? (e.g., in what ways are the modelers/stakeholders insiders or outsiders? Are there power imbalances?) |
2. Framing | What narratives underlie the formulation of the problem or research questions? What kinds of solutions do these narratives invite? What are the principal entities and actors in these narratives? Which groups might be (dis)advantaged by this framing? Who has decided about this framing? What theories and/or relationships is the conceptual model predicated on? If relevant, are there alternative understandings? |
3. Inputs | Who has decided about which information is relevant for the simulation? If information was excluded, why? How could data (e.g., for model inputs, calibration, or validation) represent or mask historical patterns or drivers of inequity? |
4. Quantification | What model variables are subjective or latent constructs? What alternative interpretations of these exist? Could the inclusion/exclusion of model processes misrepresent or lead to bias against certain groups? |
5. Interpretation | Who interpreted the model outputs? How could pre-conceived understandings or objectives have biased model interpretations or conclusions? How does the interpretation relate to the original model purpose? Considering any limitations revealed in the above reflections, could there be alternative interpretations of model outputs? How are model outputs communicated, and to whom? Can key stakeholders access and understand the modeling results? |
Pathway 3: Assess equity in agent-based models
Overview
The final action pathway is about how the agent-based model itself engages with equity elements and outcomes. Agent-based models can be used to conceptualize the causes of inequities, better understand their implications, and investigate mechanisms toward overcoming them (Campbell et al. 2015). As models are nested within science and society, the ultimate goal here is to use equity-oriented model assessments (i.e., the equity representation within the model) to advance equity outcomes at the science-society interface, for instance by generating knowledge to inform equitable decisions in the target system.
For this action pathway, we review previous agent-based model applications and synthesize a typology of approaches for modeling equity across the three dimensions of justice (Table 3). Rather than providing prescriptive instructions, the typology aims to facilitate future equity-oriented ABM applications by outlining the distinct approaches that have been taken and the kinds of equity-oriented questions that can be asked using agent-based models. Nevertheless, the review and typology reveal several overarching considerations, which we distill as recommendations for best practice in Table 4.
We conducted a keyword search in Scopus using the terms ("agent-based" OR "agent based") AND (model*) AND (*equit* OR disparit* OR *justice* OR *fairness*). The search returned 344 results (on 31st March 2021). Within these articles, we restricted our focus to those that apply an agent-based model and indicate clearly within the abstract some link to equity in the model’s design or application. We positioned each valid article (\(n=141\)) within our definition of equity, including the dimension(s) of justice assessed, the objects and subjects of equity, and the fairness principle. Filtering and coding were completed by the lead author (i.e., not appraised by a second coder). As such, we did not intend this as a formal literature review, but as a scoping search to reveal general trends.
The articles retained within our sample applied agent-based models in a diverse array of systems (Appendix 2), ranging from abstracted, historical analyses of socio-cultural evolution to detailed, forward-looking assessments of policies for climate change mitigation. Dominant framings of (un)fairness included environmental justice, economic inequality, and disparities in health outcomes and access to urban services. Although rarely explicitly stated, these framings most frequently conceptualized fairness as either applying preference to those with the greatest need (i.e., a needs-based fairness principle) or achieving equality in outcomes between groups (i.e., egalitarianism). The modeled outcomes, i.e., equity of what, generally represented measures of social goods (e.g., wealth, resources) or human capabilities (e.g., access to employment, membership in social groups). The modeled dimensions of agent heterogeneity, i.e., equity between whom, included both continuous measures of variability within a type (e.g., wealth, resources) and discrete, categorical identities (e.g., race, stakeholder type). Descriptive statistics and the full list of citations are included in Appendix 2.
Recognitional justice
Recognitional justice describes the identities and perspectives acknowledged and valued by various components of society (Fraser 1995). Agent-based models can engage with recognitional justice through their representation of heterogeneity in agent attributes (i.e., identities) as well as objectives or decision-making procedures (i.e., perspectives). Although many agent-based models represent actor heterogeneity, only four articles within our sample (3% of total) explicitly engaged with recognitional justice (Table 3). All of these articles assessed the implications of broadening the modeling scope to include vulnerable group identities, such as the behavior of slum residents (Adiga et al. 2018) or the behavior of Black men who have sex with men (Goodreau et al. 2017). For example, Adiga et al. (2018) show that explicitly representing the household sizes and network structures of slum dwellers in Delhi, India, is necessary to achieve more equitable public health outcomes. When these characteristics were not represented, their model underestimated the risk to slum dwellers.
These examples leverage ABM as a virtual laboratory for testing and demonstrating the equity impacts of different recognitional assumptions. Because this in principle requires no methodological extension beyond representing agent heterogeneity (either in innate capabilities, behavioral preferences, or how they are treated differently by system-level structures), future ABM research could relatively easily examine questions of recognitional justice. However, reflexivity and stakeholder engagement will be critical to such efforts, in order to reduce the risk of mis-characterizing components of socio-cultural identity.
Procedural justice
Procedural justice describes the fairness in people’s inclusion in and ability to influence decision-making (Tyler 2000). Agent-based models are particularly well-poised to incorporate procedural injustices because they can explicitly represent nuanced decision-making processes (e.g., diverse behavioral theories and behavioral heterogeneity within a specific theory). Accordingly, models focusing on procedural justice were moderately common in our search results, occurring in 41 (29%) of the retained articles.
Within these articles, we observed five principal approaches for dealing with procedural justice (Table 3). The most common of these was to represent heterogeneity and/or fairness in individual-level decision-making processes. For instance, several models focusing on cultural evolution specified agents to have heterogeneous or dynamic preferences with respect to individual satisfaction and system-wide resource distributions (Motchoulski 2021) (Table 3). Although these dynamics are solely at the level of an individual agent, they relate to procedural justice because certain agents may have higher or lower capacity to influence system-level dynamics through their own decisions.
The second-most common approach takes the procedural focus to a slightly larger scale: group interactions. Here, agents interact with each other more directly, for instance by sharing or competing over communal resources (Klein et al. 2017; Schank et al. 2015) (Table 3). Under this approach, procedural (in)justice arises from distributed interactions, rather than a top-down decision-making process. Power dynamics play an important role in these kinds of interactions and can be surprisingly simple to represent. For example, Mahault et al. (2017) model resource transfers within a population of agents and implement a version of the ‘Matthew effect,’ whereby the power to acquire wealth grows super-linearly with accumulated wealth. Beyond the possession of physical or material resources, other forms of capital, such as the ability to influence others’ decisions (Dávid-Barrett & Dunbar 2014), can confer power and thereby represent procedural justice.
A more complicated form of decision-making, identified in only four articles in our sample, is governance. Governance is a form of collective decision making in which people jointly collaborate on system-level decisions. This is different from the above approach (i.e., group interactions) in that the decisions are centralized, rather than distributed. Agents may have diverging preferences, making it difficult to come to an overarching decision. Deliberation processes can be modeled and help to achieve better consensus and governance outcomes (Choi & Robertson 2014), but some cases may require a form of adjudication (an authoritative process for reaching a common decision; Table 3). Different adjudicative mechanisms can be employed and modeled, such as a plurality vote (choose the most popular option; Motchoulski 2021) or stricter majority or supermajority requirements (50% or more in consensus; Choi & Robertson 2014). These mechanisms may disadvantage minority groups, due to their smaller cumulative voting power.
The fourth and fifth approaches represent procedural justice through top-down processes, rather than bottom-up decisions of individual agents. The fourth approach models decisions made by a single system-level agent, which may systematically favor or disadvantage certain population groups, for example by preferentially locating environmental dis-amenities in communities with low privilege (Eckerd et al. 2017) or allocating work to the best performing workers (Sobkowicz 2016). Due to the focus on a single decision-maker, this approach is less unique to agent-based models and is conceptually similar to objective functions in optimization problems. Yet, agent-based models can be used to examine both the distributional effects of top-down procedural inequities (see the following subsection) as well as explore bottom-up responses to top-down management decisions (Farhadi et al. 2016) (Table 3).
The fifth approach is more methodologically oriented and focuses on procedural injustices introduced by the simulation design itself. In particular, the order in which agent processes are executed can affect both agent-level and system-level outcomes (Page 1997) (Table 3). Computational approaches such as multithreading may inadvertently lead some agents to execute early within each time step (Welch & Ekwaro-Osire 2010), thus creating a system representation that may include unfairness but not necessarily accurately represent the system. Although these features pose a risk when not adequately considered, they also introduce an opportunity to represent mechanisms of power and privilege through simulation design.
Distributional justice
Distributional justice describes the fairness in the allocation of goods in society (Rawls 2001). Any model in which agents experience heterogeneous state variables therefore implicitly deals with distributional justice. This is a feature of almost all agent-based models, and was the most prominent dimension of justice within our sample, being explicitly analyzed in 117 of the articles (83%). We structure our discussion of these articles according to three principal modeling objectives: the conditions leading to distributional injustice, the distributional injustice itself, and its implications.
There are many reasons to explore the conditions leading to distributional injustice. For example, understanding the set(s) of equity-promoting or equity-degrading conditions can be useful for identifying equitable policies or interventions among a large set of candidates (de Wildt et al. 2020). Here, tools such as ‘scenario discovery’ have been used to find combinations of input parameters leading to an outcome of interest (de Wildt et al. 2020). Similarly, agent-based models have been used to identify the behavioral conditions that give rise to desirable or undesirable system-level outcomes, such as sustainable resource management (Schindler 2012) or social stratification (Dávid-Barrett & Dunbar 2014). In some cases, the set of hypothesized mechanisms may be insufficient to describe observed levels of distributional inequality (Goodreau et al. 2017), motivating consideration of a wider range of factors.
The approach for analyzing distributional justice itself depends primarily on the type of agent heterogeneity being modeled. In the simplest case of a single agent type, distributional justice is assessed through variability within the type, for example a Lorenz curve or Gini index to represent wealth inequality (Mahault et al. 2017). When heterogeneity exists in other agent characteristics (i.e., there is a diversity of agent types Page (2010)), distributional analyses generally relate an outcome measure to an agent attribute. Here, if agent attributes are discrete, a common approach is to compute disparities between the categorical classes, such as two racial groups (Orr et al. 2014). If, instead, the relevant agent attribute is continuous, it is possible to assess distributional outcomes across the continuous attribute (Williams et al. 2020), but studies often discretize it through stratification (e.g., high-income and low-income Auchincloss et al. (2011)). In such cases, bias can be introduced through the adopted boundaries between agent groups (Smart 2019).
Several articles within our sample focused on the implications of distributional inequality. Two of these were based on economic disparities, and specifically their potential to lead to conflict and instability within the financial system (Cardaci 2018; Kustov 2017). More generally, due to the iterative nature of agent-based models, distributional injustice in one time step can affect subsequent model processes, in turn affecting subsequent distributional patterns. Thus, many other applications implicitly model the implications of distributional injustice over time.
One final consideration relevant to distributional justice is how it relates to other outcome measures. Studies frequently reported tradeoffs between equity and economic outcomes (Henry & Brugger 2017; Malik et al. 2015; Ponsiglione et al. 2015), equity and environmental outcomes (Filatova et al. 2011), or between stakeholder objectives (Farhadi et al. 2016). Only one study in our sample explicitly reported win-win outcomes across equity and other dimensions (Bell et al. 2016). Thus, agent-based models are useful tools for characterizing such multidimensional relationships and can be combined with other methods, such as multi-objective optimization, to identify conditions that minimize tradeoffs across multiple outcomes (Farhadi et al. 2016).
Dimension of justice | Modeling or assessment approach | Example |
Recognitional (\(n=4\)) | Assess the implications of representing vulnerable group characteristics (\(n=4\)) | Adiga et al. (2018) model the spread of influenza in Delhi, India. They contrast two different network configurations: one that treats slum and non-slum regions the same, and one that represents slum-specific demographics and behaviors. They find that ignoring slum attributes can lead to a 30-55% overestimation in vaccination efficacy, thereby demonstrating the importance of representing vulnerable group characteristics and behaviors in both models and public health policy. |
Procedural (\(n=41\)) | Model heterogeneity in individual decision-making processes (\(n=20\)) | Schank et al. (2015) model the evolutionary origins of cooperation within societies. In their model, agents play the “dictator game,” where one agent decides how to divide a resource with another anonymous agent. They examine the conditions under which individual preferences toward cooperation, which are observed empirically but conflict with some behavioral theories, are selected for in a group-based society. |
Model group interactions (\(n=13\)) | Mahault et al. (2017) model different mechanisms for regulating power imbalances in an artificial society. When no constraints are imposed on wealth transfer between agents, the agent population invariably becomes polarized, whereby the agents with more opportunity accumulate all of the resources. As further constraints are imposed on wealth transfer, agents’ ‘frustration,’ a measure of the degree to which they act to reduce unsatisfied aspirations, mediates the effects of power imbalances within the society. | |
Model system-level decision-making processes (\(n=5\)) | Eckerd et al. (2017) model the emergence of environmental injustice in a community through the siting decisions of environmental amenities and dis-amenities. They vary the extent to which top-down decisions about amenity location are driven by cost versus community privilege (e.g., dis-amenities preferentially selecting locations with low community privilege). They find that amenities exert important influences on environmental injustice, meaning that a pure focus on the politics of dis-amenity siting is insufficient. | |
Model governance mechanisms (\(n=4\)) | Motchoulski (2021) examines disagreements between agents’ conceptions of distributive justice and the effectiveness of different ‘adjudicative’ governance mechanisms (an authoritative process for reaching a common decision, e.g., through a third party) in resolving these disagreements. In their model, agents have heterogeneous and dynamic preferences toward individual satisfaction (i.e., self-interest regarding how many resources they receive) and distributive justice (i.e., the distribution of resources throughout society). Agents respond to the outcomes of adjudicative mechanisms by adjusting the relative weights placed on self-interest and justice. | |
Assess the effects of simulation methodology (\(n=2\)) | Page (1997) examines the importance of the simulation updating procedure in affecting agent outcomes. They compare emergent system dynamics under two conditions: one where agent states are updated in a random order and one where the updating order is determined by the agents’ relative utility increases (a.k.a. “incentive based asynchronous updating”). The two different conditions contribute to vastly different model dynamics. | |
Distributional (\(n=117\)) | Stratify outcomes by group identity (\(n=60\)) | Smart (2019) models ‘colorism,’ the prejudice toward allocating privilege to lighter skin color, in the context of policing in the US criminal justice system. Their model contains three citizen groups: lights, mediums, and darks. They found that aggressive policies to counteract colorism yielded counterintuitive distributional effects between groups. Specifically, agents in the middle of the skin color spectrum experienced higher rates of incarceration. The results demonstrate the importance of broadening the description of colorism to include those in the middle of the color spectrum. |
Assess the conditions leading to inequality (\(n=40\)) | de Wildt et al. (2020) examine the conditions that give rise to inequality through conflicts in agents’ capabilities (a measure of the freedom to achieve wellbeing) in the deployment of decentralized energy systems. Their model operationalizes the capability approach of Nussbaum & Sen (1993) and their analysis examines a broad range of energy system and geographic scenarios. They find distinct classes of capability conflicts: sometimes conflicts are inherent to organizational characteristics of energy systems; sometimes conflicts are specific to a type of population (e.g., affluent); and sometimes conflicts occur between population groups. | |
Assess population-level distributional effects (\(n=17\)) | Williams et al. (2020) model the effects of drought on food security in a population of heterogeneous farming households. They examine how the Gini index (representing the distribution of food security throughout the population) evolves in the wake of the drought, and the effects of several interventions on this measure of inequality over time. | |
Assess the implications of inequality (\(n=3\)) | Cardaci (2018) models the implications of inequality for the 2007-08 financial crisis in the United States. Their model represents how inequality in income can lead to expenditure cascades that result in accumulation of household debt, increasing the fragility of the economy and paving the way for a financial collapse. | |
Applying the pathways: Guidelines for best practice
Drawing from the above analyses, we identified several principles that aim to guide effective action within each pathway (Table 4). For engaging stakeholders, the list summarizes what we perceive as the most pertinent principles for mitigating inequity. More detailed discussions on principles for effective stakeholder engagement exist elsewhere (C. Steger, Klein, et al. 2021; Voinov et al. 2016). Given the scoping nature of this paper, the principles in Table 4 are not intended as exhaustive propositions. Rather, they provide an initial set of suggestions that can guide future research and be refined and expanded.
Action pathway | Suggestions for best practice |
1: When engaging stakeholders… | a. Involve stakeholders early to develop trust and allow them to influence the research b. Adjust modeling expectations toward a process for shared learning c. Consider stakeholder identities to adequately represent diversity (e.g., in personal characteristics, role in the target system, objectives) d. Consider potential power imbalances and the risks these may introduce |
2: When acknowledging positionality and bias… | a. Use the questions in Table 1 to guide reflections b. Reflection should be iterative and on-going, rather than post-hoc c. Consider stakeholder positionality d. Develop a positionality and bias document. Primarily use this document to guide internal reflections. For additional transparency, publish (components of) the document in academic articles e. Ensure that reflections do not become perfunctory |
3: When assessing equity in agent-based models… | a. Make clear any ethical principles implicit in the model’s purpose or interpretation b. Extend heterogeneity beyond agent attributes to also represent unequal treatment in model processes c. Consider the risks of mis-characterizing or only partially characterizing actor heterogeneity d. Consider how modeling and analysis choices may generate or mask injustice (e.g., agent execution order, stratification thresholds, averaging) e. Consider the multiple scales at which procedural injustice may arise (individual decision-making; interactions and power dynamics; system processes and governance) f. Consider how equity relates to other outcomes of interest (e.g., trade-off / synergy) g. Equity can be both an input to and an output of the model analysis |
Discussion
Mainstreaming modeler and stakeholder reflexivity
A core conceptual advance in our framework and pathways is the centrality of modeler reflexivity, which is rarely practiced in ABM research. The modeler assumes a powerful role in the modeling process and—irrespective of their particular socio-cultural identity—they leverage tacit knowledge that can contain bias and preconceptions. Reflexivity is therefore important to mitigate the risk of such biases contributing to inequity.
With this understanding, we (the authors) acknowledge that many of the arguments made in this paper are shaped by our own positionalities. We believe that there is a need to understand inequality in society and how structures and policies affect vulnerable populations. As many of us identify with historically privileged identities (e.g., white, male, affluent), we see an ethical obligation to reflect on how such mechanisms can result in discrimination. That being said, we have been careful to not uncritically elevate the perspectives of disadvantaged people above those with greater privilege; all people have prejudices that shape their decisions. The reflection questions (Table 1) aim to initiate the reflexive process and elucidate how the prejudices of people involved in the modeling process may affect model inputs, structures, outputs, interpretations, and applications.
We believe that the mainstreaming of reflexivity in research that makes use of ABM is possible for two reasons. First, agent-based modelers are already particularly self-critical and open to streamlining of methodology and practice. This has in large part led to the establishment of detailed reporting standards, such as the ODD protocol and TRACE documentation (Grimm et al. 2014, 2020; Müller et al. 2013). Such existing standards already promote some degree of reflexivity by encouraging modelers to reflect on and document their assumptions and model design decisions. Our suggestions complement this by encouraging reflection on how positionality may impinge on such decision-making.
Second, there is a societal demand for reflexivity. Recent events (e.g., in the United States, the Black Lives Matter movement and the rise of hate crimes against Asian Americans and Pacific Islanders due to COVID) have challenged institutional actors to reflect on their positionality, biases, and (hidden) forms of discrimination. Such a societal impetus also played a role in the mainstreaming of participatory modeling, which was in part due to the increasing complexity of modern decision problems that demanded integration of more diverse ways of knowing (Pretty 1995).
Learning from the experience of the ODD documentation’s uptake, which was facilitated both by follow-up publications providing revised guidance and the insistence of peer reviewers, we believe that academic peer reviewers could play a similarly important role in a diffusion of responsible use of reflexivity in ABM. Reviewers of project proposals and funding organizations may have the greatest leverage toward this end, as they can encourage the recommended proactive use of reflexivity at the stage of project design. For peer reviewers of academic journal articles, the late stage at which they comment on an article risks making reflexivity perfunctory. However, we believe that post-hoc reflection is better than no reflection. Positionality and bias statements may therefore need to initially enter as retrospective considerations in journal articles that inspire subsequent proactive efforts.
Future research is needed to test whether and how modeler reflexivity improves modeling outcomes. Our hope is that reflexivity can illuminate blind spots, thereby improving the quality of model representations and any inferences drawn from them. Stakeholder engagement has been demonstrated to achieve better decision outcomes and greater learning (Reed 2008), facilitating its uptake by scientists and funding organizations (Arnott et al. 2020). A similar future for reflexivity is possible. Research on this topic should also be attentive to how requirements for and benefits of reflexivity may vary for different modeling purposes and levels of empirical embeddedness.
Opportunities and considerations when modeling equity
The results of our keyword search demonstrate a considerable precedent for applying models to assess equity. This suggests that assessing equity is not limited by methodological constraints. We found several distinct approaches for assessing equity (Table 3), and we hope these approaches and the general recommendations (Table 4) can inspire and inform future research on this theme.
We observed a very uneven spread of model applications across the different dimensions of justice. In particular, ABM research could more deeply engage with issues of socio-cultural recognition, i.e., how society differentially treats different socio-cultural identities (e.g., race) and thereby contributes to disparities in outcomes. This research should be particularly attentive to the risk of ‘tokenizing’ heterogeneity, i.e., attempts to incorporate agent diversity may miss important dimensions of difference that can affect model and system outcomes. Our results also contained relatively few models dealing with cross-scale power dynamics or governance, which are important to many real-life contexts. Although this may partly reflect the terms used in our keyword search (e.g., see Bourceret et al. 2021), future research could nevertheless integrate such dynamics more deeply, as well as explore social thresholds and systemic shifts in power balances (Mathias et al. 2020; Schlüter et al. 2021).
When modeling equity, it may be necessary to modify other modeling practices. We have already stated that the action pathways should not operate in isolation, and assessing equity in a model may require deeper stakeholder engagement or reflexivity. There remain conceptual questions around whether incorporating equity in a model affects requirements for model validation, as well as the extent to which it is possible to quantitatively represent modeler positionality and bias in uncertainty or sensitivity analysis. To make progress on this, future research could use agent-based models to formalize and explore multiple understandings of reality (Leach et al. 2018; Lempert & Turner 2021; Wilson 2001), potentially building on concepts such as equifinality that are already common in modeling.
Pragmatic constraints and generalizability of our recommendations
As with any call for more rigorous modeling practice, our recommendations (Table 4) have the potential to add considerable burden to modelers operating with limited time, financial, and social resources. It is therefore important to consider the balance of benefits and costs of integrating equity considerations through any action pathway. We have emphasized that reflexivity provides value in itself, meaning equity can be improved without substantive changes to the model or the modeling process. Moreover, given that many agent-based models already represent actor heterogeneity, adopting an equity lens may simply require the researchers to be cognizant of any normative judgements attached to such heterogeneity (i.e., articulating the fairness principle), or perhaps preferentially maintaining an intentionally neutral stance. Additional effort is required to assess and mitigate inequities throughout the modeling process (e.g., by broadening the problem framing or process descriptions), but doing so early within the model design process is likely to yield the largest benefit to cost ratio.
Equity considerations may be more or less relevant in different modeling contexts. Agent-based models are applied for many reasons (e.g., system understanding, prediction, social learning; Edmonds (2017)) and are equally diverse in terms of levels of realism and knowledge integration (O’Sullivan et al. 2016; Schlüter et al. 2019). It might seem intuitive that equity considerations are most relevant for highly applied models that address current societal issues. However, this is not necessarily the case. For example, Sugarscape (Epstein & Axtell 1996) presents a highly abstracted description of society, but sheds considerable insight into power dynamics and the emergence of inequality. Should Epstein and Axtell have engaged with real-world “stakeholders” in developing their model? Perhaps not. However, their unique positionalities unquestionably shaped how they interpret the world and approached the modeling. Ultimately it is difficult to develop generalized guidelines for when equity and the action pathways could or should be considered, and we leave it as a task for modelers to reflect on in their individual contexts.
It remains a question how our framework and action pathways generalize to other computational modeling approaches, for instance system dynamics, economic equilibrium, state-and-transition models, or bioeconomic simulations. The third pathway (assess equity in agent-based models) is relatively specific to ABM, as it is underpinned by agent-based models’ ability to represent heterogeneity and nuanced decision-making processes, which are frequently limiting factors in other modeling paradigms (Emmerling & Tavoni 2021). However, the overall framework (Figure 1) as well as the ‘engage stakeholders’ and ‘acknowledge positionality and bias’ action pathways are likely relevant in other computational modeling domains, as they rest on a more general description of a modeler who is nested within a socio-political context. Finally, we have demonstrated how ABM is particularly suited to model equity dynamics, but our framework does not help to determine whether ABM is an appropriate methodological paradigm for the given research context (Schlüter et al. 2019); agent-based models require extensive time and expertise to develop, validate, and apply (Voinov et al. 2018), and these resource demands can be prohibitive.
Conclusions
Historical, current, and future societal inequities demand that greater attention be paid to equity within modeling research. Relative to other modeling approaches, ABM is uniquely positioned to incorporate such considerations. Agent-based modelers therefore have a unique opportunity to lead the way in equity-oriented modeling practice and application. To facilitate this meta-agency, this paper sought to develop an initial understanding of the equity-ABM interface through two contributions: a conceptual framework and a set of action pathways.
The conceptual framework uses an analogy of the modeler as a “filter and a lens” to emphasize the potential effects of modeler positionality on introducing bias throughout the modeling process. The framework thereby positions the modeler as a powerful actor and a key locus for affecting equity outcomes, both in the model itself and in its interaction with science and society.
The framework introduces three action pathways for incorporating equity considerations in ABM research: engage stakeholders, acknowledge positionality and bias, and assess equity in agent-based models. By reviewing the current state of practice, we found that ABM research already engages with equity, but to varying extents in each action pathway. There is thus ample precedent and existing knowledge from which future work can draw. To complement this knowledge and to facilitate the mainstreaming of equity considerations, we provided a set of reflection questions for encouraging reflexivity and a typology of approaches for modeling equity in agent-based models. Future work can build on these guidelines and test their value in different modeling contexts.
The challenge of incorporating equity into ABM is by no means insurmountable. We have underscored that improving equity need not require drastic changes to the direction or focus of the modeling project. Rather, it minimally requires reflecting on how the problem is being framed and how this is conditioned by researcher positionality, as well as transparency around the inherent subjectivity in model design and outputs.
Nevertheless, advancing equity outcomes through ABM is a large endeavor that will take time and concerted effort. By engaging with the action pathways, even when only a subset of these or as a post-hoc consideration, modelers can begin to push the needle toward this goal. By acting as role models for equitable modeling practice, agent-based modelers can expand the reach of ABM and demonstrate its usefulness to researchers interested in equity but not experienced with ABM. Taken together, we believe the equity-ABM interface is a fruitful area for future research and a mechanism through which ABM can achieve greater societal impact.
Acknowledgements
TW was supported by funding from the Rackham Predoctoral Fellowship and is grateful to discussions at the Peach Street Writing Group, which provided the head space and original impetus for this paper. BM was supported by funding from the German Federal Ministry of Education and Research (BMBF) within the research project NamTip (01LC1821D) and by the European Union’s Horizon 2020 research and innovation programme under grant agreement No. 817501 (BESTMAP). NM was supported by the U.S. National Science Foundation through the award INFEWS-1856054. The authors would also like to thank Gunnar Dressler and Ruchie Pathak for their assistance in preparing the positionality and bias documents.Appendices and Supplementary Materials
The Appendices and Supplementary Materials can be accessed at: https://www.jasss.org/25/3/1/SI3.pdf. Here, is an additional "positionality and bias template" WORD file.
References
ADIGA, A., Chu, S., Eubank, S., Kuhlman, C. J., Lewis, B., Marathe, A., Marathe, M., Nordberg, E. K., Swarup, S., Vullikanti, A., & Wilson, M. L. (2018). Disparities in spread and control of influenza in slums of Delhi: Findings from an agent-based modelling study. BMC Open, 8(1). [doi:10.1136/bmjopen-2017-017353]
AODHA, L., & Edmonds, B. (2017). 'Some pitfalls to beware when applying models to issues of policy relevance.' In B. Edmonds & R. Meyer (Eds.), Simulating Social Complexity: A Handbook (pp. 801–822). Berlin Heidelberg: Springer International Publishing. [doi:10.1007/978-3-319-66948-9_29]
ARNOTT, J. C., Neuenfeldt, R. J., & Lemos, M. C. (2020). Co-producing science for sustainability: Can funding change knowledge use? Global Environmental Change, 60, 101979. [doi:10.1016/j.gloenvcha.2019.101979]
ARNSTEIN, S. R. (1969). A ladder of citizen participation. Journal of the American Institute of Planners, 35(4), 216–224. [doi:10.1080/01944366908977225]
ASIEDU, E., Karlan, D., Lambon-Quayefio, M., & Udry, C. (2021). A call for structured ethics appendices in social science papers. Proceedings of the National Academy of Sciences, 118(29), e2024570118. [doi:10.3386/w28393]
AUCHINCLOSS, A. H., Riolo, R. L., Brown, D. G., Cook, J., & Diez Roux, A. V. (2011). An agent-based model of income inequalities in diet in the context of residential segregation. American Journal of Preventive Medicine, 40(3), 303–311. [doi:10.1016/j.amepre.2010.10.033]
AUGUSIAK, J., van den Brink, P. J., & Grimm, V. (2014). Merging validation and evaluation of ecological models to ’evaludation’: A review of terminology and a practical approach. Ecological Modelling, 280, 117–128. [doi:10.1016/j.ecolmodel.2013.11.009]
AYLLÓN, D., Railsback, S. F., Gallagher, C., Augusiak, J., Baveco, H., Berger, U., Charles, S., Martin, R., Focks, A., Galic, N., Liu, C., E., v. E., Nabe-Nielsen, J., Piou, C., Polhill, J. G., Preuss, T. G., Radchuk, V., Schmolke, A., Stadnicka-Michalak, J., … Grimm, V. (2021). Keeping modelling notebooks with TRACE: Good for you and good for environmental research and management support. Environmental Modelling & Software, 136, 104932.
BARNAUD, C., & van Paassen, A. (2013). Equity, power games, and legitimacy: Dilemmas of participatory natural resource management. Ecology and Society, 18(2), 21. [doi:10.5751/es-05459-180221]
BARRETEAU, O., Antona, M., D’Aquino, P., Aubert, S., Boissau, S., Bousquet, F., Daré, W., Etienne, M., Le Page, C., Mathevet, R., Trébuil, G., & Weber, J. (2003). Our companion modelling approach. Journal of Artificial Societies and Social Simulation, 6(2), 1: https://www.jasss.org/6/2/1.html.
BECK, E. C., Birkett, M., Armbruster, B., & Mustanski, B. (2015). Data-driven simulation of HIV spread among young men who have sex with men: Role of age and race mixing and STIs. Journal of Acquired Immune Deficiency Syndromes, 70(2), 186–194. [doi:10.1097/qai.0000000000000733]
BELL, A. R., Ward, P. S., & Shah, M. A. A. (2016). Increased water charges improve efficiency and equity in an irrigation system. Ecology and Society, 21(3), 23. [doi:10.5751/es-08642-210323]
BOURCERET, A., Amblard, L., & Mathias, J. D. (2021). Governance in social-ecological agent-based models: A review. Ecology and Society, 26(2), 38. [doi:10.5751/es-12440-260238]
BÜSCHER, B., & Ramutsindela, M. (2015). Green violence: Rhino poaching and the war to save Southern Africa’s peace parks. African Affairs, 115(458), 1–22.
CAMPBELL, H. E., Kim, Y., & Eckerd, A. (2015). Rethinking Environmental Justice in Sustainable Cities: Insights from Agent-Based Modeling. London: Routledge.
CARDACI, A. (2018). Inequality, household debt and financial instability: An agent-based perspective. Journal of Economic Behavior and Organization, 149, 434–458. [doi:10.1016/j.jebo.2018.01.010]
CHOI, T., & Robertson, P. J. (2014). Deliberation and decision in collaborative governance: A simulation of approaches to mitigate power imbalance. Journal of Public Administration Research and Theory, 24(2), 495–518. [doi:10.1093/jopart/mut003]
CLARK, W. C., & Harley, A. G. (2020). Sustainability science: Toward a synthesis. Annual Review of Environment and Resources, 45(1), 331–386. [doi:10.1146/annurev-environ-012420-043621]
CORBETT-DAVIES, S., Pierson, E., Feller, A., Goel, S., & Huq, A. (2017). Algorithmic decision making and the cost of fairness. Proceedings of the 23rd ACM SIGKDD International Conference on Knowledge Discovery and Data Mining. [doi:10.1145/3097983.3098095]
DAVIES, J. B. (2008). Combining microsimulation with CGE and macro modelling for distributional analysis in developing and transition countries. International Journal of Microsimulation, 2(1), 49–56. [doi:10.34196/ijm.00011]
DÁVID-BARRETT, T., & Dunbar, R. I. M. (2014). Social elites can emerge naturally when interaction in networks is restricted. Behavioral Ecology, 25(1), 58–68.
DE Wildt, T. E., Chappin, E. J. L., van DE Kaa, G., HerDEr, P. M., & van DE Poel, I. R. (2020). Conflicted by DEcarbonisation: Five types of conflict at the nexus of capabilities and DEcentralised energy systems iDEntified with an agent-based moDEl. Energy Research & Social Science, 64, 101451. [doi:10.1016/j.erss.2020.101451]
DIALLO, S. Y., Wildman, W. J., & LeRon Shults, F. (2019). 'Human simulation: A transdisciplinary approach to studying societal problems.' In S. Y. DIALLO, W. J. Wildman, F. L. Shults, & A. Tolk (Eds.), Human Simulation: Perspectives, Insights, and Applications (pp. 3–19). Berlin Heidelberg: Springer International Publishing. [doi:10.1007/978-3-030-17090-5_1]
ECKERD, A., Kim, Y., & Campbell, H. E. (2017). Community privilege and environmental justice: An agent-based analysis. The Review of Policy Research, 34(2), 144–167. [doi:10.1111/ropr.12214]
EDMONDS, B. (2017). 'Different modelling purposes.' In B. Edmonds & R. Meyer (Eds.), Simulating Social Complexity: A Handbook (pp. 39–58). Springer International Publishing. [doi:10.1007/978-3-319-66948-9_4]
EMMERLING, J., & Tavoni, M. (2021). Representing inequalities in integrated assessment modeling of climate change. One Earth, 4(2), 177–180. [doi:10.1016/j.oneear.2021.01.013]
EPSTEIN, J. M., & Axtell, R. (1996). Growing Artificial Societies: Social Science from the Bottom Up. Cambridge, MA: The MIT Press. [doi:10.7551/mitpress/3374.001.0001]
FARHADI, S., Nikoo, M. R., Rakhshandehroo, G. R., Akhbari, M., & Alizadeh, M. R. (2016). An agent-based-Nash modeling framework for sustainable groundwater management: A case study. Agricultural Water Management, 177, 348–358. [doi:10.1016/j.agwat.2016.08.018]
FILATOVA, T., Voinov, A., & van der Veen, A. (2011). Land market mechanisms for preservation of space for coastal ecosystems: An agent-based analysis. Environmental Modelling & Software, 26(2), 179–190. [doi:10.1016/j.envsoft.2010.08.001]
FINLAY, L. (1998). Reflexivity: An essential component for all research? The British Journal of Occupational Therapy, 61(10), 453–456. [doi:10.1177/030802269806101005]
FRASER, N. (1995). From redistribution to recognition? Dilemmas of justice in a “postsocialist” age. Available at: http://bibliopreta.com.br/wp-content/uploads/2018/01/Fraser-Redistribution-Recognition-Dilema-1.pdf.
FRASER, N. (2009). Scales of Justice: Reimagining Political Space in a Globalizing World. New York, NY: Columbia University Press.
GOODREAU, S. M., Rosenberg, E. S., Jenness, S. M., Luisi, N., Stansfield, S. E., Millett, G. A., & Sullivan, P. S. (2017). Sources of racial disparities in HIV prevalence in men who have sex with men in Atlanta, GA, USA: A modelling study. The Lancet HIV, 4(7), 311–320. [doi:10.1016/s2352-3018(17)30067-x]
GRIMBLE, R., & Chan, M. K. (1995). Stakeholder analysis for natural resource management in developing countries. Natural Resources Forum, 19(2), 113–124. [doi:10.1111/j.1477-8947.1995.tb00599.x]
GRIMM, V., Augusiak, J., Focks, A., Frank, B. M., Gabsi, F., Johnston, A. S. A., Liu, C., Martin, B. T., Meli, M., Radchuk, V., Thorbek, P., & Railsback, S. F. (2014). Towards better modelling and decision support: Documenting model development, testing, and analysis using TRACE. Ecological Modelling, 280, 129–139. [doi:10.1016/j.ecolmodel.2014.01.018]
GRIMM, V., & Railsback, S. F. (2013). Individual-Based Modeling and Ecology. Princeton, NJ: Princeton University Press.
GRIMM, V., Railsback, S. F., Vincenot, C. E., Berger, U., Gallagher, C., DeAngelis, D. L., Edmonds, B., Ge, J., Giske, J., & Groeneveld, J. (2020). The ODD protocol for describing agent-based and other simulation models: A second update to improve clarity, replication, and structural realism. Journal of Artificial Societies and Social Simulation, 23(2), 7: https://www.jasss.org/23/2/7.html. [doi:10.18564/jasss.4259]
GROENEVELD, J., Müller, B., Buchmann, C. M., Dressler, G., Guo, C., Hase, N., Hoffmann, F., John, F., Klassert, C., Lauf, T., Liebelt, V., Nolzen, H., Pannicke, N., Schulze, J., Weise, H., & Schwarz, N. (2017). Theoretical foundations of human decision-making in agent-based land use models – A review. Environmental Modelling & Software, 87, 39–48. [doi:10.1016/j.envsoft.2016.10.008]
HAMMOND, R. A., & Axelrod, R. (2006). The evolution of ethnocentrism. The Journal of Conflict Resolution, 50(6), 926–936. [doi:10.1177/0022002706293470]
HARTSHORN, M., Kaznatcheev, A., & Shultz, T. (2013). The evolutionary dominance of ethnocentric cooperation. Journal of Artificial Societies and Social Simulation, 16(3), 7: https://www.jasss.org/16/3/7.html. [doi:10.18564/jasss.2176]
HÄMÄLÄINEN, R. P., Miliszewska, I., & Voinov, A. (2020). Leadership in participatory modelling – Is there a need for it? Environmental Modelling & Software, 133, 104834.
HENRY, A. D., & Brugger, H. I. (2017). 'Agent-based explorations of environmental consumption in segregated networks.' In C. G. Díaz & C. Olaya (Eds.), Social Systems Engineering: The Design of Complexity (pp. 199–214). Hoboken, NJ: John Wiley & Sons. [doi:10.1002/9781118974414.ch10]
HOLMES, A. G. D. (2020). Researcher positionality – A consideration of its influence and place in qualitative research – A new researcher guide. Shanlax International Journal of Education, 8(4), 1–10.
INMAN, S., Esquible, J., Jones, M., Bechtol, W., & Connors, B. (2021). Opportunities and impediments for use of local data in the management of salmon fisheries. Ecology and Society, 26(2), 26. [doi:10.5751/es-12117-260226]
JACOBS, A. Z., & Wallach, H. (2021). Measurement and fairness. Proceedings of the 2021 ACM Conference on Fairness, Accountability, and Transparency. [doi:10.1145/3442188.3445901]
JANSSEN, M. A., Pritchard, C., & Lee, A. (2020). On code sharing and model documentation of published individual and agent-based models. Environmental Modelling & Software, 134, 104873. [doi:10.1016/j.envsoft.2020.104873]
KLEIN, E. S., Barbier, M. R., & Watson, J. R. (2017). The dual impact of ecology and management on social incentives in marine common-pool resource systems. Royal Society Open Science, 4(8). [doi:10.1098/rsos.170740]
KONOW, J. (2003). Which is the fairest one of all? A positive analysis of justice theories. Journal of Economic Literature, 41(4), 1188–1239. [doi:10.1257/002205103771800013]
KUSOW, A. M. (2003). Beyond indigenous authenticity: Reflections on the insider/outsider debate in immigration research. Symbolic Interaction, 26(4), 591–599. [doi:10.1525/si.2003.26.4.591]
KUSTOV, A. (2017). How ethnic structure affects civil conflict: A model of endogenous grievance. Conflict Management and Peace Science, 34(6), 660–679. [doi:10.1177/0738894215613035]
LEACH, M., Reyers, B., Bai, X., Brondizio, E. S., Cook, C., Díaz, S., Espindola, G., Scobie, M., Stafford-Smith, M., & Subramanian, S. M. (2018). Equity and sustainability in the Anthropocene: A social–ecological systems perspective on their intertwined futures. Global Sustainability, 1. [doi:10.1017/sus.2018.12]
LEACH, M., Scoones, I., & Stirling, A. (2010). Dynamic Sustainabilities: Technology, Environment, Social Justice. London: Routledge. [doi:10.4324/9781849775069]
LEBLOND, N., & Trottier, J. (2016). Performing an invisibility spell: Global models, food regimes and smallholders. The International Journal of Sociology of Agriculture and Food, 23(1).
LEMOS, M. C., Arnott, J. C., Ardoin, N. M., Baja, K., Bednarek, A. T., Dewulf, A., Fieseler, C., Goodrich, K. A., Jagannathan, K., Klenk, N., Mach, K. J., Meadow, A. M., Meyer, R., Moss, R., Nichols, L., Sjostrom, K. D., Stults, M., Turnhout, E., Vaughan, C., … Wyborn, C. (2018). To co-produce or not to co-produce. Nature Sustainability, 1(12), 722–724. [doi:10.1038/s41893-018-0191-0]
LEMPERT, R. J., & Turner, S. (2021). Engaging multiple worldviews with quantitative decision support: A robust decision-Making demonstration using the lake model. Risk Analysis: An Official Publication of the Society for Risk Analysis, 41(6), 845–865. [doi:10.1111/risa.13579]
LE PAGE, C., & Perrotton, A. (2018). 'KILT: A modelling approach based on participatory agent-Based simulation of stylized socio-Ecosystems to stimulate social learning with local stakeholders.' In G. Sukthankar & J. A. Rodriguez-Aguilar (Eds.), Autonomous Agents and Multiagent Systems (pp. 156–169). Berlin Heidelberg: Springer. [doi:10.1007/978-3-319-71679-4_3]
MAHAULT, B., Saxena, A., & Nisoli, C. (2017). Emergent inequality and self-organized social classes in a network of power and frustration. PLoS ONE, 12(2), e0171832. [doi:10.1371/journal.pone.0171832]
MALIK, A., Crooks, A., Root, H., & Swartz, M. (2015). Exploring creativity and urban development with agent-based modeling. Journal of Artificial Societies and Social Simulation, 18(2), 12: https://www.jasss.org/18/2/12.html. [doi:10.18564/jasss.2722]
MATHIAS, J. D., Anderies, J. M., Baggio, J., Hodbod, J., Huet, S., Janssen, M. A., Milkoreit, M., & Schoon, M. (2020). Exploring non-linear transition pathways in social-ecological systems. Scientific Reports, 10(1), 4136. [doi:10.1038/s41598-020-59713-w]
MCDERMOTT, M., Mahanty, S., & Schreckenberg, K. (2013). Examining equity: A multidimensional framework for assessing equity in payments for ecosystem services. Environmental Science & Policy, 33, 416–427. [doi:10.1016/j.envsci.2012.10.006]
MCINTOSH, P. (2020). White privilege and male privilege: A personal account of coming to see correspondences through work. Privilege and Prejudice: Twenty Years with the Invisible Knapsack, 7. [doi:10.4324/9781351133791-3]
MEHRABI, N., Morstatter, F., Saxena, N., Lerman, K., & Galstyan, A. (2019). A survey on bias and fairness in machine learning. ACM Computing Surveys, 54(6), 1–35. [doi:10.1145/3457607]
MILNER, H. R. (2018). Race, culture, and researcher positionality: Working through dangers seen, unseen, and unforeseen. Educational Researcher, 36(7), 388–400. [doi:10.3102/0013189x07309471]
MOTCHOULSKI, A. (2021). Adjudicating distributive disagreement. Synthese, 198(7), 5977–6008. [doi:10.1007/s11229-019-02443-y]
MURPHY, K. J., Ciuti, S., & Kane, A. (2020). An introduction to agent-based models as an accessible surrogate to field-based research and teaching. Ecology and Evolution, 10(22), 12482–12498. [doi:10.1002/ece3.6848]
MURRAY, E. J., Robins, J. M., Seage, G. R., Freedberg, K. A., & Hernán, M. A. (2017). A comparison of agent-Based models and the parametric G-Formula for causal inference. American Journal of Epidemiology, 186(2), 131–142. [doi:10.1093/aje/kwx091]
MÜLLER, B., Bohn, F., Dressler, G., Groeneveld, J., Klassert, C., Martin, R., Schlüter, M., Schulze, J., Weise, H., & Schwarz, N. (2013). Describing human decisions in agent-based models – ODD + D, an extension of the ODD protocol. Environmental Modelling & Software, 48, 37–48.
NOBLE, S. U. (2018). Algorithms of Oppression. New York: New York University Press.
NUSSBAUM, M., & Sen, A. (1993). The Quality of Life. Oxford: Oxford University Press.
OBERMEYER, Z., Powers, B., Vogeli, C., & Mullainathan, S. (2019). Dissecting racial bias in an algorithm used to manage the health of populations. Science, 366(6464), 447–453. [doi:10.1126/science.aax2342]
O’NEIL, C. (2016). Weapons of Math Destruction: How Big Data Increases Inequality and Threatens Democracy. New York, NY: Crown Publishing Group.
ORR, M. G., Galea, S., Riddle, M., & Kaplan, G. A. (2014). Reducing racial disparities in obesity: Simulating the effects of improved education and social network influence on diet behavior. Annals of Epidemiology, 24(8), 563–569. [doi:10.1016/j.annepidem.2014.05.012]
O’SULLIVAN, D., Evans, T., Manson, S., Metcalf, S., Ligmann-Zielinska, A., & Bone, C. (2016). Strategic directions for agent-based modeling: Avoiding the YAAWN syndrome. Journal of Land Use Science, 11(2), 177–187. [doi:10.1080/1747423x.2015.1030463]
PAGE, S. E. (1997). On incentives and updating in agent based models. Computational Economics, 10(1), 67–87.
PAGE, S. E. (2010). Diversity and Complexity. Princeton, NJ: Princeton University Press.
POLHILL, J. G., Hare, M., Bauermann, T., Anzola, D., Palmer, E., Salt, D., & Antosz, P. (2021). Using agent-based models for prediction in complex and wicked systems. Journal of Artificial Societies and Social Simulation, 24(3), 2: https://www.jasss.org/24/3/2.html. [doi:10.18564/jasss.4597]
PONSIGLIONE, C., Roma, V., Zampella, F., & Zollo, G. (2015). 'The fairness/efficiency issue explored through El Farol Bar model.' In J. Gil-Aluja, A. Terceño-Gómez, J. C. Ferrer-Comalat, & J. M. Merigó-Lindahl (Eds.), Scientific Methods for the Treatment of Uncertainty in Social Sciences. Berlin Heidelberg: Springer. [doi:10.1007/978-3-319-19704-3_26]
PRETTY, J. N. (1995). Participatory learning for sustainable agriculture. World Development, 23(8), 1247–1263. [doi:10.1016/0305-750x(95)00046-f]
RAJKOMAR, A., Hardt, M., Howell, M. D., Corrado, G., & Chin, M. H. (2018). Ensuring fairness in machine learning to advance health equity. Annals of Internal Medicine, 169(12), 866–872. [doi:10.7326/m18-1990]
RAWLS, J. (2001). Justice as Fairness: A Restatement. Cambridge, MA: Harvard University Press.
REED, M. S. (2008). Stakeholder participation for environmental management: A literature review. Biological Conservation, 141(10), 2417–2431. [doi:10.1016/j.biocon.2008.07.014]
SCHANK, J. C., Smaldino, P. E., & Miller, M. L. (2015). Evolution of fairness in the dictator game by multilevel selection. Journal of Theoretical Biology, 382, 64–73. [doi:10.1016/j.jtbi.2015.06.031]
SCHELLING, T. C. (1971). Dynamic models of segregation. The Journal of Mathematical Sociology, 1(2), 143–186.
SCHINDLER, J. (2012). Rethinking the tragedy of the commons: The integration of socio-psychological dispositions. Journal of Artificial Societies and Social Simulation, 15(1), 4: https://www.jasss.org/15/1/4.html. [doi:10.18564/jasss.1822]
SCHLAGER, E. (2019). A comparison of frameworks, theories, and models of policy processes. In P. A. Sabatier (Ed.), Theories of the policy process (pp. 293–319). London: Routledge. [doi:10.4324/9780367274689-10]
SCHLÜTER, M., Lindkvist, E., & Basurto, X. (2021). The interplay between top-down interventions and bottom-up self-organization shapes opportunities for transforming self-governance in small-scale fisheries. Marine Policy, 128, 104485.
SCHLÜTER, M., Müller, B., & Frank, K. (2019). The potential of models and modeling for social-ecological systems research: The reference frame ModSES. Ecology and Society, 24(1), 31.
SCHMOLKE, A., Thorbek, P., DeAngelis, D. L., & Grimm, V. (2010). Ecological models supporting environmental decision making: A strategy for the future. Trends in Ecology & Evolution, 25(8), 479–486. [doi:10.1016/j.tree.2010.05.001]
SHULTS, F. L., & Wildman, W. J. (2019). 'Ethics, computer simulation, and the future of humanity.' In S. Y. Diallo, W. J. Wildman, F. L. SHULTS, & A. Tolk (Eds.), Human Simulation: Perspectives, Insights, and Applications (pp. 21–40). Berlin Heidelberg: Springer International Publishing. [doi:10.1007/978-3-030-17090-5_2]
SIKOR, T., Martin, A., Fisher, J., & He, J. (2014). Toward an empirical analysis of justice in ecosystem governance: Justice in ecosystem governance. Conservation Letters, 7(6), 524–532. [doi:10.1111/conl.12142]
SMART, H. (2019). Operationalizing a conceptual model of colorism in local policing. Social Justice Research, 32(1), 72–115. [doi:10.1007/s11211-018-0318-5]
SMITH, L. T. (2013). Decolonizing Methodologies: Research and Indigenous Peoples. London: Zed Books.
SOBKOWICZ, P. (2016). Agent based model of effects of task allocation strategies in flat organizations. Physica A: Statistical Mechanics and Its Applications, 458, 17–30. [doi:10.1016/j.physa.2016.04.003]
STEGER, C. E., Alemu, S. C., Boone, R. B., Dullo, B. W., Evangelista, P., Gebrehiwot, K., & Klein, J. A. (2022). Collaborative agent-based modeling for managing shrub encroachment in an Afroalpine grassland.
STEGER, C., Hirsch, S., Cosgrove, C., Inman, S., Nost, E., Shinbrot, X., Thorn, J. P. R., Brown, D. G., Grêt-Regamey, A., Müller, B., Reid, R. S., Tucker, C., Weibel, B., & Klein, J. A. (2021). Linking model design and application for transdisciplinary approaches in social-ecological systems. Global Environmental Change: Human and Policy Dimensions, 66, 102201. [doi:10.1016/j.gloenvcha.2020.102201]
STEGER, C., Klein, J. A., Reid, R. S., Lavorel, S., Tucker, C., Hopping, K. A., Marchant, R., Teel, T., Cuni-Sanchez, A., Dorji, T., Greenwood, G., Huber, R., Kassam, K. A., Kreuer, D., Nolin, A., Russell, A., Sharp, J. L., Šmid Hribar, M., Thorn, J. P. R., … Waiswa, D. (2021). Science with society: Evidence-based guidance for best practices in environmental transdisciplinary work. Global Environmental Change: Human and Policy Dimensions, 68, 102240. [doi:10.1016/j.gloenvcha.2021.102240]
SUN, Z., Lorscheid, I., Millington, J. D., Lauf, S., Magliocca, N. R., Groeneveld, J., Balbi, S., Nolzen, H., Müller, B., Schulze, J., & Buchmann, C. M. (2016). Simple or complicated agent-based models? A complicated issue. Environmental Modelling & Software, 86, 56–67. [doi:10.1016/j.envsoft.2016.09.006]
TOL, R. S. J., Downing, T. E., Kuik, O. J., & Smith, J. B. (2004). Distributional aspects of climate change impacts. Global Environmental Change: Human and Policy Dimensions, 14(3), 259–272. [doi:10.1016/j.gloenvcha.2004.04.007]
TYLER, T. R. (2000). Social justice: Outcome and procedure. International Journal of Psychology: Journal International de Psychologie, 35(2), 117–125. [doi:10.1080/002075900399411]
UN General Assembly. (2015). Transforming our world: The 2030 Agenda for Sustainable Development. Available at: https://sdgs.un.org/2030agenda.
VOINOV, A., & Bousquet, F. (2010). Modelling with stakeholders. Environmental Modelling & Software, 25(11), 1268–1281. [doi:10.1016/j.envsoft.2010.03.007]
VOINOV, A., Jenni, K., Gray, S., Kolagani, N., Glynn, P. D., Bommel, P., Prell, C., Zellner, M., Paolisso, M., Jordan, R., Sterling, E., Schmitt Olabisi, L., Giabbanelli, P. J., Sun, Z., Le Page, C., Elsawah, S., BenDor, T. K., Hubacek, K., Laursen, B. K., … Smajgl, A. (2018). Tools and methods in participatory modeling: Selecting the right tool for the job. Environmental Modelling & Software, 109, 232–255. [doi:10.1016/j.envsoft.2018.08.028]
VOINOV, A., Kolagani, N., McCall, M. K., Glynn, P. D., Kragt, M. E., Ostermann, F. O., Pierce, S. A., & Ramu, P. (2016). Modelling with stakeholders - Next generation. Environmental Modelling & Software, 77, 196–220. [doi:10.1016/j.envsoft.2015.11.016]
VOINOV, A., Seppelt, R., Reis, S., Nabel, J. E. M. S., & Shokravi, S. (2014). Values in socio-environmental modelling: Persuasion for action or excuse for inaction. Environmental Modelling & Software, 53, 207–212. [doi:10.1016/j.envsoft.2013.12.005]
WEISKOPF, S. R., Harmáčkovó, Z. V., Johnson, C. G., Londoño-Murcia, M. C., Miller, B. W., Myers, B. J. E., Pereira, L., Arce-Plata, M. I., Blanchard, J. L., Ferrier, S., Fulton, E. A., Harfoot, M., Isbell, F., Johnson, J. A., Mori, A. S., Weng, E., & Rosa, I. (2022). Increasing the uptake of ecological model results in policy decisions to improve biodiversity outcomes. Environmental Modelling & Software, 149, 105318. [doi:10.1016/j.envsoft.2022.105318]
WELCH, L., & Ekwaro-Osire, S. (2010). Fairness in agent based simulation frameworks. Journal of Computing and Information Science in Engineering, 10(1), 011002. [doi:10.1115/1.3290764]
WILL, M., Dressler, G., Kreuer, D., Thulke, H., Grêt‐Regamey, A., & Müller, B. (2020). How to make socio‐environmental modelling more useful to support policy and management? People & Nature, 3(3), 560–572. [doi:10.1002/pan3.10207]
WILLIAMS, T. G., Guikema, S. D., Brown, D. G., & Agrawal, A. (2020). Resilience and equity: Quantifying the distributional effects of resilience-enhancing strategies in a smallholder agricultural system. Agricultural Systems, 182, 102832. [doi:10.1016/j.agsy.2020.102832]
WILSON, S. (2001). What is an indigenous research methodology? Canadian Journal of Native Education, 25(2), 175–179.
ZELNER, J., Masters, N. B., Naraharisetti, R., Mojola, S., & Chowkwanyun, M. (2022). There are no equal opportunity infectors: Epidemiological modelers must rethink our approach to inequality in infection risk. PLoS Computational Biology, 18(2), e1009795. [doi:10.1371/journal.pcbi.1009795]
ZHANG, J., & Robinson, D. T. (2021). Replication of an agent-based model using the replication standard. Environmental Modelling & Software, 139, 105016. [doi:10.1016/j.envsoft.2021.105016]
ZUCMAN, G. (2019). Global wealth inequality. Annual Review of Economics, 11(1), 109–138. [doi:10.1146/annurev-economics-080218-025852]