An Agent-Based Model for Assessing the Resilience of Drug Trafficking Organizations to Law Enforcement Interventions

and
Università Cattolica del Sacro Cuore di Milano, Italy
Journal of Artificial
Societies and Social Simulation 27 (3) 3
<https://www.jasss.org/27/3/3.html>
DOI: 10.18564/jasss.5430
Received: 17-Jul-2023 Accepted: 20-May-2024 Published: 30-Jun-2024
Abstract
The resilience and resistance of criminal networks, particularly drug trafficking organizations, remain crucial issues in contemporary society. Existing studies have unrealistically modelled law enforcement interventions and fail to capture the complexity of the adaptations of criminal networks. This study introduces MADTOR, the first agent-based model that examines the responses of drug trafficking organizations to different types of law enforcement interventions. MADTOR addresses previous research gaps by enabling more realistic simulations of law enforcement interventions, modeling adaptations by organizations based on real-world operations, and allowing comparisons of different interventions. To demonstrate the possible applications of MADTOR, we assess the impact of arresting varying proportions of members on the resilience of drug trafficking organizations. Our results reveal the disruptive impact of arresting even a few members, and a non-linear relationship between the share of arrested members and disruptive impact, with diminishing returns as the proportion increases. Surviving organizations face increasing recovery difficulties as more members are arrested. These findings contribute to the development of strategies for effective interventions against drug trafficking.Introduction
Despite decades of stringent policies against drug trafficking, the illicit drug trade remains one of the most pressing societal issues in the contemporary world. Law enforcement agencies worldwide dedicate significant efforts to identify and apprehend drug traffickers. While seizures and arrests may inflict losses on drug trafficking organizations, their profitability allows them to endure and recruit new members. However, these organizations operate within complex environments and to survive over time, they must confront, respond to, and adapt to changing conditions (Decker & Chapman 2008; Kleemans 2014; Morselli 2009b; Morselli & Roy 2008; Wood 2017). Moreover, due to the illegal nature of their activities, criminal networks are inherently vulnerable, leading them to evolve in response to internal and external factors (Ayling 2009; Bouchard 2007; Everton & Cunningham 2013; Morselli 2009b; Morselli & Petit 2007).
A growing body of literature on criminal network resilience demonstrates that drug trafficking organizations often withstand disruptions caused by law enforcement agencies by replacing members and modifying their operations, capitalizing on social relationships within their environment (Berlusconi 2013, 2022; Diviák et al. 2022; Fabiani & Behlendorf 2021; Morselli & Petit 2007). However, our understanding of the resilience of criminal networks remains limited. Many studies have overlooked the realistic modelling of law enforcement intervention, rather focusing on the impact of arresting a few selected members based on network metrics. Moreover, when studies have attempted to model the adaptation of criminal networks after law enforcement actions, they have relied on general network algorithms. These approaches fail to capture the complexity of real-world scenarios.
Agent-based modelling (ABM) is a valuable approach to bridge these knowledge gaps by modelling the organizational and operational adaptations of drug trafficking organizations in response to major law enforcement interventions. ABMs capture the complex dynamics and behavior of individual agents, but also how organizations recruit new members to replace those arrested, adjust their operational strategies and leverage social connections to maintain resilience (Diviák 2023).
To address these gaps, our study introduces MADTOR (Model for Assessing Drug Trafficking Organizations Resilience), the first ABM that examines the responses of drug trafficking organizations to different types of law enforcement interventions. MADTOR effectively addresses previous gaps through its key features, i.e., the simulation of law enforcement interventions includes simultaneous arrests of multiple actors based on investigation information, explicit modelling of adaptations made by drug trafficking organizations, incorporating observed reactions from a real-world police operation, and the ability to test different law enforcement interventions, enabling comparisons of their impact on network resistance and resilience. We have demonstrated the application of MADTOR by assessing how arresting varying proportions of members affects the resistance and resilience of drug trafficking organizations. Specifically, we aimed to answer questions regarding the impact on an organization’s survival, the number of active members and its financial resources.
Our experimental results demonstrate that the arrest of a few members can lead to the disruption of a considerable number of drug trafficking organizations. However, we also discovered a non-linear relationship between the share of arrested members and the disruptive impact, with diminishing returns as the proportion of arrested members increases. Furthermore, surviving organizations encounter increasing difficulties in their recovery as the percentage of arrested members grows. These findings could facilitate the development of targeted strategies that effectively combat drug trafficking, thus mitigating its detrimental societal consequences.
The remainder of this study is structured as follows: the next section provides background information on the resilience and resistance of criminal networks and highlights how our study addresses specific gaps in past research. Section 3 presents the MADTOR model, and the analytical strategy employed. In Section 4, we present the results of the application of MADTOR, assessing the impact of arresting different proportions of members on the resilience of drug trafficking organizations. The closing section discusses the results, acknowledges its limitations, and suggests directions for future research.
Background
The resilience of criminal networks
Criminal organizations, just as legitimate ones, operate in complex environments. They must face, react and adapt to changing conditions to survive over time (Decker & Chapman 2008; Kleemans 2014; Morselli 2009b; Morselli & Roy 2008; Wood 2017). However, criminal networks are exposed to specific vulnerabilities due to the illegal nature of their activities. This often leads them to evolve over time in response to internal and external factors (Ayling 2009; Bouchard 2007; Duijn et al. 2014; Everton & Cunningham 2013; Morselli 2009b; Morselli & Petit 2007). Internal factors can involve the recruitment or abandonment of members due to conflict or personal choice, group reorganization, or interaction with other organizations. External factors include illicit market evolutions or external shocks due to law enforcement interventions.
The concepts of resistance and resilience contribute to the analysis of how criminal organizations react to attempts of law enforcement disruption. Resistance refers to a system’s ability to withstand environmental disturbances without affecting its operations, while resilience involves actively adapting and coping with the disturbance (Prezelj & Doerfel 2017; Reghezza-Zitt et al. 2012). In a similar vein, Bakker et al. (2012) proposed a framework for differentiating two types of resilience in criminal networks. Robustness refers to the ability of a criminal network to sustain its functions and operations even under attack, demonstrating resistance. On the other hand, rebounding describes a criminal network’s capacity to withstand external shocks over time by transforming and adapting, demonstrating resilience.
Empirical study of criminal networks has significant challenges in observing how these networks respond to law enforcement interventions. Typically, police operations involve arresting most known members of a criminal group after extensive investigations, making it rare to observe cases where law enforcement has arrested a substantial portion, but not all the group, leading to a major disruption event (Berlusconi 2022; Morselli & Petit 2007). Despite these difficulties, research continues to analyze the disruption and resilience of criminal networks in order to understand their strategies and vulnerabilities. This knowledge can assist law enforcement in planning and implementing more effective interventions (Bouchard 2007).
Studies on criminal network disruption have examined the impact of the hypothetical removal of certain actors (Agreste et al. 2016; Castiello et al. 2017; Cavallaro et al. 2020; Duxbury & Haynie 2018; Morselli & Roy 2008; Wood 2017). They found that targeted removal of key actors, such as brokers, highly connected actors and members with significant human capital, is more effective than random removal. However, these studies often neglected the dynamic nature of networks and used simplified approaches that simulate the sequential removal of one or few actors based on network metrics. This approach fails to realistically operationalize law enforcement interventions, which typically involve the arrest of a greater number of suspects based on extensive investigations and evidence of their involvement in criminal activities (e.g., Calderoni 2012, 2014b; Berlusconi 2022). While occasional arrests may occur, they often target lower-level actors rather than key individuals. Research indicates that these occasional arrests have minimal impact on criminal networks (Calderoni 2014a, 2014b; Morselli 2010).
Other studies have considered criminal network reactions to these disruption attempts (see Carley 2006; Bright et al. 2017; Diviák 2023; Duijn et al. 2014; Duxbury & Haynie 2018, 2019; Keller et al. 2010). The findings of this research demonstrated that the inclusion of network adaptation significantly reduced the effectiveness of disruption attempts, highlighting the importance of understanding network dynamics. However, these studies often focused on removing a limited number of actors selected through network metrics, and employed general network algorithms to model adaptation, which may not fully capture the actual responses of real criminal networks to law enforcement operations.
Only a few studies have examined network resilience by observing actual organizational adaptations (see Morselli & Petit 2007; Berlusconi 2013; Diviák et al. 2022; Fabiani & Behlendorf 2021; Manzi 2019). These studies demonstrated the adaptability of criminal networks in response to law enforcement interventions, employing strategies such as replacing key members, reducing criminal activities, and altering operational methods. Furthermore, they also emphasized the role of preexisting social relations in enhancing criminal network resilience. However, these studies focused on specific real-world law enforcement interventions, neglecting the exploration of alternative intervention strategies or of different intensities for the same type of interventions. Thus, our understanding of whether the studied networks would have responded differently to various disruption strategies remains limited.
In conclusion, the current understanding of criminal network resistance and resilience suffers from three key gaps. Firstly, most studies have focused on the removal of a few actors based on network metrics, neglecting the fact that most law enforcement interventions arrest a greater number of individuals. Secondly, limited attention has been paid to the resilience strategies employed by criminal networks, often relying on general network algorithms. Thirdly, the few existing studies on actual organizational adaptations have not extensively compared different intervention strategies and intensities. These gaps in our knowledge hinder a comprehensive understanding of criminal network resistance and resilience.
The current study
This study has two main objectives: (i) to introduce MADTOR (Model for Assessing Drug Trafficking Organizations Resilience), the first ABM that examines the responses of drug trafficking organizations (DTOs) to different law enforcement interventions, and (ii) to demonstrate the practical application of MADTOR by assessing the effects of arresting different proportions of drug trafficking organizations’ members on the resistance and resilience of the criminal network.
Our study effectively addresses the three aforementioned gaps in understanding criminal network resistance and resilience. First, we simulated law enforcement interventions simultaneously arresting multiple actors, based on information available during investigations. Second, we explicitly modelled the reaction strategies of drug trafficking organizations based on observed reactions in a real-world police operation against a large-scale drug trafficking organization. Third, MADTOR allowed us to test different types and intensities of law enforcement interventions, facilitating comparisons of their impact on network resistance and resilience. Our focus on drug trafficking is driven by its global significance, ongoing efforts to combat it, and existing evidence on the resistance and resilience of criminal networks involved in this activity (Berlusconi 2013; Ekici 2014; Kenney 2007; Kostelnik & Skarbek 2013; Manzi 2019; Morselli & Petit 2007; Swanström 2007, 2004; UNODC 2022; Von Lampe 2012; Williams 1998).
ABMs are suitable to study crime, as it allows for experimentation without the ethical, data protection, safety and security concerns associated with real-world experiments (Calderoni et al. 2022; Groff et al. 2019). ABMs are valuable tools for policy evaluation, testing of criminological theories, developing strategies to combat criminal activities and implement effective crime prevention policies. Large-scale real-world experiments and tests are often infeasible due to these concerns (Calderoni et al. 2022; Groff & Mazerolle 2008). To overcome them, ABMs have gained popularity in various crime-related applications. These include predicting urban crime patterns and evaluating different preventive measures (e.g., Groff 2007; Bosse & Gerritsen 2010; Wang et al. 2014; Weisburd et al. 2017; Zhu & Wang 2021), studying the recruitment and withdrawal dynamics in organized crime (e.g., Calderoni et al. 2022; Acconcia et al. 2014), and countering protection racketeering criminal groups (e.g., Elsenbroich & Badham 2016; Elsenbroich 2017; Nardin et al. 2016; Székely et al. 2018).
A few studies used ABMs to explore the macro and micro dynamics of drug trafficking and dealing (e.g., Magliocca et al. 2019, 2022; Dray et al. 2008; Romano et al. 2009). Most of these studies examined macro or micro level drug trafficking but did not model the specific adaptation strategies of drug traffickers. To the best of our knowledge, only Duxbury & Haynie (2019), Duxbury & Haynie (2020) and Diviák (2023) applied ABMs to assess the resilience of different types of criminal networks. Duxbury & Haynie (2019) examined the robustness and recovery time of criminal networks involved in different criminal activities.
However, this study modelled network relational patterns assuming stochastic probabilities for tie formation and actor recruitment, and it omitted the modelling of the crime commission processes. Secondly, it simplified disruption strategies, removing only one member at a time based on network metrics unavailable to law enforcement agencies during investigations. Thirdly, it lacked a baseline scenario and initiated the first disruption event at time 0, making it difficult to establish whether the observed trends were attributable to the disruption or if they could have occurred naturally. Lastly, the study’s outcome measures focused on the impact on relational patterns, disregarding the groups’ ability to sustain their activities (Duxbury & Haynie 2019).
In a second study, the same authors explored the effects of disruption attempts on drug exchanges over the darknet, which has distinct characteristics compared to offline drug trafficking (Duxbury & Haynie 2020). In a recent study, Diviák (2023) examined network disruption and recovery mechanisms in a street gang co-offending network. The study highlighted the potential for network adaptations to mitigate the impact of law enforcement interventions. However, it primarily focused on general co-offending relations within street gangs. Overall, while prior research generated valuable insights on the dynamics of criminal network resilience, no simulation study has yet explored more complex real-world scenarios with a specific focus on drug trafficking networks.
In conclusion, this study aims to introduce MADTOR and demonstrate its practical application in evaluating the impact of arresting different numbers of members on the resistance and resilience of drug trafficking organizations. In particular, we address the following questions: What is the impact of arresting varying proportions of members on the resistance and resilience of a drug trafficking organization? Specifically, how does it affect the survival of the group, the number of active members and the organization’s revenues? Previous research suggests that drug trafficking organizations, driven by the pursuit of profits, are capable of withstanding certain adversities and losses. Operating in a hostile environment, they are cognizant of the risks associated with their illicit activities and they factor in losses inherent to the trade when making decisions (Basu 2014; Iyavarakul 2021; Kleemans 2014). This means that, in the MADTOR simulations, drug trafficking organizations should absorb the occasional arrest of few members as a standard cost of engaging in illegal activities (resistance). However, for the arrest of a greater number of members, the entire organization’s survival may be jeopardized. At this point, drug traffickers may need to significantly adapt their operations to maintain continuity (resilience). In some cases, certain organizations may lack the necessary economic or human resources to adapt effectively, resulting in the permanent disruption of the group (Bakker et al. 2012; Butera 2009; Carpenter et al. 2001). While MADTOR allows for testing various additional scenarios, we will explore these further experiments in future research to maintain brevity.
Methodology
Data sources
The primary source of qualitative and quantitative information to empirically inform MADTOR was the Operation Beluga pretrial court order, a judicial document spanning 984 pages (Tribunale di Napoli 2013). Court orders include many details about the suspects, their criminal activities and their organization, providing in-depth information that is also valuable for research purposes, as evidenced by several studies relying on such sources (Bright et al. 2015; Calderoni 2012, 2014a; Morselli 2009a). Operation Beluga resulted from a 5-year investigation into the Camorra’s Di Lauro clan operating in Naples between the end of 2007 and the spring of 2013. Initially led by drug trafficker Paolo Di Lauro, the clan exerted control over the Secondigliano and Scampia neighborhoods. In 2002 Paolo became a fugitive and his sons took over the organization, but their leadership was less respected and rival groups threatened the clan’s dominance (Brancaccio 2014; Direzione Investigativa Antimafia 2012). Operation Beluga focused on the organization’s activities during the conflicts arising from the Di Lauro brothers’ struggles to maintain control (Direzione Investigativa Antimafia 2012; Tribunale di Napoli 2013).
The criminal group targeted in Operation Beluga (hereinafter the Beluga group), engaged in various illicit activities, including drug trafficking, firearms trafficking and illegal gambling. Yet, the seizure of 172 accounting books revealed the majority of their revenue came from drug trafficking (Tribunale di Napoli 2013). The group organized the retailing of drugs into two distinct open-air areas within the Rione Terzo Mondo. Praga Magica Street exclusively sold hashish and marijuana, while Il Barbiere di Siviglia Street specialized in cocaine and crack sales. The drug dealing operations were meticulously organized, with detailed records of the quantity and type of drugs sold by each retailer. Affiliates involved in drug trafficking and packaging received a fixed weekly payment, while those involved in street-level drug dealing received daily payments based on a percentage of their sales (Tribunale di Napoli 2013). The revenues enabled the clan to acquire weapons, corrupt public officials, provide financial support to incarcerated affiliates’ relatives, cover legal expenses for charged affiliates, rent warehouses for cars, drugs, and arms, and make personal purchases (Tribunale di Napoli 2013). Overall, the extensive information in the Beluga court order effectively informed MADTOR, providing the primary source of comprehensive information on criminal actors, activities and the economic aspects of drug trafficking and dealing for a prolonged period. Furthermore, one peculiarity of Operation Beluga was that during the investigation, at the end of 2010, the authorities arrested eight of the most prominent members of the Di Lauro clan. The arrests had a major impact on the criminal group and provided a unique opportunity to observe the organization’s resistance and resilience.
The development and calibration of MADTOR also relied on other sources. The first source was UNODC’s cocaine wholesale price data (UNODC 2008, 2009, 2010). A second source was the empirical literature on drug trafficking, which guided the modeling of disruption attempt features, elements considered in drug acquisition decisions, and the selection of criminal collaborators.
MADTOR’s reliance on data from the Beluga court order may raise concerns that it is biased by specific dynamics of the Beluga group. However, there are arguments suggesting that this bias should not significantly impact the model’s applicability to other drug trafficking organizations. One challenge is that the Beluga group exhibited characteristics of Mafia-type organizations. Unlike other Mafias, the Camorra comprises various urban clans and groups that form fluid coalitions, lacking a central coordinating body (Brancaccio 2014; Reuter & Paoli 2020; Scaglione 2016). This similarity to non-Mafia drug trafficking organizations in terms of structure and dynamics mitigates the potential bias. The Beluga group shared similarities with other drug trafficking organizations (Curtis 1996; Desroches 2005, 2007; Duijn et al. 2014; Kenney 2007; Natarajan et al. 2015; Natarajan & Belanger 1998). Its organizational structure aligns with communal business and corporate models, emphasizing cultural values, task division, and hierarchical structures.
The group also focused on regional and retail distribution, consistent with specialization observed in drug trafficking organizations. Their modus operandi prioritized profit maximization, even at the expense of lower-level members, employing protective strategies commonly found in similar cases: working in small teams, compartmentalizing information based on the "need-to-know" principle, assigning risky tasks to lower-ranking members, utilizing multiple channels for drug acquisition, processing, and sales, and ensuring consistent payments to minimize the risk of betrayal. Another challenge arises from the Beluga group’s involvement in other criminal activities, potentially blurring drug trafficking-specific dynamics. However, the detailed information in the Beluga court order allows for the isolation of factors influencing drug trafficking activities and precise distinction of costs and revenues related to different drugs. Overall, while we acknowledge that the Beluga court order substantially influenced the construction of the model, our arguments suggest that the Beluga group was comparable to many other large, structured drug trafficking organizations. Furthermore, MADTOR code is freely accessible so that the model can be easily adapted to different contexts and types of organizations.
MADTOR: Model for Assessing Drug Trafficking Organizations Resilience
Preliminary assumptions
The simulation of DTO criminal activities and their environment required four specific assumptions.
First, MADTOR focuses on cocaine. In the real world, drug trafficking organizations differ in the type of drug they deal with, and a single organization can be involved in trafficking multiple drugs simultaneously. However, trafficking and selling different drugs influence a variety of dynamics including countries of acquisition, processes of manufacturing, risks to conducting the business, and costs and revenues. These factors also shape the structure of the organizations involved in the trafficking and dealing processes. This precludes the possibility of developing a single model aggregating the trafficking and dealing of multiple drugs, unless substantially simplifying the modeling of drug-specific dynamics by assuming a uniform approach to the trafficking and dealing of different drugs. In addition, considering that available data, both from the literature and from the Beluga court order, are significantly richer in relation to cocaine than to other drugs,1 the focus on cocaine allows a simulation suitably close to reality in its input data and dynamics.
Second, MADTOR focuses on cocaine trafficking and dealing and disregards cocaine production and large-scale smuggling. The Beluga group was not involved in this stage of the drug market. Furthermore, previous research has indicated that cocaine production is often performed by groups solely in charge of this step and possibly of trafficking from production countries to destination countries. These groups have limited influences in the subsequent wholesale and regional distribution phases, the main focus of the present study (Benson & Decker 2010; Curtis 1996; Decker & Chapman 2008; Natarajan et al. 2015; Natarajan & Belanger 1998; Reuter 2014; Reuter & Haaga 1989; Zaitch 2002).
Third, the “KISS” (i.e., “Keep it simple, stupid!”) ABM principle demands reducing the complexity of drug trafficking and dealing activities to the minimum. Indeed, a simple model is critical to disentangle and interpret the effects of the hypothesized mechanisms (Axelrod 1997; Groff et al. 2019). In MADTOR, drug trafficking and dealing activities comprise 1) drug acquisition, 2) drug processing and packaging, 3) drug sales, and 4) accounting of expenses. These are the main steps of drug trafficking and dealing, as shown by criminal scripts of drug trafficking (Bright & Delaney 2013; Chiu et al. 2011; Le 2013), and the literature on the social organization of drug trafficking (Calderoni 2012, 2019; Natarajan 2000, 2006; Natarajan & Belanger 1998). Furthermore, the Beluga court order provided very detailed description on these steps (Tribunale di Napoli 2013).
The final assumption concerns law enforcement interventions. Firstly, we assumed that drug trafficking organizations regularly encounter minor disruption attempts, where law enforcement may arrest a maximum of one member per month. Secondly, each drug trafficking organization experienced a major disruptive event at the end of the second year.
Overview of the model
MADTOR was developed using version 6.2.0 of NetLogo (Wilensky 1999, 2021) and simulates the daily activities of the members of a drug trafficking organization for 5 years (Figure 1) (Manzi 2024). During this period, the offenders face the threat of law enforcement interventions intended to jeopardize their trafficking and dealing activities. Each simulation tick (the time measurement unit in NetLogo) is one day of real life, and the different activities occur after a different number of ticks. For example, traffickers attempt drug purchases every thirty ticks (i.e., once a month), the traffickers and packagers receive their wages every 7 ticks (i.e., once a week), and the retailers sell unit-dose packages of drugs to end users every tick (i.e., daily).
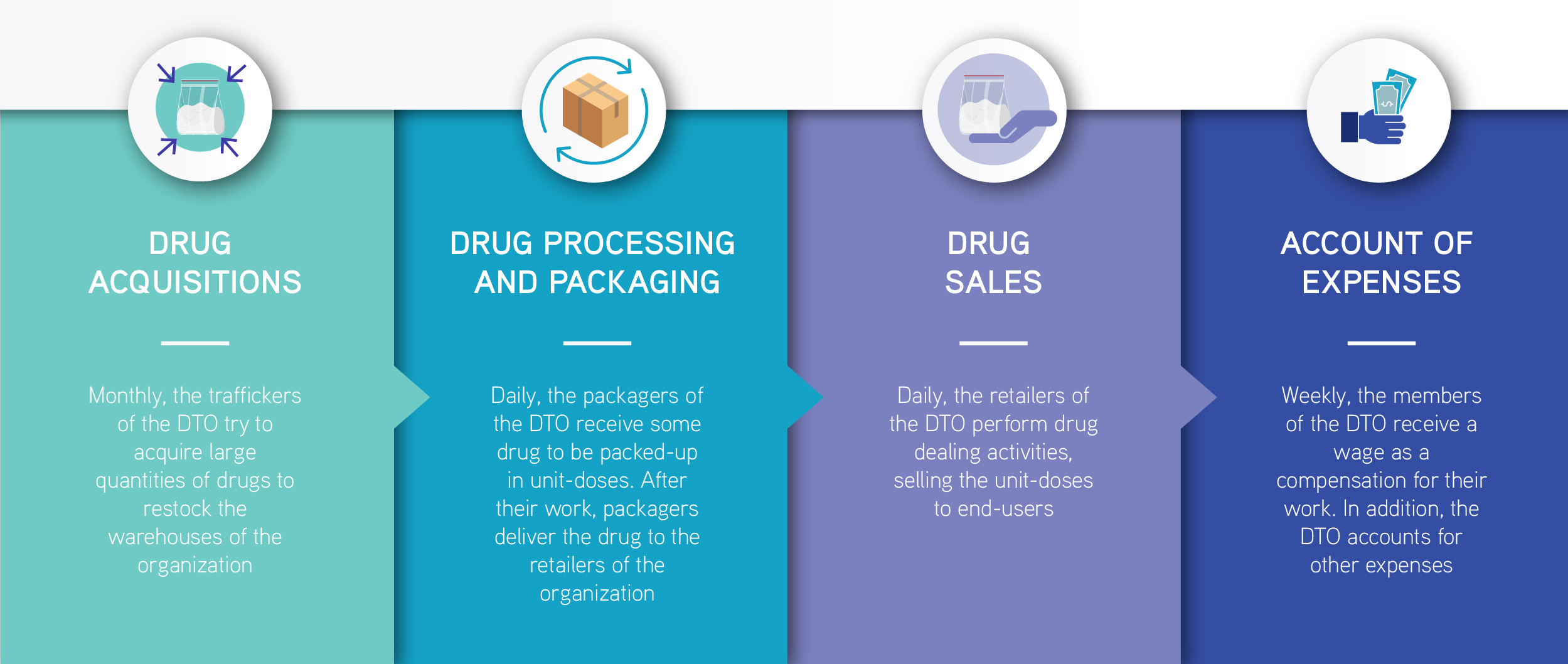
During the setup phase, the model imports relevant data. This includes information related to the structure and features of the drug trafficking organization (members details and relational patterns) and data related to the drug trafficking and dealing activities (quantities of drug stored and sold, drug wholesale and retail costs, drug prices for end users, and members’ rewards). The model updates these data every thirty simulated days to reflect the evolving structure of the Beluga group and the surrounding environment over time.2
At the beginning of the simulation, the drug trafficking organization comprised 44 members, each with a specific task: 5 members involved in drug acquisition, 5 members in drug processing and packaging, and 34 members in drug sales. The composition of the criminal workforce may change over time due to recruitments and defections. Recruitment occurs based on the organization’s workload needs, while defection can result from personal choices, arrests by law enforcement, or member deaths.
The members of the organization possess three key attributes, inspired by the literature (Duxbury & Haynie 2019; Weisburd et al. 2017): criminal expertise, organizational visibility, and internal proximity. Furthermore, each dyad of member has a collaboration record. These variables are detailed in Table 1 and in the online model documentation. Members’ interactions and drug exchanges are influenced by these factors. The model updates these attributes daily to reflect any fluctuations in the level of criminal expertise and evolving patterns of member interaction.
Attribute | Definition |
---|---|
Task | It outlines the specific activities carried out by members within the organization. Members are designated as either traffickers, packagers, or retailers, and these tasks are not interchangeable. |
Drug | Quantity of drugs stored by each member at a specific time point (simulation tick). |
Criminal expertise | It is a general measure of the members’ criminally relevant skills and abilities. Each actor is assigned a level of criminal expertise, determined by a normal distribution that is normalized between 0 and 1. |
Collaboration record (dyadic) | It assesses the trust levels between any two members of the organization. It is the number of prior exchanges between each pair of members. Every drug exchange between two actors increments their collaboration record by 1. |
Organizational visibility | It evaluates each member’s activity within the organization and therefore its internal and external visibility. It measures the difference between the degree of an actor and the degree of the most central actor in the organization (Duxbury & Haynie 2019; Freeman 1979). |
Internal Proximity | It measures members’ capacity to reach all other members in the organization. It is calculated using closeness centrality, a network analysis metric (Freeman 1979). |
Availability | Binary attribute indicating whether each member is available to receive drugs at a specific time point; it factors in the maximum daily workload that packagers and retailers can sustain. Traffickers do not possess an availability attribute, as it is unrelated to their duties. |
The first activity is drug acquisition. On a monthly basis, the organization’s traffickers assess the feasibility of procuring drugs based on several factors: the current stockpile in organization’s warehouses (greater quantity reduces the urgency for new acquisitions), the attractiveness of wholesale prices (higher prices discourage acquisition), and market conditions such as drug availability and perceived risk of apprehension (adverse conditions reduce the likelihood of acquisition). The traffickers acquire drugs on a probability basis, relying on the computation of a composite index considering the abovementioned factors. The outcome of the acquisition attempt is influenced by the level of criminal expertise of the trafficker involved, with higher skills increasing the likelihood of a successful acquisition. On average, across the simulations, the traffickers acquire the drugs at the wholesale level for 40.45 €/g (SD: 4.24). Following each acquisition attempt, successful or unsuccessful, the model updates the level of traffickers’ criminal expertise. Positive outcomes enhance their skills, while negative outcomes result in a decrease (for further details on the computation see the online model documentation).
The second activity is making unit-dose drug packages. Daily, the packagers of the group receive large quantities of drugs from the traffickers, they package them into drug doses, and transfer the doses to the retailers. MADTOR simulates the processing and packaging of drugs through two drug exchanges, one from traffickers to packagers and another from packagers to retailers. Between these exchanges, packagers are responsible for packaging the drug doses. The model imposes a maximum daily workload that packagers can receive/deliver. MADTOR determines the members involved in drug exchanges relying on the criminal expertise, organizational visibility, internal proximity and collaboration record (refer to the online model documentation for more details).
The third activity is drug sales. Retailers sell unit-dose packages to consumers and collect revenues, based on the evidence from Operation Beluga. The organization can sustain an average daily volume of sales of almost 1,900 cocaine doses of 0.25 g each, sold at an average price of approximately 32€per dose. Each retailer is also constrained by a maximum daily profit of 500€. The model randomizes the exact number of doses within a range determined by the minimum and maximum daily sales of the Beluga group. Retailers retain a fixed percentage (18%) of the revenues as compensation and transfer the remaining funds to the leaders of the group.
The last activity is accounting of costs and expenses. Instead of specific members, MADTOR just elaborates the financial situation of the organization by accounting of revenues and expenses. The only source of revenues is the sale of drugs, while the expenses comprise three categories: wages for traffickers and packagers (whose salaries are unrelated to drug dealing activities), payments to the families of arrested or deceased members, and variable expenses such as bribes, legal fees, and warehouse rent. Additionally, the bosses of the organization withdraw money from the organization’s cashbox as their personal profits.
Law enforcement interventions and disruption of drug trafficking organizations
During the five-year simulation, the drug trafficking organization faces both minor and major law enforcement action. Minor actions occur on a probability basis and involve the monthly arrest of an unspecified member, while major actions occur after two years and result in a larger police operation. In major actions, the law enforcement randomly arrests a share of the members and confiscates the drug in their possession. The share can take the following values: 0%, 5%, 10%, 20%, 30%, 40%, 50%, 60%, 70%, 80%, and 90%. The occurrence of the major action after two years serves two purposes: firstly, it enhances the robustness of results by providing an opportunity to observe the drug trafficking organization’s operations and potential modifications in the two years leading up to the disruption attempt. This allows for a more comprehensive understanding of the internal functioning of the organization. Secondly, the two-year period aligns with real-world scenarios, particularly with the timing of the major disruption attempt that targeted the Beluga group. This calibration of the model to resemble actual events aids its accuracy and relevance to real-world situations. By replicating the timing of a real disruption attempt, the model can capture key dynamics and patterns closer to operational settings, further validating its effectiveness.
While law enforcement agencies rarely select targets for arrest through algorithms such as network centrality metrics as done by some studies, we recognize that their selection processes are not random. In this study, our primary goal was to introduce the internal mechanisms of MADTOR and demonstrate them in a straightforward scenario. We employed the random selection method with the purpose of parsimony and of simplicity (adhering to the KISS principle), to focus on the impact of arresting different proportions of the organization’s members. A more sophisticated approach –would create more realistic arrest scenarios - would incorporate various factors including the organization’s preference for security over efficiency, the visibility and vulnerability of its members to law enforcement agencies, the roles of each member within the organization, their abilities to evade detection and the resources available to law enforcement agencies. These issues would substantially complicate the simulations and we opted to address them in other studies. For example, in Manzi & Calderoni (2024), we analyzed the impacts of targeting individuals based on their specific roles within the drug trafficking organization.
Law enforcement disruption attempts have significant consequences for the operations of the drug trafficking organization. They can severely damage the organization’s financial resources and workforce availability, ultimately leading to disruption of the group, i.e., the organization can no longer perform drug trafficking and dealing. Disruption can occur due to economic inefficiency or law enforcement interventions. Economic inefficiency can lead to disruption when the organization fails to generate sufficient profits to sustain its operations. This disruption occurs if the financial liquidity of the organization reaches zero or lower values. This can result from insufficient drug acquisitions, low sales, excessive expenses and other factors. Economic inefficiency may occur independently or subsequently to the arrests. Disruption due to law enforcement intervention, occurs when the arrests induce scarcity of drugs or workforce and jeopardize the organization’s criminal activities. Law enforcement interventions directly disrupt drug trafficking organizations by rendering them unable to continue their criminal activities, due to the loss of drugs and members and the inability to quickly recruit replacements. When law enforcement arrests all members performing one specific task, the organization can try and recruit new members for 30 ticks, corresponding to one month. Afterwards, if the recruitment is unsuccessful, the organization is disrupted.
In most simulated cases (almost 97% of disrupted organizations), economic inefficiency caused the disruption. The arrest never targeted all the members, making it unlikely for drug trafficking organizations to completely cease their operations. However, the arrests often reduce the organization, or the members performing specific tasks, to their minimum, making the criminal activities economically unsustainable in the short to medium term. Although it is the most frequent cause of disruption, economic inefficiency is strongly linked to arrests, which indirectly lead to the failure of organization.
Simulation strategy and data analysis
To investigate how the arrest of different proportions of members affect the resistance and resilience of drug trafficking organizations, we ran eleven MADTOR sub-models corresponding to different arrest scenarios for the major law enforcement actions at the end of year 2 (0%, 5%, 10%, 20%, 30%, 40%, 50%, 60%, 70%, 80%, and 90%). We simulated each sub-model 1,000 times to achieve robust results while ensuring a reasonable computing time for running the whole experiment. We ran each simulation for 1,825 ticks, corresponding to 5 years of simulated time, a sufficient period to observe the resilience and resistance of drug trafficking organizations after the major arrest.
We operationalized the concept criminal network resilience and resistance presented in the background section into three dimensions. First, we chose the share of active drug trafficking organizations for the ability to endure disruption dealing with major threats. Second, we considered the number of members as a measure of the ability to react quickly and efficiently to law enforcement interventions. Third, we measured the ability to maintain primary functions and activities unaltered through the revenues of the drug trafficking organizations (Ayling 2009; Bouchard 2007; Duxbury & Haynie 2019) (Table 2). We compared the trends of the three indicators for the different arrest scenarios, considering the 0% arrest scenario as the baseline. While we expected that the average trends would be indistinguishable before the major law enforcement action, we focused on the differences after the disruption attempts imputing them to the impact of the law enforcement intervention.
Dimension of Criminal Network Resilience | Resilience Indicator | Interpretation |
---|---|---|
Endure disruption | Share of active drug trafficking organizations | A measure of the resilience of drug trafficking organizations in withstanding law enforcement interventions. A higher share indicates a greater ability to endure disruptions over time. |
React quickly and efficiently | Number of members | An indicator of drug trafficking organizations’ stability and strength. A larger member count suggests a more robust organization. Following a disruption, this metric provides insights into the organization’s ability to recruit new members, indicating a quick and efficient recovery from the shock. |
Maintain primary functions and activities unaltered | Revenues | A proxy for drug trafficking organizations’ capacity to sustain their illicit trade. Significant reductions in revenues following arrests indicate an inability to maintain drug trafficking and dealing operations. |
We aggregated the resilience indicators at each step across simulations of the same sub-model. To improve the readability and visualization of the results, for the number of members and revenues we computed the monthly mean of daily values and then averaged across all simulations of the same arrest scenario. At the beginning of the simulation, every indicator is the average of 1,000 simulations, while afterwards is draws on fewer simulations due to progressive disruption of some organizations.
To test the significance of the differences observed among the different arrest scenarios compared to the baseline with 0% arrest, we relied on randomization-based two-sample Student’s t tests.3 We computed randomization-based t tests with 100 repetitions for the two years before the arrests and for the 3 years after the arrests to assess the significance of the differences in the simulations over time. We expected non-significant differences before, and significant differences after the arrests. Furthermore, for the number of members and the revenues of the drug trafficking organizations, we also performed the same tests for the average monthly values, to identify significant differences in specific months.4 Randomization-based t tests allows the identification of differences provoked by law enforcement interventions. Indeed, two combinations should be indistinguishable (apart from the effects of the stochastic elements included in the model) before the disruption attempts; conversely, after an attempt at disruption, it should be possible to observe the impact, if any, of the simulated interventions.5
Calibration of the model and sensitivity tests
We developed MADTOR with the aim of reconstructing plausible dynamics related to drug trafficking and dealing. This includes understanding the performance of criminal activities and members’ behaviors. To calibrate the model, we heavily relied on available quantitative data, primarily sourced from the Beluga court order. Additionally, we incorporated qualitative information obtained from both the court order and relevant criminological theories and literature.
During the calibration process, to prevent an excessively deterministic approach, we permitted simulated values to fluctuate within the range defined by the minimum and maximum values observed in the actual data. Table 3 shows the distribution of members across different roles as documented in the Beluga court order alongside the median values derived from simulations during the initial three years. These years were selected to capture dynamics before the impact of simulated law enforcement interventions. Overall, we observed a commendable level of congruence, with simulated values slightly below the real ones. This difference may be attributed to the influence of minor law enforcement interventions not explicitly documented in the Beluga court order.
Year | |||
---|---|---|---|
1 | 2 | 3 | |
Beluga members | 44 | 59 | 66 |
MADTOR, median members | 44 | 57 | 64 |
Beluga traffickers | 5 | 13 | 16 |
MADTOR, median traffickers | 5 | 12 | 15 |
Beluga packagers | 5 | 13 | 13 |
MADTOR, median packagers | 5 | 10 | 13 |
Beluga retailers | 34 | 33 | 37 |
MADTOR, median retailers | 34 | 36 | 37 |
Similarly, the scale of drug trafficking and dealing activities closely aligns with that of the Beluga group. In the second year, the simulated organizations show a minimum of 1,376 drug doses sold daily, slightly surpassing the Beluga group’s reported 1,370 doses in May 2010. The simulated maximum, reaching 2,328 doses, compares with one of the Beluga group’s most lucrative weekend in June 2010, where they recorded 2,340 doses daily. On average, the simulations depict organizations selling 1,846 doses daily, falling within the range defined by the minimum and maximum reported values for the Beluga group.
The calibration of MADTOR also involved figures extrapolated from the Beluga court order that cannot be directly validated, such as the number of drug acquisitions, revenues, and the amount of drugs in stock. However, given that the calibration primarily relies on empirical data for the most crucial aspects of the model (e.g., organizational structure, workload, wholesale and retail cocaine prices, members’ wages, expenditures and profits), as well as insights from the criminological literature (e.g., the establishment of criminal working relations), it is plausible that the obtained outcomes realistically depict the drug trafficking and dealing activities of the organization (see the online model documentation for further details on calibration).
To evaluate MADTOR’s robustness against variations in several model parameters, we conducted a series of sensitivity tests (Table 4). We modified the assignment of criminal expertise, which was not based on empirical evidence, to see whether random distribution may affect the simulation. We hypothesized that this variation should not substantially affect the main result as the criminal expertise is combined with other factors in the model. We removed the monthly minor law enforcement actions to test whether smaller periodic arrests influenced the main results. Our hypothesis is that this test should yield a slight improvement of the share of active drug trafficking organizations across all sub-models, baseline included. We also explored the sensitivity of MADTOR to variations in the wholesale prices of drugs, events that may periodically occur due to major law enforcement seizures or combinations of factors along the supply chain (Caulkins & Reuter 2010; Reuter et al. 2021). We considered the repercussions of a 10% fluctuation in wholesale drug prices.6 Given the slightly inelastic consumption of cocaine, we expect that a 10% reduction in wholesale prices would result in reduced retail prices, reduced unit margins, and overall decreased economic performance. Conversely, increased cocaine cost would result in higher margins and profitability (Caulkins 2021; Reuter et al. 2021).
Each test was replicated 100 times for every arrest scenario, amounting to almost 80 hours of computational effort.
No. | Test | Description | Computational time |
---|---|---|---|
1 | Criminal expertise | Actors’ criminal expertise level is assigned randomly at the simulation start instead of following a normal distribution | 25h30’ |
2 | Minor law enforcement actions | Monthly minor law enforcement actions are removed from the simulations | 24h |
3 | Wholesale drug price 10% increase - Midpoint model | 10% increase in the wholesale cost for cocaine. The retail price increases following a midpoint model. Elasticity of units sold to price variation is -0.84. | 12h |
4 | Wholesale drug price 10% decrease - Midpoint model | 10% decrease in the wholesale cost for cocaine. The retail price increases following a midpoint model. Elasticity of units sold to price variation is -0.84. | 18h |
Results
The results indicated a positive correlation between the percentage of members arrested and the percentage of disrupted organizations (Table 5 and Figure 2). In the baseline scenario, with no arrests, only about 73% of the initial 1,000 organizations were active at the end of year 5.7 All the arrest scenarios significantly reduced the survival of drug trafficking groups, ranging from about 37% survival for arresting 5% of the members to about 6% for arresting 90% of the members. Most organizations are disrupted within a year of the arrests (during year 3), afterwards the trend is comparable to the baseline for years 4 and 5. Randomization t-tests among the monthly shares of active organization across the different arrest scenarios showed no statistically significant differences in the distributions before the arrests (year 1 and 2); conversely, most distributions are different in the period after the arrests (year 3 to 5) (Figure 7 in the Appendix).
Share of members arrested | Start | Year 1 | Year 2 | Year 3 | Year 4 | Year 5 | Change% Year 5 vs. Year 2 |
---|---|---|---|---|---|---|---|
0% | 1000 | 982 | 867 | 801 | 767 | 737 | -14.99% |
5% | 1000 | 986 | 858 | 412 | 396 | 368 | -57.11% |
10% | 1000 | 982 | 848 | 398 | 365 | 339 | -60.02% |
20% | 1000 | 982 | 836 | 317 | 273 | 250 | -70.10% |
30% | 1000 | 983 | 877 | 298 | 278 | 251 | -71.38% |
40% | 1000 | 987 | 865 | 256 | 240 | 227 | -73.76% |
50% | 1000 | 986 | 859 | 235 | 210 | 196 | -77.18% |
60% | 1000 | 985 | 866 | 216 | 203 | 191 | -77.94% |
70% | 1000 | 985 | 851 | 155 | 132 | 126 | -85.19% |
80% | 1000 | 982 | 849 | 129 | 113 | 109 | -87.16% |
90% | 1000 | 976 | 842 | 88 | 72 | 64 | -92.40% |
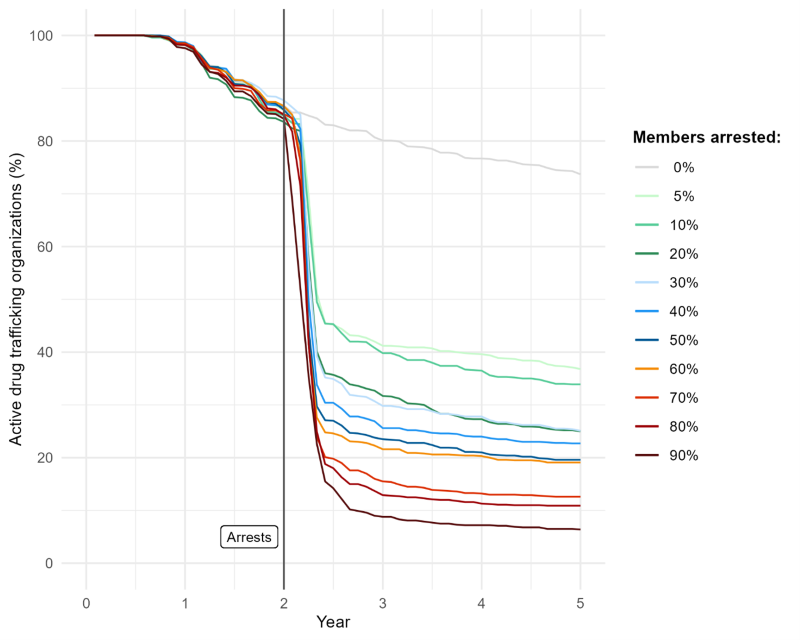
In addition to survival, we examined the number of members as a proxy for the drug trafficking organizations’ capacity to react quickly and efficiently to the arrests. For simplicity, we focused on four scenarios: the baseline and the arrest of 10%, 40% and 80% of the members (Figure 3 and Table 6 in the Appendix). We report the results for all the scenarios in Figure 8 in the Appendix. Initially, groups had 44 members and grew to around 65 members by year 2. In the baseline scenario, growth slowed between year 3 and 5, reaching about 68 members by the simulation’s end. The arrest scenarios affected member numbers proportionally, with statistically significant impacts until year 5 based on the results of the randomization t-test of monthly average number of members (Figure 3 and Table 7 in the Appendix). In the 10% and 40% scenarios, member numbers nearly recovered the baseline by year 5, albeit with slower recovery in the 40% scenario. However, the 80% scenario resulted in substantially smaller surviving organizations, nearly 13 members fewer than the baseline after three years.
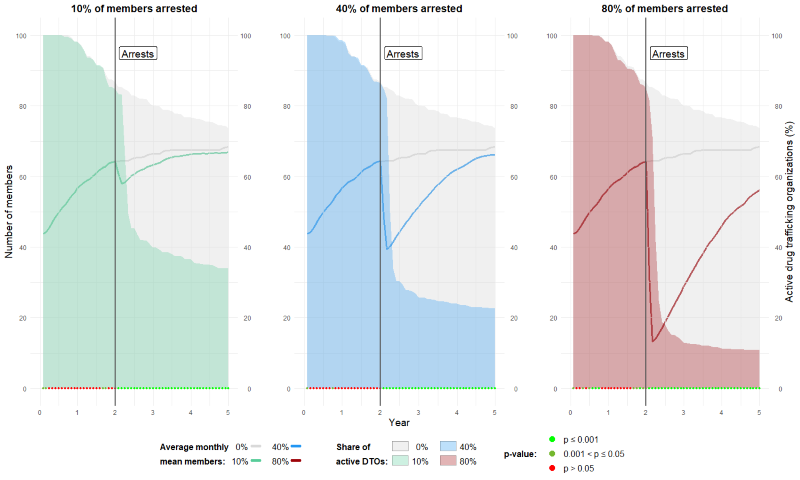
Finally, we analyzed the revenues of drug trafficking organizations (again we focused on the 10%, 40%, and 80% arrest scenarios, refer to Figure 9 in the Appendix for all scenarios) as an indicator of their operational capacity (Figure 4). Starting around 27k€ of daily revenues, all organizations experienced a peak after setup, reaching about 36k€ before the arrests. Subsequently, in the baseline scenario resources increased more slowly, reaching about 40k€by the simulation’s end. (Table 8 in the Appendix). The arrest scenarios caused substantial revenue decreases due to seizures and missed drug sales in the six months following arrests. In the 10% scenario, revenues drop from about 36k€to 25k€after the arrests. However, surviving organizations quickly recovered, and by the second half of the third year they had regained the revenues levels of the baseline. In the 40% scenario, revenues plummeted to 15k€, and it took the surviving groups a full year to fully recover from this setback. The 80% scenario resulted in a substantial decline in revenue, reaching as low as 5k€. As a consequence, the remaining organizations required over two years to reach revenue levels comparable to the baseline.
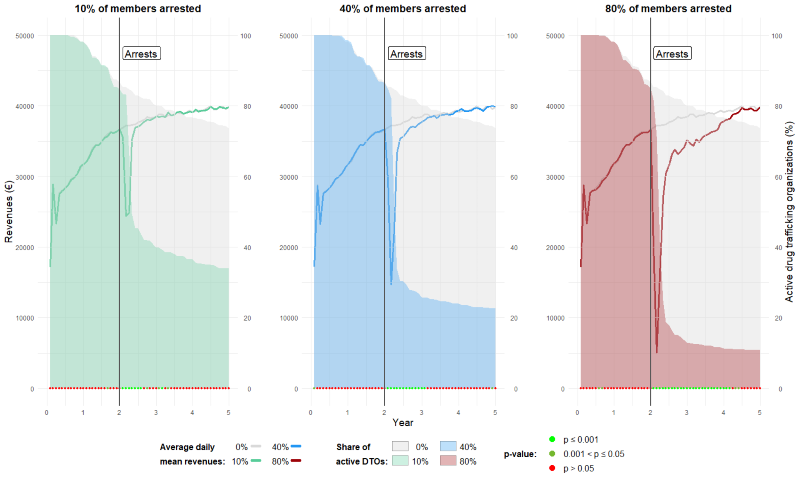
The results from sensitivity tests indicate the overall robustness of the main findings to variations in different parameters (Figures 5 and 6). Across all tests, it was consistently observed that the baseline scenario yielded a higher proportion of active organizations compared to any of the arrest scenarios. Additionally, the intensity of arrests showed a positive and consistent correlation with the share of active organizations, with minor fluctuations likely due to the fewer runs compared to the main models. Conversely, the number of members and the revenues did not report substantial departure from the main models.
Nevertheless, modifying certain parameters affected all scenarios by either increasing or decreasing the number of active organizations. Test 1, closely resembling the main models, confirmed our hypothesis that the attribution of actors’ levels of criminal expertise did not significantly impact the results. Test 2 revealed that removing minor law enforcement actions did not alter the relative ranking of arrest scenarios. Yet, the failure rate of drug trafficking organizations tends to improve, except for major arrests, suggesting that the rate of organization failure was at least partially influenced by minor law enforcement actions. Tests 4 and 5 highlighted the sensitivity of results to market conditions, consistently with our expectations: a 10% increase in the wholesale cost of drugs resulted in the organizations increasing retail prices and obtaining higher margins per unit. Increased margins offset the reductions in units sold, resulting in a higher survival rate. Conversely, a 10% decrease in the price of wholesale cocaine led to narrower margins, significantly affecting the survival of organizations.
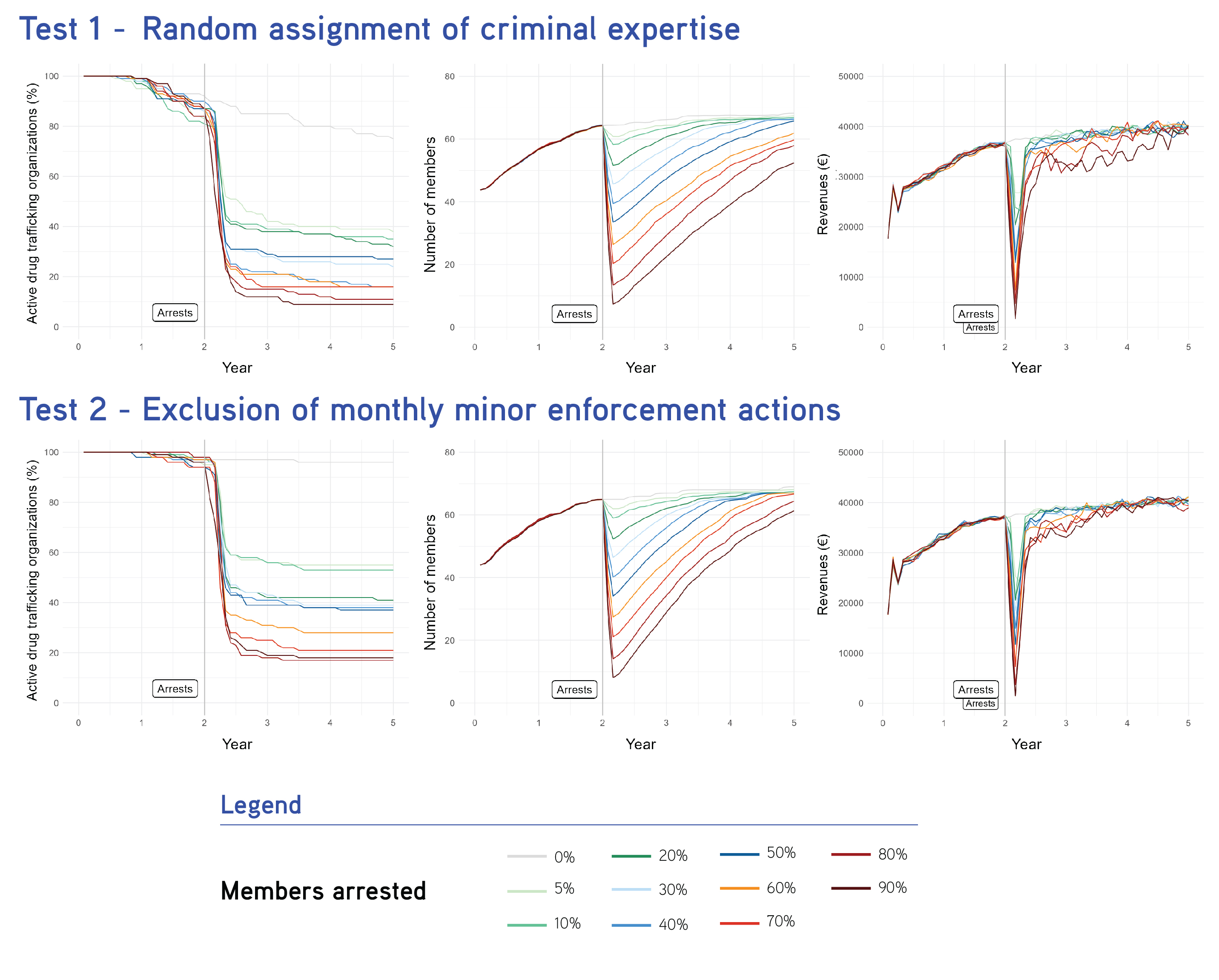
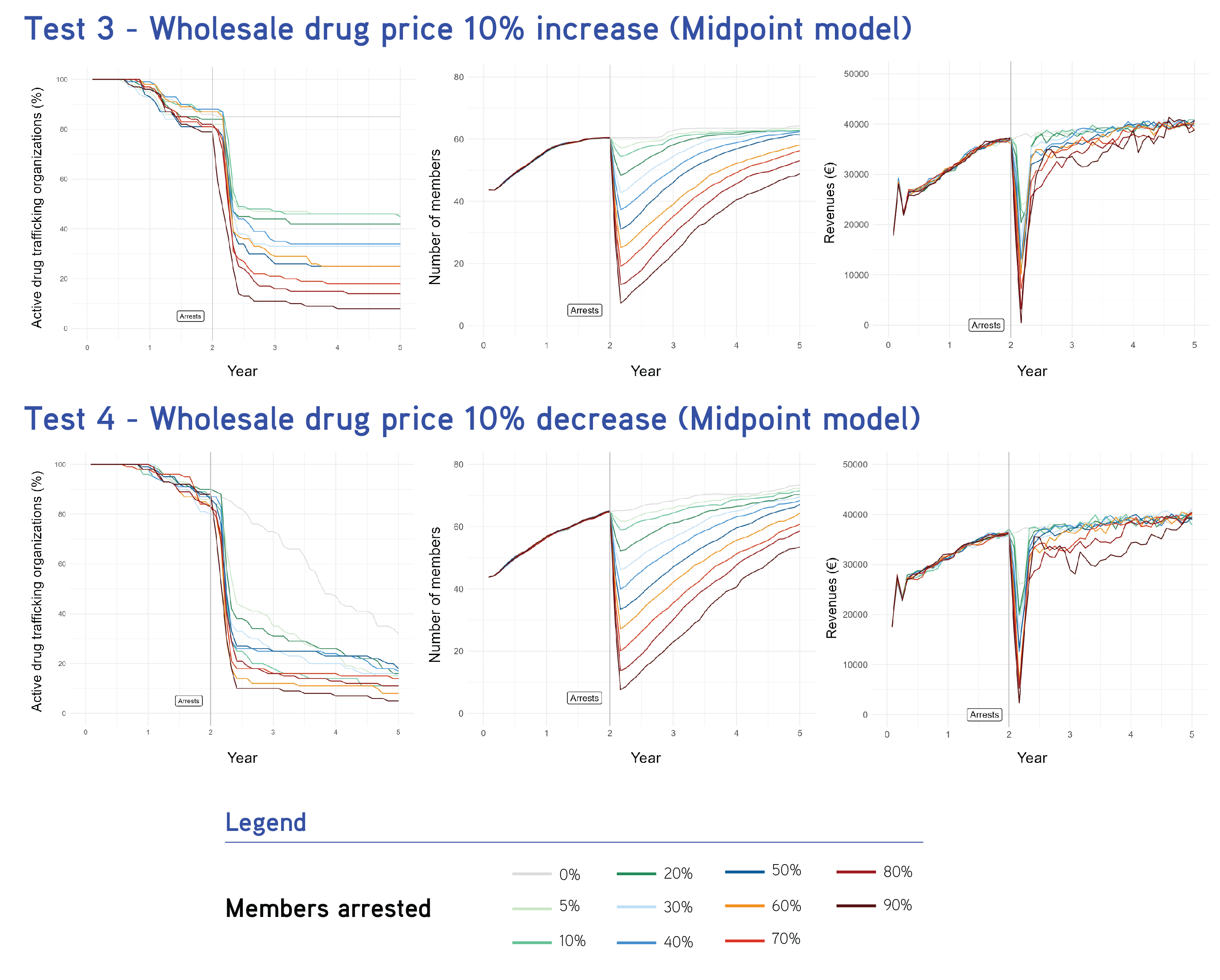
Discussion and Conclusion
We found that the proportion of members of the drug trafficking organizations arrested had a significant impact on the groups’ resistance and resilience. As the proportion of arrested members increased, the organizations faced greater difficulties in surviving and responding to disruptions. However, the impact was not directly proportional to the share of arrested members. Even the arrest of a small percentage of members disrupted a substantial portion of the drug trafficking organizations. For instance, arresting 5-10% of members already halved the number of surviving organizations compared to the baseline scenario (Table 5). While a higher share of arrested members increased the disruption rate, the incremental effects were relatively smaller. The disruptive impact occurred within six months of the arrest, during which the disruption rates increased compared to the baseline. Although drug trafficking organizations managed to survive for a few months, they ultimately failed to achieve long-term sustainability.
Additionally, organizations that survived disruptions faced increasing difficulties as the share of arrested members increased. In terms of member numbers, these organizations struggled to fully recover to baseline levels. In all the three arrest scenarios, surviving organizations never fully reached the baseline. However, in the 10% scenario, simulations indicated near recovery after 1.5 years from the arrest, whereas it took them approximately 2.5 years to approach similar levels in the 40% scenario. In the 80% scenario, recovery never came close to the baseline. Regarding revenue levels, organizations were able to bounce back, but at varying paces. In the 10% scenario, organizations reached baseline revenues within six months, while it took about a year in the 40% scenario. However, in the 80% scenario, recovery took over two years. Overall, the 10% scenario showed minimal adaptations among surviving drug trafficking organizations, whereas the other scenarios indicated longer-lasting impacts.
The main results were robust to modifications including the removal of minor arrests and changes in the actors’ levels of criminal expertise. Furthermore, the survival and performance of the drug trafficking organizations were substantially affected by fluctuations in the wholesale cost of cocaine. Our tests model assumed that the drug trafficking organization would react to exogenous changes in the wholesale costs by tranferring a share of the change to the consumer (a midpoint scenario between the additive and multiplicative models discussed in the literature) and that this would automatically influence the volume of sales. We noted however, that organizations may react in several ways to similar market developments. More specific results of the impact of costs changes along the supply chain would require additional modelling considering, for example, the possibility to expand or reduce the workforce, increase/decrease salaries, change cocaine purity levels while maintaining the retail price. We plan to address these scenarios in future research.
In addition to market dynamics, we also found that the roles of the arrested members during major law enforcement actions affected the organizations’ survival. Consistent with the results of another study using MADTOR (Manzi & Calderoni 2024), organizations reaching the end of the simulations reported less traffickers and more packagers arrested.8 Previous research has repeatedly emphasized the importance of traffickers for the internal functioning of drug trafficking organizations (Bright et al. 2017; Calderoni 2012; Castiello et al. 2017; Duijn et al. 2014; Duxbury & Haynie 2018, 2019; Morselli & Roy 2008; Wood 2017). Our results are in line with these studies, highlighting the critical role of traffickers in the operations of drug trafficking networks.
These findings highlight the critical impact that even limited law enforcement actions can have on the operations of drug trafficking organizations. They are consistent with research suggesting that drug prohibition generates substantial positive effects on the structure of illicit drug markets, and that minimal enforcement suffices to capture the gains from prohibition (Babor et al. 2018; Pollack & Reuter 2014; Reuter et al. 2016). The non-linear relationship between the proportion of arrested members and disruption challenges the notion that higher arrest percentages lead to proportionally greater disruption. For instance, in our simulations increasing the arrests from 40% to 80% of the members resulted in a marginal disruption of less than 20% (227 vs. 109 active organizations by the end of the simulations out of 1,000 starting organizations, respectively, as shown in Table 5). This impact prompts a reevaluation of law enforcement strategies and the allocation of resources. From a disruption-focused perspective, even a limited number of arrests may significantly impact the long-term viability of most drug trafficking organizations. This observation raises questions about the efficacy of escalating law enforcement efforts, considering the potential imbalance between their impact on the drug market and the societal costs they entail. Nonetheless, it is evident that drug law enforcement objectives also encompass the enforcement of the law, deterrence, incapacitation, and asset recovery. By targeting a larger share of members, law enforcement can expand the identification and recovery of their illicit assets, further destabilizing their organizations and depriving them of the financial resources necessary to sustain their operations. Thus, increasing the share of arrested members may serve these objectives.
Regarding organizations surviving disruption, the proportion of arrested members negatively affects their resilience. When faced with the arrest of only a small portion of their members, surviving organizations make slight adaptations and continue business as usual, as criminal networks are known to tolerate minor adversities due to operating in a hostile environment and accepting certain risks (Kleemans 2014). However, when arrests target 80% of members, surviving organizations must undergo significant organizational changes to continue pursuing their primary goals. For instance, they must engage in substantial recruitment efforts to rebuild their workforce, which requires access to a social environment with individuals possessing specific criminal skills (Duijn et al. 2014; Spapens 2010). If it finds replacements, an organization experiencing such a massive turnover rate may not resemble its former self. In our simulations, organizations that survived arrests of 80% of their members ended up with over three-quarters newcomers. Such high turnover can lead to significant modifications in the organizations’ ambitions, attitudes, and relational patterns, transforming their original distinctive traits in response to the crisis (Butera 2009). Even if these substantially altered organizations survive the arrests and demonstrate resilience, most of their members at the time of the arrests will be imprisoned. Overall, while at the group level the surviving organizations may be resilient to disruption, at the individual level law enforcement interventions may carry heavy consequences for the initial members.
Our findings provide a complementary perspective to past research on criminal organizations, which often highlights their high levels of resilience (e.g., Morselli & Petit (2007; Berlusconi 2013; Diviák et al. 2022; Manzi 2019)). Previous studies focused on successful cases of resilience, analyzing exceptional organizations that survived and even thrived under law enforcement pressure. In contrast, our study expands the analysis of resistance and resilience to a larger number of simulated groups, demonstrating that most drug trafficking organizations are unable to survive the arrest of members. Nonetheless, we did find evidence that a minority of surviving organizations may exhibit resilience, continuing their criminal involvement with or without implementing reactive strategies (Berlusconi 2013; Bright et al. 2017; Carley 2006; Diviák et al. 2022; Duijn et al. 2014; Manzi 2019).
Our study is limited by the simplified simulations of drug trafficking and dealing. However, it substantially expands previous ABMs in the field of drug trafficking (Dray et al. 2008; Duxbury & Haynie 2019, 2020; Magliocca et al. 2019, 2022; Romano et al. 2009). Specifically, it explicitly models the crime commission processes and simulates the arrest of multiple actors, it models the reaction strategies of drug trafficking organizations including the recruitment of new members and the establishment of new ties and it enables the testing of different types and intensities of law enforcement strategies.
Furthermore, MADTOR focused on cocaine, and caution should be exercised in extending the results to other drugs. Lastly, we also acknowledge that MADTOR’s reliance on information retrieved from Operation Beluga to empirically inform a general model of drug trafficking organizations’ resilience limits the external validity of its results. However, we believe that the value of this study extends beyond its immediate findings. It is the first attempt to model drug trafficking organizations’ reactions to law enforcement interventions, systematically outlining and operationalizing various features of these organizations. The model documentation and code are publicly available to encourage further expansion and adaptation by scholars.
Future research can explore multiple directions. First, MADTOR enables to explore additional aspects of the resilience of drug trafficking organizations, including e.g., the impact of targeting members with different tasks, or the impact of different organizational arrangements of drug trafficking organizations along the security versus efficiency trade-off (Morselli et al. 2007). Second, future efforts can expand the data for model calibration and validate the results using additional case studies. Third, MADTOR concentrates on the resistance and resilience of individual organizations within the drug market, neglecting the high-level dynamics that could emerge in the drug market following the demise of certain organizations. The dissolution of an organization could potentially incentivize other actors to enter the market to meet consumer demand. Incorporating such dynamics would have necessitated specific knowledge and data, presently unavailable, significantly elevating the model’s complexity. Future studies may explore these and other potential market dynamics resulting from disruption efforts.
In conclusion, our study offers valuable insights into the resistance and resilience of drug trafficking organizations while presenting the structure and applications of the MADTOR ABM. Arresting even a small proportion of members can lead to significant disruption within drug trafficking organizations. Yet the non-linear relationship between arrest rates and disruption reveals diminishing returns as the proportion of arrested members increases. Surviving organizations encounter mounting challenges in their recovery as the share of arrested members rises. These findings align with past research on drug prohibition and minimal enforcement. Future research could expand upon our study by exploring additional aspects of resilience and validating the results through diverse case studies, further enhancing the utility of MADTOR. This will facilitate the development of targeted strategies, including the identification, tracing, and recovery of illicit assets and proceeds of crime, which can effectively aid in combating drug trafficking, thus minimizing its detrimental societal consequences.
Model Documentation
The peer-reviewed code, the ODD+D protocol and a detailed narrative documentation of the model are available and downloadable at the following link: https://www.comses.net/codebases/2ff1e89b-c1ba-44df-9cfc-34484b603e0a/releases/1.1.0/.Acknowledgements
Both authors contributed to the study conception and design. DM wrote the simulation code in NetLogo, prepared, and analysed the data. Both authors drafted the manuscript and approved the final manuscript. We would like to thank the anonymous reviewers who took the time to provide extremely valuable comments on our article. Their invaluable suggestions have substantially contributed to improving the quality of the work.Funding
This article has received support by Project RECOVER - Mutual recognition of freezing and confiscation orders between efficiency and the rule of law, co-funded by the Justice Programme of the European Union 2021-2027 (JUST-2022-JCOO) under grant agreement No. 101091375.Appendix
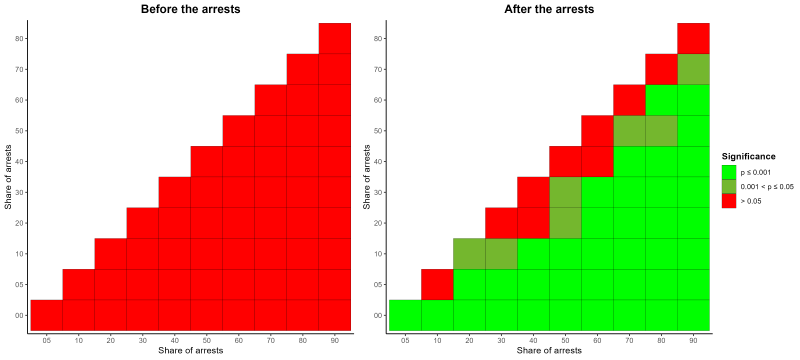
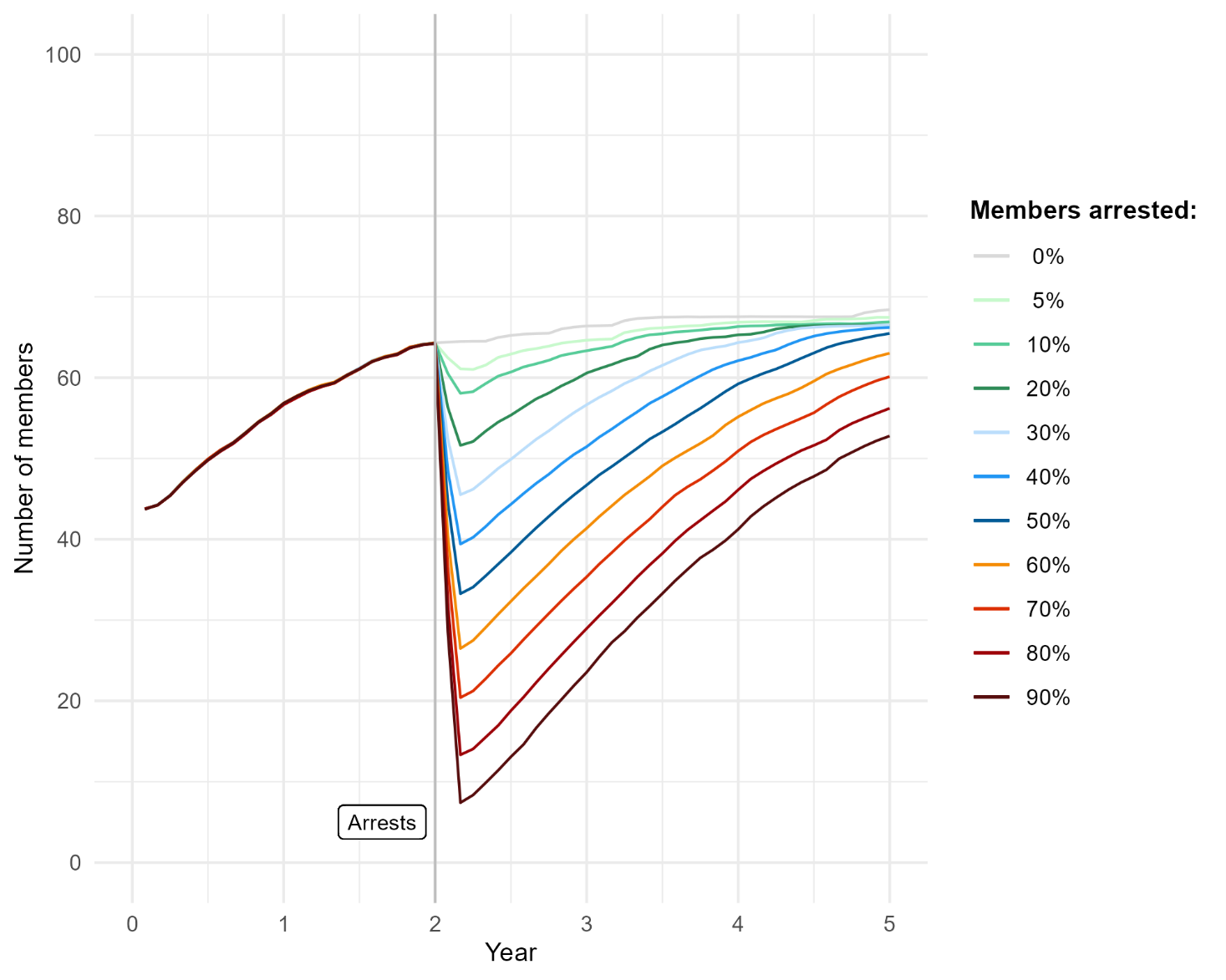
Arrest scenario | Year | Active organizations | Min | Max | Mean | SD |
---|---|---|---|---|---|---|
Start | 1000 | 43.53 | 44.00 | 43.78 | 0.23 | |
1 | 982 | 47.97 | 59.97 | 56.75 | 1.84 | |
2 | 867 | 58.50 | 65.00 | 64.30 | 0.83 | |
0% | 3 | 801 | 61.97 | 67.00 | 66.39 | 0.68 |
4 | 767 | 64.50 | 68.00 | 67.53 | 0.52 | |
5 | 737 | 64.97 | 69.00 | 68.42 | 0.64 | |
Start | 1000 | 43.53 | 44.00 | 43.77 | 0.23 | |
1 | 982 | 50.47 | 59.97 | 56.74 | 1.86 | |
2 | 848 | 58.50 | 65.00 | 64.24 | 0.83 | |
10% | 3 | 398 | 59.53 | 65.00 | 63.33 | 0.97 |
4 | 365 | 62.50 | 68.00 | 66.32 | 0.79 | |
5 | 339 | 64.53 | 68.93 | 66.89 | 0.75 | |
Start | 1000 | 43.00 | 44.00 | 43.77 | 0.23 | |
1 | 987 | 50.50 | 59.97 | 56.76 | 1.84 | |
2 | 865 | 59.53 | 65.00 | 64.29 | 0.81 | |
40% | 3 | 256 | 43.50 | 57.43 | 51.46 | 2.55 |
4 | 240 | 52.47 | 65.53 | 62.10 | 2.68 | |
5 | 227 | 59.50 | 67.00 | 66.21 | 1.22 | |
Start | 1000 | 43.53 | 44.00 | 43.76 | 0.23 | |
1 | 982 | 49.97 | 59.97 | 56.65 | 1.87 | |
2 | 849 | 60.50 | 65.00 | 64.22 | 0.81 | |
80% | 3 | 129 | 21.53 | 38.47 | 28.96 | 3.00 |
4 | 113 | 37.97 | 52.97 | 46.12 | 3.16 | |
5 | 109 | 46.97 | 64.00 | 56.20 | 3.33 | |
Before the arrests | After the arrests | |||||
---|---|---|---|---|---|---|
Compared scenarios | Group 1 Mean (SD) | Group 2 Mean (SD) | Significance | Group 1 Mean (SD) | Group 2 Mean (SD) | Significance |
0%-10% | 55.74 (6.64) | 55.70 (6.63) | n.s. | 66.75 (1.19) | 64.29 (2.70) | *** |
0%-40% | 55.74 (6.64) | 55.73 (6.66) | n.s. | 66.75 (1.19) | 56.15 (8.53) | *** |
0%-80% | 55.74 (6.64) | 55.64 (6.65) | n.s. | 66.75 (1.19) | 37.47 (13.5) | *** |
10%-40% | 55.70 (6.63) | 55.73 (6.66) | n.s. | 64.29 (2.70) | 56.15 (8.53) | *** |
10%-80% | 55.70 (6.63) | 55.64 (6.65) | n.s. | 64.29 (2.70) | 37.47 (13.5) | *** |
40%-80% | 55.73 (6.66) | 55.64 (6.65) | n.s. | 56.15 (8.53) | 37.47 (13.5) | *** |
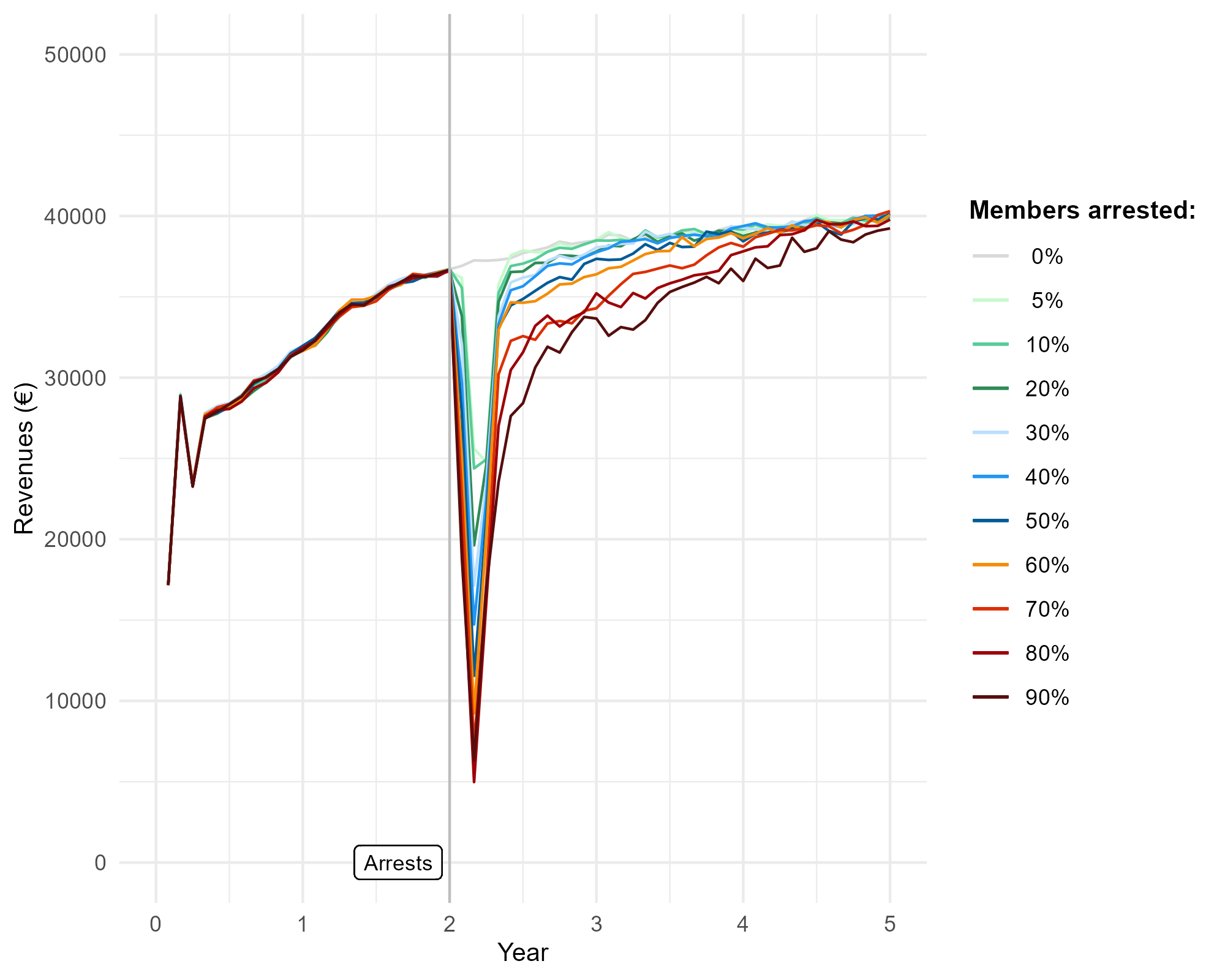
Arrest scenario | Year | Active DTOs | Min | Max | Mean | SD |
---|---|---|---|---|---|---|
Start | 1000 | 15.64 | 18.68 | 17.15 | 0.51 | |
1 | 982 | 14.43 | 42.23 | 31.72 | 4.48 | |
2 | 867 | 24.38 | 45.12 | 36.71 | 2.91 | |
0% | 3 | 801 | 26.85 | 46.68 | 38.48 | 2.88 |
4 | 767 | 28.67 | 47.3 | 39.15 | 2.85 | |
5 | 737 | 29.71 | 47.99 | 39.87 | 3.18 | |
Start | 1000 | 15.6 | 18.63 | 17.16 | 0.49 | |
1 | 982 | 16.85 | 41.78 | 31.75 | 4.6 | |
2 | 848 | 25.01 | 44.35 | 36.72 | 2.95 | |
10% | 3 | 398 | 30.28 | 44.77 | 38.49 | 2.84 |
4 | 365 | 30.2 | 46.25 | 39.16 | 3.01 | |
5 | 339 | 29.8 | 46.97 | 39.83 | 2.99 | |
Start | 1000 | 15.59 | 18.73 | 17.2 | 0.48 | |
1 | 987 | 14.12 | 40.96 | 31.71 | 4.48 | |
2 | 865 | 23.49 | 43.57 | 36.71 | 3.02 | |
40% | 3 | 256 | 26.34 | 44.63 | 37.76 | 3.5 |
4 | 240 | 31.05 | 45.42 | 39.38 | 2.91 | |
5 | 227 | 31.96 | 46.43 | 39.98 | 2.99 | |
Start | 1000 | 15.77 | 18.67 | 17.16 | 0.48 | |
1 | 982 | 12.8 | 41.32 | 31.86 | 4.58 | |
2 | 849 | 25.45 | 43.5 | 36.66 | 2.93 | |
80% | 3 | 129 | 15.58 | 46.33 | 35.22 | 5.8 |
4 | 113 | 21.54 | 44.3 | 37.81 | 3.83 | |
5 | 109 | 29.93 | 45.92 | 39.78 | 3.07 | |
Before the arrests | After the arrests | |||||
---|---|---|---|---|---|---|
Compared scenarios | Group 1 Mean (SD) | Group 2 Mean (SD) | Significance | Group 1 Mean (SD) | Group 2 Mean (SD) | Significance |
0%-10% | 31.49 (4.68) | 31.43 (4.65) | n.s. | 38.77 (0.86) | 37.87 (3.43) | * |
0%-40% | 31.49 (4.68) | 31.50 (4.68) | n.s. | 38.77 (0.86) | 37.04 (5.07) | * |
0%-80% | 31.49 (4.68) | 31.42 (4.69) | n.s. | 38.77 (0.86) | 34.30 (7.18) | *** |
10%-40% | 31.43 (4.65) | 31.50 (4.68) | n.s. | 37.87 (3.43) | 37.04 (5.07) | n.s. |
10%-80% | 31.43 (4.65) | 31.42 (4.69) | n.s. | 37.87 (3.43) | 34.30 (7.18) | *** |
40%-80% | 31.50 (4.68) | 31.42 (4.69) | n.s. | 37.04 (5.07) | 34.30 (7.18) | * |
Footnotes
- The literature provides a variety of information on the trafficking of cocaine and criminal organizations active in it. These studies focus on cocaine trafficking routes (Benítez et al. 2019; Caunic et al. 2011; Eventon & Bewley-Taylor 2016; Kenney 2007; Magliocca et al. 2019); on the financial management and profitability of cocaine markets (Hall & Antonopoulos 2017; O’Hagan & McNicholl 2015; Terenghi 2022); and on the structure and social organization of the actors involved in the activity (Calderoni 2012, 2014b; Calderoni et al. 2014; Chandra & Joba 2015; Giménez-Salinas Framis 2013; Hofmann & Gallupe 2015; Johnson et al. 1992; Morselli & Petit 2007; Natarajan 2000; Paoli et al. 2013; Reuter & Haaga 1989; Roks et al. 2021; Stevanović 2020; Damián Zaitch 2002; D. Zaitch 2002).↩︎
- The Beluga court order provides data and information to update the calibration of MADTOR for the first two simulated years. After this timeframe, model parameters are estimated following some logarithmic functions, the ones that best fitted the empirical data after the examination of several functional forms. See the online model documentation for further details.↩︎
- Randomization-based t tests are a nonparametric technique that relies on data permutation to infer significant differences among groups. It is well suited to testing differences among simulated data since it makes no assumptions about the data distribution and does not require a random sample of data from a general population. Randomization-based t tests also allow the computation of empirical p values (i.e., based on observed rather than theoretical data) (Mohr et al. 2022; Nichols & Holmes 2007).↩︎
- The share of active drug trafficking organizations out of the initial 1000 organizations is not an average of multiple values and it is meaningless to compute monthly t-tests.↩︎
- The authors performed the analyses using version 4.1.3 of R studio and the following R packages: tidyverse (Wickham et al. 2019), stringr (Wickham 2019), ggpubr (Kassambara 2020), ggplot2 (Wickham 2016), gtable (Wickham & Pedersen 2019), grid (R Core Team 2022).↩︎
- The literature reports that drug trafficking organizations may react to these events by adopting two pricing dynamics: an ‘additive model’ where changes in wholesale prices directly translate to equivalent changes in retail prices, or a ‘multiplicative model’ where the percentage increase in wholesale prices proportionally affects retail prices. Given the uncertainty in the literature about the most appropriate dynamic, and to maintain brevity, we focused a midpoint model, averaging the results of the additive and multiplicative models (Caulkins & Reuter 2010; Reuter et al. 2021). To assess how these price changes impact consumer demand, we incorporated a consumption elasticity of -0.84, implying that a 10% increase in the retail price would cause a -8.4 decrease in sold units (Payne et al. 2020). Results for the additive and multiplicative models are available upon reasonable request to the authors.↩︎
- Even in the baseline scenario some DTOs cannot maintain their criminal involvement for the whole simulated period. The failure of these organizations is linked to their ability to perform drug trafficking and dealing proficiently from an economic point of view.↩︎
- The differences in the mean number of traffickers arrested during the major law enforcement action between runs reaching the end of the simulation and those who did not were statistically significant according to two-tailed, two samples t-test performed across all arrest scenarios. The only exception was for the 10% arrest scenario. The same tests for the mean number of packagers were always statistically significant. The same tests for retailers were never statistically significant except for the 90% arrest scenario.↩︎
References
ACCONCIA, A., Immordino, G., Piccolo, S., & Rey, P. (2014). Accomplice witnesses and organized crime: Theory and evidence from Italy. The Scandinavian Journal of Economics, 116(4), 1116–1159. [doi:10.1111/sjoe.12080]
AGRESTE, S., Catanese, S., De Meo, P., Ferrara, E., & Fiumara, G. (2016). Network structure and resilience of mafia syndicates. Information Sciences, 351, 30–47. [doi:10.1016/j.ins.2016.02.027]
AXELROD, R. (1997). Advancing the art of simulation in the social sciences. Obtaining, analyzing, and sharing results of computer models. Complexity, 3(2).
AYLING, J. (2009). Criminal organizations and resilience. International Journal of Law, Crime and Justice, 37(4), 182–196. [doi:10.1016/j.ijlcj.2009.10.003]
BABOR, T. F., Caulkins, J., Fischer, B., Foxcroft, D., Humphreys, K., Medina-Mora, M. E., Obot, I., Rehm, J., Reuter, P., Room, R., Rossow, I., & Strang, J. (2018). Drug Policy and the Public Good. Oxford: Oxford University Press. [doi:10.1093/oso/9780198818014.001.0001]
BAKKER, R. M., Raab, J., & Milward, H. B. (2012). A preliminary theory of dark network resilience. Journal of Policy Analysis and Management, 31(1), 33–62. [doi:10.1002/pam.20619]
BASU, G. (2014). Concealment, corruption, and evasion: A transaction cost and case analysis of illicit supply chain activity. Journal of Transportation Security, 7(3), 209–226. [doi:10.1007/s12198-014-0140-8]
BENÍTEZ, G. J., Chandra, S., Cuadros Veloza, L. W., & Díaz Cárdenas, J. D. (2019). Following the price: Identifying cocaine trafficking networks in Colombia. Global Crime, 20(2), 90–114.
BENSON, J. S., & Decker, S. H. (2010). The organizational structure of international drug smuggling. Journal of Criminal Justice, 38(2), 130–138. [doi:10.1016/j.jcrimjus.2010.01.001]
BERLUSCONI, G. (2013). Law enforcement and criminal network resilience: The impact of law enforcement action on the structure of mafia-Related drug trafficking networks. Università Cattolica del Sacro Cuore, PhD Thesis.
BERLUSCONI, G. (2022). Come at the king, you best not miss: Criminal network adaptation after law enforcement targeting of key players. Global Crime, 23(1), 44–64. [doi:10.1080/17440572.2021.2012460]
BOSSE, T., & Gerritsen, C. (2010). Social simulation and analysis of the dynamics of criminal hot spots. Journal of Artificial Societies and Social Simulation, 13(2), 5. [doi:10.18564/jasss.1498]
BOUCHARD, M. (2007). On the resilience of illegal drug markets. Global Crime, 8(4), 325–344. [doi:10.1080/17440570701739702]
BRANCACCIO, L. (2014). Paese che vai, clan che trovi. Limes. Rivista italiana di geopolitica - Quel che resta dell’Italia
BRIGHT, D. A., & Delaney, J. J. (2013). Evolution of a drug trafficking network: Mapping changes in network structure and function across time. Global Crime, 14(2–3), 238–260. [doi:10.1080/17440572.2013.787927]
BRIGHT, D. A., Greenhill, C., Britz, T., Ritter, A., & Morselli, C. (2017). Criminal network vulnerabilities and adaptations. Global Crime, 18(4), 424–441. [doi:10.1080/17440572.2017.1377614]
BRIGHT, D. A., Greenhill, C., Reynolds, M., Ritter, A., & Morselli, C. (2015). The use of actor-Level attributes and centrality measures to identify key actors: A case study of an Australian drug trafficking network. Journal of Contemporary Criminal Justice, 31(3), 262–278. [doi:10.1177/1043986214553378]
BUTERA, F. (2009). Il Cambiamento Organizzativo: Analisi e Progettazione. Roma: Ed. Laterza.
CALDERONI, F. (2012). The structure of drug trafficking mafias: The ’Ndrangheta and cocaine. Crime, Law and Social Change, 58(3), 321–349. [doi:10.1007/s10611-012-9387-9]
CALDERONI, F. (2014a). Identifying mafia bosses from meeting attendance. In A. J. Masys (Ed.), Networks and Network Analysis for Defence and Security (pp. 27–48). Cham: Springer International Publishing. [doi:10.1007/978-3-319-04147-6_2]
CALDERONI, F. (2014b). Strategic positioning in mafia networks. In C. Morselli (Ed.), Crime and Networks, Criminology and Justice Studies (pp. 163–181). London: Routledge.
CALDERONI, F. (2019). Transnational organized crime networks. International and Transnational Crime and Justice, 175. [doi:10.1017/9781108597296.029]
CALDERONI, F., Campedelli, G. M., Székely, A., Paolucci, M., & Andrighetto, G. (2022). Recruitment into organized crime: An agent-Based approach testing the impact of different policies. Journal of Quantitative Criminology, 38(1), 197–237. [doi:10.1007/s10940-020-09489-z]
CALDERONI, F., Skillicorn, D., & Zheng, Q. (2014). Inductive discovery of criminal group structure using spectral embedding. Information & Security: An International Journal, 31, 49–66. [doi:10.11610/isij.3102]
CARLEY, K. (2006). Destabilization of covert networks. Computational & Mathematical Organization Theory, 12, 51–66. [doi:10.1007/s10588-006-7083-y]
CARPENTER, S., Walker, B., Anderies, J. M., & Abel, N. (2001). From metaphor to measurement: Resilience of what to what? Ecosystems, 4(8), 765–781. [doi:10.1007/s10021-001-0045-9]
CASTIELLO, M., Mosca, M., & Villani, S. (2017). How the resilience analysis of criminal networks may help to improve the effectiveness of public policies to contrast organized crime.
CAULKINS, J. P. (2021). Radical technological breakthroughs in drugs and drug markets: The cases of cannabis and Fentanyl. International Journal of Drug Policy, 94, 103162. [doi:10.1016/j.drugpo.2021.103162]
CAULKINS, J. P., & Reuter, P. (2010). How drug enforcement affects drug prices. Crime and Justice, 39(1), 213–271. [doi:10.1086/652386]
CAUNIC, I., Suciu, F., & Muntele, I. (2011). European Union: Destination and transit area for cocaine trafficking. Romanian Review on Political Geography, 13, 149–156.
CAVALLARO, L., Ficara, A., De Meo, P., Fiumara, G., Catanese, S., Bagdasar, O., Song, W., & Liotta, A. (2020). Disrupting resilient criminal networks through data analysis: The case of Sicilian Mafia. PLoS One, 15(8), e0236476. [doi:10.1371/journal.pone.0236476]
CHANDRA, S., & Joba, J. (2015). Transnational cocaine and heroin flow networks in Western Europe: A comparison. International Journal of Drug Policy, 26(8), 772–780. [doi:10.1016/j.drugpo.2015.04.016]
CHIU, Y. N., Leclerc, B., & Townsley, M. (2011). Crime script analysis of drug manufacturing in clandestine laboratories: Implications for prevention. British Journal of Criminology, 51(2), 355–374. [doi:10.1093/bjc/azr005]
CURTIS, R. S. (1996). The war on drugs in Brooklyn, New York: Street-Level drug markets and the tactical narcotics team. Available at: https://www.proquest.com/openview/fca8de45fac2eb2b2fe12ffd60974d9b/1?pq-origsite=gscholar&cbl=18750&diss=y.
DECKER, S. H., & Chapman, M. T. (2008). Drug Smugglers on Drug Smuggling: Lessons from the Inside. Philadelphia, PA: Temple University Press.
DESROCHES, F. J. (2005). The Crime That Pays: Drug Trafficking and Organized Crime in Canada. Toronto: Canadian Scholars’ Press.
DESROCHES, F. J. (2007). Research on upper level drug trafficking: A review. Journal of Drug Issues, 37(4), 827–844. [doi:10.1177/002204260703700405]
DIREZIONE Investigativa Antimafia. (2012). Relazione del ministero dell’Interno al parlamento sull’attività svolta e sui risultati conseguiti dalla direzione investigativa antimafia - 1° semestre 2011.
DIVIÁK, T. (2023). Structural resilience and recovery of a criminal network after disruption: A simulation study. Journal of Experimental Criminology, 2023.
DIVIÁK, T., van Nassau, C. S., Dijkstra, J. K., & Snijders, T. A. B. (2022). Dynamics and disruption: Structural and individual changes in two Dutch Jihadi networks after police interventions. Social Networks, 70, 364–374.
DRAY, A., Mazerolle, L., Perez, P., & Ritter, A. (2008). Policing Australia’s “Heroin Drought”: Using an agent-Based model to simulate alternative outcomes. Journal of Experimental Criminology, 4(3), 267–287. [doi:10.1007/s11292-008-9057-1]
DUIJN, P. A. C., Kashirin, V., & Sloot, P. M. A. (2014). The relative ineffectiveness of criminal network disruption. Scientific Reports, 4(1). [doi:10.1038/srep04238]
DUXBURY, S. W., & Haynie, D. L. (2018). Building them up, breaking them down: Topology, vendor selection patterns, and a digital drug market’s robustness to disruption. Social Networks, 52, 238–250. [doi:10.1016/j.socnet.2017.09.002]
DUXBURY, S. W., & Haynie, D. L. (2019). Criminal network security: An agent-based approach to evaluating network resilience. Criminology, 57(2), 314–342. [doi:10.1111/1745-9125.12203]
DUXBURY, S. W., & Haynie, D. L. (2020). The responsiveness of criminal networks to intentional attacks: Disrupting darknet drug trade. PLoS One, 15(9), e0238019. [doi:10.1371/journal.pone.0238019]
EKICI, B. (2014). International drug trafficking and national security of Turkey. Journal of Politics and Law, 7(2), 113.
ELSENBROICH, C. (2017). The Addio Pizzo movement: Exploring social change using agent-Based modelling. Trends in Organized Crime, 20(1–2), 120–138. [doi:10.1007/s12117-016-9288-x]
ELSENBROICH, C., & Badham, J. (2016). The extortion relationship: A computational analysis. Journal of Artificial Societies and Social Simulation, 19(4), 8. [doi:10.18564/jasss.3223]
EVENTON, R., & Bewley-Taylor, D. (2016). An overview of recent changes in cocaine trafficking routes into Europe. Background Paper for EU Drug Markets Report.
EVERTON, S. F., & Cunningham, D. (2013). Detecting significant changes in dark networks. Behavioral Sciences of Terrorism and Political Aggression, 5(2), 94–114. [doi:10.1080/19434472.2012.725225]
FABIANI, M. D., & Behlendorf, B. (2021). Cumulative disruptions: Interdependency and commitment escalation as mechanisms of illicit network failure. Global Crime, 22(1), 22–50. [doi:10.1080/17440572.2020.1806825]
FREEMAN, L. C. (1979). Centrality in social networks. Conceptual clarification. Social Networks, 1, 215–239. [doi:10.1016/0378-8733(78)90021-7]
GIMÉNEZ-SALINAS Framis, A. (2013). Illegal networks or criminal organizations. Structure, power, and facilitators in cocaine trafficking structures. In C. Morselli (Ed.), Crime and networks. London: Routledge.
GROFF, E. R. (2007). Simulation for theory testing and experimentation: An example using routine activity theory and street robbery. Journal of Quantitative Criminology, 23(2), 75–103. [doi:10.1007/s10940-006-9021-z]
GROFF, E. R., Johnson, S. D., & Thornton, A. (2019). State of the art in agent-Based modeling of urban crime: An overview. Journal of Quantitative Criminology, 35(1), 155–193. [doi:10.1007/s10940-018-9376-y]
GROFF, E. R., & Mazerolle, L. (2008). Simulated experiments and their potential role in criminology and criminal justice. Journal of Experimental Criminology, 4(3), 187–193. [doi:10.1007/s11292-008-9058-0]
HALL, A., & Antonopoulos, G. A. (2017). “Coke on Tick”: Exploring the cocaine market in the UK through the lens of financial management. Journal of Financial Crime, 24(2), 181–199. [doi:10.1108/jfc-07-2015-0037]
HOFMANN, D. C., & Gallupe, O. (2015). Leadership protection in drug-Trafficking networks. Global Crime, 16(2), 123–138. [doi:10.1080/17440572.2015.1008627]
IYAVARAKUL, T. (2021). Are drug dealers less risk averse? Evidence from micro-Level data from Thailand. NIDA Graduate School of Development Economics Working Paper Series No. 003
JOHNSON, B. D., Hamid, A., & Sanabria, H. (1992). Emerging models of crack distribution. Drugs, Crime, and Social Policy: Research, Issues, and Concerns
KASSAMBARA, A. (2020). Ggpubr: ’ggplot2’ based publication ready plots. Available at: https://CRAN.R-project.org/package=ggpubr.
KELLER, J. P., Desouza, K. C., & Lin, Y. (2010). Dismantling terrorist networks: Evaluating strategic options using agent-Based modeling. Technological Forecasting and Social Change, 77(7), 1014–1036. [doi:10.1016/j.techfore.2010.02.007]
KENNEY, M. (2007). The architecture of drug trafficking: Network forms of organisation in the Colombian cocaine trade. Global Crime, 8(3), 233–259. [doi:10.1080/17440570701507794]
KLEEMANS, E. R. (2014). Theoretical perspectives on organized crime. In L. Paoli (Ed.), Oxford Handbook of Organized Crime (pp. 32–52). Oxford: Oxford University Press. [doi:10.1093/oxfordhb/9780199730445.013.005]
KOSTELNIK, J., & Skarbek, D. (2013). The governance institutions of a drug trafficking organization. Public Choice, 156(1–2), 95–103. [doi:10.1007/s11127-012-0050-x]
LE, V. (2013). Understanding the operational structure of Southeast Asian drug trafficking groups in Australia. Queensland University of Technology, PhD Dissertation.
MAGLIOCCA, N. R., McSweeney, K., Sesnie, S. E., Tellman, E., Devine, J. A., Nielsen, E. A., Pearson, Z., & Wrathall, D. J. (2019). Modeling cocaine traffickers and counterdrug interdiction forces as a complex adaptive system. Proceedings of the National Academy of Sciences, 116(16), 7784–7792. [doi:10.1073/pnas.1812459116]
MAGLIOCCA, N. R., Price, A. N., Mitchell, P. C., Curtin, K. M., Hudnall, M., & McSweeney, K. (2022). Coupling agent-Based simulation and spatial optimization models to understand spatially complex and co-Evolutionary behavior of cocaine trafficking networks and counterdrug interdiction. IISE Transactions, 1–14. [doi:10.1080/24725854.2022.2123998]
MANZI, D. (2019). The resilience of a Camorra network under law enforcement pressure. Università Cattolica del Sacro Cuore, Milano.
MANZI, D. (2024). MADTOR: Model for assessing drug trafficking organizations resilience. Available at: https://doi.org/10.25937/XT1V-6B16.
MANZI, D., & Calderoni, F. (2024). The resilience of drug trafficking organizations: Simulating the impact of police arresting key roles. Journal of Criminal Justice, 91, 102165. [doi:10.1016/j.jcrimjus.2024.102165]
MOHR, D. L., Wilson, W. J., & Freund, R. J. (2022). Chapter 14 - Nonparametric methods. In D. L. MOHR, W. J. Wilson, & R. J. Freund (Eds.), Statistical Methods (Fourth Edition) (pp. 651–683). New York, NY: Academic Press. [doi:10.1016/b978-0-12-823043-5.00014-x]
MORSELLI, C. (2009a). Hells angels in springtime. Trends in Organized Crime, 12(2), 145–158. [doi:10.1007/s12117-009-9065-1]
MORSELLI, C. (2009b). Inside Criminal Networks. New York, NY: Springer New York.
MORSELLI, C. (2010). Assessing vulnerable and strategic positions in a criminal network. Journal of Contemporary Criminal Justice, 26(4), 382–392. [doi:10.1177/1043986210377105]
MORSELLI, C., Giguère, C., & Petit, K. (2007). The efficiency/Security trade-off in criminal networks. Social Networks, 29(1), 143–153. [doi:10.1016/j.socnet.2006.05.001]
MORSELLI, C., & Petit, K. (2007). Law-Enforcement disruption of a drug importation network. Global Crime, 8(2), 109–130. [doi:10.1080/17440570701362208]
MORSELLI, C., & Roy, J. (2008). Brokerage qualifications in ringing operations. Criminology, 46(1), 71–98. [doi:10.1111/j.1745-9125.2008.00103.x]
NARDIN, L. G., Andrighetto, G., Conte, R., Székely, A., Anzola, D., Elsenbroich, C., Lotzmann, U., Neumann, M., Punzo, V., & Troitzsch, K. G. (2016). Simulating protection rackets: A case study of the Sicilian Mafia. Autonomous Agents and Multi-Agent Systems, 30(6), 1117–1147. [doi:10.1007/s10458-016-9330-z]
NATARAJAN, M. (2000). Understanding the structure of a drug trafficking organization: A conversational analysis. Illegal Drug Markets: From Research to Prevention Policy.
NATARAJAN, M. (2006). Understanding the structure of a large heroin distribution network: A quantitative analysis of qualitative data. Journal of Quantitative Criminology, 22(2), 171–192. [doi:10.1007/s10940-006-9007-x]
NATARAJAN, M., & Belanger, M. (1998). Varieties of drug trafficking organizations: A typology of cases prosecuted in new york city. Journal of Drug Issues, 28(4), 1005–1025. [doi:10.1177/002204269802800410]
NATARAJAN, M., Zanella, M., & Yu, C. (2015). Classifying the variety of drug trafficking organizations. Journal of Drug Issues, 45(4), 409–430. [doi:10.1177/0022042615603391]
NICHOLS, K., & Holmes, A. (2007). Non-Parametric procedures. In K. Friston, J. Ashburner, S. Kiebel, T. NICHOLS, & W. Penny (Eds.), Statistical Parametric Mapping (pp. 253–272). New York, NY: Academic Press. [doi:10.1016/b978-012372560-8/50021-8]
O’HAGAN, A., & McNicholl, S. (2015). Treading the fine white line: Cocaine trafficking. Forensic Research & Criminology International Journal, 1(2). [doi:10.15406/frcij.2015.01.00009]
PAOLI, L., Greenfield, V. A., & Zoutendijk, A. (2013). The harms of cocaine trafficking: Applying a new framework for assessment. Journal of Drug Issues, 43(4), 407–436. [doi:10.1177/0022042613475614]
PAYNE, J., Fleming, C., Manning, M., & Pham, H.-T. (2020). The price elasticity of demand for illicit drugs: A systematic review. [doi:10.52922/ti04800]
POLLACK, H. A., & Reuter, P. (2014). Does tougher enforcement make drugs more expensive? Addiction, 109(12), 1959–1966. [doi:10.1111/add.12497]
PREZELJ, I., & Doerfel, M. L. (2017). Resilience in a complex and unpredictable world. Journal of Contingencies and Crisis Management, 25(3), 118–122. [doi:10.1111/1468-5973.12177]
R Core Team. (2022). R: A language and environment for statistical computing. R Foundation for Statistical Computing.
REGHEZZA-ZITT, M., Rufeat, S., Djament-Tran, G., Le Blanc, A., & Lhomme, S. (2012). What resilience is not: Uses and abuses. Cybergeo: European Journal of Geography, 2012. [doi:10.4000/cybergeo.25554]
REUTER, P. (2014). Drug market and organized crime. In L. Paoli (Ed.), Oxford Handbook of Organized Crime (pp. 32–52). Oxford: Oxford University Press.
REUTER, P., & Haaga, J. (1989). The Organization of High-Level Drug Markets: An Exploratory Study. Santa Monica, CA: Rand.
REUTER, P., & Paoli, L. (2020). How similar are modern criminal syndicates to traditional mafias? Crime and Justice, 49(1), 223–287. [doi:10.1086/708869]
REUTER, P., Pardo, B., & Taylor, J. (2021). Imagining a Fentanyl future: Some consequences of synthetic opioids replacing heroin. International Journal of Drug Policy, 94, 103086. [doi:10.1016/j.drugpo.2020.103086]
REUTER, P., Pollack, H. A., & Pardo, B. (2016). If tougher enforcement cannot reliably raise drug prices, what are appropriate goals and metrics? After the Drug Wars. Report of the LSE Expert Group on the Economics of Drug Policy.
ROKS, R. A., Bisschop, L., & Staring, R. (2021). Getting a foot in the door. Spaces of cocaine trafficking in the port of Rotterdam. Trends in Organized Crime, 24(2), 171–188. [doi:10.1007/s12117-020-09394-8]
ROMANO, D. M., Lomax, L., & Richmond, P. (2009). NARCSim: An Agent-Based Illegal Drug Market Simulation. 2009 International IEEE Consumer Electronics Society’s Games Innovations Conference. [doi:10.1109/icegic.2009.5293584]
SCAGLIONE, A. (2016). Cosa Nostra and Camorra: Illegal activities and organisational structures. Global Crime, 17(1), 60–78. [doi:10.1080/17440572.2015.1114919]
SPAPENS, T. (2010). Macro networks, collectives, and business processes: An integrated approach to organized crime. European Journal of Crime, Criminal Law and Criminal Justice, 18(2), 185–215. [doi:10.1163/157181710x12659830399653]
STEVANOVIĆ, K. (2020). Social ties between criminal networks in cocaine trafficking in Europe. Crimen, 11(3), 325–345.
SWANSTRÖM. (2007). The narcotics trade: A threat to security? National and transnational implications. Global Crime, 8(1), 1–25. [doi:10.1080/17440570601121829]
SWANSTRÖM, N. (2004). Organized crime in central asia: Its impact on Europe. Jane’s Homeland Security and Resilience Monitor, 3(3).
SZÉKELY, A., Nardin, L. G., & Andrighetto, G. (2018). Countering protection rackets using legal and social approaches: An agent-Based test. Complexity, 1–16.
TERENGHI, F. (2022). The financial management of cocaine trafficking in Italy. European Journal of Criminology, 19(6), 1501–1520. [doi:10.1177/1477370820980448]
TRIBUNALE di Napoli. (2013). Ordinanza Applicativa di Misure Cautelari Personali 30 Aprile 2013.
UNODC. (2008). Heroin and cocaine prices in Europe and USA. Available at: https://dataunodc.un.org/drugs/heroin_and_cocaine_prices_in_eu_and_usa-2017.
UNODC. (2009). Heroin and cocaine prices in Europe and USA. Available at: https://dataunodc.un.org/drugs/heroin_and_cocaine_prices_in_eu_and_usa-2017.
UNODC. (2010). Heroin and cocaine prices in Europe and USA. Available at: https://dataunodc.un.org/drugs/heroin_and_cocaine_prices_in_eu_and_usa-2017.
UNODC. (2022). World drug report 2022.
VON Lampe, K. (2012). Transnational organized crime challenges for future research. Crime, Law and Social Change, 58(2), 179–194. [doi:10.1007/s10611-012-9377-y]
WANG, N., Liu, L., & Eck, J. E. (2014). Analyzing crime displacement with a simulation approach. Environment and Planning B: Planning and Design, 41(2), 359–374. [doi:10.1068/b37120]
WEISBURD, D., Braga, A. A., Groff, E. R., & Wooditch, A. (2017). Can hot spots policing reduce crime in urban areas? an agent-Based simulation: Hot spots policing and urban area crime reduction. Criminology, 55(1), 137–173. [doi:10.1111/1745-9125.12131]
WICKHAM, H. (2016). Ggplot2: Elegant Graphics for Data Analysis. New York, NY: Springer-Verlag New York.
WICKHAM, H. (2019). Stringr: Simple, consistent wrappers for common string operations. Available at: https://CRAN.R-project.org/package=stringr. [doi:10.32614/rj-2010-012]
WICKHAM, H., Averick, M., Bryan, J., Chang, W., McGowan, L., François, R., Grolemund, G., & others. (2019). Welcome to the Tidyverse. Journal of Open Source Software, 4(43), 1686. [doi:10.21105/joss.01686]
WICKHAM, H., & Pedersen, T. L. (2019). Gtable: Arrange “Grobs” in tables. Available at: https://CRAN.R-project.org/package=gtable.
WILENSKY, U. (1999). NetLogo. Center for Connected Learning and Computer-Based Modeling, Northwestern University, Evanston, IL. Available at: http://ccl.northwestern.edu/netlogo/.
WILENSKY, U. (2021). The NetLogo 6.2.0 user manual. Available at: https://ccl.northwestern.edu/netlogo/docs/.
WILLIAMS, P. (1998). The nature of drug-Trafficking networks. Current History, 97(618), 154. [doi:10.1525/curh.1998.97.618.154]
WOOD, G. (2017). The structure and vulnerability of a drug trafficking collaboration network. Social Networks, 48, 1–9. [doi:10.1016/j.socnet.2016.07.001]
ZAITCH, D. (2002). Bosses, brokers and helpers. Labour and business relations amongst Colombian cocaine traffickers. Amsterdams Sociologisch Tijdschrift, 29(4), 502–529.
ZAITCH, D. (2002). Trafficking Cocaine: Colombian Drug Entrepreneurs in the Netherlands. Berlin Heidelberg: Springer Science & Business Media.
ZHU, H., & Wang, F. (2021). An agent-Based model for simulating urban crime with improved daily routines. Computers, Environment and Urban Systems, 89, 101680. [doi:10.1016/j.compenvurbsys.2021.101680]