[In]Credible Models – Verification, Validation & Accreditation of Agent-Based Models to Support Policy-Making

, ,
and
aDepartment of Computer Science and Media Technology, Malmö University, Sweden; bInternet of Things and People Research Center, Malmö University, Sweden.
Journal of Artificial
Societies and Social Simulation 27 (4) 4
<https://www.jasss.org/27/4/4.html>
DOI: 10.18564/jasss.5505
Received: 18-Dec-2023 Accepted: 16-Sep-2024 Published: 31-Oct-2024
Abstract
This paper explores the topic of model credibility of Agent-based Models and how they should be evaluated prior to application in policy-making. Specifically, this involves analyzing bordering literature from different fields to: (1) establish a definition of model credibility -- a measure of confidence in the model's inferential capability -- and to (2) assess how model credibility can be strengthened through Verification, Validation, and Accreditation (VV&A) prior to application, as well as through post-application evaluation. Several studies have highlighted severe shortcomings in how V&V of Agent-based Models is performed and documented, and few public administrations have an established process for model accreditation. To address the first issue, we examine the literature on model V&V and, based on this review, introduce and outline the usage of a V&V plan. To address the second issue, we take inspiration from a practical use case of model accreditation applied by a government institution to propose a framework for the accreditation of ABMs for policy-making. The paper concludes with a discussion of the risks associated with improper assessments of model credibility.Introduction
Agent-based Models (ABMs) are increasingly considered a useful tool for policy-making (Belfrage et al. 2024; Cairney 2016; Calder et al. 2018; Gilbert et al. 2018; Nespeca et al. 2023). When utilizing ABMs to underpin policy prescriptions, ensuring the model’s validity and credibility becomes crucial. This dual assurance guarantees the alignment between the model and the target system while instilling confidence in their alignment. Model credibility could be understood to be drawn from the perception that the model has been sufficiently validated for a specific application (Fossett et al. 1991). Therefore, if a model is not perceived to be credible, it is unlikely to be used by policy actors, and rightfully so, since they are ultimately accountable for the outcome (Onggo et al. 2019). It is possible that a sufficiently validated model lacks credibility or that an invalid model are perceived to be credible (Law 2022). This makes it critical to ensure that credibility assessments are conducted using rigorous procedures and appropriate information to guarantee model accuracy and suitability prior to application. Since inaccurate credibility assessments could lead to harmful outcomes or missed opportunities this evaluation could be conceptualized as a type-I and type-II error problem (Balci 1994; Schruben 1980).
The white cells in Figure 1 pose no application-related concerns, as the models are accurately identified as either suitable or unsuitable for policy-making. In the worst case scenario, models are erroneously perceived as credible and are used as decision support (type-II error), also known as the model user’s risk (Balci 1994). Edmonds & Aodha (2019) draw attention to an applied fishery model that was erroneously deemed credible, which produced inflated predictions of the cod stock’s reproductive rate. This ultimately resulted in over-fishing and the near-collapse of the cod population in the North Atlantic. On the other hand, there is a possibility that sufficiently credible models, which are capable of effectively addressing problems, are erroneously perceived to lack credibility (type-I errors), also referred to as the model builder’s risk (Balci 1994). Type-I errors represents a missed opportunity, something the ABM community should actively strive to prevent. Many publications that present ABMs motivate the importance of their contribution by stating that their models can be used as decision support. However, it is less common that articles report that their models are applied in practice (Belfrage et al. 2024). Assuming that these models are indeed sufficiently credible for use, the Agent-based Modelling community should put forth meaningful efforts to minimize type-I errors, enabling valuable societal innovation.
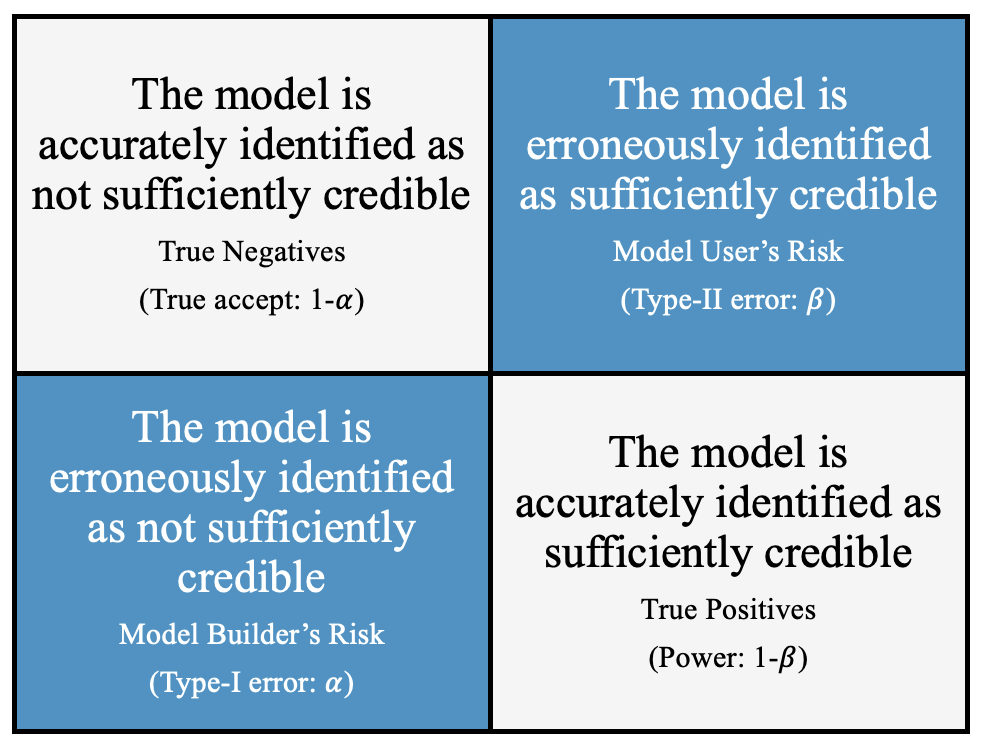
From a terminological perspective, while parts of the literature raise concerns about lacking credibility in some simulation studies (Fossett et al. 1991; Pawlikowski et al. 2002) or entire simulation approaches (Waldherr & Wijermans 2013), there remains a lack of common understanding on how credibility at the model level is determined (Onggo et al. 2019). What further compounds this issue is that the literature on the credibility of ABMs also overlaps with the literature on trust and ABMs (Harper et al. 2021; Yilmaz & Liu 2022). Trust is often considered as an all-encompassing attribute given to the model itself, the stakeholders, and the modellers to describe a wide variety of phenomena. Thus, trust is used to describe a range of different factors, from something that is perceived and attained through the demonstration of sufficient verification and validation (V&V), to the competence and intentions of modellers and stakeholders, as well as the interpersonal relationships among the involved parties (Harper et al. 2021). Accordingly, trust is occasionally used interchangeably with credibility, as well as other aspects related to simulation projects, which are influenced by a multitude of different processes. This lack of clarity is not only an impediment to effective communication but also poses a potential hindrance to future research and the application of ABMs.
From an applied perspective, recent challenges in using ABMs for policy-making highlight a lack of established procedures. Shortly after the outbreak of COVID-19, Squazzoni et al. (2020) issued a call to action, concerned about the poor rigor and transparency of ABMs and their potential to mislead policy actors. Subsequent reviews of COVID-19 models confirmed low levels of model documentation and validation (Lorig et al. 2021). Another critique of many epidemiological models used to guide COVID-19 interventions is the lack of effort in evaluating these policies after application (Winsberg et al. 2020). Other challenges of using ABMs in policy-making include collaborative issues between modellers and policy actors. Beyond the issue of credibility, these involve the understandability of technical solutions, model/project scope, project management, and political considerations (Belfrage et al. 2022). Furthermore, a review analyzing the application of ABMs in policy-making dating back to the year 2000 further reported low levels of model transparency, validation, and formal communication of findings to policy actors prior to their application. It also noted a complete absence of mentions regarding formal quality assessment procedures conducted by public administrations before model application (Belfrage et al. 2024).
With this background, two key challenges can be understood as impeding the use of ABMs as a tool for policy-making. The first challenge is terminological: defining what constitutes model credibility. The second challenge is procedural and evaluative: determining strategies for accurately assessing the credibility of models before application and evaluating their accuracy after application. Accordingly, the first part of this paper reviews literature from modelling and simulation (M&S) to delineate model credibility from related concepts. Building on these insights, the second part of the paper focuses on research and applied practices in verification, validation, and accreditation (VV&A), as well as policy and model evaluation, to design an accreditation framework. For clarity, this work does not call for a one-to-one correspondence between the model and the target system, nor does it suggest that simulated results should be applied uncritically and unequivocally in the real world. On the contrary, by institutionalizing accreditation and evaluation procedures in public administrations, our hope is that policy actors will gain a better understanding of how these insights were generated and how ABMs could applied in practice.
This paper seeks to address the following research questions:
- How can the application and evaluation of credible agent-based models for policy-making be facilitated?
- How can the concept of model credibility be understood in the context of simulation models?
- What measures can be taken in an attempt to mitigate the model builder’s risk for Agent-based Models?
- What measures can be taken in an attempt to mitigate the model user’s risk for Agent-based Models?
The next section provides an overview of verification, validation, and accreditation, clarifying the goals of related activities. It presents work from the M&S literature on credibility and provides a definition of model credibility. The third section outlines how modellers could improve documentation of Agent-based Models to mitigate risks for model builders. In the fourth section, we delve into how accreditation is applied by the US Coast Guard in practice and what insights can be elucidated from this procedure. Building on the insights attained from this procedure and the M&S literature, we propose an accreditation framework for ABMs intended to reduce the risk for model users. This is followed by a reflective discussion in section five, concerning the risks associated with applying ABMs as decision-support tools in the absence of VV&A. Lastly, we conclude by recounting some key aspects useful for modellers to consider when engaging with policy actors and provide suggestions for future work.
A Conceptual Overview of VV&A
M&S research indicates that model credibility is tightly linked to VV&A practices: verification, validation, and accreditation. Verification, validation, and accreditation are explained separately, but they are tightly interlinked; well-documented V&V processes are essential for accreditation. While V&V are commonly applied in most ABMs projects, accreditation is less established in the literature.1 Verification refers to the process of ensuring that the model is specified as intended and does not include any bugs or misspecifications. More practically this involves employing preemptive practices such as effective data management (Wickham 2016) and modular programming, but also intermediate model output testing, utilizing animation techniques and stress testing (Sargent 2010). Validity, on the other hand, can be understood as a property of inference (Cook et al. 2002). Accordingly, model validation constitute tests of varying formality that are employed to ensure that the model behaves consistently with the target system (Balci 1994).
Accreditation refers to an institutionalized process for model assessment, performed by accreditors on the behest of an organization, to ensure that a simulation model is sufficiently credible to be applied as a decision-support tool. This process assigns responsibility within the organization for how the model is applied to a given problem setting (Law 2022). In the realm of M&S, accreditation has a long history in military applications, aiding weapons acquisition, military training, and army deployment. As a result, the primary organizational focus for accreditation of computer simulations has been on military institutions such as the US Department of Defense (Fossett et al. 1991). The US Department of the Navy defines accreditation as "The official certification that a model or simulation and its associated data are acceptable to be used for a specific purpose" (DON 2019).
The relationship between the different elements of VV&A is depicted in Figure 2 diagram. Verification relates to appropriately formalizing a logical representation of the target system from a conceptual model. Since the model is not constructed directly from the target system itself but rather from interpretations of the target system and partial evidence, it must be validated to ensure alignment with the target system, thus allowing for inference (Sargent 2010). This is done by taking comparison measurements of the model and target systems to minimize the differences between the input, transformations and outputs (Balci 1994). Accreditation is then performed by accreditors who evaluate whether the model, in part or as a whole, can be generalized to the application context. Accordingly, accreditation ensures that the model can effectively guide new policy implementations within the target system (Law 2022).
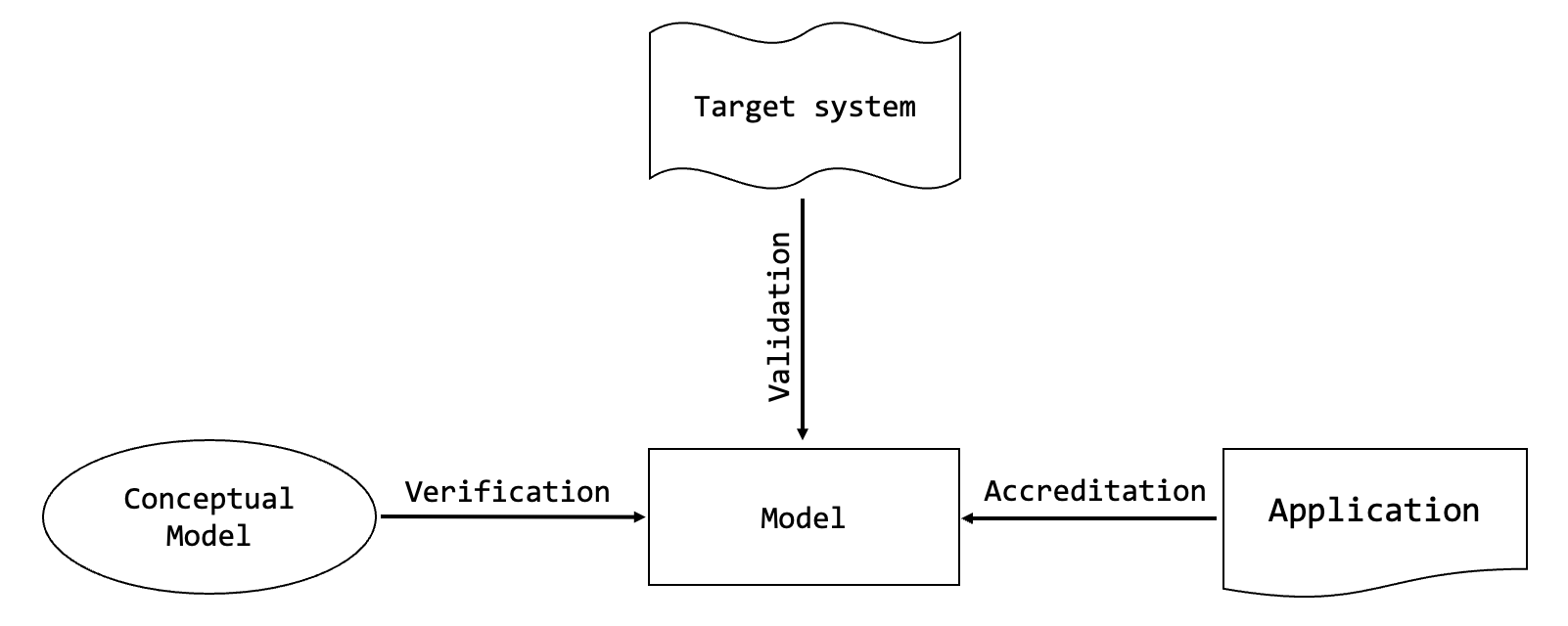
VV&A \(\rightarrow\) Model Credibility
The M&S literature indicates that there are several different factors related to VV&A which may influence model credibility. Being able to identify and evaluate these factors is essential for model assessment. However, model assessment is an expensive and time-consuming procedure. Accordingly, not all models need to be accredited, as not all models are employed in decision support. Nevertheless, if model developers anticipate their models undergoing comprehensive accreditation, it may serve as a motivating factor to implement meticulous V&V practices and maintain rigorous documentation (Fossett et al. 1991). Proper model documentation containing information about the model’s inner workings and its assumptions alongside V&V tests is often stressed to be a prerequisite for model assessment (Balci 1994; Law 2022).
Model assessment is often performed by independent actors to guarantee impartiality and increase objectivity. These actors often work at the behest of the organization seeking to employ the model for a specific purpose. This is also sometimes referred to as independent verification and validation, and is recommended for large-scale models involving multiple teams. Independent V&V can be conducted continuously during model development or once after the model is complete (Fossett et al. 1991). In the continuous approach, model development only progresses after the independent team is satisfied with the V&V at each pre-defined step (Sargent 2010). However, other post-implementation suggestions, such as Schruben (1980)’s experiment, has been proposed to increase objectivity of the evaluation. Inspired by the Turing test, the experiment tests whether domain experts can distinguish between shuffled reports derived from the target system and the simulated system.
Balci (1994) developed the framework “The Life Cycle of a Simulation”, which entails continuous credibility evaluation using different verification, validation & testing techniques during participatory approaches. Testing refers to the process of revealing model inaccuracies to be ameliorated. Balci (1994) also states that "Credibility of simulation results not only depends on model correctness, but also is significantly influenced by accurate formulation of the problem". The work outlines a taxonomy for six different categories of testing techniques ranging from informal testing, relying heavily on human reasoning e.g., Schruben’s experiment and face validation, to formal testing and providing mathematical proof of correctness. One-time testing of models is described to be a poor strategy by analogously underscoring that one final examination applied to teaching would not help students ameliorate their academic shortcomings. Rather, continuous testing facilitates back-and-forth communication to improve model deficiencies rather than making it a pass-or-fail test at the end of the project. This step-wise testing procedure should be planned by a group referred to as the Simulation Quality Assurance within the organization to ensure the model’s quality (Balci 1994).
NASA’s chapter on simulation credibility leads with a quote by Emanuel James "Jim" Rohn, stating that: "Accuracy builds credibility" (Mehta et al. 2016). According to NASA, simulation credibility is evaluated using both quantitative and qualitative methods. The qualitative evidence constitutes circumstantial aspects whereas the quantitative aspect is aimed at measuring the errors of the input, the model, and the parameters to achieve quantified simulation credibility. The acceptability of this error estimate as compared to the referent determines the overall acceptability of the model. This is linked to simulation validation and quantifying the uncertainty of the simulation and is achieved with simulation verification. This entails calculating the uncertainty of the input and the model, the referent uncertainty, and the aggregation of all uncertainties (Mehta et al. 2016).2
There is one article on the subject of accreditation and ABMs by Duong (2010). This article emphasizes that credibility of ABMs is attained through accreditation and highlights that the VV&A practices employed by the military are not entirely suitable for social systems. One reason for this is that many subject matter experts are unfamiliar with computational social science concepts, which could affect informal validation criteria such as face validation. Another issue lies in the difference that models applied for military training tend to entail a priori known processes, while many ABM aim to generate insights into unforeseen events. Additionally, ABMs are subject to high levels of uncertainty compared to physics applications due to the inherent uncertainty in social systems (Duong 2010; Edmonds 2017; Martin et al. 2016). This makes high-level resolution predictions difficult, where good social theory can often, at best, inform us about what patterns we might expect to observe. This work argues that developing better-suited criteria for social simulation applications are needed and proposes performing a train-test split procedure of data to ensure that social simulation models are not over-fitted (Duong 2010).
The M&S literature also indicates the potential for positive externalities as improving V&V on the modeller side greatly benefits VV&A on the decision-making side, and vice versa. This is because expectations of rigorous model assessment could incentivize modellers to provide more thorough V&V documentation (Fossett et al. 1991), while better V&V documentation, in turn, facilitates model assessment for organizations seeking to apply models (Law 2022). Finally, there are also challenges related to uncertainties in social systems applications e.g., stemming from bounded rationality. These uncertainties need to be taken into account when accrediting ABMs to minimize the model user’s risk. However, if policy actors are aware of the sources behind these uncertainties, this can allow for more informed decision-making (Duong 2010).
The definitions of model credibility presented in Table 1 indicate a shared understanding of this concept. Model credibility tends to be characterized according to two general patterns. Firstly, model credibility refers to the level of confidence in the accuracy of the model in relation to the target system. If stakeholders are willing to apply the model to inform a decision, the model is perceived to be credible. However, this misses a set of models, as models which are not applied as decision-support can also be perceived to be (un)credible. Secondly, NASA’s definition of model credibility is described as a quantified measure of the error and uncertainty associated with the model’s accuracy in relation to the target system. Thus, whether the definition is based on a quantitative measure or a more subjective interpretation, such as a stakeholder’s confidence, it pertains to the model’s ability to generate insights that are generalizable to the target system.
Definition | Reference |
---|---|
A simulation model and its results have credibility if the decision-maker and other key project personnel accept them as ‘correct’. | (Law 2022) |
Model credibility is reflected by the willingness of persons to base decisions on information obtained from the model. | (Schruben 1980) |
Model credibility is concerned with developing […] the confidence [stakeholders] require in order to use a model and in the information derived from that model. | (Sargent 2010) |
Model or simulation credibility is the user or decision maker’s confidence in the model or simulation, and verification, validation, and accreditation (VV&A) is the necessary way to build up model or simulation credibility. | (Liu et al. 2005) |
Credibility is the level of confidence in [a simulation’s] results. | (Fossett et al. 1991) |
The credibility of a simulation is quantified by its accuracy in terms of uncertainty. | (Mehta et al. 2016) |
Fossett et al. (1991) cuts to the core of the issue by stating: “To say that simulation results are credible implies evidence that the correspondence between the real world and simulation is reasonably satisfactory for the intended use” (Fossett et al. 1991). Accordingly, the credibility of a model is dependent upon its perceived inferential capability. This implies that the credibility of a model is closely linked to causal inference, where causal processes can be generalized across UTOS – units (agents to people), treatments (digital interventions to physical interventions), outcomes (simulated outcomes to real outcomes) and settings (in-silico to in-situ setting) – so that insights from the model can be inferred to the target system (Cook et al. 2002). This means that a sufficiently validated model implies credibility, but that this direction cannot be reversed. Accordingly, model credibility cannot exist in the absence of validity without there being insufficient information about the model.
With this in mind, we define model credibility as: a measure of confidence in the model’s inferential capability. Thus, while model validation procedures seek to maximize the alignment between the model and the target system, credibility assessment focuses on determining whether the model possesses the inferential capacity needed for a specific application. Thoroughly testing models and keeping well-documented records are crucial to establish the inferential capability and limitations of a model. Accordingly, precise credibility assessments of models hinge on thorough V&V documentation related to their usage, while ensuring accuracy to reduce the model user’s risk. Consequently, confirming that a model’s credibility is not overestimated, and preventing that the model receives any ill-gotten gains that could mislead policy actors, is of equal importance. Overconfidence in a model’s credibility effectively overestimates the model’s inferential capability, potentially leading to type-II errors and harmful outcomes. Therefore, we argue that developing VV&A practices for Agent-based Modelling is essential to advance its role as a decision-support tool.
Next, we turn to the second challenge of the paper: introducing strategies for assessing the credibility of models before application and evaluating their accuracy after application. We start by introducing the ‘V&V plan’ aimed at reducing the model builder’s risk, before moving on to motivate and explain the use of accreditation and model evaluation to reduce the model user’s risk.
The Verification and Validation Plan
This section introduces and argues for the usage of a Verification and Validation (V&V) Plan during the course of any larger modelling project, in particular those aiming to support policy-making. Similar in purpose to the type of quality assurance plan often used in software testing (Lewis & Veerapillai 2004), this document details the planned and performed V&V over the entire project. It is intended not only as an aid for modellers, but also as a window into the V&V process for the stakeholder and accreditation unit. The V&V plan is a living document that is continuously updated throughout the project to include any tests, their outcomes, and potential changes that the model undergoes.
As model validation and verification are complex processes, the creation and maintenance of the V&V plan will likely require a considerable amount of effort from the modellers. Nevertheless, there are several reasons why a project might benefit from its existence. Firstly, it requires the modellers to address the question of V&V early on in the project, rather than saving it for the end. It is crucial that verification and validation is performed continuously during the modelling process, lest major issues within the model could pass by undetected until it is too late to solve them (Balci 1994). Keeping a detailed, updated plan for the process can help ensure that all parts of the model are tested as the project progresses. Planning ahead also means that parts of the V&V process which might be time-consuming, e.g., acquiring data or getting in contact with domain experts for expert validation, will be started in time.
Secondly, the V&V plan can double as documentation of the process. It is a well-known issue that V&V documentation in ABM models is often lacking (see for instance, Balbi & Giupponi 2009; Belfrage et al. 2024; Lorig et al. 2021; Schulze et al. 2017), with many papers either skimming through vital aspects of the process or not mentioning them whatsoever. An updated V&V plan contains all relevant information and could easily be included as an appendix at publication. In this regard the plan has a similar function to the TRACE framework (Grimm et al. 2014), a standardised format for documentation and note-keeping during a modelling project, though it is concerned with the entire modelling process and not just V&V. Similar to TRACE documentation, the V&V plan could also help encourage good V&V practise, seeing as how it lays the entire process open for scrutiny.
Lastly, this document can greatly contribute to transparency and insight into the project and its V&V process for stakeholders and the accreditation unit and thus, assuming the process holds sufficient quality, increase the model’s credibility. Even if a stakeholder might not understand the technical details, it serves as evidence that the matter of evaluating and increasing the model’s validity is taken seriously. Establishing and communicating the V&V plan early also means that any questions or requests from the stakeholders can be addressed in time.
Creating a universal V&V plan that can be applied to any modelling project would be impossible. The diverse application areas of Agent-based modelling give raise to widely different models and modelling processes, and the V&V process needs to be adapted to these. Still, some general components which need to be included or addressed in the document can be identified. Though not all of these might be relevant for all Agent-based simulation studies, modellers need to be able to properly argue for a component’s irrelevancy for its exclusion to be justified. The identified components are as follows:
- Usage and adaptation of existing protocols or frameworks. Progress has been made towards developing protocols or frameworks to cover all or part of the V&V process, both with regard to Agent-based modelling in general (Barde & Der Hoog 2017; Klügl 2008; Troost et al. 2023) and to specific application areas (Azar & Menassa 2012; Drchal et al. 2016; Ronchi et al. 2016). The establishment and usage of standard V&V protocols in different domains could help structure the process; either way, their usage needs to be documented.
- Conceptual model validation. This refers to the process of ensuring that the conceptual model adequately reflects the real-world system studied (Sargent 2010). Validation of the conceptual model is ideally performed before the model is implemented, though it might have to be revisited in case the conceptual model is changed during the course of the project. In the cases where it is possible, this validation could take the form of assumptions being statistically tested or mathematically derived. It is not uncommon, however, that assumptions made during the development of ABMs cannot be quantitatively tested; in this case, qualitative approaches might be necessary to evaluate the conceptual model’s validity. Law (2022) suggest a thorough documentation and systematic walk-through of model assumptions, algorithms and data together with stakeholders and subject matter experts.
- Implemented model verification. There exists a large number of established verification techniques (Whitner & Balci 1989), as well as methods and approaches from “traditional” software testing (Basili & Selby 1987; Hutcheson 2003) that can be applied here. As noted above, verification needs to happen during the entire course of the model’s implementation, not merely at the end.
- Robustness analysis. Similar to yet distinct from conceptual model validation, robustness analysis is the investigation of to what extent results of the model stem from the model’s assumptions and implementation (Weisberg 2006). Despite being an important part of understanding a model, there exist no formalized methods for performing this analysis (Railsback & Grimm 2019), though some general approaches have been suggested (see for instance Grimm & Berger 2016; Pignotti et al. 2013). Understanding the effects of the choices and assumptions made on the model’s results is crucial in showing that the model actually reflects the studied system.
- Statistical validation. This refers to validating the model through validating its output data, using statistical methods. One of the most common way of doing this is by comparing data generated by the model with empirical data (see for instance (Windrum et al. 2007) for methods for this). It is not uncommon, however, that such an approach is not possible for an Agent-based model, be it because of the lack or inaccessibility of empirical data or a level of model abstraction which does not lend itself to real-world comparisons. Uncertainty and sensitivity analyses (Railsback & Grimm 2019; ten Broeke et al. 2016) of model results might then be more feasible.
- Model dynamics validation. There are several reasons as to why statistical validation of a model is usually insufficient. For one, as mentioned above, a shortage of empirical data often makes fit-to-data validation impossible in the first place. Secondly, levels of high uncertainty and path dependencies in models mean that output data might be insufficient in order to evaluate the model. In addition, the white-box nature and (generally) high level of descriptiveness in ABMs mean that conclusions often can be drawn not only from model outputs but its internal dynamics as well. This is especially true in models created for educational purposes or highly conceptual models where understanding the dynamics of a system is the main point of the simulation and any potentially generated data files are borderline irrelevant. It then becomes crucial to ensure that the inner workings of the model sufficiently well capture the dynamics of the simulated system and that conclusions drawn from these are valid (David et al. 2017). This typically calls for more qualitative methods than statistical validation.
- Expert involvement. Involving subject matter experts in the V&V process can be highly useful for both conceptual model validation and model dynamics validation (David et al. 2017). In order for the validation process to be transparent and rigorous, it becomes important for this collaboration to be structured and well-documented. Examples of this type of documentation could be detailed information on involved experts, interview questions or transcripts, or detailed records of what parts of the model and its results have been “approved”.
- Update logs. This refers to a record of all major revisions of the V&V plan as the project progresses. As explained above, the plan is intended to be a living document; changes are to be expected as the model is being developed and used, with new tests being called for and previously planned ones being deemed as unfeasible or irrelevant. Still, these changes need to be documented, not the least for the sake of the accreditation unit and involved stakeholders.
The Function of Accreditation in Public Administrations
Accreditation provides public administrations with procedures to ensure the credibility of models used in policy decisions, thereby enhancing accountability, transparency, and reliability in the decision-making process. The purpose of accreditation is to ensure that the model attains sufficient credibility for its application by the organization looking to use it (Liu et al. 2005). Through model assessment and the completion of the accreditation process, due diligence is carried out by the organization, enabling it to investigate how to best apply insights generated from the model and take responsibility for the outcome (Law 2022). Accordingly, accreditation can be understood as a ‘peer-review’ process for models prior to application. This means that accreditation should not be carried out by the model developers themselves but by independent evaluators, better aligning the ‘application process’ with scientific principles.
This process also enables public administrations to work more collaboratively with developers in an open and systematic manner. Democratically, the absence of institutional procedures that clearly specify how decisions are reached i.e., how models are evaluated, risks eroding accountability and transparency in the decision-making process (Schmidt 2013). Applicatively, the absence of an a priori specified process for model evaluation in public administrations could lead to less reliable evaluations and increase the model user’s risk. Providing formal reports and model documentation to public administrations enables them to independently justify the appropriateness of their decisions when applying the model. In the absence of sufficient model documentation, accountability becomes difficult for public administrations and risks putting modellers in an undesirable position, as they might be the only party capable of demonstrating the model’s credibility and justifying the appropriateness of its application (Belfrage et al. 2024). Such situations should be avoided, as accountability must remain with elected officials and the public administrations tasked with this responsibility (Knill & Tosun 2020). Additionally, prior research indicates a lack of clarity regarding the goals of collaborative simulation-based policy projects and the responsibilities of stakeholders, leading to challenges in collaboration between modellers and policy actors. Clear accreditation routines, aligning expectations between parties, could prevent these challenges (Belfrage et al. 2022).
Recognizing the importance of credible models in policy-making, public administrations are increasingly establishing routines for their application. In 2015, the UK government introduced the Aqua Book: Guidance on Producing Quality Analysis for Government to Ensure Quality Assurance. Similar to accreditation, the goal of quality assurance is to establish the credibility of models before their utilization in decision-making (HM Treasury 2015).3 Recently, the European Commission also formulated a set of questions for policy actors to consider before using a simulation model to inform policy-making (European Commission and Joint Research Centre 2023). However, military institutions, such as the US Department of Defense and its various branches, have been using computer simulation models to support decision-making since at least the 1950s (Fossett et al. 1991). Consequently, the Department of Defense has developed rigorous protocols for VV&A and has institutionalized several functions to accredit models (DON 1999). To illustrate how accreditation can be implemented in practice, we provide an overview of the US Coast Guard’s accreditation process.
The US Coast Guard’s accreditation process
The US Department of the Navy describes that VV&A is an integral part throughout a simulation project and a model’s life cycle. VV&A is used to define requirements while encompassing the model’s conceptualization, design, implementation, application, modification, and maintenance. The accreditation process is aimed at facilitating collaborative research, standardization, interoperability, acquisition, and the reuse of models (DON 2019). Older documents from the US Coast Guard (U.S. Coast Guard 2006), which draw on many of the best practices from the DOD, describe that confidence in a simulation is justified based on three criteria:
- Model assumptions are well documented and accurate;
- The M&S results are consistent, stable, and repeatable;
- That the correspondence between the model behaviour and target system is well understood.
The efforts put into the VV&A process and the implementation of M&S results should be commensurate with the importance and risk associated with a specific use-case. To ensure that these standards are met, the US Coast Guard has tasked its Chief of Staff with establishing and maintaining VV&A policies, standards, procedures, and guidelines for M&S applications. However, the accreditation process itself is described as highly flexible and is determined and approved by the Accreditation Authority based on the application’s intended use.4 The model can be accredited as a whole or with limitations. The Accreditation Authority is composed of an individual or a group within the organization, whose positions reflect the importance, risk, and impact of the application. The Chief of the Office of Performance Management and Decision Support is responsible for developing a plan to train personnel in accreditation and providing advice to the M&S Program Manager (M&S PM) and accreditation authority on the VV&A process. Additionally, there is a group of experts known as the M&S Advisory Council, which can provide recommendations to the Accreditation Authority (U.S. Coast Guard 2006).
The US Coast Guard’s accreditation process is depicted in Figure 3. First the appropriateness for applying a simulation model to the specific problem is evaluated (Identify: Problem). After this, the use for the specific simulation model is determined and documented (Design: Conceptual Model). In step 3 the acceptability criteria for accreditation is specified with its intended use in regard. Also, guidelines for future V&V activities are outlined (Specify: Requirements & Guidelines). When requirements and guidelines have been defined, the accreditor is selected – this actor is tasked with formulating an accreditation plan (Select: accreditor). The accreditation plan is then approved by the accreditation authority (Approving: Accreditation Plan). Step 6 entails receiving the final accreditation decision which is a written document describing how the simulation model can be applied (Receiving: Accreditation). The final step only applies to the continuous use of models, which requires that the a model’s maintenance is assured during the time of its deployment. This is done by defining and providing the M&S PM with modification threshold values. If these threshold values are exceeded, this will prompt the M&S PM to notify the Accreditation Authority with any potential modifications, which then initiates the process to attain new accreditation (Maintaining: Accreditation Thresholds) (U.S. Coast Guard 2006).
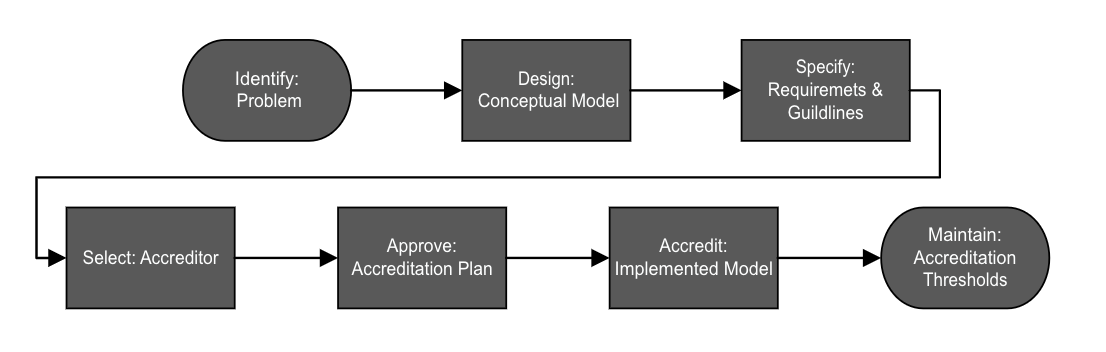
If consensus cannot be reached within this process, the issue is flagged, and the final verdict is given by the chief of staff. The VV&A process should be initiated as early as possible during the project and requires standardized documentation (DON 1999). Naturally, this also depends on whether an already existing model is used or if it is designed from scratch. The M&S PM is the principal, responsible for planning and managing the resources for the project and V&V aspects tied to maintaining model configurations of specific M&S capacities. The M&S PM retains the VV&A plans, artifacts, reports and the final accreditation decision. The M&S PM also appoints the developer and the Verification and Validation responsible (V&V responsible) and later approves the V&V Plan developed by the V&V responsible. The V&V responsible’s main task is to provide evidence demonstrating the model’s fitness and to ensure that V&V is carried out appropriately (U.S. Coast Guard 2006). The accreditor conducts accreditation assessments and is responsible for creating an accreditation plan, specifying how the model is accredited, which is then approved by the accreditation authority. The accreditor also ensures that a V&V responsible is provided with the necessary guidance about the testing required to certify the model (U.S. Coast Guard 2006).
Accreditation for agent-based models
In this section, we synthesize insights from the previous sections with specific knowledge in the field of ABMs and public administration to propose a general accreditation framework. We underscore that this framework offers a stylized depiction that can facilitate in understanding and establishing accreditation routines, but that it should not be seen as a finalized prescription in itself. In fact, the policy cycle, which is used to inform the policy actor activities in this framework, has received much criticism for over-simplifying the actual policy-making process. However, the policy-cycle is still commonly used theoretical framework for understanding and explaining policy-making in the political sciences (Cairney 2016; Jann & Wegrich 2017). Accordingly, decision-making processes may vary across organizations due to differences in governance structures or applied procedures (Cairney 2016; Colebatch 2018), and should, therefore, be tailored to the specific needs of each setting.
In the context societal outcomes generated from human interactions ABMs are among the few viable modelling options that allow users to explore counterfactual scenarios. Consequently, it is not surprising that ABMs have been frequently employed to assess potential policy interventions through in-silico analysis of COVID-19 policies. However, existing model assessment procedures appear to be tailored toward predictable systems, particularly physical ones (Duong 2010; Edmonds 2016). Social systems, on the other hand, which involve higher levels of uncertainty – partly due to bounded rationality and incomplete data – are notoriously difficult to predict outcomes for (Edmonds 2017; Martin et al. 2016). While it may not be possible to eliminate these uncertainties, which negatively affect external validity, computer simulations provide full control of inputs and environment offering stronger internal validity. This allows for the internal ranking of policies through counterfactual analysis, identifying the most to least effective policy intervention in the system under the specific set of assumptions made. However, it is the modeller’s responsibility to clearly communicate the various reasons why this ‘simulated policy ranking’ may not be valid for the target system.
To support public administrations in establishing accreditation routines, we present a general collaboration framework for accrediting ABMs, distinct from a modelling framework, illustrated in Figure 4. Given that policy evaluation serves as a prerequisite for model evaluation, this framework facilitates the dual assessment of both the implemented policy and the model. Each swim-lane represents a series of discrete actions, denoted by solid lines, carried out by three distinct actors: the modellers, the policy actors, and the accreditation unit. These actors interact with one another throughout the duration of a simulation-based policy project, as illustrated by the dashed lines connecting the swim-lanes. The different actions were defined by applying insights from the previously cited literature and the stages of the policy cycle (Cairney et al. 2019). Similarly to the US Coast Guard’s accreditation process, we assume that each stage is successfully completed. Therefore, in reality, there is more feedback between the different discrete stages than the diagram reflects.
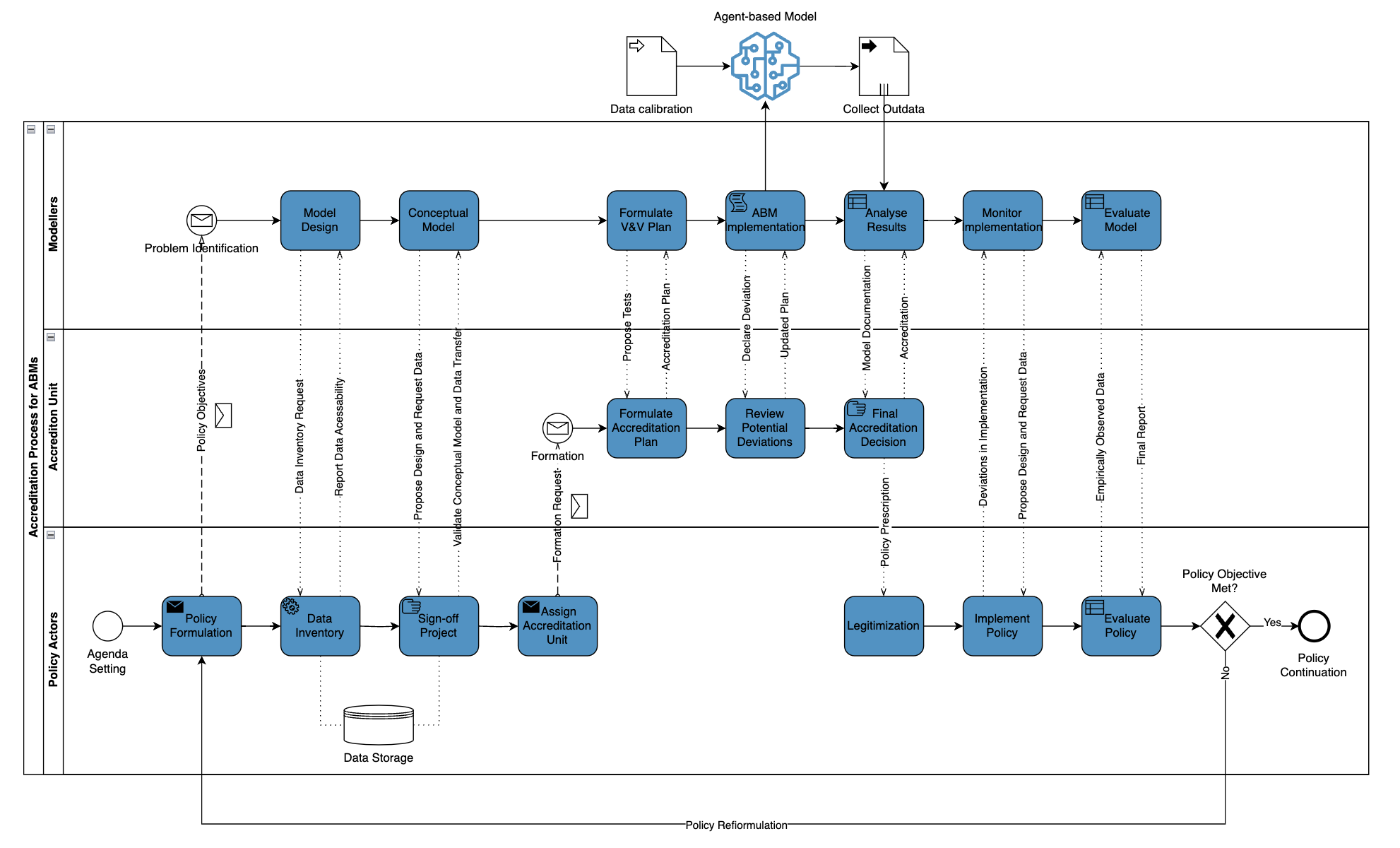
The conceptualization phase
The accreditation unit’s primary responsibility is to assess existing model information and determine what tests are necessary to establish the model’s credibility for addressing a specific problem. This is accomplished through the creation of an accreditation plan that outlines the prerequisite tests and their corresponding acceptance criteria. Upon formulating the accreditation plan, modellers can proceed to establish the V&V plan which should incorporate, at minimum, the tests mandated to achieve accreditation. While the team of modellers can propose suitable tests to the accreditation unit, the unit itself should be comprised of individuals who can judge the appropriateness of various tests based on the conceptual model. Accordingly, it is important that the personnel included in the accreditation unit have a set of competencies that not only cover expertise in ABMs, but also the application area and all other relevant aspects to the model. To increase objectivity, it is advisable that members of the accreditation unit should be distinct from the developers of the model and policy actors involved in the project. The use of independent accreditors more accurately mirrors the scientific process, which otherwise risk being bypassed prior to application, as many articles report after the fact (Belfrage et al. 2024). Accordingly, by establishing accreditation routines, independent evaluation is still carried out before application, which could serve to mitigate the model user’s risk.
The implementation phase
After the V&V plan has been established the model is implemented using domain expertise and available data for calibration. The implementation may also uncover necessary deviations from the conceptual model, which might impact the accreditation plan. Consequently, any changes made to the model should undergo review to determine if alternative or additional tests are required. Accordingly, continuous testing and frequent meetings with the collaboration parties can be useful to gather valuable feedback during the stage of model development (Balci 1994). Once the model has undergone analysis and test results have been obtained, any relevant model documentation should be submitted to the accreditation unit. Risk assessment can benefit from documenting unquantifiable sources of uncertainties as circumstantial considerations. After which, the accreditation unit can review the accumulated material and accredit a specific part of the model or as a whole. Upon obtaining results, policy actors may need to legitimize the policy prescription. While evidence-based policy-making does confer legitimacy (Cairney 2016), this may not always suffice e.g., certain solutions can be politically unfeasible (Belfrage et al. 2022).
If the ABM has already been developed and published, providing a V&V document including all model testing could facilitate the accreditation unit’s work. While this makes the accreditation unit’s work asynchronous to the modellers – impeding feedback between the two – this enables the organization to review several models comparatively which might provide additional insights (Page 2008). The testing and documentation of the V&V document should ideally be comprehensive including relevant and sufficient testing so that it in-of-itself is satisfactory to demonstrate the model’s credibility. This could allow the accreditation unit to evaluate the model’s credibility post-hoc. However, if the accreditation unit were to decide that additional tests are required, the modellers should be contacted for further testing. This process also present a valuable opportunity to evaluate models in an applied setting. The reason for this is that the model forecast is known a-priori and can be evaluated against the observed outcome of the target system (Edmonds 2017). Accordingly, applying ABMs and this routine in practice serves as an opportunity to evaluate both the policy prescription and the model itself.
The subsequent phase involves the actual implementation of the policy prescription, a purpose for which the application of ABMs holds promising potential but remains seemingly limited. Policy implementation is sometimes referred to as the missing link between policy-making and policy evaluation in the policy literature (Knill & Tosun 2020). This step is of importance since even a well-defined policy prescription in terms of content can still perform poorly if implemented improperly in practice. The implementation structure can involve numerous actors across different administrative levels, all of whom directly affect the delivery of the policy (Cairney 2016; Howlett & Lejano 2013; Turnbull 2018). This could result in differences between the simulated and observed outcomes during model evaluation. Therefore, observed differences might not be isolated to errors of the model but may also arise due to errors in the target system. Thus, data collection during policy implementation becomes valuable, and also allows policy actors to monitor the implementation process and detect any adverse effects.
The evaluation phase
The evaluation phase seeks to asses whether the pre-specified policy objectives were met after policy implementation (Knill & Tosun 2020). The COVID-19 pandemic highlighted the need for increasing evaluation of simulation informed policy interventions (Winsberg et al. 2020). One of the challenges associated with the evaluation of policy-modelling and ABS is that the evaluation must adopt a two-sided approach to be effective. This involves evaluating both the policy intervention/implementation and the policy model to identify potential sources of error and gain insights for future improvements. For example, if the policy implementation does not proceed as planned, this may result in unexpected deviations arising from within the target system. This means that it is essential to assess both model and the target system to determine where any deviations arise from. Evaluation also impacts the type of model used, as models intended for continuous use must be maintained to ensure consistent system performance (U.S. Coast Guard 2006).
The evaluation of public policy is crucial to establish whether tax-payer money is spent effectively (Knill & Tosun 2020). Naturally, this means that the organization must have completed the policy implementation step and sent all data to the modellers as this is used for model evaluation. However, evaluating applied models in different policy areas and learning from past experiences is likely crucial to the future success of ABMs as a decision-support tool (Belfrage et al. 2024). Accordingly, evaluation is not solely about measuring "how inaccurate the model was," but rather about assessing how effectively the insights gained from the model were utilized. While there are many qualitative assessments of ABMs, there are two general types of statistical validation techniques that can be performed on the outcome variables to establish the alignment between the model and the target system. These techniques are aimed at measuring the accuracy of the data generating process or the outcome (Windrum et al. 2007).
Policy evaluation of the implemented policy is subsequently conducted by calculating the policy effectiveness \((PE)\) through the subtraction of the empirically observed outcome from the policy objective \((P_{obj})\), \((PE = P_{obj} - Ce_{o})\). If the calculated policy effectiveness is equal to or greater than the policy objective \((PE \ge P_{obj})\) the policy is considered suitable for continuation. Conversely, if the calculated policy effectiveness is less than the policy objective \((PE<P_{obj})\) it suggests the possibility of discontinuation or reformulation of the implemented policy (Knill & Tosun 2020). Upon receiving the final report, encompassing all essential documentation, the public administration assumes ownership of the solution. This enables the organization to attach the final report and other relevant information to their decision guidance documents. In the event of inquiries regarding the model or its application, the organization can autonomously provide evidence justifying its decision.
Accreditation: An Example
This section provides a step-by-step example of how the accreditation framework presented in Figure 4 could be applied in a policy-making situation. We choose the COVID-19 pandemic and the question of whether schools should remain open in a city as the decision being contemplated. The reason we choose this scenario is twofold. First, during the pandemic, there were many models built with this question in mind and several were used for designing interventions, yet, the type of accreditation argued for in this paper was rarely (if ever) performed (Lorig et al. 2021). Second, the entire process of modelling for aiding policy actors find the best response to the pandemic turned out to be highly challenging, largely due to the urgency for answers and lack of information about the disease. By demonstrating that the presented framework can be adapted and use even in this situation, we thus hope to prove that accreditation is both feasible and useful in most policymaking scenarios, not only textbook ones. We save validating the framework through applying it in a real-world study for future works.
- The COVID-19 pandemic hits. Policy actors in a city are faced with the question of how the city’s schools should operate during the pandemic: should they close all schools entirely, leave them open at limited capacity, at full capacity or is there some other solution? A number of potential strategies are identified.
- As part of their gathering of policy support, the policy actors contact a group of modellers to simulate how the different suggested interventions would affect the spread of the disease. Notably this requires that the policy actors and modellers have already formed a connection beforehand (Johansson et al. 2023).
- The modellers begin to work on a conceptual model. Upon request, they receive data on the city’s schools and students, as well as more information from the policy actors about what interventions they would or would not consider.
- The modellers propose a conceptual model to the policy actors. What is suggested is a agent-based model, where agents can jump between different networks, each network corresponding to a different social context (family, work/school, friends and so on). Furthermore, each agent has a “social satisfaction” which depletes according to some rules if they do not receive sufficient social stimulation. If an agent is too lonely, they might choose not to comply with current interventions.
- The policy actors confirm that the suggested model would fill their needs. The scope and assumptions of the models are made clear. For instance, children’s learning curves are not considered, the estimates of transmission risk are still very uncertain and so on.
- The competences required for the accreditation unit are identified. It is decided that in order to be able to properly accredit the model, the unit needs to possess competence in both epidemiology, sociology or psychology, as well as modelling and simulation in general.
- An accreditation unit is formed. The policy actors reach out to one epidemiologist, one sociologist and one expert in agent-based modelling, neither of which have a close connection to the modellers. These have a meeting with both the policy actors and the modellers and are briefed on the model and its intended use.
- The unit creates the accreditation plan. This document lists the tests and information the accreditation unit needs in order to be able to evaluate the model. This includes: some proof of alignment between the model and the real world (at least logical, not necessarily numerical); uncertainty, sensitivity and robustness analysis of the model; as well as information about all assumptions made, both epidemiological and behavioral, as to be able to ensure face validity.
- After receiving the accreditation plan, the modellers form the V&V plan, outlining how the model will be verified and validated over the course of the development process. This plan includes all the required tests from the accreditation plan, information on when and how it will be performed as well as results once they have been performed. It also contains additional tests, such as common verification methods, which were not explicitly requested in the accreditation plan but still aims to assure the quality of the model.
- The model is implemented. Some simplifications need to be made in how the epidemic spread works, for computational reasons. This is communicated to the accreditation unit and the V&V plan is updated.
- The accreditation unit accredits the model. To their help, they have the V&V plan provided by the modellers. Of course, the accreditation unit cannot conclusively say that all conclusions drawn from the model will be true; this would not be possible even for the best of models given the many unknowns of the pandemic and large simplifications. However, as long as these shortcomings are communicated, the model should still be able to aid more than mislead.
- The results from the model are analyzed. From running the model, it seems that while the closure of schools would decrease the spread somewhat, it will have grave effect on the happiness of the children. If this loneliness would drive children to meet others more often in other social contexts, this would negate the benefits of closing schools. This is demonstrated to and discussed with the policy actors.
- Apart from the simulation, the policy actors have received additional decision support from other sources. After taking all available material into account, they still decide to close the city’s schools early on during the pandemic, but with plans to open them again gradually.
- The outcome of the intervention is monitored. Of course, it is near impossible to know what would have happened had a different policy been implemented instead, but it should still be possible to gain some insights in whether the chosen policy was the correct one and whether the conclusions drawn from the model were accurate.
Some details are worth extra attention in the example above. First, the accreditation unit is not trying to ascertain that the model is correct. Apart from the larger epistemological discussion of whether correctness or even accuracy can exist or be proven in a model (many argue otherwise (Ahrweiler & Gilbert 2005)), the limitations in time and data makes such a goal unfeasible. Instead, what the accreditation unit is aiming to do is to compare the model with the current state of knowledge, with state-of-the-art models and theories within epidemiology, as well as to evaluate whether the model is suited for its intended use. Second, the step of identifying what competences are needed from the accreditation unit is crucial for the model accreditation to be exhaustive. In general, the knowledge required for accrediting agent-based models can be expected to include modelling and simulation in general, the system being modelled, and human behavior within said system. Third, the purpose of the model is not to decide the best intervention, but to guide policy actors in finding it. In this case (as in most), it is reasonable to assume that there exists plenty of expertise and decision support beyond what the model provides, which will inform the final decision. Any model which does more good than harm in this guiding is a good model.
Discussion
It is often underscored that models are but abstractions of real systems and that the parameters that are included or omitted in the model can have a large influence on its results. Model credibility can be said to depend on the perceived appropriateness and correctness of these design choices in relation to a referent. This puts high demands on the model’s validation process to ensure that the model possesses the inferential capability to accurately portray the target system and its processes, thereby yielding valuable insights. However, as high system complexity tend to translate into more complicated models, these can be difficult to comprehend. This might tempt individuals to search for heuristics to infer a model’s credibility, thereby reducing cognitive workload and avoiding information overload. Accordingly, it is not a stretch to imagine that this situation could be particularly pronounced during times of crisis, such as the COVID-19 pandemic, when policy actors were under considerable pressure to act quickly.
In situations like these, established accreditation routines can provide public administrations with procedural support and help determine a model’s credibility before application, while transparently allowing them to communicate their evaluation process. Without the ability to independently assess the credibility of simulation models, one could argue that the organization lacks the institutional capability to apply models in practice. If public administrations are unable to identify insufficiently credible models, it raises the question of whether it is safe for them to apply simulation models. This consideration should certainly be recognized as fundamental for all organizations looking to use any type of software system to inform their decision-making. Furthermore, it is also crucial that scientific work adheres to established standards and undergoes independent peer-review, ensuring that modellers do not review their own work before application. Accordingly, institutionalizing accreditation routines within public administrations would help to better align the use of ABMs in policy-making with scientific principles.
The Agent-based Modelling community could play a crucial role in assisting public administrations in establishing accreditation routines, enabling them to independently make more reliable assessments of a model’s credibility before application. While establishing accreditation routines could be understood as the responsibility of public administrations, it is in the interest of both modellers and policy actors alike that they are adopted. Apart from the societal risk, there is also a risk for both parties involved when collaborations are conducted outside of predetermined procedures, as this can erode legitimacy and displace responsibility. The hard lesson learned from events like the L’Aquila earthquake is that communicating scientific evidence and uncertainty in a nuanced manner is difficult, especially in presence of strong political interests. This was demonstrated in the most unfortunate way possible in the now infamous L’Aquila trial where scientists were sentenced to prison for their involvement in crises management (Cocco et al. 2015). To avoid similar outcomes in the future, collaborations between policy actors and scientists require a shared understanding of the rules of the game, and that public administrations are able to independently and capably evaluate insights produced by models prior to their application.
Conclusions
In this paper, our motivation has been to safely facilitate the growing momentum behind the use of ABMs to help policy actors understand social systems and address societal challenges. By assisting public administrations in establishing accreditation processes for ABMs in policy-making, the ABM community could help increase societal preparedness for future crises. Towards this end, we have gathered related knowledge spanning different domains, including modelling and simulation, experimental methodology, agent-based modelling and simulation, as well as public administration and policy analysis.
These insights contributed towards shaping our understanding of model credibility as: a measure of confidence in the model’s inferential capability. Therefore, it is crucial to assess model credibility before application to understand the model’s capabilities and limitations. This means that assessments of model credibility must be based on information pertaining to the model itself, rather than that of the modellers, to avoid missing the target. The relationship between V&V and accreditation also suggests significant potential for positive externalities: improving V&V documentation greatly benefits accreditation, and the prospect of accreditation could incentivize rigorous V&V practices. By improving V&V documentation, the Agent-based Modelling community could also make a meaningful effort to reduce the model builder’s risk and occurrence of type-I errors, where models are mistakenly perceived as lacking credibility. Additionally, besides facilitating more accurate credibility assessments of Agent-based Models and increasing their practical applicability, improving V&V documentation standards could also serve to indirectly propel the advancement of V&V practices for ABMs.
Type-II errors in model credibility occur when models are erroneously perceived as sufficiently credible (model user´s risk). Historically, such errors have had disastrous effects, something which could continue in the future if meaningful actions are not taken. We conclude that this may indicate that some public administrations do not possess the technical capability to independently discriminate between insufficiently and sufficiently credible models. Beyond technical considerations, the absence of clearly specified procedures for model assessment may lead to arbitrary model evaluations and reduce the transparency of the decision-making process (Schmidt 2013). Moreover, if public administrations fail to acquire the necessary model documentation, they will likely struggle to independently justify their decisions, thus undermining accountability. Based on the current study we recommend considering the following key points when engaging with policy actors to maximize the likelihood of positive project outcomes:
- Clarify the goals of the project and explicate the responsibilities of the involved parties beforehand, so to align the expectations between Agent-based modellers and policy actors.
- Provide examples of accreditation and support public administrations in developing their own accreditation processes.
- Provide rigorous V&V testing and comprehensive model documentation that permit public administrations to independently perform credibility assessments.
- Communicate uncertainties that cannot be measured in quantifiable terms through the presentation of qualitative evidence in a contextual manner.
- Communicate model results, forecasts, and uncertainties in a nuanced way to ensure that the model’s inferential capabilities and limitations are well understood.
- Evaluate the model’s performance after application to identify explanations for any potential deviations and learn from past experiences.
While this work has relied on existing procedures, such as the accreditation procedures of the US Coast Guard, future efforts should include a use case or an evaluation to validate this accreditation framework. Accordingly, future work could involve direct engagement with policy actors to assess the feasibility of incorporating accreditation routines into policy-making processes. However, going forward, we firmly believe that establishing quality assurance practices and accrediting ABMs is preferable to continuing without them. Our hope is that increasing V&V documentation and establishing accreditation will advance methodological discussions on using ABMs as a decision-support tool.
Acknowledgements
This work was partly supported by the Wallenberg AI, Autonomous Systems and Software Program – Humanities and Society (WASP-HS) funded by the Marianne and Marcus Wallenberg Foundation and the Marcus and Amalia Wallenberg Foundation. We would like to express our gratitude to our colleagues at the Lorentz Workshop on Agent-based Simulations for Societal Resilience in Crisis Situations, held in early 2023, for their valuable feedback which helped to inform this work.
We extend our gratitude to the editor and reviewers of JASSS for their hard work and valuable feedback, which have significantly improved the quality of this article.
Notes
- A Scopus search including the terms "Agent-based Model" and "accreditation" yielded only three results. This search was conducted on 27/4-2023↩︎
- NASA uses the term coding verification to refer to the standard definition of verification.↩︎
- For an explanation of the relationship between the Aqua Book and ABMs, see Edmonds (2016).↩︎
- This text has adapted two of the terms used for two of the functions in this process. While the original text refers to the accreditor as an ‘accreditation agent’ and the V&V responsible as the ‘V&V agent,’ this literature has already reserved the term ‘agent’.↩︎
References
AHRWEILER, P., & Gilbert, N. (2005). Caffè Nero: The evaluation of social simulation. Journal of Artificial Societies and Social Simulation, 8(4), 14.
AZAR, E., & Menassa, C. C. (2012). Agent-based modeling of occupants and their impact on energy use in commercial buildings. Journal of Computing in Civil Engineering, 26(4), 506–518.
BALBI, S., & Giupponi, C. (2009). Reviewing agent-based modelling of socio-ecosystems: A methodology for the analysis of climate change adaptation and sustainability. University Ca’Foscari of Venice, Dept. of Economics Research Paper Series.
BALCI, O. (1994). Validation, verification, and testing techniques throughout the life cycle of a simulation study. Annals of Operations Research, 53, 121–173.
BARDE, S., & Der Hoog, S. van. (2017). An empirical validation protocol for large-scale agent-based models. Bielefeld Working Papers in Economics and Management.
BASILI, V. R., & Selby, R. W. (1987). Comparing the effectiveness of software testing strategies. IEEE Transactions on Software Engineering, 12, 1278–1296.
BELFRAGE, M., Lorig, F., & Davidsson, P. (2022). Making sense of collaborative challenges in agent-based modelling for policy-making. 2nd Workshop on Agent-based Modeling and Policy-Making (AMPM 2022).
BELFRAGE, M., Lorig, F., & Davidsson, P. (2024). Simulating change: A systematic literature review of agent-based models for policy-making. Conference Proceedings: 2024 Annual Modeling and Simulation Conference (ANNSIM 2024). Washington DC, USA, May 20-23, 2024.
CAIRNEY, P. (2016). The Politics of Evidence-Based Policy Making. Berlin Heidelberg: Springer.
CAIRNEY, P., Heikkila, T., & Wood, M. (2019). Making Policy in a Complex World. Cambridge: Cambridge University Press.
CALDER, M., Craig, C., Culley, D., De Cani, R., Donnelly, C. A., Douglas, R., Edmonds, B., Gascoigne, J., Gilbert, N., Hargrove, C., Hinds, D., Lane, D. C., Mitchell, D., Pavey, G., Robertson, D., Rosewell, B., Sherwin, S., Walport, M., & Wilson, A. (2018). Computational modelling for decision-making: Where, why, what, who and how. Royal Society Open Science, 5(6), 172096.
COCCO, M., Cultrera, G., Amato, A., Braun, T., Cerase, A., Margheriti, L., Bonaccorso, A., Demartin, M., De Martini, P. M., Galadini, F., Meletti, C., Nostro, C., Pacor, F., Pantosti, D., Pondrelli, S., Quareni, F., & Todesco, M. (2015). The L’Aquila trial. Geological Society, London, Special Publications, 419(1), 43–55.
COLEBATCH, H. K. (2018). The idea of policy design: Intention, process, outcome, meaning and validity. Public Policy and Administration, 33(4), 365–383.
COOK, T. D., Campbell, D. T., & Shadish, W. (2002). Experimental and quasi-Experimental designs for generalized causal inference. Boston, MA: Houghton Mifflin.
DAVID, N., Fachada, N., & Rosa, A. C. (2017). Verifying and validating simulations. In B. Edmonds & R. Meyer (Eds.), Simulating Social Complexity: A Handbook (pp. 173–204). Berlin Heidelberg: Springer.
DON. (1999). Verification, validation, and accreditation (VV&A) of models and simulations. Available at: https://www.cotf.navy.mil/wp-content/uploads/2021/01/5200_40.pdf.
DON. (2019). Department of the navy modeling, simulation, verification, validation, and accreditation management. Available at: https://www.secnav.navy.mil/doni/Directives/05000%20General%20Management%20Security%20and%20Safety%20Services/05-200%20Management%20Program%20and%20Techniques%20Services/5200.46.pdf.
DRCHAL, J., Čertickỳ, M., & Jakob, M. (2016). Data driven validation framework for multi-agent activity-based models. Multi-Agent Based Simulation XVI: International Workshop, MABS 2015, Istanbul, Turkey, May 5, 2015, Revised Selected Papers 16.
DUONG, D. (2010). Verification, validation, and accreditation (VV&A) of social simulations. Spring Simulation Interoperability Workshop, Orlando.
EDMONDS, B. (2016). Review of The Aqua Book: Guidance on producing quality analysis for government. Available at: https://www.jasss.org/19/3/reviews/7.html.
EDMONDS, B. (2017). Different modelling purposes. In B. Edmonds & R. Meyer (Eds.), Simulating Social Complexity: A Handbook (pp. 39–58). Berlin Heidelberg: Springer.
EDMONDS, B., & Aodha, L. nı́. (2019). Using agent-based modelling to inform policy - What could possibly go wrong? Multi-Agent-Based Simulation XIX: 19th International Workshop, MABS 2018, Stockholm, Sweden, July 14, 2018, Revised Selected Papers 19.
EUROPEAN Commission and Joint Research Centre. (2023). Using models for policymaking. Publications Office of the European Union. 10.2760/545843
FOSSETT, C. A., Harrison, D., Weintrob, H., & Gass, S. I. (1991). An assessment procedure for simulation models: A case study. Operations Research, 39(5), 710–723.
GILBERT, N., Ahrweiler, P., Barbrook-Johnson, P., Narasimhan, K. P., & Wilkinson, H. (2018). Computational modelling of public policy: Reflections on practice. Journal of Artificial Societies and Social Simulation, 21(1), 14.
GRIMM, V., Augusiak, J., Focks, A., Frank, B. M., Gabsi, F., Johnston, A. S., Liu, C., Martin, B. T., Meli, M., Radchuk, V., Thorbek, P., & Railsback, S. F. (2014). Towards better modelling and decision support: Documenting model development, testing, and analysis using TRACE. Ecological Modelling, 280, 129–139.
GRIMM, V., & Berger, U. (2016). Robustness analysis: Deconstructing computational models for ecological theory and applications. Ecological Modelling, 326, 162–167.
HARPER, A., Mustafee, N., & Yearworth, M. (2021). Facets of trust in simulation studies. European Journal of Operational Research, 289(1), 197–213.
HM Treasury. (2015). The Aqua Book: Guidance on producing quality analysis for government. HMGovernment, London, UK.
HOWLETT, M., & Lejano, R. P. (2013). Tales from the crypt: The rise and fall (and rebirth?) of policy design. Administration & Society, 45(3), 357–381.
HUTCHESON, M. L. (2003). Software Testing Fundamentals: Methods and Metrics. Hoboken, NJ: John Wiley & Sons.
JANN, W., & Wegrich, K. (2017). Theories of the policy cycle. In F. Fischer & G. J. Miller (Eds.), Handbook of Public Policy Analysis (pp. 69–88). London: Routledge.
JOHANSSON, E., Nespeca, V., Sirenko, M., van den Hurk, M., Thompson, J., Narasimhan, K., Belfrage, M., Giardini, F., & Melchior, A. (2023). A tale of three pandemic models: Lessons learned for engagement with policy makers before, during, and after a crisis. Review of Artificial Societies and Social Simulation.
KLÜGL, F. (2008). A validation methodology for agent-based simulations. Proceedings of the 2008 ACM Symposium on Applied Computing.
KNILL, C., & Tosun, J. (2020). Public Policy: A New Introduction. London: Bloomsbury Publishing.
LAW, A. M. (2022). How to build valid and credible simulation models. IEEE 2022 Winter Simulation Conference (WSC).
LEWIS, W. E., & Veerapillai, G. (2004). Software Testing and Continuous Quality Improvement. Boca Raton, FL: Auerbach Publications (CRC Press).
LIU, F., Yang, M., & Wang, Z. (2005). Study on simulation credibility metrics. Proceedings of the Winter Simulation Conference, 2005.
LORIG, F., Johansson, E., & Davidsson, P. (2021). Agent-based social simulation of the COVID-19 pandemic: A systematic review. Journal of Artificial Societies and Social Simulation, 24(3), 5.
MARTIN, T., Hofman, J. M., Sharma, A., Anderson, A., & Watts, D. J. (2016). Exploring limits to prediction in complex social systems. Proceedings of the 25th International Conference on World Wide Web.
MEHTA, U. B., Eklund, D. R., Romero, V. J., Pearce, J. A., & Keim, N. S. (2016). Simulation credibility: Advances in verification, validation, and uncertainty quantification. National Aeronautics and Space Administration (NASA).
NESPECA, V., Comes, T., & Brazier, F. (2023). A methodology to develop agent-Based models for policy support via qualitative inquiry. Journal of Artificial Societies and Social Simulation, 26(1), 10.
ONGGO, B. S., Yilmaz, L., Klügl, F., Terano, T., & Macal, C. M. (2019). Credible agent-based simulation - An illusion or only a step away. IEEE 2019 Winter Simulation Conference (WSC).
PAGE, S. (2008). The Difference: How the Power of Diversity Creates Better Groups, Firms, Schools, and Societies. Princeton, NJ: Princeton University Press.
PAWLIKOWSKI, K., Jeong, H.-D., & Lee, J.-S. (2002). On credibility of simulation studies of telecommunication networks. IEEE Communications Magazine, 40(1), 132–139.
PIGNOTTI, E., Polhill, G., & Edwards, P. (2013). Using provenance to analyse agent-based simulations. Proceedings of the Joint EDBT/ICDT 2013 Workshops.
RAILSBACK, S. F., & Grimm, V. (2019). Agent-Based and Individual-Based Modeling: A Practical Introduction. Princeton, NJ: Princeton University Press.
RONCHI, E., Kuligowski, E. D., Nilsson, D., Peacock, R. D., & Reneke, P. A. (2016). Assessing the verification and validation of building fire evacuation models. Fire Technology, 52, 197–219.
SARGENT, R. G. (2010). Verification and validation of simulation models. Proceedings of the IEEE 2010 Winter Simulation Conference.
SCHMIDT, V. A. (2013). Democracy and legitimacy in the european union revisited: Input, output and “throughput”. Political Studies, 61(1), 2–22.
SCHRUBEN, L. W. (1980). Establishing the credibility of simulations. Simulation, 34(3), 101–105.
SCHULZE, J., Müller, B., Groeneveld, J., & Grimm, V. (2017). Agent-based modelling of social-ecological systems: Achievements, challenges, and a way forward. Journal of Artificial Societies and Social Simulation, 20(2).
SQUAZZONI, F., Polhill, J. G., Edmonds, B., Ahrweiler, P., Antosz, P., Scholz, G., Chappin, E., Borit, M., Verhagen, H., Giardini, F., & Gilbert, N. (2020). Computational models that matter during a global pandemic outbreak: A call to action. Journal of Artificial Societies and Social Simulation, 23(2), 10.
TEN Broeke, G., van Voorn, G., & LigTENberg, A. (2016). Which sensitivity analysis method should I use for my agent-based model? Journal of Artificial Societies and Social Simulation, 19(1), 5.
TROOST, C., Huber, R., Bell, A. R., van Delden, H., Filatova, T., Le, Q. B., Lippe, M., Niamir, L., Polhill, J. G., Sun, Z., & Berger, T. (2023). How to keep it adequate: A protocol for ensuring validity in agent-based simulation. Environmental Modelling & Software, 159, 105559.
TURNBULL, N. (2018). Policy design: Its enduring appeal in a complex world and how to think it differently. Public Policy and Administration, 33(4), 357–364.
U.S. Coast Guard. (2006). Verification, validation and accreditation (VV&A) of models and simulations (M&S). Available at: https://media.defense.gov/2017/Mar/13/2001710628/-1/-1/0/CI_5200_40.PDF.
WALDHERR, A., & Wijermans, N. (2013). Communicating social simulation models to sceptical minds. Journal of Artificial Societies and Social Simulation, 16(4), 13.
WEISBERG, M. (2006). Robustness analysis. Philosophy of Science, 73(5), 730–742.
WHITNER, R. B., & Balci, O. (1989). Guidelines for selecting and using simulation model verification techniques.
WICKHAM, H. (2016). Data Analysis. Cham: Springer International Publishing.
WINDRUM, P., Fagiolo, G., & Moneta, A. (2007). Empirical validation of agent-based models: Alternatives and prospects. Journal of Artificial Societies and Social Simulation, 10(2), 8.
WINSBERG, E., Brennan, J., & Surprenant, C. W. (2020). How government leaders violated their epistemic duties during the sars-cov-2 crisis. Kennedy Institute of Ethics Journal, 30(3), 215–242.
YILMAZ, L., & Liu, B. (2022). Model credibility revisited: Concepts and considerations for appropriate trust. Journal of Simulation, 16(3), 312–325.