An Agent-Based Model of Hierarchical Information-Sharing Organizations in Asynchronous Environments

,
and
Utah State University, United States
Journal of Artificial
Societies and Social Simulation 27 (2) 2
<https://www.jasss.org/27/2/2.html>
DOI: 10.18564/jasss.5328
Received: 23-May-2022 Accepted: 23-Jan-2024 Published: 31-Mar-2024
Abstract
Most organizations use command hierarchies—the type of hierarchy depicted in a common organizational chart—but it is not well understood why and how environments make this structure useful. One possibility is that command hierarchies provide positive net benefits when groups of agents must respond to changes in the environment, particularly when each group member’s local conditions are similar and somewhat synchronous. We ask: How does the performance of hierarchical groups vary with changing environments? We build an agent-based model to better understand the strengths and weaknesses of hierarchy for groups faced with these changes in space and time. In these environments, a local worker has more information about local conditions, but a manager has more information about overall conditions. We show that command hierarchy outperforms non-hierarchy in many synchronous and asynchronous environments, including those where local conditions differ substantially and would seem to make a manager’s “big picture” input much less useful to workers. In these more asynchronous environments, a manager’s view of overall conditions does give useful information to workers, with crucial caveats: workers must have the autonomy to judge the accuracy and relevance of manager input to their local work, or they perform worse than non-hierarchical groups. This autonomy enables the organization to learn. Relatedly, we also find increased agent memory is important for performance in all environments. Our model reveals that environments that vary locally can cause unavoidable tension between the views of front-line workers and managers, or local offices and head offices; even perfect agents find themselves in an inevitable computational dilemma. The best organizational strategy to manage this dilemma is continuing to provide manager input while enabling some degree of worker autonomy.Introduction
A key criticism of organizational hierarchy is that when local information is scaled up and aggregated, it may lose critical local patterns, result in unrealistic assumptions (Gupta 2008), or result in a mismatch between management expectations and local context (Durose 2009). But is this inevitable, and how much does the environment matter in this mismatch? Most organizations with hierarchy must coordinate among local and higher-level entities, such as non-supervisory and supervisory employees – here called workers and managers – or local and head offices. In these organizations, a local worker has more information about local conditions, but a manager likely has more information about overall conditions. This suggests that environmental conditions do play a role in this mismatch, even if the nature of that role is unclear. Further, practical formalizations of command hierarchy – e.g., organizational charts, chains of command – require effort in creation and maintenance; these costs suggest hierarchy must confer some benefits in some situations. Yet, one such class of situations may be environments where groups must deal with environmental change. To assess whether information flow in a command hierarchy generates benefits that outweigh the costs of forming and maintaining command hierarchies in dynamic environments, we build and analyze a computational model.
Hierarchical organizations are ubiquitous in human culture (Bas & Sebastian-Galles 2021; Guinote et al. 2015; Halevy et al. 2011; Thomsen 2020), yet what researchers mean by “hierarchy” varies. Zafeiris & Vicsek (2017) (revising Lane 2006), provide a useful 3-category typology based on different arrangements or relations among entities: 1) order, as in an ordered set or ranking; 2) nested, where entities are within entities, including specific-to-general classifications like taxonomies and systems-in-systems; 3) control or flow, where relations between entities form an acyclic, directed graph of influence, in whole or part. In the context of human organizations, we prefer to call the control/flow type of hierarchy a command hierarchy so that full obedience is not implied. Although people inhabit organizations that simultaneously exhibit all of these types of hierarchy, an essential property of organizations is their governance of action (Schatzki 2006), and command hierarchy via superordinate and subordinate roles is the relational structure that defines the formal structure of decision-making in most organizations (Scott & Davis 2007). This role-based command structure – versus levels of ranked relations of social prestige – also existed in administrative structures thousands of years ago (Duncan 2021; Nissen et al. 1993; Papazian 2013), indicating a deep history of command hierarchies in human organizational behavior.
We focus on command hierarchy in this research to investigate how its structure affects group performance. Rank hierarchy is of less importance to operational decision-making: Bob’s boss Alice commands him because of her place directly above him in the command hierarchy, not because of her rank in the organization more broadly. Nested hierarchies in organizations are not so much social relations but groupings of positions that result from command structures with purviews: the Chief Information Officer necessarily has a different branch of the organization than the Chief Operating Officer, with different nested subunits within it. Thus, rank and nested hierarchies have less relevance than command hierarchies in understanding ground-level decision-making in organizations. Further, focusing on command hierarchy alone allows greater analytical clarity in understanding its particular role within the decision-making of organizations.
Removing social power while retaining a simple hierarchy
We recognize that there are multifaceted influences within command hierarchies, including the social power of workers and supervisors alike. However, to disentangle any structural benefits of command hierarchy from issues of social power, we eliminate social power within the model to focus on the structure command hierarchy creates. For the purposes of our inquiry, we define command hierarchy as hierarchical information flow among decision-makers. The presence of decision-makers keeps this a social inquiry; if they could not decide, they would be nothing more than actuators in a control system.
If the worker-view versus manager-view is an inherent tension in command hierarchies, we should see that tension emerge in a model where environmental changes are navigated by a “perfect” organization – one where the people are equally competent, without any intention to free-ride or capture resources, with perfect communication ability, and with perfect recall. We now review the simplest set of factors we see as necessary to build such a model, and how the model relates to previous agent-based models of hierarchy.
Local knowledge
Workers incorporate local knowledge into their decision-making in ways that matter for organizational performance. Plant workers (Colombo & Delmastro 2004), public workers (Durose 2011), and international workers (Eckhard 2021) have all been found to use local, often tacit, knowledge to adapt their work to local conditions. Further, local knowledge is important in human organizational systems at different scales (for example, in anthropology, see Scott 1998; Acheson 2011; Boyd et al. 2011; Romano et al. 2020; and in commons research see Young et al. 2008; Cosens & Gunderson 2018).
Organizational memory
Organizational memory is a key factor in understanding command hierarchy where workers and managers learn a changing environment. In practice, organizational memory is “written” with shared knowledge among interpersonal networks (Hardt 2018; Siciliano 2015), business processes and their cues (Kluge & Gronau 2018), expertise, databases, and documentation (Fiedler & Welpe 2010; Hardt 2018), to name a few. More abstractly, organizations create knowledge by putting this experience in context (Argote & Miron-Spektor 2011), and they demonstrate learning when encoded experiences influence their behavior (Greve 2017). Taken altogether, these may be seen as a functionalist perspective on organizational memory as opposed to interpretive, performative, or critical (Foroughi et al. 2020) views. While the functionalist view may be considered a managerialist preoccupation with the utility of memory for organizational performance (Rowlinson et al. 2010), it remains the most appropriate approach for understanding command hierarchy where, indeed, we are interested in both the effect of managers and organizational performance. Thus, we model organizational memory by having individual memories that, when interacting together, influence organizational performance.
Communication
Communication is essential for better group performance and cooperation (Balliet 2010; Janssen et al. 2014; Pavitt 2018; Sally 1995). More broadly, organizations enable and constrain collective action through communication (Cooren et al. 2011; Kuhn & Ashcraft 2003) that generates common understandings and trust-building (Ostrom 2005), reveals preferences (Ertac & Gurdal 2019), links knowledge (Eckhard 2021), forms organizational memory (Fiedler & Welpe 2010), and makes leadership possible (Glowacki & von Rueden 2015). Thus, communication lies at the heart of organizations and organizational processes.
Autonomy
Generally, effective organizational decision-making depends on the collocation of uncovered information and the right to act on that information (Chang & Harrington 2006; Ostrom 2005). Autonomy enables workers to employ local knowledge and inter-organizational communication to influence performance.
Changing environments
There is a strong need in organizational science to understand, theoretically, how and under what conditions organizations persist in the face of environmental transitions while others do not (O’Reilly & Tushman 2008), what tradeoffs organizations make in adapting to changing environments (Hong & Lee 2018), and how organizational adaptation emerges (Chaffin et al. 2014). To further address this gap, we focus on changing environments – both in time and space – rather than static, rugged landscapes (e.g., Levinthal 1997; Chang & Harrington 2000; Ornstein et al. 2020).
Many types of organizations – and networks of organizations – face challenges with changing environments, both biophysical and social, and usually some combination of the two. The impacts of climate change on human organizations provides one entry point for considering of the diversity of organizations that must deal with these challenges. These include natural disasters (Stute et al. 2020), wildfire regimes (Yocom Kent et al. 2017), seasonal park visitation (Horne et al. 2022), proliferation of invasive species (Liebhold 2012), shifting production of crops (Mehrabi & Ramankutty 2019), and supply chain shocks (Baldwin & Freeman 2022). These are all quite different, but share a core of environmental change that, while not homogenous, was found to have some synchrony where external information could be useful for making local decisions. For example, insect outbreaks tend to be spatially and temporally synchronized (Liebhold 2012), while wildfire regimes have synchrony that includes more variable local influence (Yocom Kent et al. 2017). Thus, many fields show a need for a foundational, context-agnostic understanding of organizations dealing with environmental changes, particularly changes that vary in synchrony among different locales. Agent-based models are particularly suited for this type of abstraction, both to act as a boundary object to bridge fields, and to act as a base where context-relevant complexities can be added.
Changing environments have received some attention in work using models to understand organizations and command hierarchy. This ranges from small roles, such as a single changing state (Zafeiris & Vicsek 2017), to larger billing such as environments of information (Meagher et al. 2003; van Zandt & Radner 2001) patterns of identical problems (Epstein 2003) and varied resource patchiness and clustering (Hooper 2018). Roughly, these models may be divided into two categories: those with benefits from information sharing/co-processing (Meagher et al. 2003; van Zandt & Radner 2001; Zafeiris & Vicsek 2017) versus those with benefits from reduced coordination costs (Epstein 2003; Hooper 2018).
Past models that consider information sharing and co-processing used simpler environments that do not exhibit varied local conditions, leaving an important gap for us to address. Our approach builds on this previous work by taking varied and/or changing environments, similar to Epstein (2003) and Hooper (2018), and considering them solely from a perspective of organizations engaging in beneficial information sharing, similar to Meagher et al. (2003) and Zafeiris & Vicsek (2017).
Team or group performance
Finally, the conditions in which hierarchy benefits teams is unclear; a meta-analysis (Greer et al. 2018) of 54 studies finds that, overall, hierarchy hurts team effectiveness, with the effect mediated by an increased conflict-enabling atmosphere. A review of lab and field experiments found similar results (Anderson & Brion 2014), more negative than positive team performance, yet mixed enough that authors conclude "it depends". Similarly, Greer et al. (2018) notes:
Given that our findings largely support dysfunctional views on hierarchy, future research is needed to understand when and why hierarchy may be more likely to live up to its purported functional benefits.
Addressing this gap, we tackle one potential source of hierarchy’s functional benefits: increases in group performance in dynamic environments.
A model to investigate the tension between local versus broader views
Our suspicion is that organizations, on the whole, cannot avoid making tradeoffs in trusting and weighing local perceptions versus broader-view manager perceptions. These tradeoffs should be visible in the performance of groups in at least some types of changing environments, and may explain when and why hierarchy is beneficial for a group. This leads us to one primary, and two related, research questions:
- In which environmental conditions (i.e., synchronous vs. asynchronous) does command hierarchy affect group performance, and how?
- How important for group performance is agent autonomy in following (or not) the manager’s input?
- How important for group performance is the weight agents put on the manager’s input versus their own experience?
Methods: Model
Purpose and overview
The goal of this model is to examine whether command hierarchy improves group performance for organizations where each worker faces changing local environments. We assume all agents are similarly limited in their capacities, which necessarily means that a local worker has more information about their own local condition than other workers, but a manager has more information about overall conditions. Workers in this environment make decisions about binary problems based on their memory of their local environment, and receive additional input from a manager who has memory about the overall past states.
The environment as a landscape of problems
In Figure 1 we visualize the one-dimensional world agents experience over time as two-dimensional landscapes, and show how simple algorithmic landscape modifications result in local conditions becoming asynchronous with one another. We create an environment that is simple while still making it possible for agents to experience different local environments in space and time. We first create a strip of cells where each one worker stands on one cell. Each worker’s cell is their local state: that state is one of two binary values, and can change its value on each time step of the model. Static environments are of no interest, so the simplest environment in our set is a synchronous environment where all local states are the same in space and change their values simultaneously over time. These environments have little complexity, since every lane is a duplicate of its neighbor lanes; see the upper-left landscape in Figure 1. To create more complex environments, we employ parameters to modify the landscape by delaying (landscapes in the bottom row in Figure 1) or inverting (landscapes to the right in Figure 1) the onset of environmental changes the agents experience relative to one another.
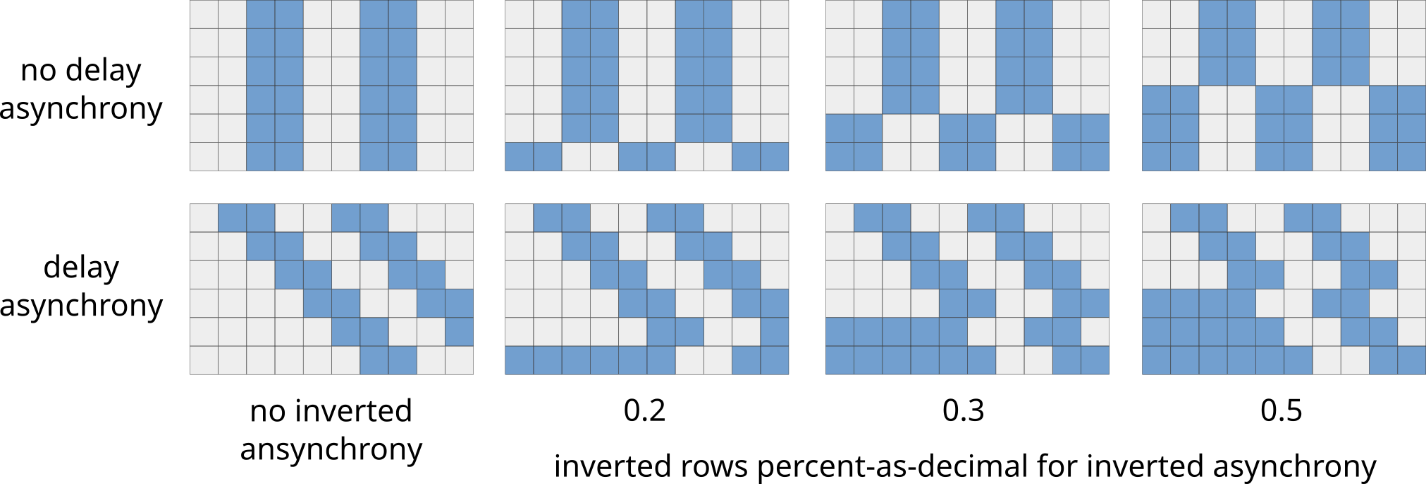
Each cell “problem” is a 0 or 1; each worker has its own lane of problems. When landscapes are synchronous, problem transitions happen across lanes simultaneously. When landscapes are asynchronous, that asynchrony is controlled by two parameters (see Figure 1): 1) delay asynchrony, which is whether to stagger (delay in transition) the problems among the lanes, so that the transition hits each worker at a different time; 2) inverted asynchrony, which is the fraction of lanes where problems are inverted to the opposite value, i.e., where the original 0s are made into 1s, and original 1s are made into 0s. Staggering transitions among workers is a milder form of asynchrony, whereas inverted rows represent very different local sub-environments from the overall environment. By varying these parameters, we can create increasingly asynchronous environments with either delayed environmental changes across agents, or some local conditions that are the inverse of the overall environment, or both.
Agents as problem-solvers on the landscape
In this model, each agent must solve one problem per round by correctly predicting the incoming local environmental state. Agents – both workers and managers – have no ability to foresee future problems, so they must rely on their memory of past problems and on input from others, as shown in Figure 2 boxes A and B. Agents have memories which store the last n problems they have seen (their memories are 3 slots to 9 slots long). When deciding on a strategy for the next problem, they consult their memory and any inputs (i.e., advice from the manager) and take the statistical mode of that set of values; when two modes exist, agents pick one at random, which adds a small degree of stochasticity to the model. Human memories are more sophisticated in how they strengthen with repetition (Hassan & Barber 2021; Zaragoza & Mitchell 1996), but the statistical mode is sufficient as a simple calculation for the dominant category by the agent, who represents a person or an entire office. Agent capabilities and preferences are homogeneous within runs, but become varied in their memories and choices as they learn their heterogeneous environment. The agents’ decision-making is focused on getting the problem in the next time step correct.
The hierarchy condition
We create a hierarchy condition in half of the simulations by including managers which receive information from, and send information to, the worker agents who are solving local problems. These manager agents process the collection of local inputs into the statistical mode and treat that as their prediction problem, then advise all worker agents based on their memory of these modes, as shown in Figure 2 box B. This communication process is a very simple form of command hierarchy, free from additional complicating factors such as social power, free-riding, punishment, and so on.
How much workers weigh their manager’s advice is manipulated either as a static value across a run – non-adjustable by agents – or as a value which agents can adjust. When adjustable, workers assess their problem response each round and can adjust the weights incrementally. Workers decide to adjust weights only when their memory and the manager input disagree. If their memory was the better choice, they up-weigh memory. If listening to the manager was the better choice, they up-weigh manager input. If both were right or both were wrong, they leave the weights unchanged.
Workers that adjust weights use the following algorithm. Let us define a number counting function \(C(x)\) such that each of its components \(i \in \{0,1\}\) counts the respective number in a 0-indexed vector of binary values:
\[ C_{i}(x) := \sum_{j \in N|x_{j} = 1} 1\] | \[(1)\] |
For example,
\[C([1 \; 0 \; 0 \; 1 \; 1]) = [2 \; 3]\] | \[(2)\] |
The counting function is applied to the worker memory vector and the manager input vector, and a weighted sum of the two is used to select the predicted environmental state \(x^{*}\) by picking the component with the highest value:
\[ x^{*} = argmax_{i \in \{0, 1\}} wC(x_{w}) + (1 - w)C(x_{m})\] | \[(3)\] |
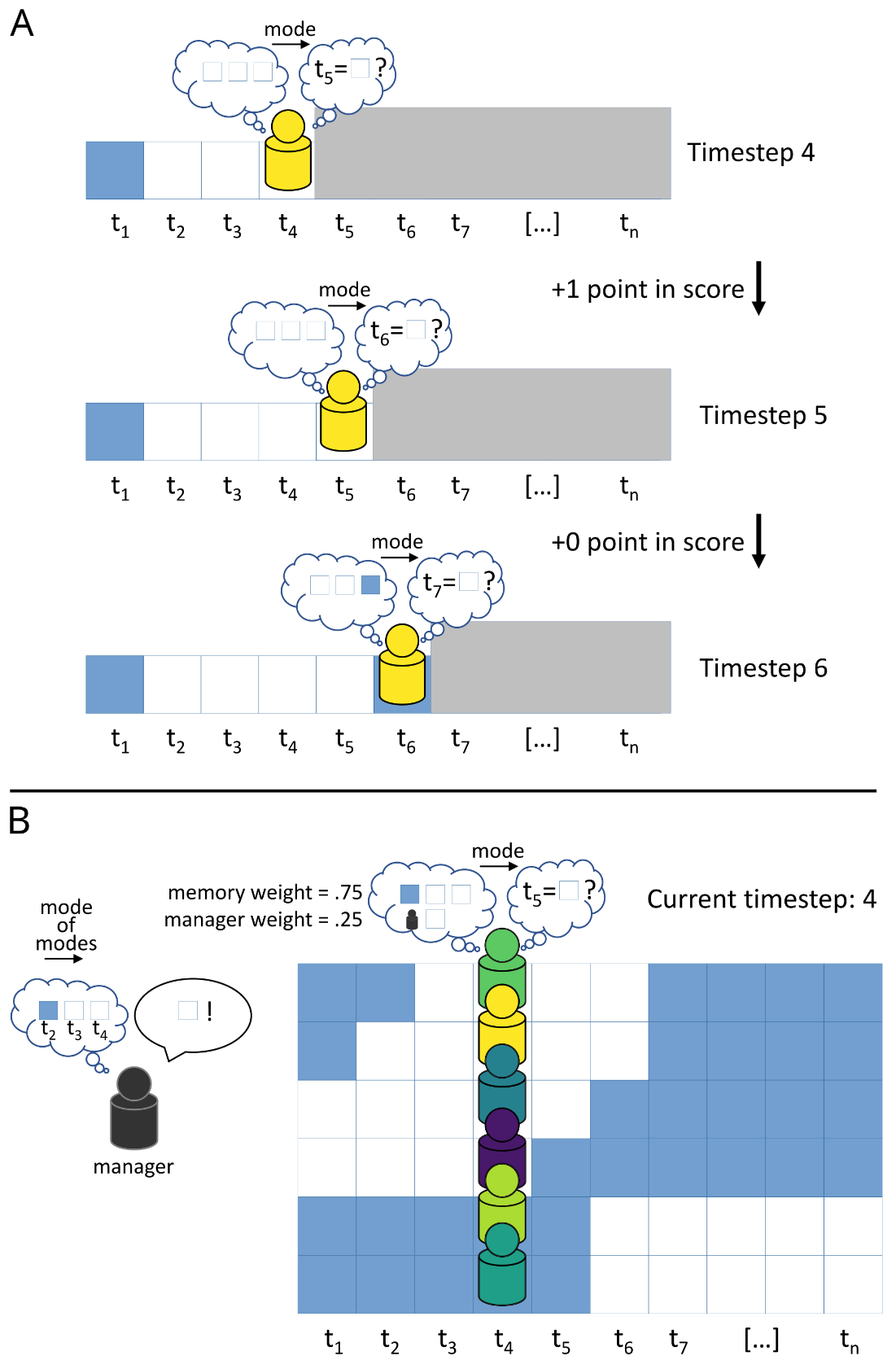
Overview of the model’s major routine
A flowchart of the model routine is shown in Figure 3. The routine of our model is as follows:
- Communicate: if the group has a manager, workers communicate their last problem to the manager, and the manager communicates the statistical mode of the set of all workers’ problems back to the workers.
- Decide solution: all agents take the statistical mode of their memory and inputs.
- View local environment and score decisions: workers compare the local environment value to their chosen solution and score one point if correct, zero points if incorrect. If workers are allowed to weigh manager input, they also adjust weights if needed.
- Store true solutions: workers store the problem in memory. The manager stores the statistical mode in memory.
- Advance to next timestep: all workers advance one problem forward, and loop to step 1, communicate. If workers have reached the end of the timesteps, all worker scores are summed for the group and the simulation ends.
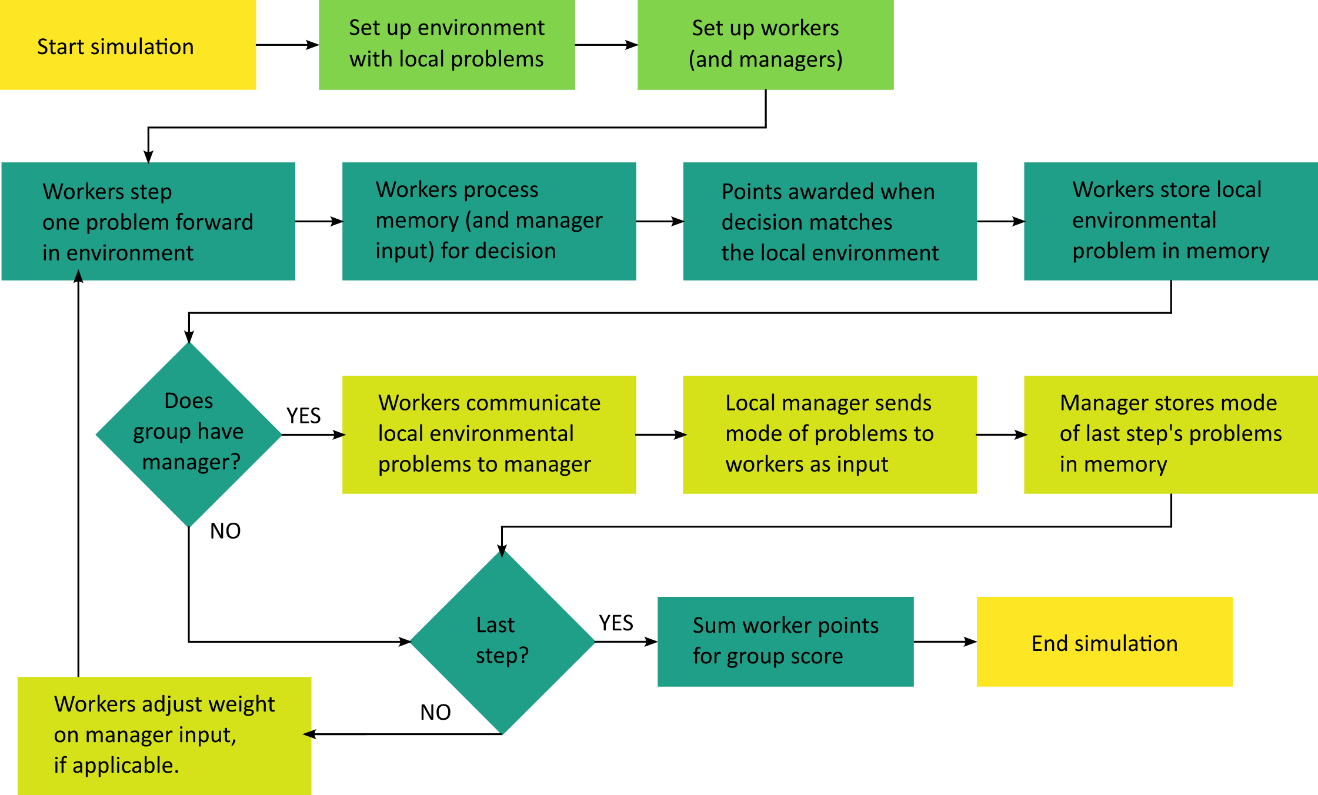
Model parameters
Table 1 contains the key parameters for understanding the model. Simulations included every combination of these parameters. We use groups of 10 workers for all runs in this analysis, as varying the number of workers would only change the spread of scores, not their rank in relation to one another. Ten workers enables score differences to be more clearly visible than it would be in smaller groups.
Parameter | Dynamic? | Possible Values | Description |
---|---|---|---|
number-of-managers | No | [0, 1] | No-hierarchy vs. hierarchy |
delayed-asynchrony | No | [0, 1] | Whether to delay environmental changes by 1 additional unit for each subsequent lane. |
inverted-asynchrony | No | [0, 0.1, 0.2, 0.3, 0.4, 0.5] | Percent-as-decimal of total lanes (locales) where all values are inverted. |
agent-mem-length | No | [3, 4, 5, 6, 7, 8, 9] | Number of past problem values each agent can store. |
weight-others-input | Yes | [0, 0.25, 0.5, 0.75] | Weight agent puts on advice from other agents versus their own memory. A weight of 0 is equivalent to ignoring advice. If agents can adjust the weight, this variable is the starting weight. |
weight-adj-increment | No | [0, 0.1, 0.2, 0.3, 0.4, 0.5] | How much an agent can adjust their input weight in one time step. |
Results
We ran 20 simulations for each unique parameter set, then calculated the mean score for those runs as the score of a typical run. The variance in score among the 20 runs per parameter set does not substantially change the results of our analysis (see Supplementary Material). To analyze how hierarchy affects performance, we group typical runs into pairs that are identical in their parameter settings except for the presence or absence of a manager in the group, then subtract the no-hierarchy group score from the hierarchy group score. The resulting score is the difference hierarchy makes for that set of conditions: a negative score indicates that hierarchy fares worse, zero indicates no difference, and a positive score indicates that hierarchy fares better than the equivalent no-hierarchy condition (see Figures 4- 7). We are most interested in the relative differences of the groups, which is most easily seen with larger groups: we use groups of 10 workers for this analysis. Smaller groups have the same differences but with smaller spread (see Supplementary Material).
Hierarchy is generally better across all environments, but with lesser advantage in more disordered environments
Our first research question asks in which environmental conditions hierarchy affects performance. Broadly, hierarchy performs better than no-hierarchy in most environments, with the caveat that specific parameters do matter. In 87% of cases, hierarchy does better than no-hierarchy; hierarchy does worse than no-hierarchy in 6% of conditions, and in 7% of conditions hierarchy makes no difference. If those replication-sets with bounds that straddle zero are considered as zero instead of their mean, hierarchy does better in 75% of cases, worse in 4%, and makes no difference in 15% (see Supplementary Material).
The two types of environmental asynchrony have different effects on performance (Figure 4). Delayed-asynchrony, a source of mild disorder in the environment, does not reduce the effectiveness of hierarchies; if anything, it gives hierarchy slightly more of a consistent advantage over no-hierarchy within most parameter combinations. However, generating stronger disorder (inverted asynchrony) in the environment decreases hierarchy’s advantage to groups.
Since the manager communicates information about other locales, that manager should be most useful when environments are synchronous, because then the information they provide is highly relevant to each worker’s own locale. If a worker puts weight on manager input in these situations, they will switch their solution to match the environmental problem sooner than they would based on their own memory of local states. This faster switching enables workers to score higher when the environment is synchronous.
Conversely, in environments with less synchrony, individual agents benefit little from information about other locales, because they are not similar. In these environments, manager’s input about overall conditions is less pertinent to each worker’s locale. Delayed asynchrony conditions lead to tighter distributions, because the workers on the leading points of environmental transitions cannot switch quickly based on manager input. By the leading points when mean when their locale is changing and yet the overall environment is in the previous state; see the top lane of the delayed environments in Figure 1. Two factors are at play: one reducing group score, and one improving it. First, groups do not score as highly because, unlike the synchronous condition, workers on the leading point lack a clear signal to switch quickly when their locale changes. Second, while workers on the leading point of the environmental transition are slower to switch to the right solution, manager input helps those workers whose locales are in the middle of the transition timing switch more quickly. Thus, while workers at these leading points score less, their information about the environment, facilitated through the manager, enables the group overall to react more quickly to transitions and score more than no-hierarchy groups.
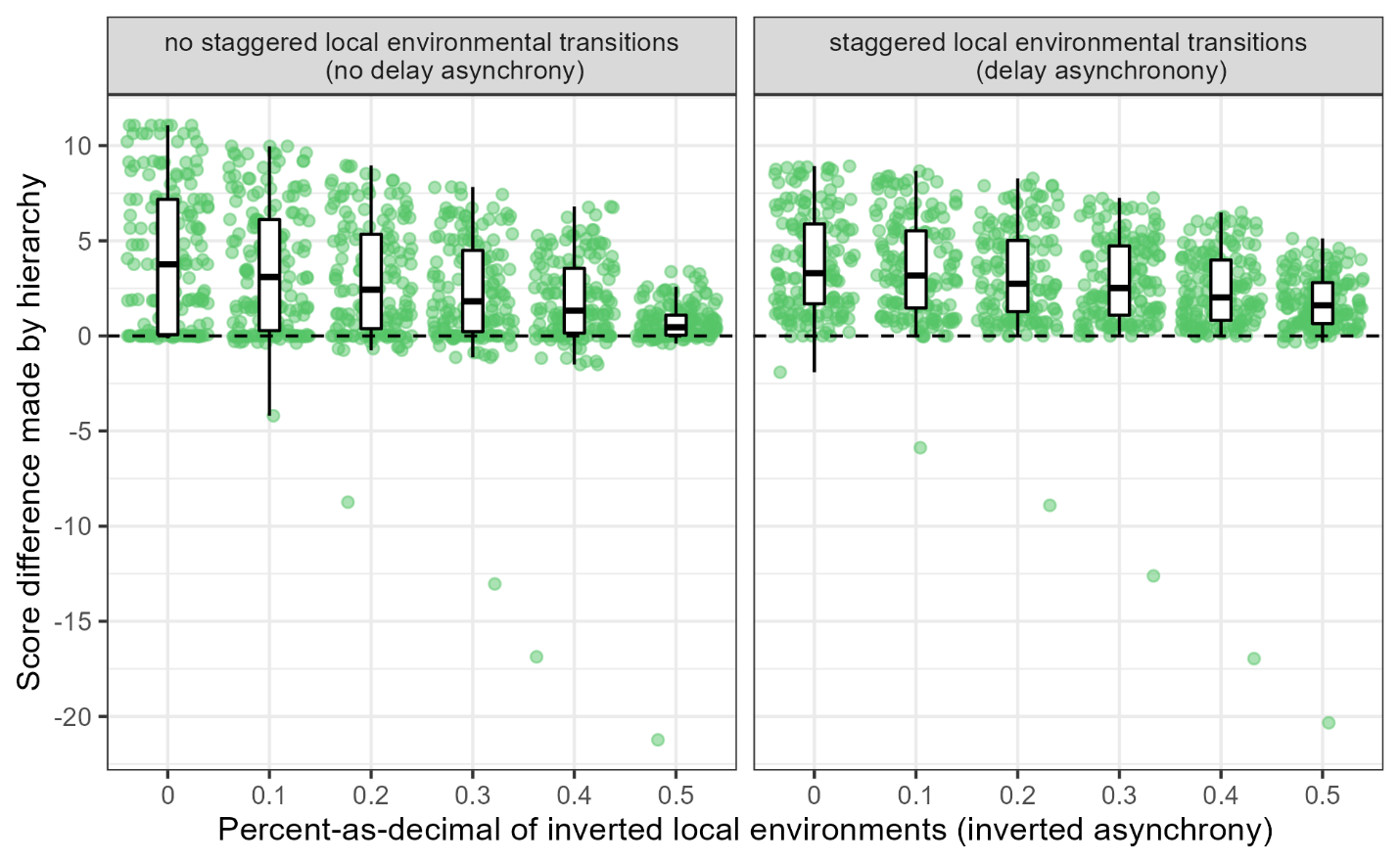
Groups generally perform better when individuals can adjust the weight they give to the manager’s input
Our second research question inquires into the importance of autonomy, modeled as agents adjusting the weight they put on the manager’s input in response to the perception of its relevance to their local conditions. Hierarchical groups with agents with this autonomy do better in nearly all conditions than non-hierarchical groups (Figure 5), including in highly asynchronous environments (those where half the lanes in the environment are inverted). Larger weight adjustment increments (e.g., 0.5) have higher standard deviations in performance, but lower increments are tighter and more skewed toward lower performance. Groups that cannot adjust the weight given to manager input earn some of the lowest scores in the simulation.
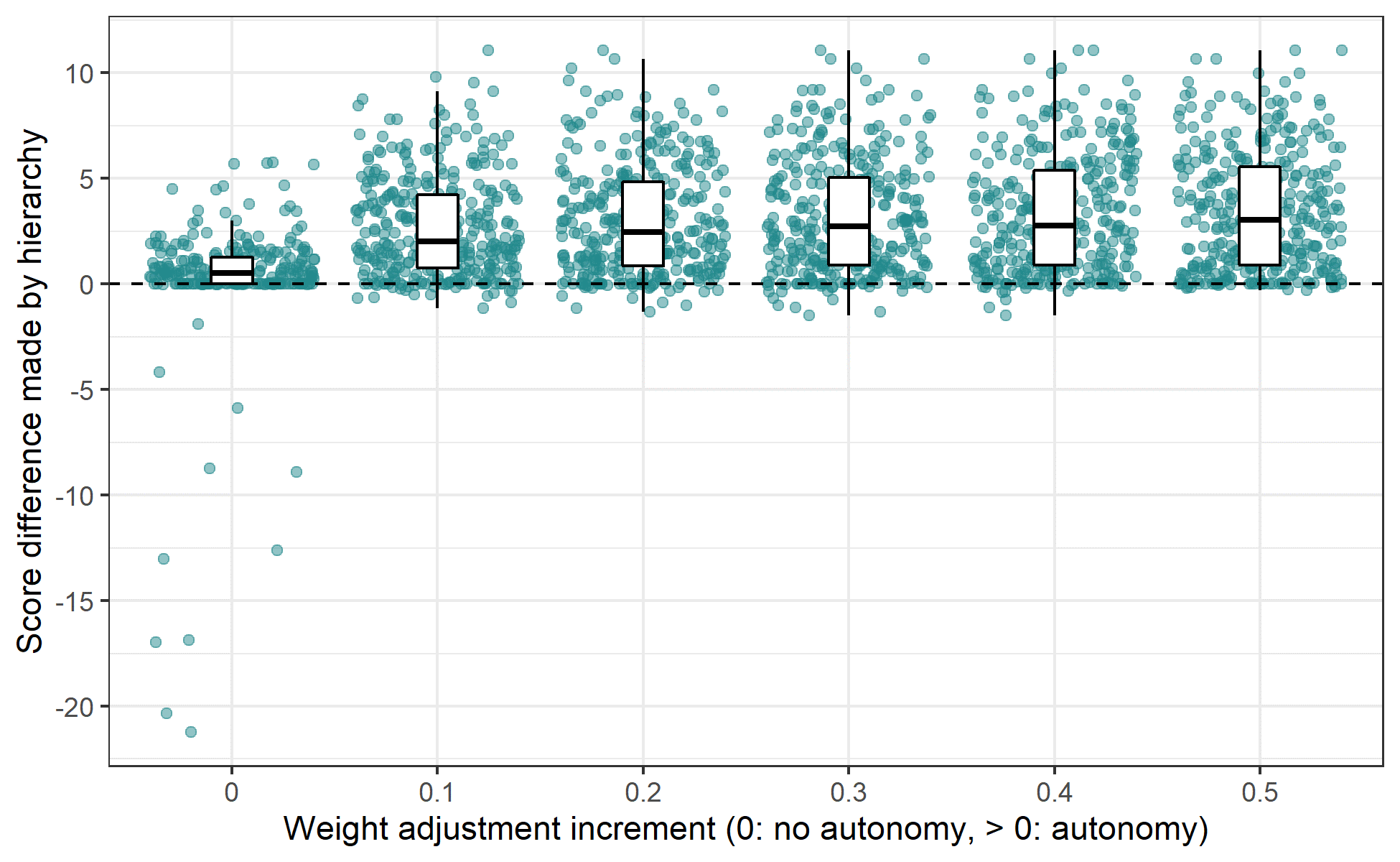
Groups benefit from starting with more weight on the manager’s input even in moderately asynchronous environments
Our third research question asks about the importance of workers placing weight on the manager’s input versus their own experience. Workers without autonomy are the primary focus of this question, as workers with autonomy can adjust the weight given to their manager over the run regardless of the starting weight. In the no-autonomy condition, most hierarchical groups with a heavier starting weight (.75 vs. .5 or less) on the manager’s input have a slight advantage over non-hierarchical groups, although this advantage lessens in more disordered environments (Figure 6). Those hierarchical groups with low memory (only 3 memory slots) perform much worse than non-hierarchical groups, especially in more disordered environments.
In the autonomy condition, hierarchical groups perform better when starting with a higher weight on the manager’s input, with one exception: again, the poor performance of groups in the low memory condition of 3 memory slots (left-column panels of Figure 7). As expected, for groups with autonomy, how much workers can adjust the weight matters more than the starting value.
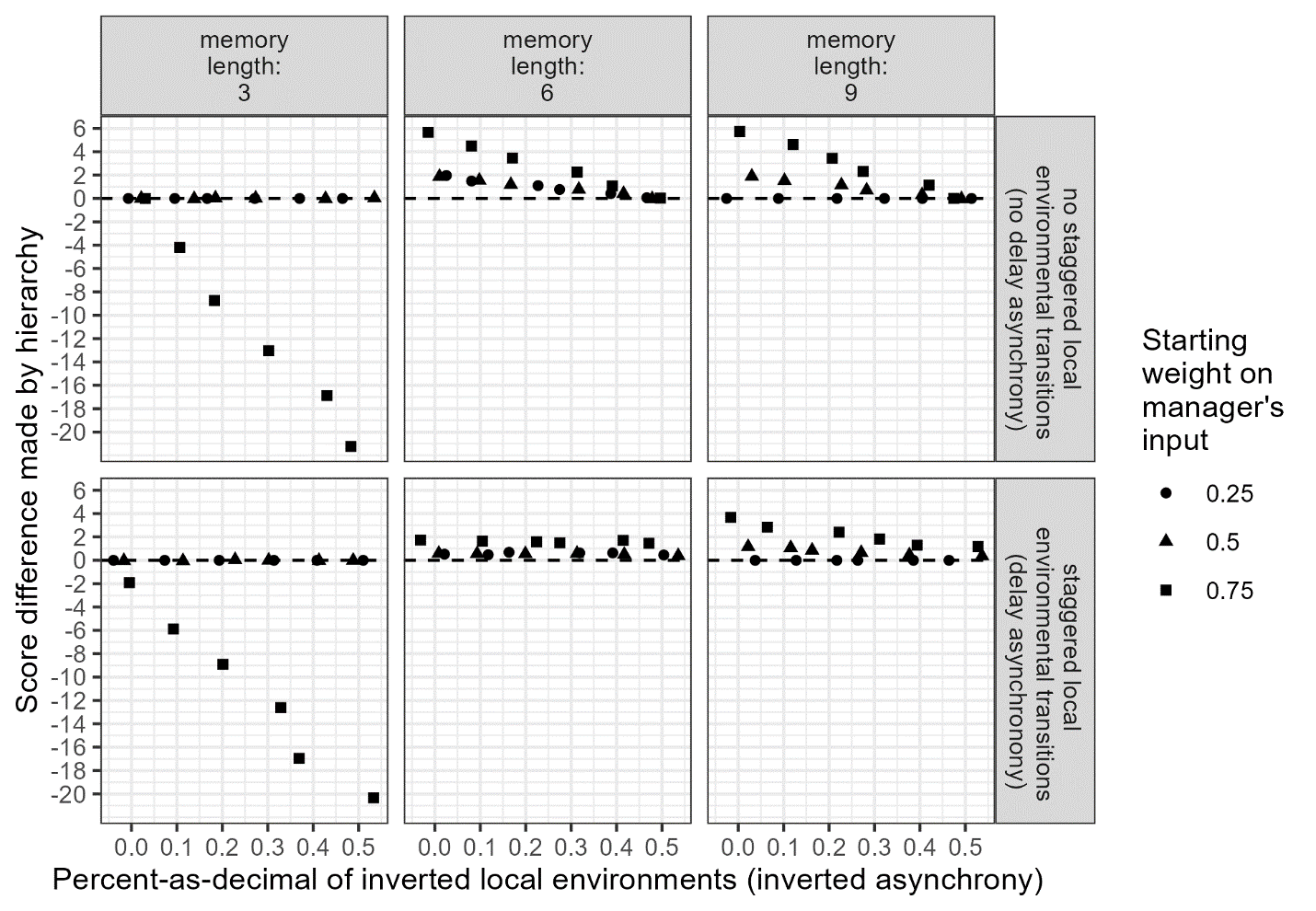
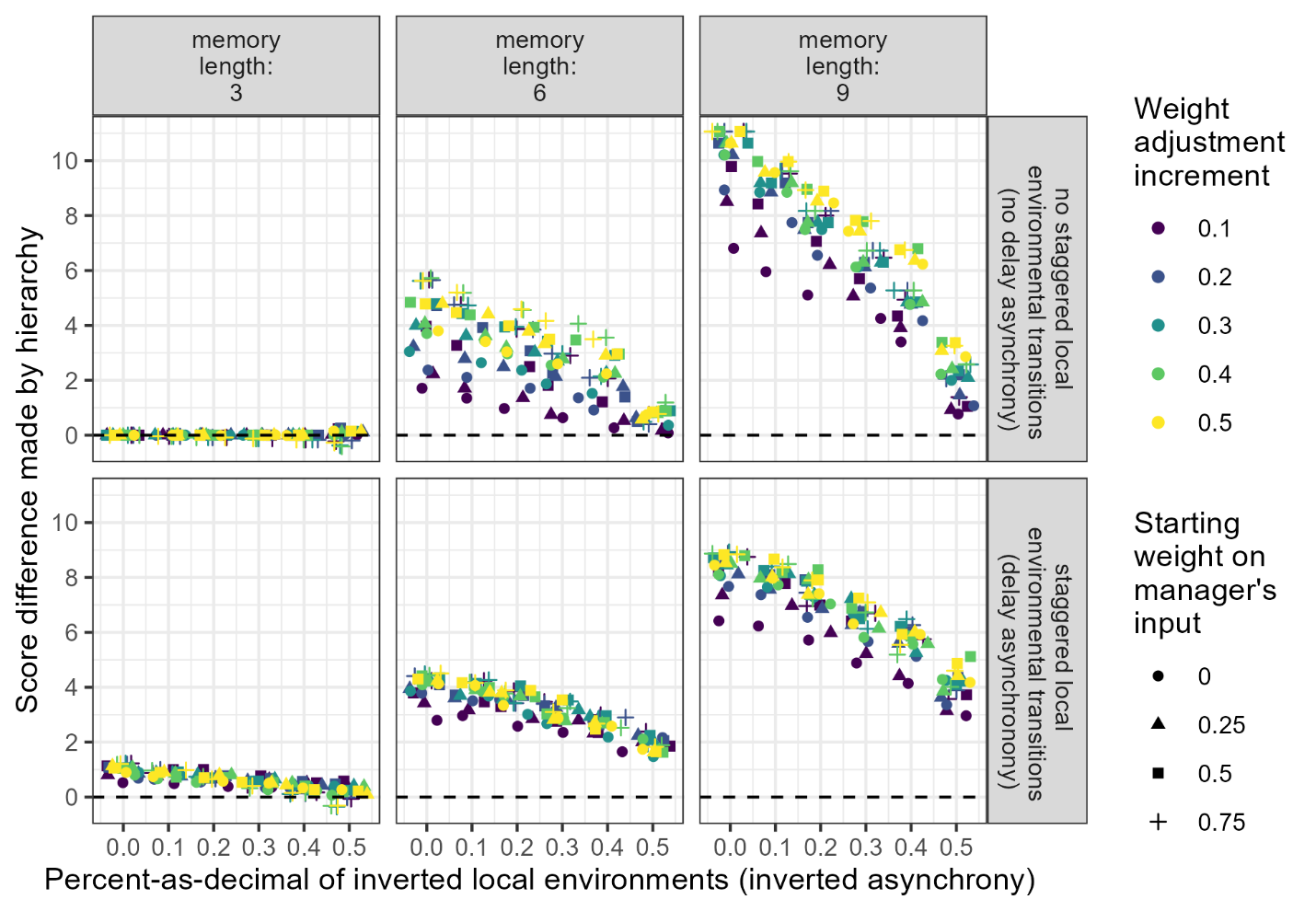
Memory length matters, especially in more synchronous environments
Although we did not initially target memory as a factor to explore in the model, our analysis shows it played a substantial role. Longer memory is correlated with better performance, as seen in Figure 6. Yet, larger memory is necessary, but not alone sufficient, for higher group performance in all environments. The biggest advantages are gained by high-worker-autonomy hierarchical groups in synchronous environments (low values on the x-axis in Figure 7). No-autonomy hierarchical groups still gain some advantage from increased memory, but far less (Figure 6). While in highly asynchronous environments the gains are not as great, larger memories combined with autonomy enable hierarchical groups some advantage; without autonomy, increased memory gives no advantage to hierarchical groups at all in asynchronous environments.
Discussion
Our model suggests that command hierarchies may be so common because they provide benefits in many different environments. It also suggests that the tension hierarchies often contain – a tension between workers and management over the right understanding of the environment – is inherent in most environments with some local variability. This tension is rooted in the two different scales of views that inevitably play out in individual and organizational decision-making. We demonstrate that considering hierarchical organizations within changing environments can substantially improve our understanding of when and why we make them.
We show that organizational hierarchies – with the right relations between management and workers – can actually benefit from the inherent computational dilemma caused by the difference between the view from the ground versus the view from the top. However, this dilemma and resulting tension is not resolved (it is unresolvable); it is only managed by the structure of relations in the organization itself (and not just the managers). This tension inherent in hierarchy questions and complicates the standard story of beneficial hierarchy – that of asymmetrical influence that provides a way to reduce conflict and generate or impose consensus (e.g., Tabary 1991; Bunderson et al. 2015; Perret et al. 2020). Our story aligns, instead, with a lesser-known tradition of seeing hierarchy as an element of collective learning (this has been proposed in economics since at least Frank Knight and Ronald Coase; see Foss 1996). These two perspectives are complementary, not mutually exclusive. However, we emphasize that our model shows not a coordination or consensus problem, but a collective computation resulting from individual/local and managerial/global views and the decision-making that results.
We add a different and complementary notion of adaptation in organizations in relation to their environments. Generally, adaptive organizations provide employees with flexibility to tailor their tasks to local information (Dessein & Santos 2006). Previous work on adaptation tends to focus on how organizations simultaneously explore and exploit an environment (O’Reilly & Tushman 2008) or how workers labor toward the same goal via task bundling (e.g., Dessein & Santos 2006); instead, our workers have different local goals that are just similar enough across the organization to enable workers to benefit from a manager’s aggregated “high-level” information. This different dynamic adds to our overall understanding of how organizations adapt, and demonstrates another way that effective organizational decision-making depends on the collocation of uncovered information and the right to act on that information (Chang & Harrington 2006; Ostrom 2005). Most real organizations operate in environments that have some local variation that could feasibly lead to differences in worker-level and manager-level views of the landscape, whether it be physical, legal, financial, or otherwise. The agents in our model, while stylized as workers, could be just as easily stylized as local offices within a multinational corporation or a national government trying to do their best while balancing the advisements of a head office. This model thus informs debates about centralized versus decentralized organizational control that seek more insight into the complexity involved with organizational forms (Beunen & Opdam 2011; Mualam 2018).
Organizations operating in varied environments may inherently be managing some unavoidable spatial and temporal scale conflict in their organizational decision-making. Indeed, internal asynchrony is common for organizations adapting and learning (Launis & Pihlaja 2007). Future research would benefit from considering how these computational dilemmas may affect broader spatial and temporal mismatches (Cumming et al. 2006). Better understanding of the drivers of these mismatches is important for resource management (e.g., Sayles & Baggio 2017), as well as public administration (e.g., Durose 2009) and business (e.g., Fayezi et al. 2014).
More abstractly, the managers help the workers by coarse-graining the environment, providing some system-level knowledge for their computations. In systems generally, coarse-grained variables act as better predictors of the local system than the states of fluctuating micro-components (Flack 2017). In systems like our hierarchical groups, this course-graining forms part of the organizational memory. In our relatively simple model, we see two important implications for future research considering organizational memory from a computational perspective: first, coarse-grained views and local views can be different subsystem memories that are useful when processed into decision-making; and second, worker autonomy plays a key role in the usefulness of organizational memory to performance. Put a different way, one may mistakenly discount the utility of different memory subsystems that conflict with one another if the decision-making processes are not considered. Decision-making processes – which arguably include autonomy as a key factor – may turn this tension into beneficial organizational learning and adaptation. We see promise in investigating organizational memory with this computational lens, and, more broadly, connecting organizational dynamics to more general systems dynamics. Both can shed light on why human organizational methods are effective, and why they persist.
Limitations and future directions
Agent-based models of human organizations usually include environments, agents, and social relations among agents, but not all can be made sophisticated and still keep analysis feasible. In this case, we tried to keep all three as simple as possible while employing the minimum factors necessary for a command hierarchy in a changing environment. A more complex model with more sophisticated agents and social relations may well have hidden or muddled the role of the environment in affecting group performance. We view this simplicity as a strength of the model, but it also signals directions for future work: how more sophisticated environments, agents, or networks may extend or enrich these findings. Our model uses two categorical states for local conditions, and could be extended to more states, or even a continuous environment that the agents experience discretely. The agents themselves make perfect decisions with perfect (although limited) memory, raising the question of how noise, imperfection, and incompetence may affect the usefulness of hierarchy. The organizational networks are simple, too, being only one manager and a set of workers. The benefits of hierarchy in our environments may shift with larger organizations containing divisions and layers of middle management, as larger companies so often have.
Although our model does not include social power relations, the sensitivity of group performance to worker autonomy in most environments leaves the door open for extending the model to investigate power dynamics, especially those related to self-serving versus group-serving behaviors (Anderson & Brion 2014). This goes beyond decision-making to connect to other areas in organization science; for example, critical perspectives on organizational memory and power can leverage a computational approach to consider how the type of environment may affect the organizational impacts of power relations. More broadly, organizational memory studies face a need to enhance standard views of organizational memory with considerations of power dynamics (Foroughi et al. 2020) – a computational approach can help bridge perspectives investigating the role of power.
Although memory was not our focus in this model, a derivative of this work could investigate organizational memory specifically. The need is there: organizational memory studies currently lack methods for finding key boundary conditions for organizational remembering and forgetting, and this is an important objective for the field (Foroughi et al. 2020). Relatedly, the groups in our model also seem to benefit from very simplistic onboarding of environmental knowledge. A higher starting weight on the manager’s input seems to help fill in some of the workers’ initial lack of experience. As expected, this supplemental "lateral" experience is more valuable in more synchronous environments. Organizational memory, similar to adaptation, needs to consider different types of challenges, from coordination to the high-view-low-view dilemma we show here.
This inherent tension between workers and managers, rooted in environmental change, may or may not be an important factor in any particular organization’s context. However, with our findings in mind, we hope that researchers who seek to understand real, messy organizations can at least be aware, if not account for, this particular tension at the heart of our finding. Even if other organizational factors outweigh this factor in most situations –itself a possibility in need of future testing – the role it plays in real organizations remains an important empirical inquiry.
Lastly, we focus only on command hierarchies in this paper rather than rank or nested hierarchies. We acknowledge that, in reality, people inhabit organizations and social relations that simultaneously exhibit all three types of hierarchy in overlapping and interacting layers. In rich, messy social reality, no pure form of hierarchy exists, but this should not deter us from trying to understand the dynamics of one type of hierarchical relation. If anything, understanding the constituents will help us understand the whole. This hierarchical model may thus act as a building block for, or bridge to, models that can exhibit traits of panarchy (Cosens & Gunderson 2018; Holling 2001), heterarchy (Crumley 1995; Cumming 2016), and/or polycentricity (Aligica & Tarko 2012; McGinnis & Ostrom 2012) that we see in real social systems.
Conclusion
We demonstrate that command hierarchies bestow advantages to organizations dealing with environmental changes across local conditions. However, in most environments, there are only small benefits (and sometimes catastrophic losses) when managers directly control workers and give them no say; higher performance results from workers receiving manager input while also having some autonomy in decision-making. Hierarchical organizations that employ this strategy can outperform non-hierarchical organizations in even highly varied environments where local environmental changes occur at different times across the organization. Thus, hierarchical organizations can still be adaptable organizations if workers have sufficient autonomy. For those environments with high degrees of synchrony among local conditions, hierarchies are able to generate the greatest performance gain over non-hierarchies. In maintaining or modifying organizations, members should not assume that tensions between ground-level workers and managers are obvious signs of dysfunction; these tensions may be doing important work that enhances the performance of the organization.
We make two broad contributions to understanding the functional benefits of hierarchy and structure in organizations. First, we show the importance of considering the local environments (in both space and time) over which an organization operates; different environments may enable and/or constrain the effectiveness of organizational structures, making hierarchy more or less useful. Second, we show that, in hierarchies, the inevitable, unresolvable tension between workers and management on the “right” view of an uncertain and changing environment can benefit organizations as a whole, if the organization has the right combination of structure and culture that enables manager input to coexist with some worker autonomy.
Supplementary Material
The model specification (ODD protocol), model code, and additional analysis can be found at: https://www.comses.net/codebase-release/42dc5eeb-b3e2-483a-b0b0-8c42e9f313fc/.References
ACHESON, J. (2011). Ostrom for anthropologists. International Journal of the Commons, 5(2), 319–339.
ALIGICA, P. D., & Tarko, V. (2012). Polycentricity: From Polanyi to Ostrom, and beyond. Governance, 25(2), 237–262. [doi:10.1111/j.1468-0491.2011.01550.x]
ANDERSON, C., & Brion, S. (2014). Perspectives on power in organizations. Annual Review of Organizational Psychology and Organizational Behavior, 1(1), 67–97. [doi:10.1146/annurev-orgpsych-031413-091259]
ARGOTE, L., & Miron-Spektor, E. (2011). Organizational learning: From experience to knowledge. Organization Science, 22(5), 1123–1137. [doi:10.1287/orsc.1100.0621]
BALDWIN, R., & Freeman, R. (2022). Risks and global supply chains: What we know and what we need to know. Annual Review of Economics, 14(1), 153–180. [doi:10.1146/annurev-economics-051420-113737]
BALLIET, D. (2010). Communication and cooperation in social dilemmas: A meta-Analytic review. Journal of Conflict Resolution, 54(1), 39–57. [doi:10.1177/0022002709352443]
BAS, J., & Sebastian-Galles, N. (2021). Infants’ representation of social hierarchies in absence of physical dominance. PLoS One, 16(2), e0245450. [doi:10.1371/journal.pone.0245450]
BEUNEN, R., & Opdam, P. (2011). When landscape planning becomes landscape governance, what happens to the science? Landscape and Urban Planning, 100(4), 324–326. [doi:10.1016/j.landurbplan.2011.01.018]
BOYD, R., Richerson, P. J., & Henrich, J. (2011). The cultural niche: Why social learning is essential for human adaptation. Proceedings of the National Academy of Sciences, 108(2), 10918–10925. [doi:10.1073/pnas.1100290108]
BUNDERSON, J. S., van der Vegt, G. S., Cantimur, Y., & Rink, F. (2015). Different views of hierarchy and why they matter: Hierarchy as inequality or as cascading influence. Academy of Management Journal, 59(4), 1265–1289. [doi:10.5465/amj.2014.0601]
CHAFFIN, B., Gosnell, H., & Cosens, B. (2014). A decade of adaptive governance scholarship: Synthesis and future directions. Ecology and Society, 19, 3. [doi:10.5751/es-06824-190356]
CHANG, M. H., & Harrington, J. E. (2000). Centralization vs decentralization in a multi-Unit organization: A computational model of a retail chain as a multi-Agent adaptive system. Management Science, 46(11), 1427–1440. [doi:10.1287/mnsc.46.11.1427.12085]
CHANG, M. H., & Harrington, J. E. (2006). Agent-Based models of organizations. In L. Tesfatsion & K. L. Judd (Eds.), Handbook of Computational Economics (pp. 1273–1337). Amsterdam: Elsevier. [doi:10.1016/s1574-0021(05)02026-5]
COLOMBO, M. G., & Delmastro, M. (2004). Delegation of authority in business organizations: An empirical test. The Journal of Industrial Economics, 52(1), 53–80. [doi:10.1111/j.0022-1821.2004.00216.x]
COOREN, F., Kuhn, T., Cornelissen, J. P., & Clark, T. (2011). Communication, organizing and organization: An overview and introduction to the special issue. Organization Studies, 32(9), 1149–1170. [doi:10.1177/0170840611410836]
COSENS, B., & Gunderson, L. (2018). An introduction to practical panarchy: Linking law, resilience, and adaptive water governance of regional scale social-Ecological systems. In B. Cosens & L. Gunderson (Eds.), Practical Panarchy for Adaptive Water Governance: Linking Law to Social-Ecological Resilience (pp. 1–16). Cham: Springer International Publishing. [doi:10.1007/978-3-319-72472-0_1]
CRUMLEY, C. L. (1995). Heterarchy and the analysis of complex societies. Archaeological Papers of the American Anthropological Association, 6(1), 1–5. [doi:10.1525/ap3a.1995.6.1.1]
CUMMING, G. S. (2016). Heterarchies: Reconciling networks and hierarchies. Trends in Ecology & Evolution, 31(8), 622–632. [doi:10.1016/j.tree.2016.04.009]
CUMMING, G. S., CUMMING, D. H. M., & Redman, C. L. (2006). Scale mismatches in social-ecological systems: Causes, consequences, and solutions. Ecology and Society, 11(1), 14. [doi:10.5751/es-01569-110114]
DESSEIN, W., & Santos, T. (2006). Adaptive organizations. Journal of Political Economy, 114(5), 956–995. [doi:10.1086/508031]
DUNCAN, G. (2021). How to Rule: The Arts of Government from Antiquity to the Present. London: Routledge.
DUROSE, C. (2009). Front-Line workers and ’Local Knowledge’: Neighbourhood stories in contemporary UK local governance. Public Administration, 87(1), 35–49. [doi:10.1111/j.1467-9299.2008.01737.x]
DUROSE, C. (2011). Revisiting Lipsky: Front-Line work in UK local governance. Political Studies, 59(4), 978–995. [doi:10.1111/j.1467-9248.2011.00886.x]
ECKHARD, S. (2021). Bridging the citizen gap: Bureaucratic representation and knowledge linkage in (international) public administration. Governance, 34(2), 295–314. [doi:10.1111/gove.12494]
EPSTEIN, J. M. (2003). Growing adaptive organizations: An agent-Based computational approach. Santa Fe Institute. Available at: https://sfi-edu.s3.amazonaws.com/sfi-edu/production/uploads/sfi-com/dev/uploads/filer/94/43/94434753-926b-4201-9cc7-f269de552fce/03-05-029.pdf .
ERTAC, S., & Gurdal, M. Y. (2019). Preference communication and leadership in group decision-making. Journal of Behavioral and Experimental Economics, 80, 130–140. [doi:10.1016/j.socec.2019.03.004]
FAYEZI, S., Zutshi, A., & O’Loughlin, A. (2014). Developing an analytical framework to assess the uncertainty and flexibility mismatches across the supply chain. Business Process Management Journal, 20(3), 362–391. [doi:10.1108/bpmj-10-2012-0111]
FIEDLER, M., & Welpe, I. (2010). How do organizations remember? The influence of organizational structure on organizational memory. Organization Studies, 31, 4. [doi:10.1177/0170840609347052]
FLACK, J. C. (2017). Coarse-graining as a downward causation mechanism. Philosophical Transactions of the Royal Society A: Mathematical, Physical and Engineering Sciences, 375(2109), 20160338. [doi:10.1098/rsta.2016.0338]
FOROUGHI, H., Coraiola, D. M., Rintamäki, J., Mena, S., & Foster, W. M. (2020). Organizational memory studies. Organization Studies, 41(12), 1725–1748. [doi:10.1177/0170840620974338]
FOSS, N. J. (1996). The “Alternative” theories of Knight and Coase, and the modern theory of the firm. Journal of the History of Economic Thought, 18(1), 76–95. [doi:10.1017/s1053837200002960]
GLOWACKI, L., & von Rueden, C. (2015). Leadership solves collective action problems in small-scale societies. Philosophical Transactions of the Royal Society B: Biological Sciences, 370(1683), 20150010. [doi:10.1098/rstb.2015.0010]
GREER, L. L., de Jong, B. A., Schouten, M. E., & Dannals, J. E. (2018). Why and when hierarchy impacts team effectiveness: A meta-analytic integration. Journal of Applied Psychology, 103(6), 591–613. [doi:10.1037/apl0000291]
GREVE, H. R. (2017). Organizational Learning and Adaptation. Oxford: Oxford University Press.
GUINOTE, A., Cotzia, I., Sandhu, S., & Siwa, P. (2015). Social status modulates prosocial behavior and egalitarianism in preschool children and adults. Proceedings of the National Academy of Sciences, 112(3), 731–736. [doi:10.1073/pnas.1414550112]
GUPTA, J. (2008). Global change: Analysing scale and scaling in environmental governance. In O. Young, L. King, & H. Schroeder (Eds.), Institutions and Environmental Change: Principal Findings, Applications, and Research Frontiers (pp. 225–258). Cambridge, MA: The MIT Press. [doi:10.7551/mitpress/9780262240574.003.0007]
HALEVY, N., Chou, E. Y., & Galinsky, A. D. (2011). A functional model of hierarchy: Why, how, and when vertical differentiation enhances group performance. Organizational Psychology Review, 1(1), 32–52. [doi:10.1177/2041386610380991]
HARDT, H. (2018). Who matters for memory: Sources of institutional memory in international organization crisis management. The Review of International Organizations, 13(3), 457–482. [doi:10.1007/s11558-017-9281-4]
HASSAN, A., & Barber, S. J. (2021). The effects of repetition frequency on the illusory truth effect. Cognitive Research: Principles and Implications, 6(1), 38. [doi:10.1186/s41235-021-00301-5]
HOLLING, C. S. (2001). Understanding the complexity of economic, ecological, and social systems. Ecosystems, 4(5), 390–405. [doi:10.1007/s10021-001-0101-5]
HONG, S., & Lee, S. (2018). Adaptive governance and decentralization: Evidence from regulation of the sharing economy in multi-level governance. Government Information Quarterly, 35(2), 299–305. [doi:10.1016/j.giq.2017.08.002]
HOOPER, P. L. (2018). Ecological and social dynamics of territoriality and hierarchy formation. The Emergence of premodern states: New perspectives on the development of complex societies. Available at: https://www.academia.edu/37965866/Ecological_and_Social_Dynamics_of_Territoriality_and_Hierarchy_Formation. [doi:10.37911/9781947864030.05]
HORNE, L., DiMatteo-LePape, A., Wolf-Gonzalez, G., Briones, V., Soucy, A., & De Urioste-Stone, S. (2022). Climate change planning in a coastal tourism destination: A participatory approach. Tourism and Hospitality Research, 23, 4. [doi:10.1177/14673584221114730]
JANSSEN, M., Tyson, M., & Lee, A. (2014). The effect of constrained communication and limited information in governing a common resource. International Journal of the Commons, 8(2), 617–635. [doi:10.18352/bmgn-lchr.473]
KLUGE, A., & Gronau, N. (2018). Intentional forgetting in organizations: The importance of eliminating retrieval cues for implementing new routines. Frontiers in Psychology, 9, 2018. [doi:10.3389/fpsyg.2018.00051]
KUHN, T., & Ashcraft, K. L. (2003). Corporate scandal and the theory of the firm: Formulating the contributions of organizational communication studies. Management Communication Quarterly, 17, 20–57. [doi:10.1177/0893318903253421]
LANE, D. (2006). Hierarchy, complexity, society. In D. Pumain (Ed.), Hierarchy in Natural and Social Sciences (pp. 81–119). Berlin Heidelberg: Springer. [doi:10.1007/1-4020-4127-6_5]
LAUNIS, K., & Pihlaja, J. (2007). Changes in production concepts emphasize problems in work-related well-being. Safety Science, 45(5), 603–619. [doi:10.1016/j.ssci.2007.01.006]
LEVINTHAL, D. A. (1997). Adaptation on rugged landscapes. Management Science, 43(7), 934–950. [doi:10.1287/mnsc.43.7.934]
LIEBHOLD, A. M. (2012). Forest pest management in a changing world. International Journal of Pest Management, 58(3), 289–295. [doi:10.1080/09670874.2012.678405]
MCGINNIS, M. D., & Ostrom, E. (2012). Reflections on Vincent Ostrom, public administration, and polycentricity. Public Administration Review, 72(1), 15–25. [doi:10.1111/j.1540-6210.2011.02488.x]
MEAGHER, K., Orbay, H., & van Zandt, T. (2003). Hierarchy size and environmental uncertainty. In M. R. Sertel & S. Koray (Eds.), Advances in Economic Design (pp. 439–457). Berlin Heidelberg: Springer. [doi:10.1007/978-3-662-05611-0_24]
MEHRABI, Z., & Ramankutty, N. (2019). Synchronized failure of global crop production. Nature Ecology & Evolution, 3(5), 5. [doi:10.1038/s41559-019-0862-x]
MUALAM, N. (2018). Playing with supertankers: Centralization in land use planning in Israel - A national experiment underway. Land Use Policy, 75, 269–283. [doi:10.1016/j.landusepol.2018.03.019]
NISSEN, H. J., Damerow, P., & Englund, R. K. (1993). Archaic Bookkeeping: Early Writing and Techniques of Economic Administration in the Ancient Near East. Chicago, IL: The University of Chicago Press.
O’REILLY, C. A., & Tushman, M. L. (2008). Ambidexterity as a dynamic capability: Resolving the innovator’s dilemma. Research in Organizational Behavior, 28, 185–206. [doi:10.1016/j.riob.2008.06.002]
ORNSTEIN, J. T., Hammond, R. A., Padek, M., Mazzucca, S., & Brownson, R. C. (2020). Rugged landscapes: Complexity and implementation science. Implementation Science, 15(1), 85. [doi:10.1186/s13012-020-01028-5]
OSTROM, E. (2005). Understanding Institutional Diversity. Princeton, NJ: Princeton University Press.
PAPAZIAN, H. (2013). The central administration of the resources in the Old Kingdom: Departments, treasuries, granaries and work centers. In J. C. Moreno García (Ed.), Ancient Egyptian Administration (pp. 41–83). Leiden: Brill. [doi:10.1163/9789004250086_004]
PAVITT, C. (2018). The path to cooperative action during group social dilemmas: A literature review, set of propositions, and model describing how the opportunity to communicate encourages cooperation. Review of Communication Research, 6(1), 54–83. [doi:10.12840/issn.2255-4165.2018.06.01.016]
PERRET, C., Hart, E., & Powers, S. T. (2020). From disorganized equality to efficient hierarchy: How group size drives the evolution of hierarchy in human societies. Proceedings of the Royal Society B: Biological Sciences, 287(1928), 20200693. [doi:10.1098/rspb.2020.0693]
ROMANO, V., Lozano, S., & Fernández-López de Pablo, J. (2020). A multilevel analytical framework for studying cultural evolution in prehistoric hunter-gatherer societies. Biological Reviews, 95(4), 1020–1035. [doi:10.1111/brv.12599]
ROWLINSON, M., Booth, C., Clark, P., Delahaye, A., & Procter, S. (2010). Social remembering and organizational memory. Organization Studies, 31(1), 69–87. [doi:10.1177/0170840609347056]
SALLY, D. (1995). Conversation and cooperation in social dilemmas: A meta-Analysis of experiments from 1958 to 1992. Rationality and Society, 7(1), 58–92. [doi:10.1177/1043463195007001004]
SAYLES, J. S., & Baggio, J. A. (2017). Social-ecological network analysis of scale mismatches in estuary watershed restoration. Proceedings of the National Academy of Sciences, 114(10), 1776–1785. [doi:10.1073/pnas.1604405114]
SCHATZKI, T. R. (2006). On organizations as they happen. Organization Studies, 27(12), 1863–1873. [doi:10.1177/0170840606071942]
SCOTT, J. C. (1998). Seeing Like a State: How Certain Schemes to Improve the Human Condition Have Failed. New Have, CT: Yale University Press.
SCOTT, W. R., & Davis, G. F. (2007). Organizations and Organizing: Rational, Natural and Open Systems Perspectives. London: Routledge.
SICILIANO, M. D. (2015). Advice networks in public organizations: The role of structure, internal competition, and individual attributes. Public Administration Review, 74(4), 548–559. [doi:10.1111/puar.12362]
STUTE, M., Maass, M., Schons, T., Kaufhold, M. A., Reuter, C., & Hollick, M. (2020). Empirical insights for designing information and communication technology for international disaster response. International Journal of Disaster Risk Reduction, 47(10159), 8. [doi:10.1016/j.ijdrr.2020.101598]
TABARY, J. C. (1991). Hierarchy and autonomy. International Journal of General System, 18(3), 244–250.
THOMSEN, L. (2020). The developmental origins of social hierarchy: How infants and young children mentally represent and respond to power and status. Current Opinion in Psychology, 33, 201–208. [doi:10.1016/j.copsyc.2019.07.044]
VAN Zandt, T., & Radner, R. (2001). Real-time decentralized information processing and returns to scale. Economic Theory, 17(3), 545–575. [doi:10.1007/pl00004119]
YOCOM Kent, L. L., Fulé, P. Z., Brown, P. M., Cerano-Paredes, J., Cornejo-Oviedo, E., Cortés Montaño, C., Drury, S. A., Falk, D. A., Meunier, J., Poulos, H. M., Skinner, C. N., Stephens, S. L., & Villanueva-Díaz, J. (2017). Climate drives fire synchrony but local factors control fire regime change in northern Mexico. Ecosphere, 8(3), e01709. [doi:10.1002/ecs2.1709]
YOUNG, O., King, L., & Schroeder, H. (2008). Institutions and Environmental Change: Principal Findings, Applications, and Research Frontiers. Cambridge, MA: The MIT Press.
ZAFEIRIS, A., & Vicsek, T. (2017). Why we live in hierarchies: A quantitative treatise. arXiv preprint. Available at: http://arxiv.org/abs/1707.01744. [doi:10.1007/978-3-319-70483-8_7]
ZARAGOZA, M. S., & Mitchell, K. J. (1996). Repeated exposure to suggestion and the creation of false memories. Psychological Science, 7(5), 294–300. [doi:10.1111/j.1467-9280.1996.tb00377.x]